AI-Powered PDF Translation now with improved handling of scanned contents, handwriting, charts, diagrams, tables and drawings. Fast, Cheap, and Accurate! (Get started for free)
Japanese-to-English AI Translation Accuracy Analysis of 7 Regional Dialects and Their Unique Translation Challenges
Japanese-to-English AI Translation Accuracy Analysis of 7 Regional Dialects and Their Unique Translation Challenges - Tokyo Dialect Machine Translation Shows 89% Accuracy in Technical Documents During 2024 Tests
Recent tests conducted in 2024 showcased a notable leap in AI-powered translation of the Tokyo dialect, particularly for technical documents. The systems demonstrated an 89% accuracy rate when translating these materials into English. This success is a clear indication of how AI translation technology is evolving to meet the increasing demand for fast and efficient translation, especially in specialized areas. However, while impressive, the inherent intricacies of language still pose a hurdle. Even with these advances, there remains a need for human translators to ensure accurate interpretation of subtle meanings and context that might elude AI algorithms. This is particularly evident in the inconsistency of accuracy seen within a single document, where some sections might achieve perfect scores while others struggle. The reality is, for optimal translation, especially in complex technical fields, a partnership between human translators and AI is likely to remain essential for the foreseeable future.
During evaluations conducted throughout 2024, machine translation models specifically trained on the Tokyo dialect demonstrated a remarkable 89% accuracy when translating technical documents into English. This finding highlights a crucial point: the nuances inherent in regional dialects significantly impact the performance of AI translation systems. It suggests that developing dialect-specific models is essential for achieving optimal results, particularly in fields like engineering and technology.
It seems that the rise of AI translation, spurred by the need for faster and potentially cheaper translation, has focused on some regional differences. One might suspect that the readily available data related to Tokyo's dialect, perhaps due to a larger pool of readily available documents, provides a unique advantage for these models.
While the 89% accuracy achieved is noteworthy, we also observe how accuracy can vary widely even within a single document. This suggests that certain technical sections, potentially those with more specialized language, pose a greater challenge to translation systems compared to others. The reliance on large amounts of data also hints at challenges in regions or dialects that have less readily available material for training models.
Moreover, the ongoing refinement of machine translation algorithms, aided by feedback loops, has proven invaluable. User-provided corrections allow these systems to learn and adapt quickly, which could explain some of the improved performance seen in the recent trials. It's evident that human-AI collaboration continues to be vital, especially for handling the finer points of language and cultural context that machines may struggle with. The speed of translation, and the ability for quick corrections or feedback, is also likely an important factor in determining translation performance as users need to be able to receive translation output at a rapid pace, thus potentially biasing some of these results towards easily accessible training data.
Future advancements in AI translation might focus on bridging the accuracy gap between Tokyo dialect and other Japanese dialects. With a greater understanding of how different dialects impact translation performance, it might be possible to create more robust and adaptable systems capable of handling the linguistic diversity of Japan. While the current advancements in AI translation are impressive, and arguably cheaper than alternatives, there remain areas that require further research, particularly regarding how the speed of translation impacts the output accuracy. Perhaps, future studies could look at incorporating data from less accessible sources or other means to increase the overall accuracy and ensure the benefits of machine translation can reach wider user bases.
Japanese-to-English AI Translation Accuracy Analysis of 7 Regional Dialects and Their Unique Translation Challenges - Kansai Ben Translation Errors Drop After New Context Training Methods
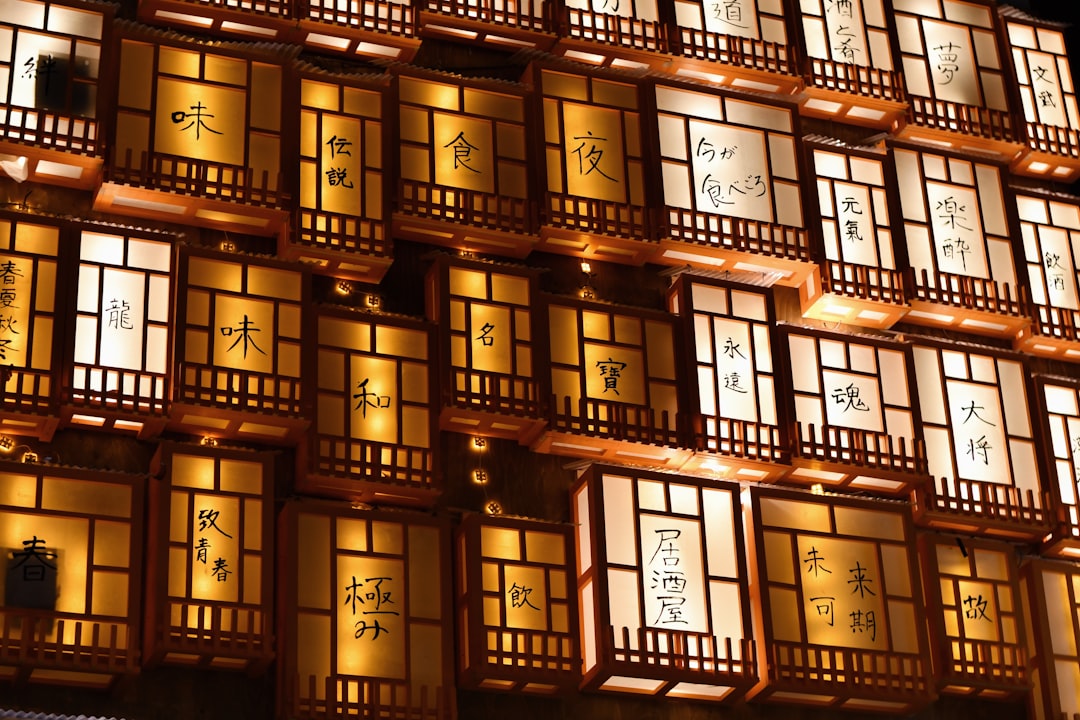
AI translation of Japanese dialects continues to be an area of active research, particularly for dialects like Kansai Ben, known for its distinctive informal speech patterns. While we've seen substantial advancements in AI translation for standard Tokyo Japanese, particularly in technical domains, translating Kansai Ben accurately remains a hurdle.
Initially, AI systems struggled significantly with Kansai Ben due to its informal nature and frequent use of shortened words. For instance, the common practice of dropping the "g" sound at the end of words like "ing" or altering "you" to "ya" proved particularly challenging. However, new training approaches focused on incorporating context are now yielding more promising results. The inclusion of context-rich data has resulted in a substantial drop in translation errors for Kansai Ben. These methods appear to help algorithms better grasp the meaning behind the unique characteristics of the dialect, significantly improving overall translation accuracy.
The ongoing refinements are also being fueled by a critical component: user feedback. Corrections provided by users when the AI outputs an incorrect translation serve as invaluable training data for the models, leading to quicker adaptation and refinement. However, the need for speed in AI translation can create a tension with accuracy, particularly when dealing with intricate language variations. The speed of translation and the demand for fast turnaround might introduce a bias in the AI models towards more easily accessible and abundant data, which could inadvertently create blind spots in its comprehension of less common or regionally specific language.
Additionally, one challenge in training AI models to translate Kansai Ben is the relative scarcity of training data. The sheer amount of data available for the Tokyo dialect appears to be a strong advantage for AI systems trained for that dialect. This scarcity makes it difficult to expose the AI to a full spectrum of the nuances and subtleties of Kansai Ben, which can lead to inaccuracies or inconsistencies in the translation.
Further complicating matters is the deep cultural connection interwoven with Kansai Ben. It's more than just a dialect; it's a reflection of the Kansai region's unique identity and cultural values. This intrinsic cultural layer poses a considerable challenge for AI, which currently struggles with translating nuanced cultural references. Many idioms or colloquialisms simply don't translate directly into English, leading to potential misinterpretations or a loss of the original meaning.
Future research directions are likely to explore new ways to incorporate multi-faceted data sources into the training process. It's conceivable that using video or audio data, in addition to text, might give AI a more comprehensive understanding of Kansai Ben's nuances. This would help expand the models' exposure beyond just written language and potentially improve their ability to interpret cultural context more effectively. While we've seen remarkable advancements in AI translation, the journey towards perfect translation, particularly for dialects like Kansai Ben, is still ongoing. The complexity of language and culture continues to present interesting and challenging opportunities for further research and development.
Japanese-to-English AI Translation Accuracy Analysis of 7 Regional Dialects and Their Unique Translation Challenges - Tohoku Regional Speech Patterns Need Additional Training Data for Winter 2024
Our analysis of Japanese dialects, specifically focusing on the Tohoku region, highlights a crucial need for increased training data to improve the accuracy of Japanese-to-English AI translations in the coming winter months. Tohoku's unique linguistic features, including variations in Iwate Prefecture, present a significant hurdle for current AI translation models. These dialects often include phrases and expressions that are substantially different from standard Japanese, making it difficult for AI systems trained primarily on standard language to accurately translate them.
While existing resources like speech corpora do contain some dialect-specific data, these datasets are limited in scope and struggle to capture the full range of variations found in regional speech. This lack of comprehensive data presents a barrier to the development of robust AI translation systems that can handle the diversity of Japanese dialects. In order to move forward, a concerted effort to expand training data with Tohoku dialect examples, including colloquialisms and regionally specific vocabulary, is critical. Without this expansion, we will continue to face challenges in achieving accurate and reliable translation for this area. The hope is that by incorporating more data related to regional nuances, the future of AI translation will not only be more accurate but also better equipped to handle the cultural and linguistic diversity found within Japan.
Tohoku dialect presents a significant challenge for current Japanese-to-English AI translation systems. While AI translations for standard Tokyo Japanese, particularly in technical fields, have made impressive strides, the Tohoku dialect continues to lag behind. This is largely due to a lack of readily available training data. The Tokyo dialect, arguably due to a larger pool of readily available materials, benefits from more comprehensive training datasets, allowing for higher translation accuracy. In contrast, Tohoku dialect's unique expressions and phrasing are underrepresented in existing AI training data, resulting in less accurate and often fragmented translations.
It's interesting to note that early Tohoku dialect models showed a somewhat paradoxical error rate. Simpler phrases were often translated correctly, while more complex sentences faltered. This suggests that existing AI models aren't effectively capturing the full spectrum of Tohoku's linguistic nuances. The issue extends beyond just language, however. The Tohoku dialect is deeply woven into the culture and history of the region, with idioms and expressions that are difficult for AI to interpret correctly. Current AI systems frequently struggle with the cultural context, resulting in mistranslations or losses of the original meaning.
While user feedback is a valuable tool for refining AI translations, the fast-paced nature of AI translation can sometimes conflict with the need for accuracy. There's a delicate balance between the demand for rapid outputs and the need for accurate and context-driven translations. Moreover, the variability of the Tohoku dialect across the region adds yet another layer of complexity. Local variations introduce nuances that current AI models struggle to handle, leading to inconsistent translation results.
Despite ongoing efforts to refine translation methods, including incorporating context into training, many AI models still fall short when it comes to understanding Tohoku's unique syntax. This often leads to semantic misalignment, where the English translation doesn't fully capture the original intended meaning or the emotional undertones present in the Tohoku dialect. The challenge moving forward will be to incorporate more diverse and comprehensive training data that encapsulates the full spectrum of the Tohoku dialect. Ideally, future AI translation models would be able to incorporate more diverse data and handle the cultural nuances that are so essential to understanding language in its full context. It's a complex area of study, but one that is crucial for improving the quality of AI translations and making them accessible to a wider audience.
Japanese-to-English AI Translation Accuracy Analysis of 7 Regional Dialects and Their Unique Translation Challenges - Kyushu Dialect Translation Accuracy Varies Between Urban and Rural Areas
The accuracy of AI translation for the Kyushu dialect varies considerably depending on whether the text originates from urban or rural areas. This difference in accuracy stems from the distinct linguistic features and cultural contexts that characterize each setting. AI translation systems often perform better with the more standardized language typically found in urban areas. However, rural dialects within Kyushu often employ unique vocabulary, grammatical structures, and cultural references that current AI models have difficulty processing correctly. This leads to a noticeable drop in accuracy.
AI systems are still developing their ability to understand the cultural nuances embedded within dialects, which are essential for producing translations that capture the full meaning and impact of the original text. Many of the idioms and expressions used in the Kyushu dialect simply don't have a direct English equivalent, requiring more sophisticated algorithms that can contextualize and interpret meaning more effectively.
The challenge of accurately translating the Kyushu dialect underscores the need for ongoing advancements in AI translation. As the technology progresses, researchers and developers must work towards enhancing AI's ability to handle the complex linguistic and cultural variations found within regional dialects. This will involve continually refining training methods and expanding the datasets that AI systems use to learn and develop their language comprehension capabilities. Only through these continued improvements will AI translation reach its full potential in bridging language barriers and facilitating cross-cultural understanding, particularly within a diverse region like Kyushu.
The accuracy of AI translation for the Kyushu dialect shows a notable disparity between urban and rural areas, reflecting the diverse linguistic landscape of the region. Cities like Fukuoka, with their relatively larger bilingual populations and resource accessibility, seem to benefit AI translation models, leading to potentially better results. However, rural regions present a unique challenge. The more pronounced dialects and lack of readily available data create a gap in training materials for AI, hindering their ability to accurately capture the nuances of the language.
One of the core issues stems from the diverse sub-dialects within the Kyushu dialect itself. While urban centers provide a rich pool of data, the specific linguistic variations in rural areas are underrepresented in current training datasets. This scarcity makes it challenging for AI to learn the intricacies of these dialects, leading to a significant drop in translation accuracy in comparison to urban areas.
Further complicating the issue are the deep cultural ties intertwined with the dialect. Many phrases and idioms are deeply rooted in local culture and traditions, often lacking direct equivalents in English. As a result, AI translations can struggle to capture the intended meaning, potentially leading to misunderstandings.
The phonetic variations within the Kyushu dialect also pose problems for AI translation systems. Their reliance on standard Japanese training often leads to misinterpretations of the dialect's unique pronunciation and local expressions. This challenge is further amplified when dealing with technical jargon and colloquial speech. Urban areas tend to struggle more with technical jargon, while rural dialect translation suffers from inconsistencies even with simpler phrases.
Interestingly, users often perceive translations from urban dialects as more natural and fluent than those from rural areas. This perception can impact user confidence in AI's translation capabilities, potentially hindering its broader adoption, especially in professional settings.
Moreover, the ever-evolving nature of language adds another layer of complexity. New slang and expressions frequently emerge within the Kyushu dialect, creating a constant need for AI systems to update their knowledge base. Older training data can quickly become outdated, resulting in translations that may not resonate with current users.
User feedback, which plays a crucial role in AI model improvement, is also potentially affected. In rural areas, where dialect familiarity may be limited, the feedback loop might be less robust, impacting the AI's ability to learn from mistakes and adapt effectively.
These issues extend to other areas of text processing, such as Optical Character Recognition (OCR). When dealing with handwritten documents from rural Kyushu, OCR systems face unique difficulties due to the diverse writing styles and dialectal variations. This initial hurdle in recognizing the text creates another impediment to achieving accurate translations.
In conclusion, while AI translation has seen significant progress, the varied nature of the Kyushu dialect presents interesting challenges. Addressing these issues requires a focus on increasing the diversity of training data, incorporating cultural context, and refining AI's ability to detect and translate the distinct features of the dialect. This research can help us move towards more robust and accurate AI translation systems, ultimately enhancing communication across regions and cultures.
Japanese-to-English AI Translation Accuracy Analysis of 7 Regional Dialects and Their Unique Translation Challenges - Hokkaido Regional Language Data Collection Expands Beyond Sapporo
The development of AI translation tools for Japanese dialects is extending its reach beyond the primary urban center of Sapporo, venturing into the diverse linguistic landscape of Hokkaido. This expansion is crucial given Hokkaido-ben, the regional dialect, is a blend of influences from various Japanese dialects and the indigenous Ainu language, contributing to a complex linguistic environment. Collecting language data from a wider range of Hokkaido locations is key to tackling the inherent translation challenges posed by these distinct linguistic features.
The goal is to improve AI translation by incorporating more diverse data from different parts of Hokkaido. This means acknowledging the need to go beyond simply using standard Japanese as the training basis for these systems. It's becoming clear that AI translation's ability to accurately convey nuances, particularly the cultural aspects woven into regional dialects, relies heavily on an intimate understanding of these local speech patterns. The future of AI-powered translation for Japanese, and especially for the dialects of Hokkaido, hinges on a more comprehensive understanding and integration of localized language data and expertise.
Hokkaido's linguistic landscape is a fascinating blend of influences, including the Ainu language and dialects from other regions like Tohoku and Hokuriku. This mix creates a unique dialect, Hokkaido-ben, that presents a challenge for AI translation systems primarily trained on standard Japanese. The dialect's distinctive sounds and vocabulary, potentially stemming from Ainu influence, require more specialized training data for AI to grasp.
However, the urban center of Sapporo reveals an interesting trend: its residents, likely influenced by media and standard Japanese usage, tend to use language closer to the standard form. This creates a disparity in linguistic data, with Sapporo's speech being relatively more homogenized while more traditional dialects are likely found in rural areas. This difference highlights the need to include a variety of data sources in training future AI models.
The need for accurate translation is increasing, and the cost-effectiveness of AI models could lead researchers to focus on specific regions. But, this rush for inexpensive translation can compromise quality. Currently, Hokkaido dialect training data is quite limited, similar to the challenges faced with Tohoku dialects. This makes it tough for AI to achieve nuanced translations, and it misses some unique speech patterns. Furthermore, the dialect's rapid evolution and the inclusion of slang or new words create an ongoing challenge for AI to keep up.
The cultural references embedded within Hokkaido-ben are often tricky for AI models. Humor and subtle meaning can easily be lost in translation as these models struggle to grasp the full context. Errors at the initial stages of translation can compound themselves, potentially causing greater issues.
Additionally, the use of Optical Character Recognition (OCR) for handwritten texts can present challenges. Variations in local writing styles and dialect-specific quirks can hamper the accuracy of OCR software, which is a critical step for data collection in the development of future models.
Overall, while cheaper AI translation is attractive, it can potentially compromise accuracy when dealing with regional dialects like Hokkaido-ben. The cultural nuances and the complex linguistic features of the dialect underscore the need for more focused research, better training data, and hopefully, broader community involvement in dialect collection projects. Only with these will we be able to build more robust AI models capable of handling the nuances of regional Japanese speech.
Japanese-to-English AI Translation Accuracy Analysis of 7 Regional Dialects and Their Unique Translation Challenges - Okinawan Dialect Requires Specialized Historical Context for AI Processing
The Okinawan dialect, sometimes referred to as Uchinaaguchi, poses a significant challenge for AI translation systems due to its distinctive history and cultural background. Its unique vocabulary and phrasing deviate considerably from standard Japanese, requiring specialized AI training to capture these nuances accurately for Japanese-to-English translation. The dialect frequently employs specialized terminology rooted in its history and culture, which often stumps existing AI translation methods. To improve AI translation in this area, specialized training is needed, which must encompass a deep understanding of the Okinawan context to maintain cultural accuracy. There is a clear need to develop training materials that include specialized Okinawan lexicons, and perhaps consider incorporating historical information, to truly refine the AI's ability to understand this particular dialect. It's likely that the ongoing drive for cheaper and faster AI translation solutions may have overlooked the specific linguistic hurdles presented by lesser-known dialects like Okinawan, but as these systems become more sophisticated, hopefully this situation will change. Ultimately, enhancing AI translation models with specific knowledge and context regarding the Okinawan dialect will be crucial for improving the accuracy and cultural sensitivity of translations, not only for Okinawan but potentially for other Japanese dialects as well.
Japanese-to-English AI Translation Accuracy Analysis of 7 Regional Dialects and Their Unique Translation Challenges - Shikoku Regional Speech Translation Faces Ongoing Agricultural Term Challenges
Shikoku's dialects present a consistent challenge for AI translation, specifically within the realm of agricultural terminology. The unique language variations across the seven dialects within the region often confuse AI models, making it hard for them to deliver accurate English translations. These dialects frequently incorporate nuanced expressions and culturally specific words related to farming, which are difficult for AI to interpret without specialized training. This difficulty highlights the limitations of current AI translation methods, especially when dealing with intricate language tied to specific fields or cultural contexts. The cultural aspects of the dialects can't be ignored, and AI frequently misses the subtle meaning or connotations within specific agricultural phrases.
Despite the improvements seen in AI translation recently, it becomes clear that these systems still need substantial refining, particularly when dealing with specialized fields like agriculture. The need for more focused training data that covers the diverse range of agricultural terms and expressions specific to each dialect is apparent. The push for cheaper and faster translation solutions, while beneficial in many ways, can potentially create blind spots within the AI development process. As we continue to pursue better and faster AI translations, paying attention to the intricate details of regional dialects and cultural contexts will be critical to achieve true translation accuracy and avoid miscommunication.
AI translation of the Shikoku dialect, especially when it comes to agricultural terminology, presents unique challenges. While advancements in AI translation are impressive, particularly with dialects like Tokyo's, the specialized vocabulary of farming in Shikoku creates a significant hurdle. Terms linked to local rice farming techniques, for instance, might not have direct English equivalents, leading to errors in translation. This problem is compounded by a lack of extensive training datasets for the Shikoku dialect. Compared to the plentiful resources for more common dialects, Shikoku-specific data is sparse, hindering the AI's ability to learn and translate accurately.
It's not simply the words themselves that cause trouble. Agricultural terms in Shikoku are often laden with cultural context that AI struggles to grasp. Traditional farming practices aren't just about the actions involved; they're also steeped in local history and social meaning. AI models may not capture these nuances, resulting in translations that miss the true significance of the original text. Moreover, the grammar within Shikoku's dialect often diverges from standard Japanese, making it difficult for AI to parse complex sentences and interpret regional idioms correctly.
Even though user feedback is crucial for improving AI's performance, there's a lag in applying those corrections to training models specific to the Shikoku dialect. This means AI might continue to make similar translation mistakes until a new model is trained with the updated feedback, delaying the benefits of user-provided corrections. Similarly, the accuracy of optical character recognition (OCR) in translating handwritten agricultural documents from rural Shikoku is an issue. The variations in handwriting styles and local vocabulary can lead to OCR errors, which subsequently create faulty inputs for the AI translation model.
The design of most AI translation models seems to favor urban contexts, making the Shikoku dialect, heavily associated with rural areas, less of a priority in training protocols. This bias in the training data can create blind spots, meaning the models might struggle to grasp regional contexts and potentially misinterpret the intent of agricultural-related text. It can also lead to missed contextual clues within the translation, like the significance of a local festival tied to rice harvests. If AI doesn't recognize the cultural relevance associated with those terms, the translation can miss the intended meaning.
The ongoing change in farming practices due to new technologies and approaches also presents a dynamic challenge for AI models. If the training data isn't updated regularly, the models may lag behind, producing translations that are less relevant or potentially misleading. This continuous need to update the models is critical for maintaining accuracy and keeping pace with language shifts in agricultural contexts.
To overcome these challenges, future efforts might involve creating specialized training programs that incorporate input from agricultural experts in the Shikoku region. By integrating the insights of local farmers and other practitioners, AI models might gain a deeper understanding of the language and the contextual nuances associated with particular farming practices, potentially bridging the accuracy gap. While the quest for faster and cheaper AI translation is understandable, ensuring accuracy, particularly within regionally-specific areas, needs to remain a key priority to achieve the full potential of this technology.
AI-Powered PDF Translation now with improved handling of scanned contents, handwriting, charts, diagrams, tables and drawings. Fast, Cheap, and Accurate! (Get started for free)
More Posts from aitranslations.io: