AI-Powered PDF Translation now with improved handling of scanned contents, handwriting, charts, diagrams, tables and drawings. Fast, Cheap, and Accurate! (Get started for free)
AI Translation Challenges Navigating Mexican and Spanish Dialect Differences
AI Translation Challenges Navigating Mexican and Spanish Dialect Differences - Regional Variations Pose Hurdles for AI Translation Models
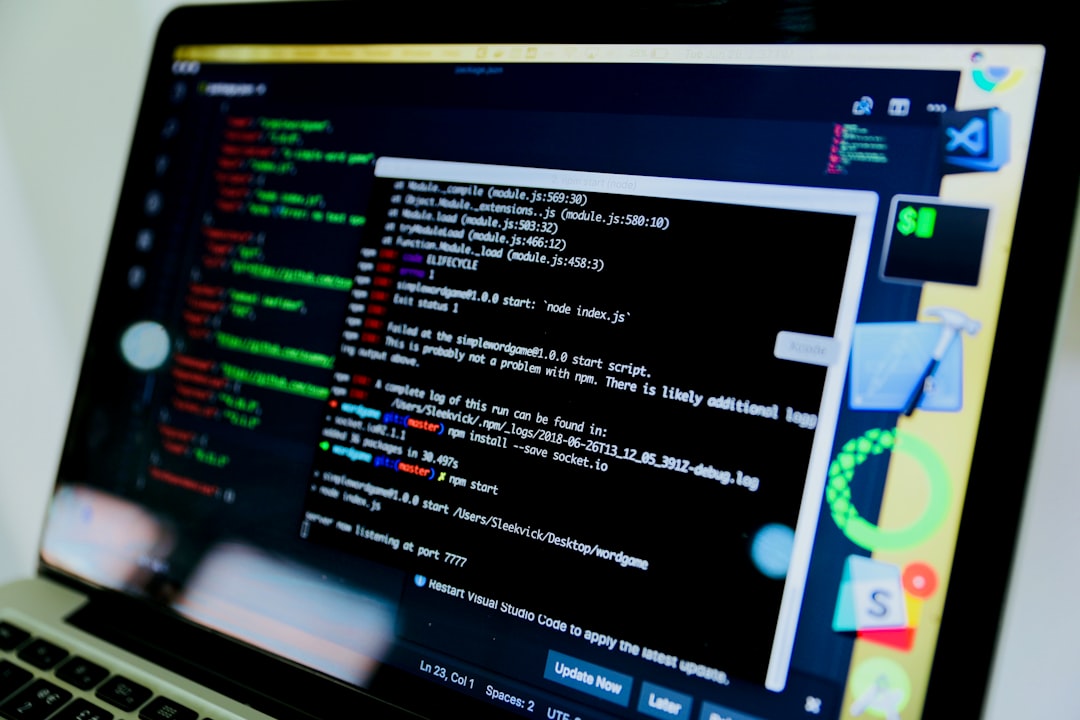
AI translation models face a significant roadblock when dealing with the diverse landscape of regional languages, particularly the differences between, say, Mexican Spanish and standard European Spanish. These models frequently stumble over the subtle variations in slang and colloquialisms common within specific regions, potentially leading to misinterpretations and a loss of the rich cultural undertones present in communication. Moreover, many AI translation systems are trained using a relatively narrow set of dialects, limiting their ability to adapt to the ever-changing nature of language. This constraint can become especially problematic as the demand for accurate, nuanced translations rises within our increasingly interconnected world. The task of retaining both linguistic intent and cultural context in translations remains a persistent challenge, highlighting the inherent limitations of solely employing AI to handle complex translation tasks.
The sheer diversity within the Spanish language presents a significant hurdle for AI translation models. With over 20 distinct dialects, each featuring unique vocabulary, pronunciations, and grammatical structures, achieving truly universal accuracy is a complex challenge. For example, a phrase like "coger" might signify "to take" in one region, yet have entirely inappropriate connotations in another. These regional variations aren't just about vocabulary; they fundamentally change the meaning of phrases and easily introduce misunderstandings if not accounted for.
Context is another major obstacle. The same word, say "papel", can mean "paper" in one instance, but "role" or "part" in another depending on the context and regional variation. These nuances demand a level of comprehension that current AI models struggle to achieve. Furthermore, Spanish from Spain frequently employs archaic verb forms that are rarely used in Latin America, creating a further translation barrier for users unfamiliar with these expressions.
The accuracy of Optical Character Recognition (OCR) also gets affected by dialectal pronunciation differences. These variations in how words are spoken can cause confusion when translating spoken language into written text, highlighting the limitations of AI in dealing with spoken language. For instance, the use of "vos" instead of "tú" in many Latin American countries marks a significant grammatical shift that AI systems must adapt to in order to accurately convey informal and formal speech.
Cultural references also pose problems for AI. A widely understood phrase in one region might be utterly foreign in another. This requires AI translation systems to develop a much deeper cultural understanding for achieving truly accurate results. The desire for both speed and accuracy in translation often creates tension. Rapid translation, driven by a need for fast turnaround times, frequently results in neglecting finer points like tone or cultural relevance, leading to errors that can drastically alter the intended meaning of the text. Furthermore, AI models are often trained on restricted datasets that might favor dominant dialects, inadvertently marginalizing less frequently used variations.
Finally, the complexities of humor, idioms, and literary expressions represent a formidable challenge for AI translation technology. A humorous phrase in one dialect might be completely lost or even misinterpreted in another. These cases clearly illustrate the current limitations of AI in replicating human understanding of cultural nuances and linguistic subtleties in translation.
AI Translation Challenges Navigating Mexican and Spanish Dialect Differences - AI Adapts to Colloquialisms in Mexican Spanish
AI translation models face a hurdle when dealing with the unique characteristics of Mexican Spanish, particularly its diverse range of slang and regional expressions. These colloquialisms often carry layers of meaning that current AI systems struggle to fully grasp, making accurate translation a challenge. The constant evolution of language, including the emergence of new slang and variations within dialects, demands a continuous learning process for AI. These systems need to adapt quickly and accurately to new trends while maintaining the subtle cultural nuances crucial for effective communication.
The ever-growing need for accurate and culturally-sensitive translations highlights the limitations of current AI models. They often struggle to fully capture the depth and richness of human language, especially when it comes to dialects like Mexican Spanish. This can lead to simplified or even inaccurate translations that lose important context. The danger is that AI translations can overlook the variety and nuances inherent in Mexican Spanish, potentially diminishing the intended meaning of the text. Achieving true translation accuracy requires a deeper understanding of regional dialects, a capability still under development for AI. Without this ongoing development, AI translation risks delivering less-than-optimal results, underselling the true diversity and character of the language.
AI's journey into accurately translating Mexican Spanish is fraught with challenges arising from the rich tapestry of colloquialisms and cultural nuances woven into the language. Phrases like "echarle ganas," while easily understood by native speakers as a motivational encouragement, can lose their impact when directly translated, highlighting the need for AI to grasp the underlying intent.
The influence of indigenous languages, notably Nahuatl and Mayan, adds another layer of complexity. Mexican Spanish is infused with unique vocabulary and expressions that stem from these linguistic roots, and an AI model not attuned to these influences risks missing crucial cultural context. Furthermore, the rapidly evolving slang and colloquialisms that characterize Mexican Spanish, unlike the more standardized European variety, present a significant hurdle for AI. Keeping pace with these changes necessitates constant model updates, which can be a daunting task for developers striving for fast and relevant adaptations.
The subtle variations in formality conveyed through the use of "tú" and "usted" also pose a problem. An AI system unable to discern the level of formality intended can misinterpret interactions, potentially leading to awkward or even offensive communication.
OCR technologies also face unique challenges with Mexican Spanish. The diversity of fonts, handwriting styles, and colloquial expressions common in handwritten texts present hurdles for accurate character recognition. These variations can impede the precise understanding of phrases, resulting in a loss of contextual meaning.
Even commonplace expressions can be misinterpreted without context. For example, "no mames," a casual Mexican phrase expressing disbelief, could be misunderstood by AI if the context isn't carefully considered, underscoring the gap between literal translation and the intended sentiment.
Cultural references in Mexican media, such as films or music, are laden with regional expressions that are often difficult for AI to translate accurately. A phrase or joke that resonates with Mexican audiences may fall completely flat or become misinterpreted when translated, revealing the critical role of cultural understanding in translation. Humor itself presents a unique obstacle. The reliance on wordplay and cultural references in Mexican humor often eludes AI's grasp, causing jokes to lose their intended impact or be misinterpreted entirely.
While advancements in machine learning empower AI to progressively learn from user interactions, making it more adaptable to colloquialisms, this process is gradual. It requires a substantial user base to capture the full range of variations, hindering immediate effectiveness. The prevalence of texting and social media adds yet another layer to the challenge. The rapid evolution of language through informal shorthand and creative spellings complicates AI translation, requiring systems to constantly adapt to capture these trends.
The path to perfect AI translation of Mexican Spanish is an ongoing journey. The complex interplay of colloquialisms, cultural references, and dynamic language evolution continue to challenge the capabilities of AI systems. Overcoming these obstacles requires a continued push for model improvement, drawing on increasingly robust datasets and leveraging techniques that can better capture the intricate nuances inherent in human language.
AI Translation Challenges Navigating Mexican and Spanish Dialect Differences - Machine Learning Tackles Pronunciation Differences
Machine learning is showing promise in tackling the challenge of diverse pronunciations across different dialects, especially within languages like Spanish where variations between, for example, Mexican and European Spanish are substantial. These pronunciation differences can significantly impact how words are perceived and translated, ultimately affecting the accuracy of AI-powered translation systems. For instance, regional variations in vocabulary, slang, and informal speech styles can lead to misinterpretations if AI models fail to accurately identify and process them. As language continues to evolve, particularly with the development of new slang and pronunciation patterns, machine learning models need to continuously adapt and update themselves to stay current. However, despite advancements, the complexities inherent in regional dialects continue to pose difficulties that require the current technology to undergo further refinement to facilitate truly accurate and effective communication across dialects. The path toward achieving seamless communication across Spanish dialects remains an ongoing effort requiring continuous technological innovation.
Machine learning models are still grappling with the complexities of pronunciation differences across Spanish dialects, especially when it comes to Mexican Spanish. For example, the seemingly simple word "casa" (house) can be pronounced quite differently in Mexico versus Spain due to regional accents, which can confuse AI systems. This can lead to the AI interpreting them as different words altogether.
These phonetic quirks can also cause headaches for Optical Character Recognition (OCR) systems. AI models trained primarily on standard European Spanish often falter when encountering handwritten or quickly typed text using regional slang or variations in Mexican Spanish. This can significantly impact translation accuracy, especially when trying to process informal communication.
Voice recognition systems frequently rely on training datasets that aren't diverse enough to cover the broad range of pronunciations and speaking styles in Mexican Spanish, resulting in AI struggling to understand verbal communication. The differences extend beyond just individual sounds, as variations in dialect can also affect word stress, and this shift can alter the overall meaning of the word. Consequently, AI algorithms trained on non-regional datasets are likely to make errors in interpretation.
Researchers are attempting to address dialectal differences using techniques like transfer learning, but there's still a ways to go. A significant hurdle is that training datasets tend to reflect existing biases, often favoring dominant dialects and not capturing the full spectrum of Mexican Spanish variation. This lack of representation skews how AI systems learn and can hinder performance.
Furthermore, AI struggles to correctly differentiate between homophones that sound alike but have distinct meanings based on pronunciation across dialects. Words like "baca" (roof rack) and "vaca" (cow), for instance, can be troublesome if the model lacks the proper phonetic training to distinguish them.
Compounding the problem is that many AI models are largely trained on data from readily available media, which often favors more mainstream dialects. This can unintentionally create models that are less adept at understanding the nuances of regional variations, particularly in lesser-known areas of Mexico. Informal communication, primarily through texting and social media, evolves rapidly, rendering training datasets obsolete quickly. Machine learning systems then face the difficult task of keeping up with constantly shifting slang and pronunciation changes in Mexican Spanish.
The issues extend beyond single words, as AI has trouble understanding idiomatic expressions due to a lack of phonetic awareness of regional dialects. Trying to interpret phrases like "dar el gatazo" (to pass something off as real), for example, results in lost context and meaning if the model isn't trained on the regional usage.
Interestingly, several AI systems also struggle with understanding the rhythm and flow of speech, often leading to unnatural and stilted translations. This highlights the need for more advanced machine learning algorithms capable of recognizing the prosodic features of language and adapting them when translating spoken words into written text, especially across different dialects.
The journey to accurate AI translations for Mexican Spanish is still ongoing. The intricate blend of colloquialisms, regional culture, and the dynamic nature of language presents a significant challenge. Addressing these hurdles requires a persistent focus on improving models, building stronger training datasets, and developing approaches better suited to grasping the intricacies of human language.
AI Translation Challenges Navigating Mexican and Spanish Dialect Differences - Cultural Context Integration in AI Translation Systems
Integrating cultural context into AI translation systems is essential for addressing the complexities of dialectal variations, especially between Mexican and Spanish. For effective cross-cultural communication, AI models need training data that authentically reflects the diverse range of language used within a culture, including slang, idioms, and regional expressions. Many AI translation systems are currently limited by training data that's either outdated or doesn't capture the full spectrum of cultural nuances. This can result in translations that miss the mark, lacking the sensitivity and understanding crucial for conveying the intended meaning across dialects. The goal is not just to translate individual words, but to faithfully reproduce the nuanced communication that is fundamental to human interaction. With continuous refinement of training data and improvements in model design, AI translation systems can progress towards successfully bridging communication barriers across cultural and linguistic differences. This continuous evolution is crucial in a world where rapid, accurate translation across diverse language communities is ever more vital.
AI translation systems often struggle to capture the full range of Spanish dialects because their training data frequently doesn't fully represent the diversity of regional variations. This can lead to less-than-accurate translations, especially when dealing with unique expressions that are vital for understanding the intended message.
The subtle variations in how formality is conveyed, like the difference between using "tú" and "usted", can significantly change the tone of a conversation. If AI models miss these nuances, they can easily misinterpret interactions, potentially creating awkward or even offensive communication.
Mexican Spanish, in particular, is constantly evolving with new slang terms and expressions popping up rapidly. This fast-paced linguistic evolution presents a significant hurdle for AI models that need to keep up with the changes. Failing to do so results in translations that might be out-of-date or plain wrong.
Things like culturally-specific locations or people (what we call "named entities") often require an understanding of the context that many current AI models simply don't have. For example, translating a reference to a regional dish or a famous person without grasping the cultural significance can produce nonsensical results.
OCR systems, which convert images of text into digital form, can stumble when faced with different handwriting styles or regional fonts. The algorithms used for character recognition aren't always great at handling the informal writing styles common in casual communication.
While machine learning is being used to improve AI's ability to handle dialects, there's a risk of unintentionally reinforcing existing biases present in the training data. This can lead to AI favoring more mainstream dialects over regional ones, making the translation process less inclusive.
The way a word is pronounced in Spanish can dramatically change its meaning based on region. For instance, a word like "papa" could mean "father" in one area and "potato" in another. Without a nuanced understanding of these pronunciation variations, AI systems can easily get confused.
Humor, which is inherently tied to cultural understanding, poses a difficult challenge for AI translation. Jokes that depend on wordplay or specific cultural references often don't translate well, leading to situations where the humor is lost or even misinterpreted.
Cultural references found in popular media, like movies or music, can be particularly problematic for translation systems. A phrase or joke that resonates with people in one region might be completely lost on people elsewhere. This highlights the need for AI to be contextually aware if it's going to accurately capture the intended meaning.
Current AI advancements rely heavily on interactions with users for machine learning improvements. However, AI still struggles to capture the full range of dialectal variations, and this limitation often means missing the small details that make language so rich and expressive.
AI Translation Challenges Navigating Mexican and Spanish Dialect Differences - Addressing Idiom Discrepancies Between Dialects
Addressing idiom discrepancies between dialects presents a significant hurdle for AI translation systems, especially when dealing with the vast array of expressions within Mexican and standard Spanish. These AI models often struggle to interpret idioms and colloquialisms like "echarle ganas" or "no mames," which can carry deep cultural meaning in one region but be misinterpreted in another. The continuous emergence of new slang and the dynamic shift in regional language styles further complicate the situation, as what's current today might be outdated tomorrow. This necessitates a constant learning process for AI, relying on real-world interactions and a continuous update of their knowledge base regarding context and cultural nuances to produce accurate and culturally sensitive translations. To truly achieve seamless communication across dialects, AI will require not only advanced algorithms but also a dedicated effort to incorporate cultural awareness and a deeper understanding of the subtleties inherent in human language.
AI translation systems face a significant challenge in accurately capturing the nuances of Spanish dialects, especially when dealing with the variations between Mexican Spanish and standard European Spanish. There are over 20 distinct varieties of Spanish, each with its own vocabulary, pronunciation quirks, and even grammatical differences. This creates a scenario where a straightforward phrase can carry completely different meanings depending on where you are in the Spanish-speaking world.
The influence of indigenous languages like Nahuatl and Maya on Mexican Spanish adds another layer of complexity for AI. These languages have contributed unique expressions and vocabulary to the Mexican dialect that are not found in standard European Spanish. Without incorporating these influences, AI systems risk missing crucial cultural context, resulting in translations that miss the mark.
The drive for rapid translation, often fueled by a need for fast turnaround times, can be detrimental to accuracy, especially when dealing with culturally specific idioms and expressions. The rush to translate can cause AI models to prioritize speed over capturing subtle cultural nuances or accurately interpreting regional variations in Mexican Spanish. Consequently, the desired meaning can easily be lost or even misinterpreted, leading to frustration or misunderstandings.
Optical Character Recognition (OCR) technology also faces challenges with dialects due to the variations in pronunciation. AI trained primarily on standard Spanish can misinterpret words in scanned texts when encountering regional slang or pronunciation changes. This can impact the overall accuracy of translation, especially in informal settings where dialects are more common.
The interpretation of phrases like "no mames" poses difficulties for AI. The meaning of the phrase can change based on context and tone of voice, factors difficult for an AI system to pick up on. As a result, AI translations could either miss the intended playful tone or unintentionally convey a harsh or offensive message.
Similarly, culturally specific references to people, places, or events can confuse AI translators. If a model lacks contextual understanding, it might misidentify or exclude these crucial elements, resulting in translations that are inaccurate or lack clarity.
Furthermore, AI grapples with homophones – words that sound alike but have different meanings depending on dialect. Words like "baca" and "vaca," for example, could easily be confused by AI if it doesn't take regional pronunciations into account.
Training data for AI often leans towards dominant dialects, creating a bias that can limit the ability of AI to understand and translate less-common regional expressions found within Mexican Spanish. This imbalance can lead to inaccurate translations that don't represent the richness and variety of dialects.
The way a word is used can dramatically change its meaning depending on the context. For instance, "papel" can refer to "paper" in one instance, but to "role" in another, based on the surrounding words. The dynamic nature of language and the need to recognize the subtle contextual changes pose difficulties for AI, often leading to mistranslations.
Finally, translating humor is a particularly difficult challenge for AI. Humor often relies on wordplay or cultural references, making it tough for AI to capture the intent. This can lead to jokes falling flat or being misinterpreted entirely, highlighting a significant limitation in AI's ability to accurately replicate human understanding of cultural and linguistic nuances. As a result, the intended humor and meaning of the translation might be completely lost, and users will be left wondering why there was a failed attempt at humorous language.
AI technology has made great strides, but there's a lot of room for improvement in capturing the complexity and richness of human language, particularly when translating between dialects. Ongoing research and development efforts are crucial to refine these models and improve the accuracy of cross-dialect communication.
AI Translation Challenges Navigating Mexican and Spanish Dialect Differences - Continuous Data Updates Key to Accurate Translations
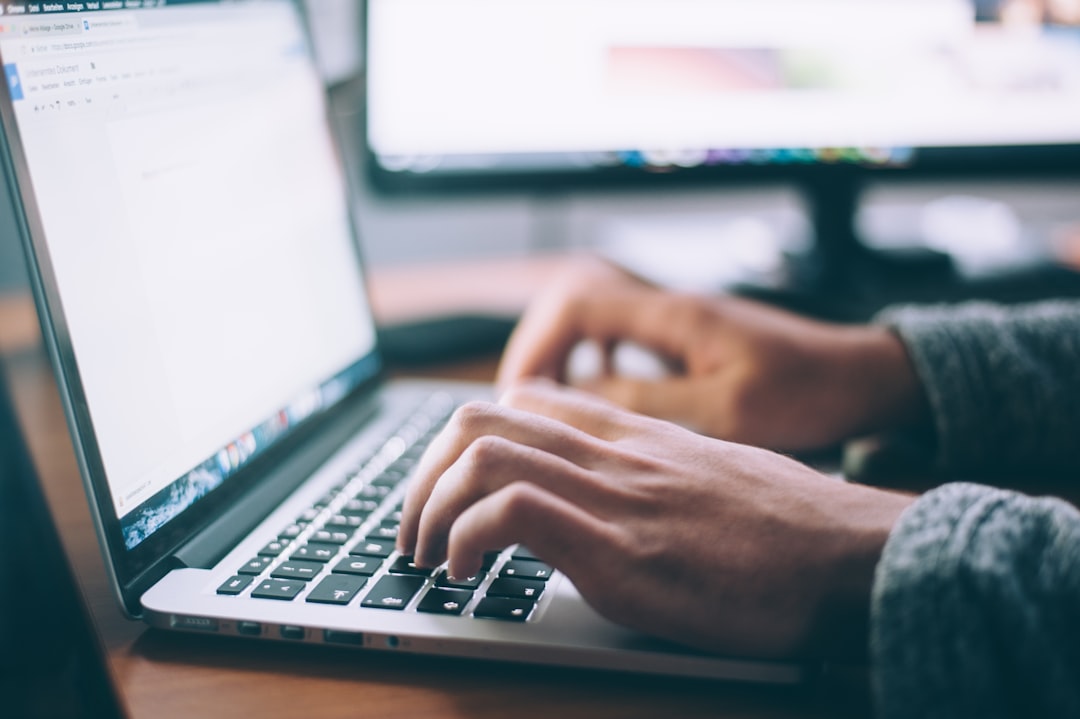
For AI to accurately translate languages, especially those with diverse regional variations like Mexican Spanish, it's crucial that the systems are constantly fed with new data. Language is always changing, with new slang, phrases, and pronunciations popping up frequently. If AI models aren't regularly updated with this evolving data, their ability to understand the subtle nuances within dialects decreases significantly. This can lead to mistranslations, causing misunderstandings and possibly losing some of the original message's important meaning. The challenge for AI is that human language is incredibly dynamic, especially in areas with a mix of cultures. AI models have to adapt to this, and it can be difficult for them to stay current with the rapid changes. To make translations both accurate and culturally relevant, AI development needs to focus on improved training methods that teach AI to be flexible and to understand cultural context better. Without this continuous effort, AI translations might not be up to par with what human translators are able to achieve.
The continuous refinement of AI translation models through ongoing data updates is proving crucial for accurate translations, especially when dealing with the dynamic nature of languages like Spanish and its diverse dialects. This adaptive learning process allows AI systems to better understand the ever-changing landscape of colloquialisms, slang, and regional variations that are prevalent in Mexican Spanish, for example. However, reliance on limited or outdated training datasets can lead to inaccurate translations, particularly when dealing with informal language and cultural references.
For instance, if a system's training focuses primarily on formal, standardized language, it might struggle to interpret everyday expressions and culturally relevant contexts. This highlights a weakness in purely relying on readily available sources for AI training, potentially leading to translations that feel stilted and miss the mark when it comes to capturing the nuances of a particular region.
Furthermore, the quality of cheaper or faster translation solutions can be compromised. By focusing solely on speed or affordability, these solutions often fall short in their ability to accurately represent the subtle meaning of idiomatic expressions. In such instances, continuous data updates and advancements in machine learning are crucial for enhancing accuracy over time.
OCR technology also benefits greatly from continuous datasets. With each new data point, these systems refine their ability to decipher patterns in regional dialects, ultimately resulting in better interpretations of informal texts and those rich with culturally-specific language like social media posts or even handwritten notes.
The variations in dialect pronunciation across different regions pose a challenge for AI voice recognition systems. For instance, the word "casa" can be pronounced quite differently in Mexico versus Spain. Consequently, for these AI models to perform effectively, continuous dialect-specific updates are essential.
Studies have indicated that cultural references within dialogues significantly impact translation accuracy. Incorporating real-world linguistic trends on a recurring basis helps AI capture the unique essence of phrases that might hold particular cultural weight in one dialect compared to another.
Despite advancements, AI translation remains limited in its ability to process nuances such as humor and idioms. This complexity requires ongoing learning from user interactions and user-generated content that incorporates humor and colloquialisms, which are vital in expanding AI’s flexibility and responsiveness.
It's worth noting that training datasets often favor dominant dialects, which can lead to biases in how AI models are trained. Addressing these biases requires the consistent introduction of a more diverse set of data, ensuring a more inclusive representation of regional variations that might otherwise be overlooked.
It's clear that AI translation systems cannot remain stagnant in the face of the constantly evolving nature of slang and idiomatic expressions. The more robust the training datasets, the better they will adapt to deliver truly high-quality translations, sensitive to the nuances of context and culture.
The potential loss of a significant portion of communication nuances in poorly trained AI translation systems underscores the importance of ongoing updates based on current cultural contexts. The ultimate goal is to minimize misunderstandings and foster effective communication across various dialects. This highlights the need for researchers and developers to prioritize comprehensive training and adaptation methods to address these challenges and ensure a future where AI-powered translation accurately captures the complexities of human language.
AI-Powered PDF Translation now with improved handling of scanned contents, handwriting, charts, diagrams, tables and drawings. Fast, Cheap, and Accurate! (Get started for free)
More Posts from aitranslations.io: