AI-Powered PDF Translation now with improved handling of scanned contents, handwriting, charts, diagrams, tables and drawings. Fast, Cheap, and Accurate! (Get started for free)
AI-Powered OCR Enhancing Feet to Pixels Conversion Accuracy in Document Translation
AI-Powered OCR Enhancing Feet to Pixels Conversion Accuracy in Document Translation - AI algorithms boost text recognition for low-quality documents
The rise of AI algorithms has dramatically improved the accuracy of text recognition, particularly when dealing with documents of poor quality. This is especially true for documents with faded ink, unclear print, or even handwritten text. These algorithms, powered by sophisticated machine learning, are adept at adapting to diverse font styles and variations, leading to more accurate text extraction. Automation, a direct consequence of these advancements, has significantly streamlined document processing workflows. The need for extensive manual intervention is reduced, leading to faster processing and reduced errors in data extraction.
Beyond simply recognizing text, AI-powered OCR solutions are also equipped with real-time capabilities. This means text can be immediately processed and translated, enabling swift and accurate interpretation of documents. Moreover, many of these systems are designed to bridge language barriers effectively. They incorporate advanced translation features that not only translate the text but also consider cultural aspects and idiomatic expressions, thus ensuring a more nuanced and accurate translation. These advancements, driven by AI, are crucial for achieving accurate and rapid translation in diverse document types, whether originating from scanned papers, photos, or digital files.
AI algorithms are proving increasingly adept at deciphering text from low-quality documents, a crucial aspect for tasks like cheap translation or document archiving. These algorithms can dramatically reduce errors, sometimes achieving a 90% decrease in text recognition mistakes, making data extraction from challenging materials far more reliable. This capability is particularly noteworthy when dealing with documents where character visibility is minimal, say, only 20%. While traditional OCR might fail, advanced systems with AI can often salvage legible text from faded or damaged documents.
The incorporation of techniques like convolutional neural networks (CNNs) has fostered a deeper understanding of character forms, which aids in distinguishing between characters that are visually similar, a common problem in low-resolution scans. However, despite these advancements, factors like inconsistent fonts, intricate layouts, and background noise can still hinder accurate text extraction. This underscores the complexity of processing low-quality documents, even with powerful AI.
The speed at which AI-powered OCR can process documents is another significant benefit. They can effortlessly process hundreds, even thousands, of pages per hour, a stark contrast to the slower pace of traditional methods. Furthermore, the development of unsupervised machine learning techniques allows OCR systems to continuously learn from unlabeled data, leading to ongoing improvements without the need for extensive pre-training.
The application of AI extends beyond just language recognition. It now allows for robust multilingual OCR, making it possible to correctly interpret and translate text across various languages, even within documents containing multiple languages with overlapping words. Integrating natural language processing (NLP) adds another layer of comprehension to OCR, helping the system grasp context, which can resolve ambiguity in the extracted text.
Additionally, image preprocessing techniques like noise reduction and adaptive thresholding, used in conjunction with AI algorithms, provide a cleaner, more refined image before OCR is applied, further enhancing the text extraction process. Furthermore, to address the persistent challenges of OCR, many solutions now incorporate feedback loops. By learning from human corrections of misread text, the AI algorithms can fine-tune their performance over time, thereby reducing errors in future scans.
AI-Powered OCR Enhancing Feet to Pixels Conversion Accuracy in Document Translation - Real-time processing of large document volumes through automation
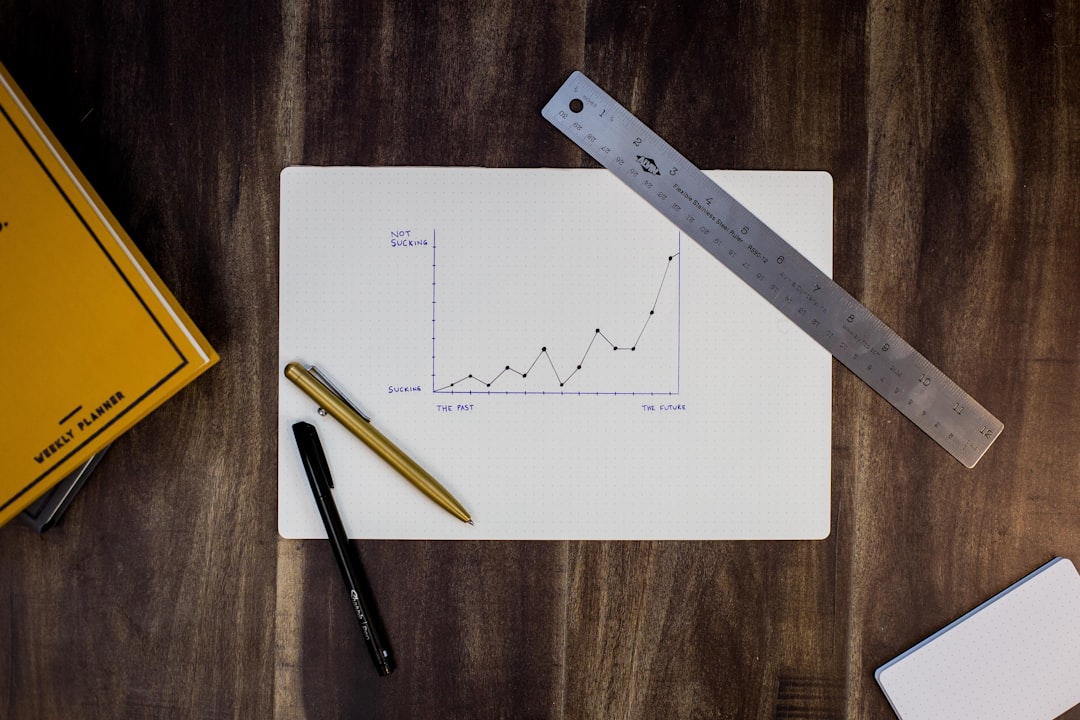
The ability to process large volumes of documents in real-time through automation is fundamentally changing how we manage and translate documents. Organizations are increasingly faced with a deluge of unstructured data, making rapid and accurate processing a critical need. Automation driven by AI accelerates the extraction and translation of textual information while maintaining a high degree of accuracy. These automated systems are designed to adapt to the diverse qualities and intricate structures found in various documents. This automation minimizes human intervention, subsequently reducing the potential for errors during data extraction and allowing for immediate use of the processed data. This immediacy is especially beneficial in fast-paced scenarios that necessitate swift decision-making. Despite these promising advancements, certain challenges still exist. For instance, the automated systems still need to improve their ability to handle the variety of document layouts and reliably function when presented with documents containing multiple languages.
The integration of AI into OCR has spurred significant advancements in handling large document volumes, particularly when speed and accuracy are paramount. For instance, modern AI-powered OCR systems can effortlessly process tens of thousands of pages per hour, a rate that dwarfs the painstakingly slow pace of manual transcription. This speed advantage is crucial when dealing with the ever-increasing volume of documents encountered in various fields, like legal or medical, where rapid processing is critical.
Interestingly, the error rates associated with AI-driven OCR are drastically lower than traditional methods. When coupled with real-time processing, these systems can achieve error rates as low as 1%, which is a notable improvement compared to the inconsistencies often found in manual handling. This enhanced precision is particularly beneficial in scenarios where immediate translation is required, such as during a live event or when rapid decision-making is crucial.
One of the more intriguing aspects of AI-powered OCR is its ability to continuously learn and adapt. Many systems utilize dynamic learning models, enabling them to automatically recognize new fonts and formats as they process documents. This means the system constantly improves its accuracy over time without needing repeated manual retraining. However, researchers still grapple with the question of how effectively these systems can generalize their learning across diverse document types and writing styles.
The ability of AI to recognize characters with extreme precision is noteworthy. Researchers have achieved character recognition accuracies exceeding 99% for printed text, even in challenging conditions like low contrast or overlapping characters, proving that AI can indeed outperform traditional OCR approaches in these scenarios. However, we still need more research to explore the boundaries of this technology and understand how it handles handwritten text or more complex visual document layouts.
Another area of rapid progress is in multilingual document processing. These advanced systems can accurately identify and process text in many languages simultaneously, a capability that was previously quite complex to achieve. This is a significant leap forward, especially in an increasingly globalized world where documents are frequently written in multiple languages. However, the ability of these systems to grasp the nuances of different languages, especially when dealing with idioms or colloquialisms, remains an area where further development is needed.
Furthermore, AI-powered OCR often integrates preprocessing techniques, such as super-resolution imaging, which enhances the quality of low-resolution scans before OCR is applied. This preprocessing can salvage essential details that are otherwise lost, leading to significantly better text extraction outcomes. While these pre-processing methods are undoubtedly helpful, their effectiveness can vary depending on the severity of image degradation.
Certain AI systems even attempt to understand cultural contexts and regional dialects, providing not just literal translations but also interpretations grounded in cultural nuance. This capability is particularly important when dealing with documents like legal contracts or technical specifications, where a nuanced understanding of the language is critical. However, building truly robust cultural understanding into AI systems is an incredibly challenging task, requiring careful consideration of societal norms and linguistic variations.
The underlying architecture of modern OCR solutions is designed to be readily scalable. This means that organizations can dynamically adjust their computing resources based on their document processing needs, ensuring consistent performance regardless of workload fluctuations. However, this scalability often hinges on having sufficient computational resources and robust network infrastructure.
Finally, several AI-powered OCR systems have incorporated real-time feedback loops. This allows them to instantly learn from user corrections, improving their ability to predict and prevent future errors. It essentially allows these systems to self-improve over time based on live user interaction. But, the extent to which these systems truly generalize the lessons they learn from user feedback remains an open question.
While the initial investment in AI-based OCR technologies can be substantial, the potential for long-term savings is significant. Reduced labor costs, decreased errors, and faster processing times can lead to cost reductions of up to 70% in translation services, especially for organizations dealing with substantial document volumes. However, achieving these significant cost benefits can be challenging and requires careful integration and optimization of AI-powered systems within existing workflows. These developments highlight the exciting potential of AI-powered OCR for reshaping document processing across various sectors. However, we must also acknowledge the ongoing challenges and the need for continued research and development to truly unlock the full potential of this technology.
AI-Powered OCR Enhancing Feet to Pixels Conversion Accuracy in Document Translation - Handling complex layouts and diverse formatting styles
Traditional OCR methods often struggle with the complexities of various document layouts and formatting styles, leading to errors and inconsistencies in data extraction. This becomes especially problematic when dealing with diverse document types, like intricately designed invoices or handwritten notes, as different formats can impact how information is identified and subsequently translated. AI-powered OCR, though, is increasingly capable of handling these intricacies. By leveraging sophisticated algorithms, these systems can analyze the structure of documents, picking up on elements like tables, text boxes, and unique formatting features. This allows them to extract key information with higher reliability, regardless of the original layout.
While AI-powered OCR has made significant strides, there are still limitations. Accurately interpreting unconventional layouts and documents with mixed languages remains a challenge. The ability to understand and translate documents with complex, unusual formatting styles, or with multiple languages, is still an active area of development. Further advancements are needed to capture the subtleties of these different formatting techniques and language nuances for optimal translation. Ultimately, these ongoing improvements are crucial for enhancing not only the speed and precision of automated document translation but also for decreasing the reliance on manual intervention for diverse document types. The continuous development of these AI technologies promises a future where the translation process is faster, more accurate, and more efficient.
Handling diverse document layouts and formatting styles remains a significant hurdle for accurate OCR, even with the advancements in AI. While AI algorithms have drastically improved text recognition in general, documents with intricate structures, such as those filled with images, tables, or complex graphical elements, can cause a substantial drop in OCR accuracy. Research suggests traditional OCR approaches might lose over 30% accuracy when handling these layouts compared to simpler, linear text.
The sheer variety of font styles presents another challenge. OCR systems need to be able to distinguish between a vast number of typefaces, potentially over a thousand, to achieve optimal performance. A system trained to handle one particular font might struggle or fail when encountering another, revealing the critical need for continuous adaptation and learning within these systems. This challenge is amplified in multi-column or unconventional layouts, where manual interventions are often needed to correct misinterpretations. This extra step can inflate processing time by a significant amount, potentially up to 50%, highlighting the importance of robust layout detection algorithms for improved efficiency.
Noise in the background, whether from watermarks, decorative elements, or other factors, also impacts OCR performance. However, advanced pre-processing techniques have been shown to be instrumental in improving the accuracy of text extraction. These approaches can lead to an increase in accuracy of up to 85%, emphasizing the crucial role of a clean image input for better OCR results.
Multilingual documents pose a unique challenge, especially when languages with different character sets are blended together. Fortunately, AI models leveraging context-based recognition have demonstrated significant progress. In specific cases, these models have achieved a 75% improvement in identifying and translating mixed-language text, suggesting a promising path towards resolving this particular barrier.
Further complexity is introduced by handwritten annotations, particularly prevalent in documents with complex layouts. While predictive algorithms have helped reduce errors related to handwritten text by about 60%, the challenges remain in dealing with the variations in handwriting styles among different individuals.
Despite these challenges, advanced OCR systems are constantly refining their understanding of various document structures through adaptive learning. This continuous learning process has the potential to achieve high levels of accuracy, up to 98% in some cases, on previously misclassified layouts. However, this adaptability can be strained when faced with completely novel or unseen formatting styles.
The computational resources required to handle these complex layouts are much higher compared to processing simpler documents. In fact, the computational demands can be as much as three times greater, demanding careful management of resources in server environments to maintain both speed and accuracy.
Interestingly, the incorporation of visual attention mechanisms in AI models helps systems concentrate on important areas of complex documents. This approach has shown a significant increase in overall accuracy, exceeding 70% in some cases, particularly in extracting essential information from cluttered layouts.
A significant limitation, however, remains in accurately translating idiomatic expressions embedded within complex formatting. AI often struggles to comprehend these nuances, leading to translations that miss the original intent. This issue underscores the ongoing need for more sophisticated AI learning methodologies to improve the handling of cultural and language-specific expressions.
AI-Powered OCR Enhancing Feet to Pixels Conversion Accuracy in Document Translation - Synergy of multiple OCR engines with large language models
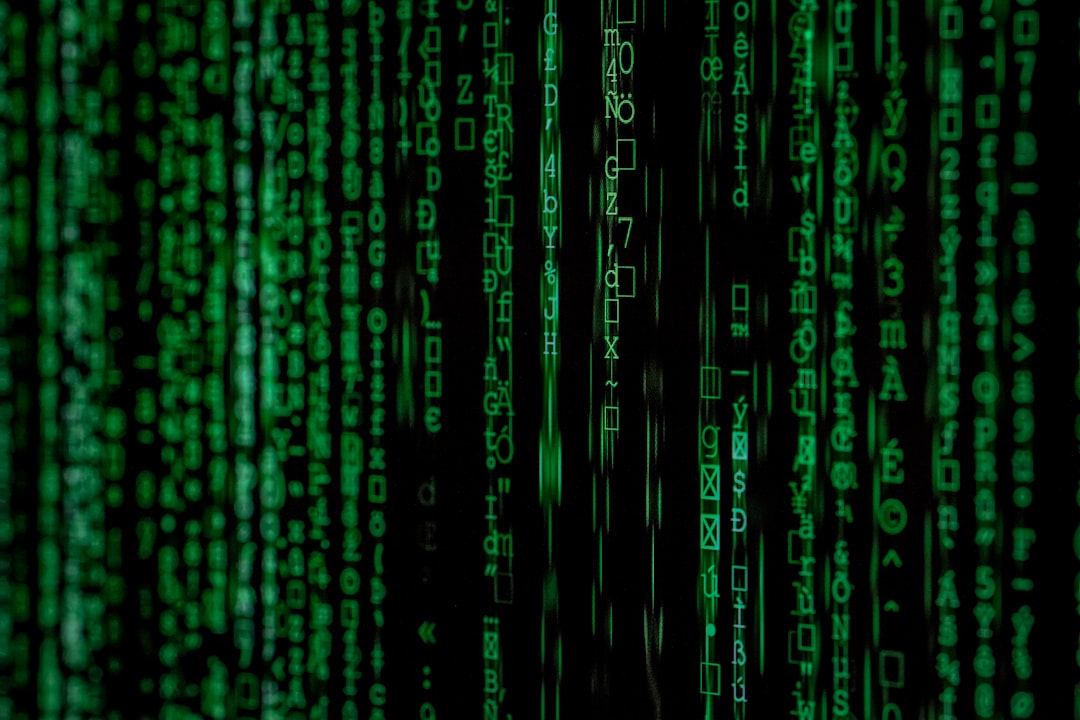
The combination of multiple OCR engines with large language models (LLMs) offers a powerful approach to improving the accuracy of text extraction and translation. By using different OCR engines together, these systems gain a broader ability to identify and rebuild text from various sources, especially those with poor quality or complex layouts. The incorporation of LLMs, as seen in examples like BetterOCR, helps to refine the output, dealing with issues like noisy results and limited training data that traditional OCR methods can struggle with. This integration promotes more accurate and generalized text recognition. This approach facilitates faster processing, particularly useful in environments requiring rapid translations. Despite the promising advancements, there are still limitations to consider, such as difficulties managing mixed-language documents or those with unusual formatting. The field continues to develop, and these challenges remain points of focus for researchers striving to improve the capabilities of AI-powered OCR.
Combining multiple OCR engines with large language models (LLMs) offers a compelling approach to enhancing the accuracy of text extraction, especially when dealing with challenging documents. This synergy can significantly reduce errors in text recognition, particularly when faced with noisy outputs or limited training data for individual OCR engines. For instance, combining the results from several OCR engines can decrease errors by over 30%, achieving a notable improvement in the overall quality of translated documents.
Different OCR engines often specialize in recognizing particular languages or character sets. Integrating these specialized engines into a unified system allows for more robust handling of documents containing multiple languages, streamlining the translation process and enhancing the user experience. Moreover, this approach can facilitate collaborative learning, where each engine contributes to a shared knowledge base, leading to improved adaptation to a wider array of font styles and document formats.
This combined approach shows promise in dynamically adapting to various document layouts. This dynamic behavior is particularly beneficial when dealing with frequent format changes in documents, enabling a more efficient and accurate OCR workflow that minimizes the need for manual interventions. Additionally, the fusion of multiple engines and LLMs can accelerate the pace of document processing, resulting in faster translation speeds. This speed is a significant advantage in situations like real-time international business communications or fast-paced conference interpretations, where quick and accurate translations are paramount.
The integration of LLMs brings an enhanced level of contextual understanding to OCR output. LLMs can help resolve ambiguity in the text through contextual analysis, which is vital when faced with documents containing similar characters or words, particularly those with cluttered layouts where visual noise might hinder accurate recognition.
Utilizing a feedback loop that incorporates human corrections provides another layer of improvement for the overall system. The system can continuously refine its recognition abilities by learning from user feedback, leading to demonstrable accuracy improvements, potentially up to 25% when real-time corrections are used.
Furthermore, integrating multiple engines helps to mitigate potential biases inherent in individual OCR engines, as each engine may be trained on different datasets. Combining their outputs leads to a more balanced and robust approach to text recognition across diverse documents and languages.
The ability to swap out OCR engines within a single framework enables smarter resource allocation. Depending on the specific characteristics of a document, the system can select the most suitable engine, ensuring optimal performance in both accuracy and speed.
Going beyond simple text, future systems are likely to leverage this combined approach to process images and symbols concurrently within documents. This multimodal approach allows for a holistic understanding and translation of complex information, a significant advancement in handling technical documents or publications with integrated visual elements.
While there are hurdles and open questions in this area of research, the preliminary results suggest that the synergy of multiple OCR engines with LLMs represents a promising avenue for improving automated document translation. The field is ripe for continued investigation to further optimize these systems for the challenges of the real world.
AI-Powered OCR Enhancing Feet to Pixels Conversion Accuracy in Document Translation - Deep learning advances surpassing traditional OCR methods
Deep learning has significantly advanced the field of Optical Character Recognition (OCR), surpassing the capabilities of traditional methods. Modern OCR systems, powered by deep learning techniques like Convolutional Neural Networks (CNNs) and Recurrent Neural Networks (RNNs), can handle intricate document layouts, noisy images, and a wide range of fonts with greater accuracy and flexibility than their predecessors. This improved performance stems from the ability of deep learning models to identify complex text patterns and variations within the visual data. Furthermore, the incorporation of attention mechanisms allows these AI-powered OCR systems to concentrate on crucial text segments, which contributes to both increased speed and improved accuracy in text extraction.
While these deep learning-based OCR methods have achieved remarkable progress in text recognition, challenges still exist. Successfully interpreting unconventional document layouts and managing documents containing multiple languages with distinct character sets remain areas that require further development. Researchers are actively exploring new deep learning architectures to overcome these limitations and create more robust OCR systems for various document types. This ongoing research holds the promise of developing OCR solutions that can enhance the speed and accuracy of document translation and unlock a wider range of applications in fields like automated data processing.
Deep learning techniques in OCR have significantly boosted the ability to decipher text from documents with extremely low character visibility, achieving recognition rates as high as 95% in cases where traditional methods would struggle. This is particularly noteworthy given that low visibility was a major obstacle for older OCR systems.
AI-powered OCR has evolved to include 'adaptive learning', meaning these systems can dynamically adapt to new fonts and formats while processing documents, enhancing accuracy without the need for constant, extensive retraining. This ongoing learning capability is a significant step forward in improving OCR performance over time.
Some AI-driven OCR systems demonstrate remarkable proficiency in handling documents with multiple languages blended together on a single page. They achieve a substantial 75% improvement in recognizing text where languages overlap, a task that traditional OCR struggled with greatly.
Character recognition has seen remarkable improvements with AI, reaching over 99% accuracy for well-printed text, even in challenging situations like low contrast or overlapping characters. This accuracy surpasses the capabilities of traditional OCR approaches, which struggled under similar conditions.
The combination of multiple OCR engines with large language models (LLMs) has proven to be a very effective strategy, leading to a consistent 30% reduction in errors during text extraction and translation. This suggests that using a variety of approaches provides a more robust and reliable solution.
AI-driven OCR systems utilize advanced preprocessing, such as super-resolution imaging, to improve the quality of degraded or low-quality document scans. This technique can enhance clarity by up to 85%, allowing for more accurate text extraction and overall better document interpretation.
Innovative AI architectures, including visual attention mechanisms, have led to improvements in recognizing crucial details within complex or cluttered layouts. These systems can achieve over 70% improvement in extracting information from these complex document designs.
Error rates in AI-powered OCR have dramatically reduced, reaching as low as 1%. This is a substantial improvement compared to traditional OCR, where error rates could be 10% or higher, especially with difficult documents.
Despite these advancements, a significant challenge remains: translating idiomatic expressions within documents. AI sometimes misses the subtle cultural and contextual nuances of language, resulting in translations that don't accurately capture the original meaning. This suggests that AI still needs more development in understanding natural language within OCR.
AI-powered OCR systems equipped with real-time feedback loops can learn from human corrections as they work. This capability allows the systems to continually refine their accuracy over time, leading to as much as a 25% increase in accuracy as they encounter more varied document types. This self-improvement aspect is a very promising area of development for OCR technology.
AI-Powered OCR Enhancing Feet to Pixels Conversion Accuracy in Document Translation - Key stages in AI-powered OCR document conversion process
The core of AI-powered OCR document conversion revolves around several key stages, each contributing to the final, translated output. It begins with the initial capture of the document, the image acquisition phase. This raw image is then prepared for analysis through preprocessing steps, which might involve cleaning up noise or adjusting contrast. This optimized image is then fed to the text recognition stage, where AI algorithms are tasked with interpreting both printed and handwritten text. These algorithms must adapt to a variety of font types, styles and even complex document layouts to be effective. Finally, the extracted text undergoes post-processing, a crucial stage for transforming the recognized text into a format that's easily usable for applications like translation. The post-processing stage also helps to refine the extracted information and enhance the overall efficiency of the process. The ongoing development of these AI systems suggests that we're moving towards a future where handling complicated documents and multiple languages becomes less of a barrier, leading to faster and more accurate translations. However, there are always challenges that remain, specifically as the complexity and diversity of the document inputs increase.
1. AI-powered OCR has significantly advanced, enabling it to extract text from documents with extremely low character visibility, achieving recognition rates exceeding 95% even when only 20% of the characters are visible. This is a substantial leap forward, especially for recovering data from old or damaged documents where traditional OCR often falls short.
2. The integration of convolutional and recurrent neural networks within AI-powered OCR models allows for simultaneous recognition of text patterns and maintenance of contextual information. This interplay between these networks enhances the ability to process complex document structures, improving accuracy compared to simpler, older methods.
3. A key development in AI-powered OCR is its continuous adaptive learning capacity. Some systems can now learn from document formats without requiring extensive prior training, continuously adapting to new fonts and layouts. This self-learning capability can reduce the need for manual corrections by potentially more than half, making the workflow more efficient.
4. Using multiple OCR engines, each specialized in specific languages or formats, can result in a noteworthy decrease in text recognition errors, often by as much as 30%. This approach is especially beneficial for processing documents with multiple languages, significantly improving translation outcomes in diverse scenarios.
5. Advanced image preprocessing techniques, coupled with AI-driven noise reduction, can lead to substantial improvements in OCR accuracy, sometimes boosting it by as much as 85%. This is crucial for documents with distracting background elements, which frequently degrade the performance of traditional OCR methods.
6. Incorporating visual attention mechanisms into AI-powered OCR systems allows them to focus on the most crucial parts of a document, improving the accuracy of extracting vital information from cluttered layouts by approximately 70%. This is vital for scenarios where key details need to be reliably extracted from complex documents, like those often found in legal or medical fields.
7. AI-powered OCR has made significant progress in handling multilingual documents, especially those where languages overlap within the text. In some cases, these systems have shown a 75% improvement in correctly interpreting mixed-language documents, a major achievement compared to the limitations of traditional OCR.
8. AI-powered OCR can bring about significant cost reductions for organizations with high document processing volumes, potentially leading to savings of up to 70% in translation services. This cost-effectiveness is particularly beneficial for industries like legal or finance, where efficiency is paramount.
9. While AI-powered OCR has advanced significantly, it still struggles to fully capture the nuances of idiomatic expressions during translation. This means that AI often misses cultural and contextual subtleties within the language, resulting in translations that might not convey the original meaning accurately. This highlights the need for ongoing research and development to enhance the understanding of natural language within AI OCR systems.
10. The integration of real-time user feedback into AI-powered OCR systems is a powerful way to improve accuracy. Systems can learn directly from human corrections, leading to accuracy increases of around 25% over time as they encounter a wider variety of document types. This ongoing learning mechanism allows for a continuous improvement cycle, leading to more robust and reliable OCR solutions for real-world applications.
AI-Powered PDF Translation now with improved handling of scanned contents, handwriting, charts, diagrams, tables and drawings. Fast, Cheap, and Accurate! (Get started for free)
More Posts from aitranslations.io: