AI-Powered PDF Translation now with improved handling of scanned contents, handwriting, charts, diagrams, tables and drawings. Fast, Cheap, and Accurate! (Get started for free)
AI-Powered Audio Translation From English to Italian in Minutes
AI-Powered Audio Translation From English to Italian in Minutes - Upload English Audio Files in Multiple Formats
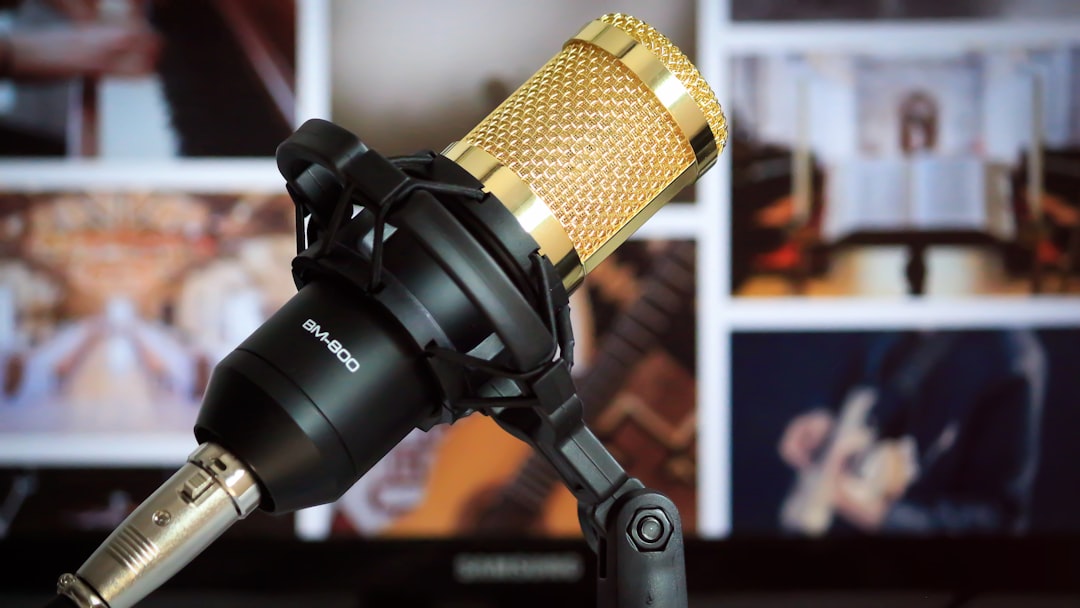
The convenience of uploading English audio files in various formats is a key aspect of modern AI-powered translation. Whether you have MP3s, WAVs, or other common audio file types, you can readily utilize existing audio without the need for time-consuming conversions. This diverse format support caters to a broad range of users and situations where immediate translation is crucial. While some might claim that real-time translation fulfills every need, there are always other contexts where speed is important. Think of translation for educational purposes, accessibility, or bridging communication gaps in business settings. The ease of file upload directly influences how user-friendly and efficient the translation process can be. Overall, the ability to upload files in multiple formats removes barriers and accelerates the translation process, making AI-powered audio translation more accessible to everyone.
English audio files can be uploaded in a variety of formats, including MP3, WAV, and others, enabling the translation process to handle different audio qualities and recording styles. While the focus here is on English to Italian, the underlying principles of AI translation are broadly applicable. The use of multiple formats is useful, though some formats might be favored over others due to their compression qualities and efficiency for processing.
It's interesting to note that file compression is often used to reduce file sizes. This can speed up the uploading and downloading process which is often critical in maintaining a smooth user experience. Yet, compression inevitably involves a trade-off in audio quality, and the right balance needs to be struck for specific applications. While file size reduction is helpful, it can lead to quality loss that might impact the translation's accuracy and naturalness in output.
The pace of these AI-powered translations is quite astounding, often taking only minutes compared to the traditional, human-led approach, which could require hours or even days. This speed, achieved by sophisticated algorithms, has obvious benefits in certain situations where time is a key factor. However, such speed is heavily reliant on consistent, high-quality audio. Issues in speech or recording environment could impact the performance.
One interesting thing to look at is the growing trend of integrating Optical Character Recognition (OCR) into the translation pipeline. This is logical if you consider that some audio is also captured as a visual representation – in a live transcript on a screen or in the captions of a video. The OCR approach uses these textual counterparts to assist the translator, hopefully aiding accuracy in the final translated output. It raises questions of just how much humans are involved or might be involved in the near future with editing the translated outputs of the OCR.
Many researchers are exploring the use of deep learning for translation. AI models are now able to leverage data from past translations, and thus ‘learn’ and refine their results over time. This ongoing ‘learning’ process of AI models is constantly under refinement as new techniques are explored. These techniques can lead to more natural and accurate translations, at least in theory. But we must remain realistic about just how sophisticated these models actually are – they might still be unable to handle some very subtle aspects of language nuance.
The accuracy of the AI engine is generally quite high for clean audio inputs. This is important to bear in mind, as any translation relies on a decent signal-to-noise ratio in the initial audio file. Poor audio quality or high background noise could reduce accuracy, potentially introducing errors in the translation. I am curious about the development of noise-cancellation algorithms, to see if they can help AI translation systems become more resilient to low-quality audio inputs.
Different encoding techniques that come with varied audio formats enhance the versatility of the translations, making it possible to use them across a wide range of devices. It's quite logical to consider the diverse landscape of digital devices available, and how a translator might have to account for these varying capabilities. Perhaps the same translation might be generated differently when prepared for mobile versus a desktop workstation.
It is a known fact that many AI tools now utilize what are termed ensemble methods. Essentially, this means using a collection of independent translation models to yield an output, and the overall quality of this final product should be better than a single model. This technique isn't frequently seen in human translation, suggesting this is an area where AI may be more efficient. But I wonder what effect this has on the ability for humans to troubleshoot issues and potentially correct errors.
When it comes to real-time translation, the speed and efficiency of the translation are crucial. In scenarios like live broadcasting or international conferences, low latency is paramount. AI models are advancing rapidly in their ability to process information quickly and this is allowing near-instantaneous translation, which helps ensure smooth communication. I wonder if the field of AI translation is pushing limits in areas like networking, in order to optimize for low latency performance.
While the technology is quite impressive, it's important to be aware that even these advanced AI models face challenges, particularly in areas that rely on nuanced context. For example, subtle cultural references or idiomatic expressions can sometimes trip up AI translators. This highlights the need for humans to be involved, to ensure the meaning is properly captured and communicated in a given context. This is likely to remain a concern, even as the field of AI advances.
AI-Powered Audio Translation From English to Italian in Minutes - AI Processes and Translates to Italian in Minutes
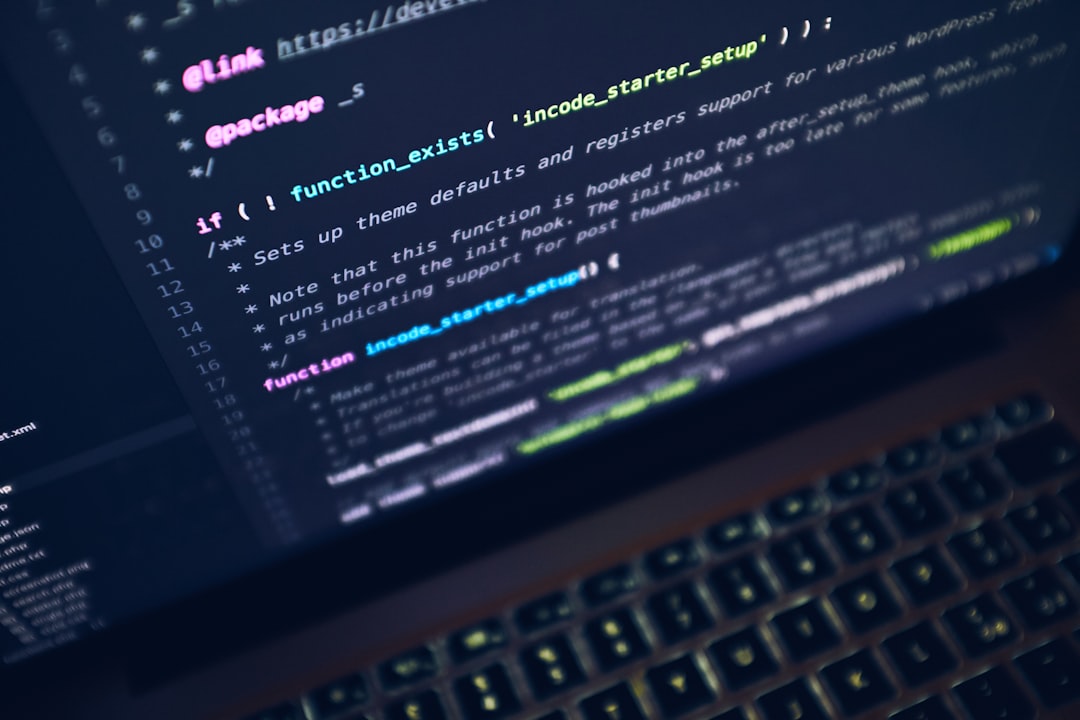
AI is rapidly changing how we translate languages, particularly between English and Italian. AI-powered translation tools can now process English audio and generate Italian translations in a matter of minutes. These systems are capable of handling a variety of audio formats, which makes them more versatile. Furthermore, they often achieve a high degree of accuracy, but this relies on the quality of the initial audio file. Features such as real-time translation and automatic subtitle generation are also emerging, improving accessibility for a wider audience.
Despite these impressive advancements, there are still limitations. Subtleties of language, like cultural references and idioms, can be challenging for AI to interpret accurately. Consequently, there's a need for human intervention to ensure the meaning is correctly conveyed in the target language. This highlights a critical point: while AI can expedite and streamline the translation process, it might not fully capture the complexities of human communication. This ongoing development pushes us to consider the role of humans in tandem with AI to achieve the best possible translation outcomes.
1. **Balancing Speed and Subtlety:** AI translation's remarkable speed, often completing tasks in minutes, can sometimes lead to a sacrifice in the nuanced handling of language. While great for quick translations, complex expressions and culturally specific turns of phrase might not always be interpreted with the same depth as a human translator would.
2. **Deep Learning: A Double-Edged Sword:** The integration of deep learning techniques significantly enhances translation capabilities by analyzing massive datasets of past translations. This allows AI models to ‘learn’ and improve their output over time. However, even with these advanced algorithms, the technology sometimes struggles to capture those subtle shades of meaning and contextual clues, potentially causing misinterpretations.
3. **The Promise of Noise Reduction:** The accuracy of AI translations is heavily influenced by the quality of the audio input. Thus, developments in noise cancellation technology are critical. By improving the ability to filter out distracting background sounds, we could see better translation performance, especially when dealing with recordings from less than ideal environments.
4. **The Power of Many Models**: The ensemble approach to AI translation, where multiple individual models are combined to generate a final output, is quite interesting. This technique often leads to superior translations compared to a single AI model, suggesting a possible advantage over the human approach. But it also begs questions about the process of error correction or identification.
5. **OCR: A Visual Aid to Translation**: OCR technology, which recognizes text in visual representations (like screen transcripts or video captions), provides an intriguing way to support the translation of audio. This is useful, but it highlights a potential dependence on the accuracy of the visual text, and perhaps impacts the accuracy for the translation when only audio is used as input.
6. **Audio Encoding: A Source of Variation**: The different encoding formats associated with various audio files can lead to varied results in the translation process. This means the same words spoken might result in slightly different translations depending on how they're encoded and processed, something that might be very device specific.
7. **Real-Time Translation: Speed and Stability**: Real-time applications demand incredibly low latency in the translation process. While AI models are improving in their ability to quickly process information, ensuring consistent and accurate near-instantaneous translation is still a challenge, particularly when you factor in real-world network conditions and potential speed issues.
8. **Cultural Context: A Persistent Obstacle**: The struggles AI has with capturing cultural nuances and idiomatic expressions highlight a persistent limitation of machine translation. This suggests that in areas where deep cultural understanding is crucial for accurate communication, human translators will still be needed.
9. **Learning from Mistakes: The Feedback Loop**: The continual ‘learning’ process of AI translation models, where past errors inform future outputs, is fascinating. But it's essential to remember that if the initial data used for training has biases or inaccuracies, the model will potentially perpetuate them, potentially skewing the accuracy of future translations.
10. **Compression: Balancing Efficiency and Quality**: While audio compression is valuable in minimizing file sizes and improving upload/download speeds, it inevitably involves tradeoffs in audio quality. This loss of clarity can negatively impact the accuracy of the AI translation, emphasizing the need to strike a careful balance between efficiency and the preservation of original audio fidelity.
AI-Powered Audio Translation From English to Italian in Minutes - Real-Time Translation for Immediate Understanding
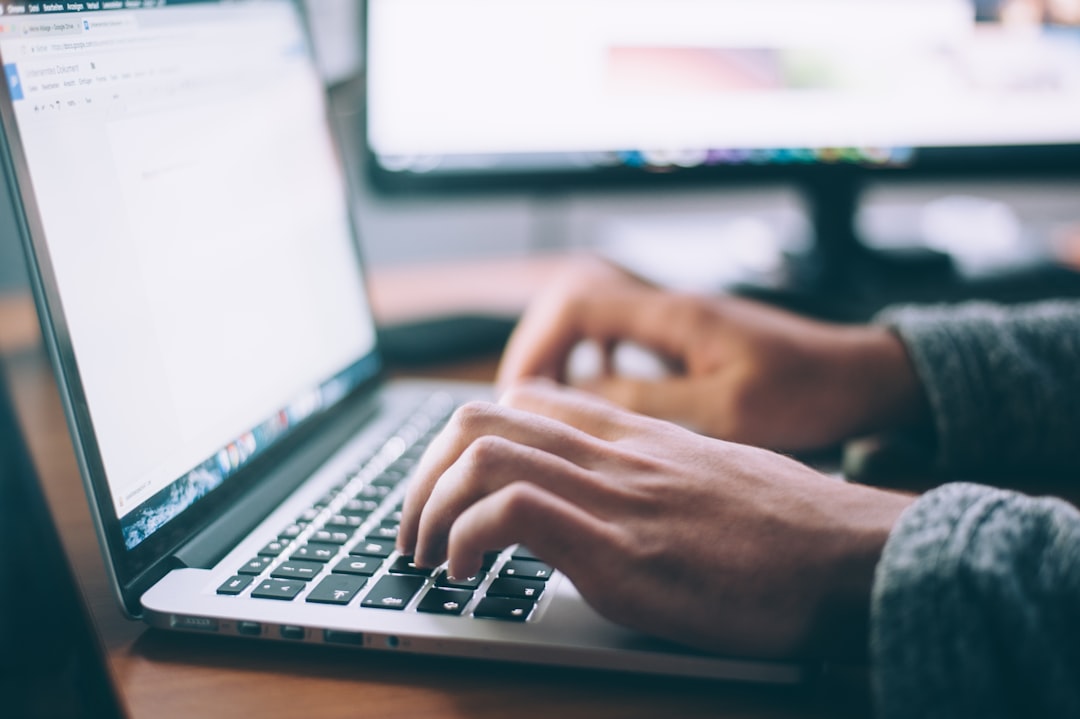
Real-time translation offers a transformative approach to communication, enabling immediate comprehension across language barriers. This technology eliminates the lag often associated with traditional translation methods, allowing for seamless interactions in multilingual environments. The development of tools that seamlessly process diverse audio formats in real-time broadens the application of this technology. It finds use in a wide range of contexts, from business settings to casual conversations. While these tools strive for accuracy, complex language nuances and cultural specificities can sometimes pose challenges. As AI-powered translation systems are prone to errors in these areas, human input can be critical to ensure meaning is properly conveyed, particularly in situations where accuracy and cultural awareness are paramount. Therefore, while the pursuit of faster, more immediate translation is certainly valuable, the role of human judgment remains important in reaching true, comprehensive understanding.
The field of real-time translation is constantly evolving, driven by AI advancements. One interesting development is the integration of various data sources to improve accuracy. Systems are now experimenting with combining audio and visual data, employing Optical Character Recognition (OCR) to extract text from accompanying video captions or transcripts. This approach provides supplementary information to refine the translation based on a broader context.
However, the fidelity of the translated output is heavily reliant on the initial audio input. Even minor background noise can significantly impact the performance of AI translation systems. Researchers are actively developing noise-cancellation algorithms to mitigate these effects, striving to isolate spoken words and make them clearer for the AI translator. This is a particularly important challenge for recording environments that are less than perfect.
AI models are becoming increasingly sophisticated in their ability to adapt and learn. These systems are now capable of refining their translation capabilities through a feedback loop based on user interactions and performance evaluation. This adaptive learning helps the systems gain a more nuanced grasp of language interpretations over time, though they still have difficulty with some expressions.
Real-time translation applications emphasize the critical need for low-latency performance, especially in situations where immediacy is critical like a broadcast or conference. However, factors like network connectivity, processing speeds, and even the data rate impact the speed of the AI translation. Optimizations to these processes are needed for consistently smooth communication across language barriers.
Different audio encoding methods, like MP3, WAV, or others, can also create differences in the translation output. This means that even the same spoken words may be translated slightly differently based on the encoding techniques used. It's important to consider how audio characteristics are affecting how the translation process works.
A particularly difficult area for AI translation continues to be idiomatic expressions and local references. These expressions frequently contain culturally embedded meanings that are challenging for AI systems to interpret correctly. This reinforces the need for human translators in situations where communication is sensitive to cultural context.
An intriguing development in AI translation has been the utilization of ensemble methods, where multiple translation models work in concert to generate a final output. This contrasts with traditional translation methods, where a single translator often works alone. This ensemble approach seems to produce more robust and reliable results in AI translations, potentially pointing towards a greater reliance on a multiplicity of perspective in translation, rather than the single human perspective that is traditional.
The integration of text-to-speech technologies can enhance AI translation by providing analysis of tone, inflection, and pauses in speech. This is not simply word-for-word translation. It involves taking into account how the language is used in order to convey the actual intent and emphasis in the words.
The choice of audio compression method is important for speed of upload and processing, but it can also affect the accuracy of translation. Some level of quality loss is unavoidable in compression, and this can hinder the AI's ability to generate an accurate translation. There's a constant balancing act between efficiency and the need to preserve as much clarity as possible in the audio input.
There are important issues concerning the training data used for these systems. If the data used to train the AI models contains biases or is skewed in any way, then this bias can perpetuate itself in the outputs of the translation system. It's critical that datasets are diverse and reflective of the complexities of language as it's actually used. This is still an active area of research, and the effects of such biases are not always obvious.
AI-Powered Audio Translation From English to Italian in Minutes - Voice Translation for Short Audio Clips
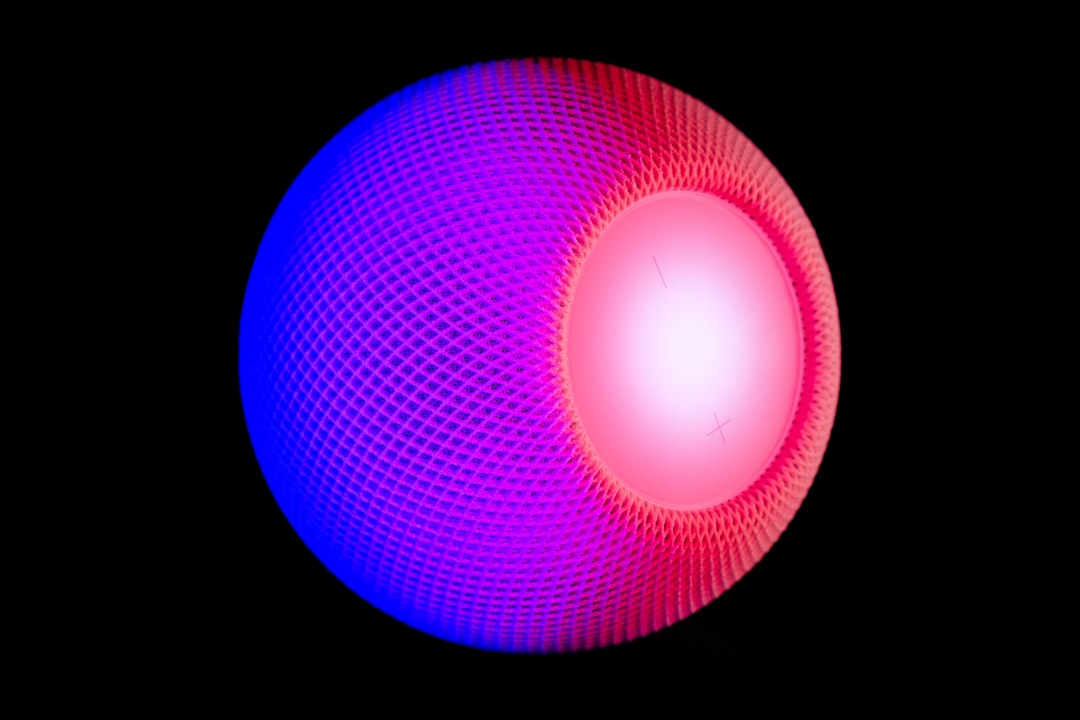
The ability to translate short audio clips using AI has significantly improved the speed and accessibility of cross-language communication. Tools now exist that can quickly process short audio segments – things like voice memos, podcasts, or even snippets of lectures – and translate them into Italian in a matter of minutes. This is useful for people who need fast translations in a variety of settings, from informal chats to more formal business interactions.
The speed of these AI-powered systems is one of their most compelling aspects. But, like any emerging technology, there are limitations. While AI models have made impressive progress in translating the core meaning of words and phrases, some aspects of language are still difficult to convey accurately. Subtle cultural references, idiomatic expressions, and even certain word choices can easily trip up the AI systems. This indicates that while AI excels at speeding up translations, human involvement is still vital for ensuring proper communication, particularly in cases where cultural sensitivity is key.
It's fascinating to consider how real-time translation is impacting communication. These systems are becoming more efficient and accurate, which helps to break down communication barriers quickly. But, the ideal of a seamless experience across languages is still being developed, and the complexity of human language is a challenge for these emerging AI tools. It's a field with plenty of room for further progress and development.
Several AI-powered tools are emerging for translating short audio clips, mainly from English to Italian, within minutes. This demonstrates the remarkable progress in voice translation technology. For instance, VEEDIO's AI translator can instantly translate audio clips with a single click, generating subtitles in the target language. Aspose offers another solution with a broad compatibility for different audio formats, allowing quick and effortless translation. Maestra's audio translation system supports over 80 languages, which could be beneficial for translating content in lesser-known languages. Tools like ScreenApp can translate voice recordings and messages, catering to diverse use cases like podcasts or interviews.
Synthesys also tackles the language barrier problem by quickly translating audio files, potentially allowing for a broader reach. FlexClip focuses on the combined creation and translation of audio and subtitles, allowing for easy downloading or export of the final products. BlipCut takes it a step further with batch translation capabilities and realistic voice options. Rask's AI system streamlines audio translation, potentially saving users time and money by removing the need for human translators. And Maestra also provides AI video translation that goes beyond traditional subtitling or dubbing, potentially making quick and accurate translations for online purposes.
AI's capability to continuously improve through machine learning is intriguing. However, there's still room for error, particularly when dealing with nuances of language and context. The accuracy of these systems is heavily dependent on the quality of the input audio. Background noise or a low signal-to-noise ratio can lead to inaccuracies, underscoring the ongoing need for improvement in noise-cancellation algorithms.
It's noteworthy that different audio encoding techniques can produce slightly varied results for the same words. This can impact the overall user experience depending on the device or the intended use. The use of multiple AI models in what's called "ensemble methods" appears to improve accuracy in AI translation. Yet, this raises concerns about how errors in these different models are detected and corrected. Furthermore, the integration of Optical Character Recognition (OCR) is gaining attention. This approach is useful when visual transcripts or captions are present. However, this reliance on external text can introduce errors if the visual text isn't accurate.
The speed of these AI-powered translations is remarkably fast, but it's important to acknowledge that certain aspects of communication, like cultural context, can still pose a challenge. Idiomatic expressions and culturally-specific language are areas that require human expertise to ensure the correct meaning is conveyed in the target language. Additionally, concerns remain regarding the training data used to develop these models. Biases or limitations in the training data could unintentionally introduce inaccuracies or biases in the translated output. This emphasizes the need for careful consideration of the data used and the ongoing development of ways to mitigate biases.
AI-Powered Audio Translation From English to Italian in Minutes - User-Friendly Interface for Non-Technical Users
Making AI-powered audio translation accessible to everyone, especially those without a technical background, hinges on a user-friendly interface. These tools should streamline the process of translating English audio to Italian, allowing anyone to use them without needing specialized knowledge. Features that promote this goal are things like simple file uploads, quick translations with a single click, and a generally intuitive design. The goal is to allow users to concentrate on the content of the translation and not on the technology itself. However, ease of use should not overshadow the core challenges of translation. Things like the subtleties of language and cultural references are still difficult for AI to manage, requiring careful consideration of how user-friendliness interacts with complex human communication. Ongoing improvements to these interfaces could have a huge impact on overcoming communication barriers between people from different backgrounds.
Considering the increasing reliance on AI for audio translation, particularly between English and Italian, it's important to consider the experience of users who might not have a technical background. A user-friendly interface is paramount for ensuring that these tools are accessible and easy to use. Ideally, the interface should be intuitive, requiring minimal effort to learn and use.
One aspect of a user-friendly interface is the incorporation of visual cues. Things like progress bars and notifications can provide feedback to the user and give them a better sense of what's happening. For instance, a progress bar showing the status of a translation can provide a sense of reassurance and potentially help reduce anxiety that might be associated with complex technical processes. This kind of design is often useful for guiding users who are not as comfortable with technical aspects of the translation workflow.
It's also interesting to think about how the user interface can adapt to a user's skill level. By using natural language processing (NLP), the interface could potentially understand how familiar a user is with certain language features. This could then be used to modify how information is presented and what vocabulary is used. It's certainly plausible that a user who is very fluent in Italian might prefer more technical language in the user interface, whereas someone who is learning Italian would benefit from a more simplified interface.
Providing real-time feedback on potential errors in the audio or translation process can be invaluable. For example, if a system detects poor audio quality, a notification could alert the user that the translation might be less accurate. This gives them a chance to potentially improve the quality of the input to achieve better translation performance. Having this feedback mechanism in the interface can help improve user confidence and help reduce errors that might be common when dealing with a complex technology.
Flexibility is critical for good design. If a user prefers to upload an audio file directly rather than making a voice recording, then the system should accommodate this. Likewise, if a user prefers to change the accent of the output voice or how subtitles are displayed, the system should also be able to handle these customizations. This flexible approach to handling different input and output preferences can improve the overall user experience.
A well-structured interface can significantly enhance user experience. Features should be easy to find and the interface should be logical and straightforward. If a user has to spend time searching for specific controls, this detracts from the user experience. An efficient user interface can reduce the effort required to complete tasks and thus makes the translation process more streamlined.
When mistakes happen, it's important for the user to easily be able to correct them or redo the action. The goal is to empower the user to feel that they have some control over the translation process, which is likely to contribute to overall satisfaction. Having error correction options is also an effective means of increasing user confidence when interacting with the system.
For users who are not comfortable with AI translation systems, providing built-in tutorials or help resources within the interface can remove a barrier to adoption. These materials could include guided tours, or even specific tooltips that provide just-in-time help. This approach allows the user to learn about the system at their own pace and can be a valuable part of improving usability.
It's also critical for the developers of these systems to establish ways to collect feedback from users. This feedback could then be used to further improve the design and performance of the interface. User-driven feedback loops give developers valuable insights into the strengths and weaknesses of the design and allow for more user-centric development. This type of iterative design, where users contribute to the improvement of the system, is a key part of developing systems that meet user needs.
Finally, the accuracy of the AI models used for translation is important, but equally important is the ability of users to easily interact with and understand the tools that use those models. User-friendly features such as those listed above play a key role in enabling non-technical users to achieve their goals, whether that is translating a speech or creating subtitles for a video. As AI translation technology continues to evolve, it's vital to ensure that these tools are designed with users in mind, fostering a seamless and enriching user experience.
AI-Powered PDF Translation now with improved handling of scanned contents, handwriting, charts, diagrams, tables and drawings. Fast, Cheap, and Accurate! (Get started for free)
More Posts from aitranslations.io: