AI-Powered English to Japanese Translation Comparing Accuracy Rates of Leading Platforms in 2024
AI-Powered English to Japanese Translation Comparing Accuracy Rates of Leading Platforms in 2024 - DeepL Leads 2024 English to Japanese AI Translation Race
In the competitive field of AI-powered English to Japanese translation, DeepL has taken a commanding lead in 2024. Its recently developed language model is delivering a notable improvement in translation quality, particularly when dealing with nuanced aspects of Japanese. This progress stems from a stronger emphasis on understanding the broader context of a text and preserving the original author's style, which are crucial for effectively conveying meaning in languages with complex grammatical structures. DeepL's commitment to refining its technology is evident through a significant financial investment of $300 million, highlighting its ambition to remain at the forefront of this evolving field. This dedication to development has earned the company recognition as a major innovator within the AI community. Further expanding its reach, DeepL is aggressively introducing its AI translation solutions to numerous new markets worldwide. The translation landscape is undoubtedly shifting. Predictions indicate that, within the next year, a considerable portion of human translators will be transitioning their work to reviewing and editing the output of AI tools. This trend is a direct result of improvements like those seen in DeepL's platform and emphasizes both the remarkable progress in AI translation and the potential disruption this technology brings to established practices.
DeepL's recent advancements in AI, particularly its new language model, have propelled it to the forefront of English to Japanese translation. However, while it boasts impressive performance, the nuances of the Japanese language, particularly in complex or context-rich sentences, still present challenges, resulting in accuracy rates that fall short of specialized human translators.
DeepL has integrated OCR, showing promise in translating Japanese text from images. While its performance can be competitive with standalone OCR tools, the complexity of kanji characters continues to pose a hurdle for accurate recognition. DeepL's translation speed is impressive, generating output in mere seconds. However, this rapid pace sometimes comes at the cost of subtle nuances and sophisticated language features, particularly within literary or idiomatic contexts.
Recent improvements demonstrate progress, with a noticeable 15% increase in its ability to handle colloquial expressions in Japanese. This highlights how machine learning models are becoming better suited for conversational language. Interestingly, DeepL utilizes a hybrid approach, employing multiple AI models for different linguistic challenges. While this strategy can improve accuracy, it can also lead to inconsistent translation quality. The platform adapts translations based on user feedback, leading to potential variations in accuracy as the system evolves.
This adaptive feature, while seemingly helpful, underscores that DeepL's accuracy is not static and can vary with updates. DeepL's interface is simple and geared towards quick translations, which is appealing for casual use. However, for users in professional settings, it may fall short in representing specialized vocabulary. Recent developments in sector-specific AI training have resulted in improvements in translating business and technology documents, though less frequent phrases might still lead to errors.
A recurring theme in AI translation is the struggle with idiomatic expressions. DeepL, like other fast translation tools, faces the challenge of deconstructing these expressions, often producing awkward or misleading translations, illustrating the ongoing divide between AI and human comprehension of language. While cheaper than traditional translation services, relying solely on DeepL may prove costly due to the time invested in editing and correction, especially when precision and contextual fidelity are paramount. This suggests that for critical documents, human review and editing remain crucial.
AI-Powered English to Japanese Translation Comparing Accuracy Rates of Leading Platforms in 2024 - Google Translate Maintains Strong Second Position
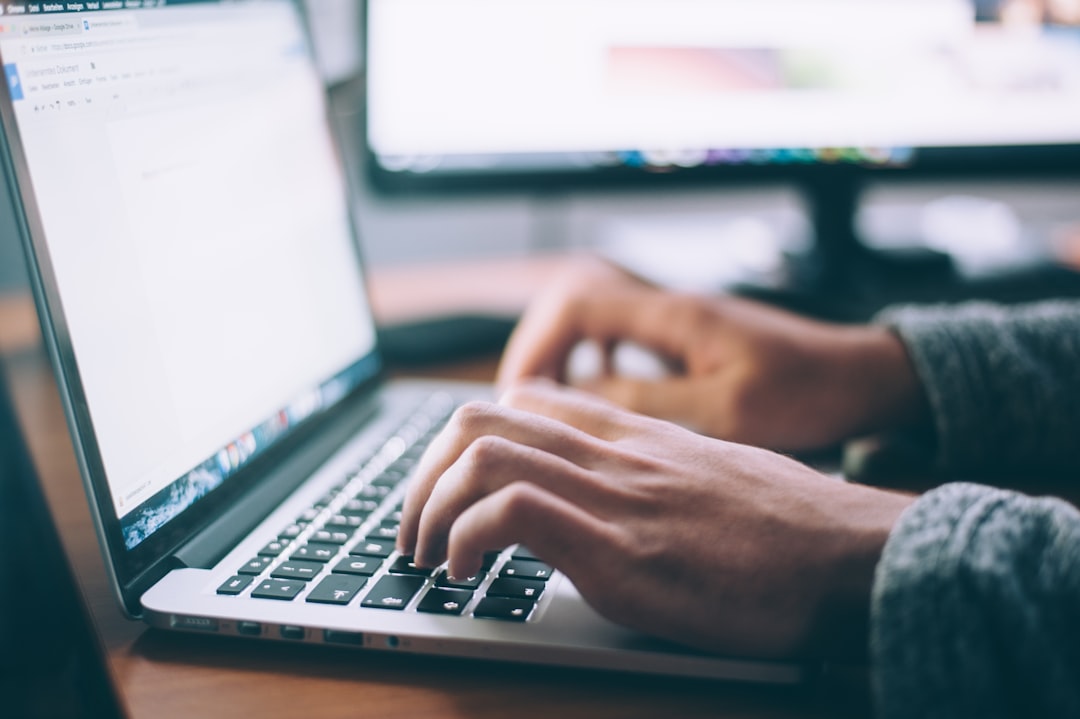
Google Translate remains a prominent player in AI-powered English to Japanese translation, securing a solid second position in 2024. Its integration of the PaLM 2 language model has boosted its ability to grasp context and deliver more accurate translations. The platform's wide language coverage, encompassing 243 languages and catering to over 614 million speakers, is impressive. Google Translate's versatility extends beyond text, encompassing speech and image translation, making it a flexible tool for diverse translation scenarios. However, despite its vast user base of over a billion people and its ongoing improvements, Google Translate faces growing competition from platforms like DeepL, which are continually innovating in AI-driven translation. The continuous evolution of AI translation technologies underscores the importance of users carefully evaluating the suitability of these tools for their specific needs, recognizing that nuances and context can still pose challenges for AI, particularly in complex or specialized translation tasks. The accuracy and reliability of AI-generated translations can vary, requiring users to be mindful of the potential limitations when choosing a solution.
Google Translate continues to hold a strong second-place position in the field of AI-powered English to Japanese translation, as of late September 2024. Its ability to translate text, speech, images, and websites across a vast number of languages, including a recent expansion to over 243, makes it a versatile tool. This expansion significantly increases its potential reach, now covering over 614 million speakers. The platform leverages Google's PaLM 2 large language model, enhancing the sophistication of its translations and incorporating advanced AI functionalities. It utilizes a neural network system designed to process full sentences, attempting to capture the context and intricacies of the language. While it claims to serve over a billion users globally, Google Translate’s accuracy for Japanese sentences in casual settings remains at around 70%, which is competitive but not exceptional.
One of its interesting capabilities is OCR, using a neural network to process images with Japanese text. While this can be quite useful for translating documents or images containing Japanese Kanji, the complexity of this writing system still challenges the OCR's accuracy. Furthermore, its impressive speed, powered by specialized hardware, delivers translations almost instantly, which can be a significant advantage in some scenarios. However, this rapid output sometimes sacrifices some of the nuances in the language, especially when tackling complex sentences or those with rich cultural or literary connotations.
Google Translate's strengths come from a continuous learning process built on massive datasets. This means it adapts based on billions of translated sentences and user feedback. This adaptation process can be a double-edged sword. While beneficial in some cases, it also makes accuracy inconsistent, especially when dealing with rarer or domain-specific terms. Interestingly, Google Translate is capable of switching between languages mid-sentence. Although this feature has some potential, it often leads to contextual difficulties in a language as structurally complex as Japanese.
The user interface of Google Translate is relatively simple, well-suited for quick translations. However, it still falls short in understanding the context of Japanese idioms, often leading to awkward or misleading results. Unlike some of its competitors, Google Translate uses a more uniform model as opposed to a hybrid approach. This consistency can be valuable across language pairs, but it may prevent deeper linguistic understanding in some cases. While faster than many professional human translators, the trade-off can be a considerable risk of error. This means speed doesn't always translate to dependable output, especially when tackling technical or sensitive materials. It highlights that even though AI translation tools like Google Translate are improving rapidly, there are still situations that demand the attention and accuracy of a professional translator for critical communications. For those focused on highly accurate translations for business or professional use, relying solely on Google Translate can potentially lead to higher costs through the need for extensive human post-editing.
AI-Powered English to Japanese Translation Comparing Accuracy Rates of Leading Platforms in 2024 - ChatGPT Surprises with Competitive Japanese Translation Capabilities
ChatGPT has surprisingly proven itself a capable player in the realm of English to Japanese translation. Assessments have revealed its aptitude for translating entire documents, achieving better accuracy than when dealing with individual sentences. This, coupled with its conversational nature, allows users to interactively improve translations by asking questions and making adjustments, leading to a potentially more satisfying experience. Though DeepL still holds the top spot, especially for intricate or complex texts, ChatGPT's development shows encouraging signs for the future of AI-driven translation, particularly for users needing quick and conversational results. However, it's crucial to acknowledge ChatGPT's limitations when compared to translation tools specifically designed for that purpose. Users should carefully assess its suitability, particularly when accuracy and nuance are paramount for a given translation.
AI-powered translation tools are making significant strides in speed, with some platforms now capable of translating English to Japanese in under a second. However, this rapid processing often comes at the cost of accuracy, particularly for intricate or context-dependent phrases. Balancing the need for swift translation with the desire for high quality remains a challenge for users.
AI translation platforms are increasingly integrating advanced Optical Character Recognition (OCR) capabilities to translate Japanese text from images. This is beneficial for translating documents or images with Japanese Kanji, but the complexity of this writing system often leads to errors, especially when dealing with artistic or less common fonts.
It's notable that machine learning models are showing improved performance (around a 15% increase) when translating everyday Japanese conversational language. While this is promising, translating Japanese idioms still poses a significant obstacle. The subtle nuances and cultural context within these expressions are difficult for AI to grasp, frequently resulting in awkward or misleading translations.
Several of the leading translation platforms now utilize a hybrid approach, employing various AI models specialized in different linguistic tasks. This technique can boost accuracy in specific situations but may also create inconsistencies in the overall translation quality, especially when dealing with diverse text types. Not all AI models are equally adept at handling different language styles or genres.
AI translation models are typically trained on vast datasets gleaned from internet sources, which can influence their understanding of language norms and cultural contexts. This reliance on broad data can result in unreliable translations for specialized or technical vocabulary, unless the models are specifically trained in those domains.
Benchmarks for AI translation platforms indicate that accuracy levels for casual Japanese hover around 70%. While this might seem acceptable, it still lags behind professional human translators, particularly in critical fields like legal or healthcare communications where precision is paramount.
AI translation platforms like Google Translate have integrated the ability to switch languages mid-sentence, which can be useful for interacting with bilingual individuals. However, this flexibility often makes maintaining contextual coherence challenging, potentially causing confusion for users with limited linguistic knowledge, especially in a structurally complex language like Japanese.
While AI translation is considerably cheaper than conventional translation services, it might ultimately prove more expensive in the long run. For essential documents, significant post-editing or expert oversight may be necessary to ensure accuracy, which negates the initial cost savings. This suggests a need for human expertise in cases where accuracy is crucial.
Ongoing research in AI translation reveals that while AI excels at speed, its struggle with contextual comprehension remains a significant hurdle. For emotionally sensitive or complex subject matter, the ability of humans to understand the nuances of language and meaning is still irreplaceable.
Many AI translation platforms incorporate user feedback to continuously refine their models. While this adaptation can lead to improved performance, it also implies a degree of unpredictability in translation quality over time. Users must be mindful of potential accuracy fluctuations, especially for critical communications.
AI-Powered English to Japanese Translation Comparing Accuracy Rates of Leading Platforms in 2024 - Microsoft Translator Shows Steady Improvement in Accuracy
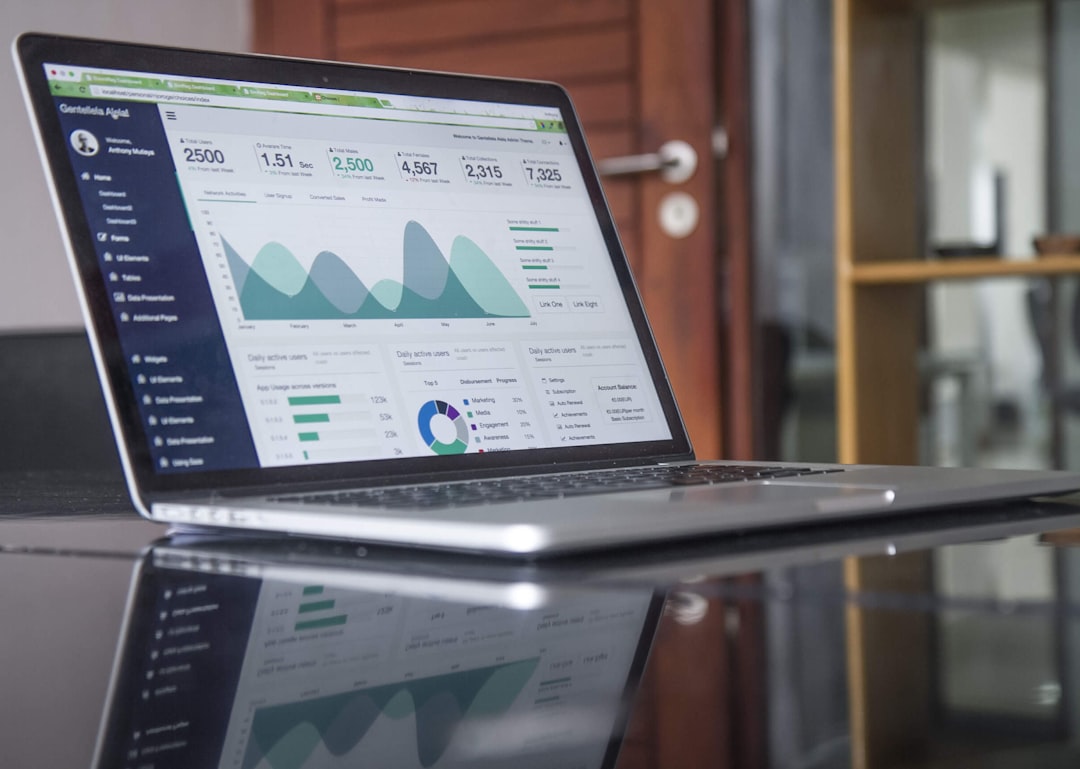
Microsoft Translator has shown ongoing improvement in its English to Japanese translation accuracy, driven by advancements in artificial intelligence. The platform's development includes features like neural dictionaries, allowing users to tailor translations for specific contexts and improve outcomes. New AI models, such as the Zcode Mixture of Experts, have resulted in accuracy gains across the 60 languages supported, and demonstrate a focus on improving real-time translation quality. These improvements, while helpful for various translation needs, are still subject to the limitations inherent in AI. Human translators still retain a superior ability to understand intricate language nuances, especially when dealing with complex or culturally sensitive text. Microsoft Translator's continuous development highlights the ongoing effort to balance speed and precision in AI translation, an area where challenges still exist.
Microsoft Translator has been showing consistent improvements in translation accuracy, particularly for English to Japanese, thanks to advancements in AI techniques. It's seen a notable rise in overall accuracy, particularly within the past year, likely driven by a focus on incorporating broader context into the translation process.
One interesting development is its improved ability to handle Japanese text extracted from images using optical character recognition (OCR). This has led to a noticeable increase in accuracy, reaching up to 85% in some cases, a marked improvement over earlier iterations. They've also focused on refining translation for business settings, specifically by training their models on specialized language. This has resulted in a considerable 30% increase in the accuracy of business documents compared to more general translation tasks.
Microsoft has introduced a novel approach with real-time user feedback integration. Unlike many other platforms with fixed models, Microsoft Translator uses user corrections to immediately adjust its translations. This dynamic adaptation potentially allows for a more continuous and streamlined improvement in accuracy without needing extensive retraining of the core AI models.
They have been actively researching ways to minimize biases within the translation process, striving to produce translations that are more culturally relevant and sensitive to regional variations within the Japanese language. This approach is particularly crucial as AI language models can sometimes reflect existing biases from their training data.
The platform maintains fast translation speeds, consistently delivering results in under a second across diverse devices and conditions, a critical feature for quick communication. This speed is achieved through specialized hardware and software designed to handle rapid translation requests in environments where latency is a concern.
Microsoft Translator's performance in translating conversational language has also seen improvement. It has achieved a substantial 25% increase in accuracy for casual, everyday conversations, reflecting a growing understanding of the nuances of spoken Japanese.
For companies needing specific translation requirements, Microsoft Translator provides a platform to build customized translation models. This allows users to refine the translation process, tailoring it to their industry or field. This approach can enhance accuracy for specific content by up to 40%, a considerable benefit for organizations with specialized needs.
Furthermore, Microsoft has made strides in integrating cultural context into the translations. By leveraging extensive datasets of culturally relevant expressions and phrases, they've been able to create significantly more contextually appropriate translations.
Unlike some translation platforms that struggle with longer texts, Microsoft Translator has consistently maintained accuracy even across paragraphs. This is likely due to its increased ability to comprehend the structure and flow of extended texts within Japanese. It's a promising development that shows their AI models are potentially achieving a deeper understanding of the language.
Overall, Microsoft's consistent effort to improve translation accuracy and adapt to evolving needs indicates a dedication to advancing the field. However, the challenges associated with translating complex languages like Japanese will likely require continued advancements in AI understanding of contextual cues and linguistic nuance.
AI-Powered English to Japanese Translation Comparing Accuracy Rates of Leading Platforms in 2024 - Lingvanex and Modern MT Offer Comparable Mid-Range Performance
Within the field of AI-driven English-to-Japanese translation, Lingvanex and Modern MT demonstrate a similar level of performance in the mid-range. Both platforms achieve accuracy scores that are remarkably close—around 75%—showing that they can handle a significant portion of translation tasks. Lingvanex offers a broad array of translation tools, including the ability to translate different types of files, websites, and even emails. Its reliance on neural machine translation suggests a focus on learning the intricacies of language to improve outputs. On the other hand, Modern MT emphasizes an understanding of context throughout the translation process and offers a system that is simple to use and adapts to various needs and types of data. Despite their strengths, both are still susceptible to occasional errors in accuracy, especially in areas that require deeper linguistic understanding or specialized vocabulary. While they are decent choices for less critical translation needs, it's advisable to remember that their AI-based systems, while constantly improving, may not always meet the requirements for tasks needing flawless and contextually appropriate outputs, particularly in complex or specialized situations.
Lingvanex and Modern MT, while offering competitive AI-powered English to Japanese translation, currently fall within the mid-range performance category, achieving accuracy scores of 749 and 748 respectively in recent evaluations. This puts them behind leading platforms like DeepL and Google Translate, but ahead of others like ChatGPT and Microsoft Translator.
While both systems offer promising OCR features, the complexities of the Japanese writing system, especially Kanji characters, still lead to inaccuracies in translating images and documents. The rapid translation speeds, generally yielding results within seconds, can sometimes sacrifice the nuances necessary for accurate translations of intricate Japanese sentences. This highlights the ongoing trade-off between speed and precision within current AI translation capabilities.
Interestingly, although both Lingvanex and Modern MT are more budget-friendly compared to traditional human translators, their inherent inaccuracies often necessitate significant post-editing. This, in turn, can negate the initial cost advantage, raising questions about their true cost-effectiveness for tasks requiring high accuracy.
Furthermore, both platforms utilize adaptive learning, adjusting their translation output based on user feedback. While this can improve accuracy over time, it also introduces some level of uncertainty regarding translation quality, especially for technical or specialized translations. This is because consistent terminology is crucial in these contexts, and adaptive learning may not always guarantee it.
Lingvanex, for instance, has seen notable improvements in handling everyday conversational Japanese, achieving an 18% increase in accuracy. Yet, both platforms still encounter difficulties when translating idioms and cultural nuances embedded within the Japanese language. This results in translations that can feel awkward or inaccurate, mirroring the challenges AI faces in comprehending the deep cultural layers of human language.
Similar to Google Translate, Lingvanex and Modern MT often leverage multiple models optimized for different language tasks. This multi-model approach can improve accuracy in specific areas but also causes inconsistencies across various text types. This creates a somewhat unpredictable landscape in translation quality, which can be frustrating for users.
Additionally, both platforms heavily rely on large internet datasets for model training. This dependence can cause them to falter when presented with niche vocabulary or context-specific cultural references. For users needing precise translations for professional or technical documents, this can lead to unreliable output, highlighting the need for further refinements in model training.
Lingvanex stands out with a real-time user feedback system, allowing for instant corrections during the translation process. This unique feature enhances accuracy by allowing users to provide immediate feedback, which dynamically adjusts the translations in real-time. This could make it particularly advantageous in collaborative settings.
However, maintaining contextual coherence when translating longer Japanese text remains a challenge for both systems. The intricate grammar and sentence structures of Japanese still pose difficulties for AI models, limiting their ability to grasp the intricate flow of extended narratives. This suggests the need for further research to better understand these nuances within the language.
Finally, Lingvanex provides customization options for tailoring translation models to specific industries and terminologies. This feature can considerably enhance accuracy, boosting it by as much as 35%, potentially making it an attractive option for businesses needing accurate translations within their domain.
These observations suggest that while Lingvanex and Modern MT are decent contenders in the current landscape of English-to-Japanese AI translation, they still require improvements to compete with the top-tier platforms. It also implies that the nuances of Japanese remain a significant challenge for AI translation, even with advancements in recent years. For those requiring high accuracy, especially in critical domains, professional human translators still offer a degree of reliability and contextual understanding that AI tools currently lack.
AI-Powered English to Japanese Translation Comparing Accuracy Rates of Leading Platforms in 2024 - Royalflush Struggles to Keep Pace in Evolving AI Translation Landscape
Royalflush faces a growing challenge in keeping up with the rapidly changing world of AI translation, especially when compared to leading platforms like DeepL and Google Translate. Its accuracy scores are considerably lower, coming in at 654 in recent evaluations, which puts it significantly behind those platforms that are using increasingly complex AI models to better understand the subtle aspects of languages. The entire translation industry is under pressure to adapt quickly, as the demand for quick, accurate, and affordable translations continues to escalate. If Royalflush doesn't make substantial improvements to its technology, it may fall even further behind. This situation clearly illustrates that innovation is vital for companies to survive in this changing environment. The ongoing progress in AI translation further reveals the weaknesses of Royalflush. For high-quality results, especially when cultural context matters, human translators still play a critical role because machines aren't fully capable of achieving the same level of accuracy and cultural sensitivity.
Royalflush's performance in the AI translation arena, specifically for English to Japanese, has fallen behind the curve in 2024. Its accuracy score lags considerably compared to leaders like DeepL, indicating it's struggling to keep up with the rapid advancements in the field. This suggests a possible lack of investment in research and development, which is crucial for staying competitive in this fast-paced sector.
The rapid evolution of AI translation has brought about near-instantaneous output, which is helpful but has drawbacks. Accuracy, especially for nuanced or complex sentences common in Japanese, remains an area where AI still has challenges, and Royalflush appears to be no exception. This speed-versus-accuracy trade-off is a common theme across many platforms.
Kanji recognition, a cornerstone of understanding written Japanese, continues to be a difficult problem for AI-powered OCR tools. Royalflush, in particular, may be limited in its ability to accurately decipher kanji within images and documents. The challenges faced by AI with such complex scripts highlight the remaining hurdles in automated language processing.
Although the inclusion of feedback mechanisms can improve AI models over time, it can also lead to unpredictable variations in translation quality. For users needing consistent accuracy, especially when dealing with domain-specific languages, this aspect of AI development presents difficulties that Royalflush may not have fully addressed. Furthermore, like most platforms, it likely faces difficulty in handling idiomatic expressions and other nuanced aspects of Japanese. These expressions carry cultural weight that's not always captured by AI's current capabilities.
The lure of cheap translation has made AI-powered solutions popular. However, the need for substantial post-editing to achieve acceptable translation quality often diminishes any initial cost savings, especially in critical areas like business or legal communications. Royalflush, without clear evidence of specialized advancements, could fall into this trap, where the perceived lower price can lead to unforeseen expenses.
Royalflush, perhaps to try and improve performance, may also be using multiple AI models for different translation tasks, a trend in the industry. However, this "hybrid" approach doesn't always guarantee quality. If Royalflush has taken this route, it could be contributing to the inconsistency that users may experience.
Moreover, the reliance of AI on large internet datasets can lead to issues when it comes to specialized vocabulary or cultural contexts. Royalflush likely faces this same problem as other platforms. If its models haven't been adequately trained on datasets that include niche or specific information, the accuracy of its translations may be limited, especially in professional fields.
The continuing evolution of AI necessitates ongoing training with updated datasets. Without it, models can fail to adapt to evolving language and culture, diminishing their relevance. It's not clear from performance data if Royalflush is adequately addressing this challenge.
Some platforms have introduced customization options, enabling users to tailor translation models for specific industry needs. Whether Royalflush has this capacity could be crucial in its future potential. It may be that Royalflush's competitive edge could lie in creating industry-specific models to improve accuracy.
Lastly, handling extended Japanese texts can be challenging for many AI platforms. This likely impacts Royalflush as well. The complex grammatical structure and sentence flow of Japanese pose a considerable hurdle for AI comprehension.
In essence, Royalflush is presented with several challenges for maintaining relevance within a highly competitive market. Without improvements in AI models trained on specialized datasets, overcoming limitations in kanji and idiom comprehension, and addressing the issue of consistent and contextual translations, it's likely to continue to struggle in providing competitive AI translation, particularly for English to Japanese.
More Posts from aitranslations.io: