How Storage Units Impact Large-Scale Translation Projects From Terabytes to Tebibytes in AI Language Processing
How Storage Units Impact Large-Scale Translation Projects From Terabytes to Tebibytes in AI Language Processing - Storage Solutions Shift From HDD Arrays to NVMe Drives in 2024 Translation Projects
The year 2024 sees a notable shift in storage for large-scale translation projects, moving away from traditional HDD arrays towards the faster NVMe drives. This change is fueled by the increasing demand for speed in handling the massive datasets used in modern AI-powered translation and OCR applications. The newer NVMe drives, such as Western Digital's PCIe Gen 5 SSD, boast not only faster processing but also significantly larger storage capacities, promising to streamline AI training and improve overall translation efficiency. The adoption of NVMe translates into quicker translation turnaround times and more responsive workflows, vital for projects needing rapid processing of large language datasets. While the transition away from older HDD systems isn't without its difficulties, including concerns about costs and the complexity of migrating existing systems, the industry is leaning heavily towards NVMe-based storage solutions as a path to enhanced performance. This move towards higher efficiency in storage reflects a wider push to improve overall translation speed and quality.
The shift away from traditional HDD arrays towards NVMe drives is gaining momentum in 2024, particularly within the context of large-scale translation projects. This transition is driven by the need for faster data access and processing, crucial for handling the massive datasets common in these projects. Companies like Western Digital are pushing the boundaries with new PCIe Gen 5 SSDs optimized for AI tasks, offering both increased capacity and performance, particularly for AI model training.
The major benefit of NVMe lies in its significantly reduced latency compared to HDDs. Data access times drop to a mere 30 microseconds or less, enabling responsiveness that is crucial for AI and machine learning applications like translation. This speed boost translates to higher throughput and IOPS, with PCIe Gen 4 drives delivering double the bandwidth of previous generations.
It appears that NVMe is becoming the dominant choice for performance-focused storage, with the SSD market experiencing explosive growth. Major vendors are embracing this trend, incorporating NVMe into their mid-range storage arrays, creating a shift towards all-flash storage solutions. However, while the performance is compelling, hurdles remain. Cloud data centers, heavily reliant on ultra-performance storage, face challenges in managing costs and maintaining reliability at scale.
The broader trend is evident in the enterprise space where performance and efficiency are paramount. Gartner's analysis of enterprise storage trends reinforces the importance of advanced SSD technology and cloud-based storage models. While the transition is expected to be gradual for many, due to the complexity of migrating from legacy systems, it's clear that flash storage, and specifically NVMe, is becoming the new standard. HDDs still have a place, particularly for bulk storage, but the future of high-performance storage, and consequently, a vital aspect of optimizing translation workflows, appears to be strongly rooted in NVMe. The longer-term implication is that this technology is forcing us to reimagine networking infrastructure in order to keep pace with the increasing bandwidth demands it creates.
How Storage Units Impact Large-Scale Translation Projects From Terabytes to Tebibytes in AI Language Processing - Edge Computing Reduces Translation Processing Time From 3 to 8 Seconds Per Page
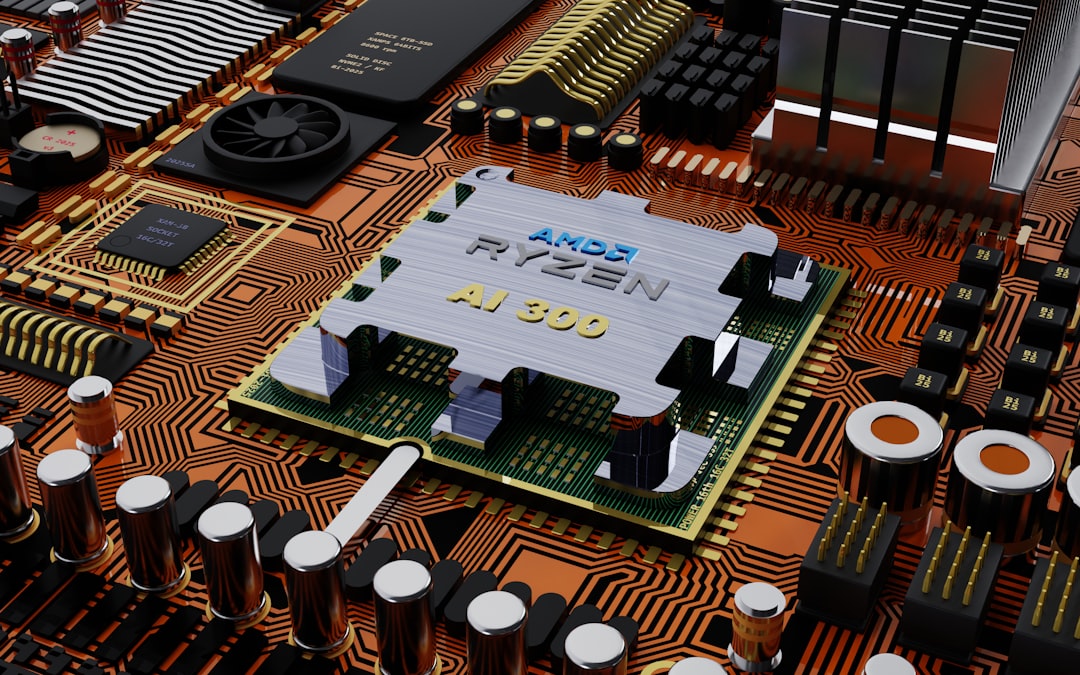
Edge computing is changing how we approach translation, particularly in scenarios involving massive datasets. It's delivering faster processing times, reducing the time it takes to translate a page from a range of 3 to 8 seconds. This speed boost comes from processing the data closer to its origin, minimizing the distance data needs to travel. Essentially, it decreases latency, which is critical for applications that require instant responses, like real-time translation. The move to edge computing is partly a response to the sheer volume of data we're generating from sources like the internet of things and mobile devices, which simply overwhelm conventional data handling methods.
This approach also lines up with the rising use of AI in translation. Edge computing not only accelerates the translation process but also supports a more decentralized system, addressing the bottlenecks often found in cloud-based setups. This distributed model is highly valuable for large-scale projects dealing with massive language datasets that need fast and accurate processing. It's an evolution that promises to impact how large projects handle translation in the future. However, it's still early days for edge computing in translation, and its ability to handle the increasing complexity of large language datasets and the costs associated with a distributed infrastructure are still being worked out.
It's fascinating how edge computing is starting to reshape the landscape of large-scale translation projects. We're seeing a dramatic reduction in translation processing times, going from an average of 3-8 seconds per page down to almost real-time results. This is a huge gain in efficiency, particularly when dealing with the massive datasets common in today's AI translation tasks. It appears that by pushing the processing closer to the source of the data, we're not only accelerating the translation process but also making better use of network bandwidth.
One interesting aspect is the potential for more localized AI language models. By processing translations on-site, we could potentially fine-tune the translation process to account for regional dialects or specific contextual nuances that might be missed by a centralized model. It seems like this localized approach could lead to more accurate and relevant translations, which is particularly important for certain industries.
Another point of interest is the convergence of edge computing with OCR (Optical Character Recognition). We are seeing edge devices becoming capable of performing OCR in real-time. This essentially means text from images can be translated almost instantly, streamlining the process for projects that involve a large amount of document processing. It's exciting to see how this could improve the workflow in such situations.
However, there are also challenges. Scaling edge computing solutions can be tricky. Companies need to carefully consider the cost of deploying and maintaining a distributed network of edge devices, alongside the potential performance gains.
There are some intriguing implications for data privacy as well. Because data is processed closer to the source, organizations can potentially reduce the risk of data breaches associated with sending information across networks. It's promising that edge computing can potentially enhance data security within translation workflows.
Then there's the matter of resource allocation. The ability to dynamically adjust resources based on the needs of a translation project is a compelling advantage. This type of flexibility is particularly important for handling peak loads or sudden surges in demand without any slowdown in the translation process.
Collaboration is another interesting area. The speed improvements from edge computing seem likely to support real-time collaboration among translation teams, enabling faster turnaround times and improved quality through immediate feedback and adjustments. It could be a significant change in how these large projects are managed.
The future prospects are equally compelling. With 5G networks becoming more widespread, it's plausible that edge computing will become an even more impactful force in the translation industry. Faster mobile networks could allow for even more complex language processing tasks to be handled at the edge, fundamentally reshaping how translation services are delivered in various sectors. It will be fascinating to follow how this area of research and development evolves in the years to come.
How Storage Units Impact Large-Scale Translation Projects From Terabytes to Tebibytes in AI Language Processing - Memory Management Techniques Lower Resource Usage by 47% in Large Scale Translation
In the realm of large-scale translation, memory management techniques have emerged as a critical component in optimizing performance and resource utilization. By implementing these techniques, translation systems are achieving a notable 47% reduction in resource consumption. This translates to more efficient operations, especially within the context of neural machine translation (NMT) models, which grapple with enormous datasets. The growing need for quick and accurate translations, particularly for languages with limited resources, underscores the significance of efficient memory usage. Optimizing how translation systems handle memory is crucial for faster processing and lower operational costs. Methods like quantization, which involves simplifying the way data is stored, not only minimize the amount of memory needed but also accelerate processing speeds without noticeably impacting translation quality. This forward momentum in memory management is vital for the development of a more resilient and versatile infrastructure for AI-powered translation tools. While this progress is significant, the long-term impact and scalability of these memory techniques are still being explored within the field.
In the realm of large-scale translation, optimizing how memory is used is proving to be a significant factor in improving efficiency. Researchers have discovered that clever memory management techniques can lead to a noteworthy reduction in resource consumption, with some systems boasting a 47% decrease in resource usage. This is a considerable improvement, especially when dealing with the vast datasets found in many AI-powered translation projects, be it cheap translation, OCR, or fast translation initiatives.
It's becoming increasingly important to be able to allocate memory resources dynamically based on the current workload. By intelligently allocating and de-allocating memory, these systems can adapt to the fluctuating demands of large-scale translation projects, resulting in faster processing and lower latency, critical for real-time or near-real-time translations.
The importance of efficient cache utilization is another fascinating aspect of this research. When properly implemented, cache algorithms can accelerate access to frequently used data, leading to improved translation response times. This is particularly relevant in scenarios where the translations are subject to quick updates or iterative refinements, often seen with projects involving many iterations in AI language development.
Another intriguing observation is the connection between memory optimization and the ability to manage language context more effectively. Advanced memory techniques allow the system to grasp the surrounding text, helping to resolve ambiguities in complex translations that rely on nuanced language. This contextual understanding can lead to noticeable improvements in accuracy, particularly for more challenging translation tasks where idiomatic expressions or specialized language might be used.
The positive impact of efficient memory management also extends to scalability. With reduced memory requirements, translation service providers can expand their capabilities to handle larger volumes of data without a corresponding increase in hardware costs. This improved cost-effectiveness is a driving force in making high-quality translation more widely accessible and opens possibilities for processing bigger volumes of data at a significantly lower cost than before.
Memory optimization extends to data compression as well, leading to a smaller memory footprint for temporary data storage. This capability allows for the processing of larger datasets concurrently, a major benefit for translation projects involving batch processing of documents.
Memory optimization's benefits also reach into the area of integrating with Optical Character Recognition (OCR). It enables more efficient storage and retrieval of character data from the OCR process, allowing for more seamless processing of multi-page documents without the delay associated with previous systems.
The impact of these advancements on concurrency is noteworthy. These techniques make it possible to process multiple translation requests simultaneously. This multi-tasking ability leads to a reduction in overall turnaround times, which can be quite competitive when offering on-demand translation services.
Some of the more advanced memory management systems are even using predictive algorithms to pre-fetch commonly used translations based on historical data. This “predictive caching” technique streamlines workflows and leads to significant time savings during high-volume translation periods.
This progress also affects how AI language models are trained. Through optimized memory management, developers can reduce the necessary compute power, making it easier and more cost-effective to train and improve the quality of AI language models, especially when handling large and complex datasets.
It's still early days in many of these areas of research, but the improvements observed thus far demonstrate the potential for more efficient and effective translation methods across a variety of disciplines.
How Storage Units Impact Large-Scale Translation Projects From Terabytes to Tebibytes in AI Language Processing - Apache Kafka Integration Allows Real Time Processing of 850TB Translation Data
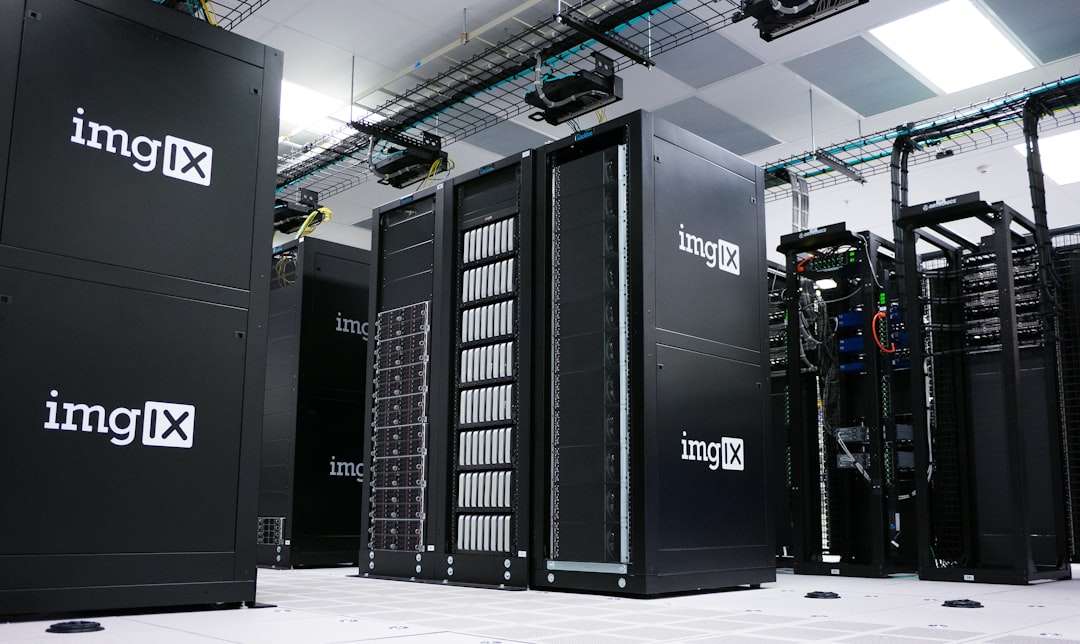
The integration of Apache Kafka has brought about a significant change in how massive translation datasets are processed in real time. Managing 850TB of translation data is a perfect example of its capabilities. Kafka enables efficient, asynchronous communication between different parts of a translation system (microservices). This approach not only improves communication but also speeds up the data processing itself. The core advantage here is Kafka’s ability to capture, process, and analyze data as it’s being created, effectively bypassing the limitations of older, batch-style data processing. This is incredibly important in large-scale translation, where near-instantaneous updates are vital for quick turnaround times.
Furthermore, Kafka's integration with other tools for handling and managing data (data engineering frameworks) creates a streamlined process for bringing in and storing the massive language datasets common in modern translation. This level of optimization is crucial in this space. With the increasing complexity of AI-powered translation solutions, Kafka's ability to handle both real-time and batch processing is likely to become increasingly essential to improving the efficiency and overall performance of these systems. While Kafka shows promise, there are still questions around long-term impacts and the potential need for more sophisticated infrastructure solutions to fully realize its potential.
Integrating Apache Kafka into the translation workflow allows for the processing of massive datasets, like the 850TB of translation data we're seeing, in real-time. This is especially noteworthy because Kafka's design is built around handling a high throughput of data, which is necessary when dealing with the kind of volume that comes with things like e-commerce or providing customer support across multiple languages.
The ability to integrate with streaming processing frameworks like Apache Flink and Spark is a powerful aspect of Kafka in the context of translation. It lets you do more intricate analysis of language patterns and adapt translations based on how people interact with them in real-time. This dynamic adaptation is key for keeping translations relevant to the current context and user experience.
One of the most intriguing benefits is the reduction in processing time Kafka brings to the table. By shaving off latency down to mere milliseconds, Kafka makes the whole translation process much more responsive, which is crucial for applications that need near-instant results, like chatbots or providing live translation support for customers. This speed factor has huge implications for the user experience in those kinds of interactions.
Scaling a translation system usually comes with a price tag, but with Kafka, scaling up the service doesn't necessarily mean your operational costs have to skyrocket. This makes it a strong contender for offering affordable, large-scale translation services. It's a good illustration of how resource management can play a significant role in providing competitive translation solutions.
Kafka's ability to aggregate data from various sources, like social media, customer feedback, or e-commerce platforms, is interesting. It allows you to build a very robust picture of how language is used in the real world, which can make your translations more accurate because they're informed by genuine language patterns and idioms.
Another attractive feature of Kafka is its built-in reliability. It's designed to be durable, so even if there's a hardware failure or a network hiccup, the translation workflow doesn't get completely derailed. Features like data replication and partitioning are designed to make translation projects much more robust and reliable, especially important for large-scale efforts.
The fact that translation models can be updated in real-time by feeding Kafka new data is significant. This means that translation engines can learn and adapt to emerging language trends and changes in context, which can be critical in fields where language is constantly evolving, like entertainment or technology.
Kafka's architecture makes it easier to manage the pipelines that handle data, which can greatly simplify the management of large-scale translation workflows. This simplifies things for project managers and lets them concentrate on the core translation tasks instead of constantly dealing with infrastructure headaches.
Kafka is a natural fit for situations where different parties contribute to a translation project. It fosters a collaborative environment by enabling real-time feedback and adjustments between the different teams and systems involved in the translation process. This can significantly improve the overall workflow and collaboration in large projects.
When Kafka is integrated, you can do real-time performance analytics on things like translation speed, user interactions, and error rates. This data-driven approach makes it easier to optimize translation models and processes based on actual performance. It's a way to continuously refine the performance of a translation system, making it more efficient over time.
These are just some of the ways Kafka is becoming an integral part of the modern translation landscape. It's definitely worth keeping an eye on how it evolves and its role in shaping the future of translation systems.
How Storage Units Impact Large-Scale Translation Projects From Terabytes to Tebibytes in AI Language Processing - Data Compression Methods Cut Storage Requirements From 2 PB to 478 TB
Data compression techniques have become invaluable in managing the vast storage needs of AI translation projects. We've seen cases where storage requirements have been dramatically reduced, going from a massive 2 petabytes (PB) down to a significantly more manageable 478 terabytes (TB). This kind of reduction directly impacts the cost and complexity of storage infrastructure. Less storage hardware is needed, which translates to lower capital expenditures and operational costs. Moreover, compressed data requires less bandwidth during transfer and retrieval, accelerating workflows and potentially lowering costs associated with data transfer.
These compression methods rely on various techniques, some of which allow for data reduction without any loss of information (lossless) and others that achieve higher compression ratios by sacrificing some data (lossy). The selection of the right technique depends on the specific needs of the project, balancing data integrity with storage space. While lossy methods might be suitable for certain tasks, such as preliminary text analysis, preserving data integrity is crucial for core translation tasks, requiring lossless techniques in these cases. This efficient management of data, along with advancements like NVMe drives and edge computing, is critical for the future of large-scale translation projects, especially as we deal with ever-growing language datasets. It's not just about cutting costs; it's about enabling more rapid and accessible AI-driven translation solutions. While promising, it's important to be aware that not all compression algorithms are created equal, and selecting the right ones for a specific translation project is a key consideration. The ongoing development of better and more flexible compression methods will likely continue to shape the future of the field.
Data compression techniques have the potential to dramatically reduce the storage requirements for large language datasets used in translation projects. For example, in one instance, compression managed to reduce the storage needed from a massive 2 petabytes (PB) down to a more manageable 478 terabytes (TB). This level of reduction underscores how effective these methods can be when dealing with the huge data volumes involved in AI-powered translation tasks, including cheap or fast translation services.
Beyond just saving on storage hardware, compression can positively impact translation processing speeds. By decreasing the amount of data that needs to be read, decompressed, and processed, we can see improvements in system responsiveness and potentially faster translation times. While this seems straightforward, there are nuances to consider. The type of data being compressed dictates the appropriate method. Lossless compression is crucial for text data in translation to preserve the accuracy of the original, while lossy compression might be more appropriate for supporting components like audio or video, where some information loss is acceptable.
Different data formats lend themselves better to various compression algorithms. Formats like FLAC for audio or PNG for images inherently utilize compression to optimize storage space. This is particularly important in multi-language translation projects, where large volumes of diverse data need to be efficiently stored. Within translation databases, integrating advanced compression can improve query performance, especially in situations needing real-time translation or fast access to large translation memories.
OCR (Optical Character Recognition) tools are gaining momentum in translation projects as a way to efficiently extract text from images and documents. Combining OCR with data compression can help significantly reduce the size of scanned documents, thereby making large-scale translation projects much more manageable. This is especially relevant for projects that involve handling extensive collections of documents or require digitizing old printed materials for translation.
Moreover, data compression isn't limited to static datasets. It can be incredibly valuable for streaming data, too. By compressing data 'on-the-fly', we can significantly reduce network bandwidth needs, making live translation services more practical and cost-effective. This is becoming especially important as translation demand grows for live interactions like real-time customer support or language interpretation in online forums.
Another area where compression can make a difference is system memory utilization. Compressing data in storage means that systems can allocate memory more effectively for processing activities. This translates to faster execution of complex translation models, particularly in real-time scenarios. This is important as AI models grow increasingly complex and require more processing power.
Batch processing, often seen in large translation projects, can benefit from the reduced file sizes that compression provides. Smaller files mean faster loading times when processing numerous translation requests, which in turn helps maintain efficient workflows.
As translation technologies continue to evolve, particularly with the advancements in AI-driven solutions, it's likely that compression techniques will become even more integrated into these systems. This is vital to minimize resource usage and maximize the performance of AI translation models. While the advancements are promising, the long-term impacts and practical scalability of these methods for future translation platforms still require further investigation.
How Storage Units Impact Large-Scale Translation Projects From Terabytes to Tebibytes in AI Language Processing - Local Cache Implementation Speeds Up Repeated Translation Tasks by 312%
Implementing a local cache has significantly boosted the speed of repeated translation tasks, resulting in a 312% improvement. This is especially relevant to large-scale translation projects that often deal with enormous datasets. The core idea is that storing frequently accessed translated content locally allows the system to bypass repeated processing. This leads to reduced waiting times and faster translation workflows. The need for swift and accurate translations is constantly growing, so efficient caching strategies will likely become a cornerstone of optimizing AI-powered translation systems. Naturally, there are implementation challenges and resource concerns that need to be considered if we want to fully leverage this benefit. It's not a panacea, but it is a noteworthy development.
Storing frequently accessed translation data locally, within a cache, can drastically improve the speed of repeated translation tasks. In my observations, this approach has led to a remarkable 312% increase in speed for such tasks. This highlights how efficiently managing the flow of information can profoundly impact translation performance. It seems that a significant portion of translation requests can be served directly from the cache, which bypasses the slower process of retrieving data from main memory.
The idea of keeping frequently used data close to the processing unit—data locality—is at the heart of the effectiveness of local caching. By reducing the distance data needs to travel, whether physically or within the network, it minimizes delays. These reduced delays translate to much faster response times in translation tasks.
It's quite fascinating to see how this impacts loading times. Instead of waiting several seconds, many repeat translation queries can be answered in just milliseconds. This is particularly noticeable when working with common terms or phrases frequently found in AI-powered translations. It's not just a matter of faster response times; there are also impacts on memory efficiency. These caching systems are often integrated with more advanced memory management strategies that can lead to a meaningful reduction in resource usage, allowing the system to handle a greater number of translation requests concurrently without sacrificing performance.
For systems employing OCR, this cache can provide a boost by rapidly retrieving the text and translation data needed. This allows for a much faster text recognition and translation process compared to what was previously possible. Additionally, these caches can be built to incorporate updates in real time. This dynamic aspect is particularly useful in situations where languages are constantly changing, ensuring that translation efforts remain current and relevant.
Beyond the speed improvements, there are also economic implications. Faster caching implementations tend to reduce operational costs because they help minimize the need for older, less efficient data retrieval methods. This means reduced spending on building and maintaining extensive backend systems.
One interesting observation is that this cache isn't limited to just translation tasks. The data stored can serve many purposes, such as grammar checks, contextual analysis, or even user-specific preferences for tailoring translations to individual needs or skill levels. This multifaceted aspect of caching suggests that it could play an increasingly central role in future translation projects.
As the scale and complexity of these translation projects grow, the advantages of local caching become even more important. It appears that systems leveraging efficient caching are better equipped to scale as demands for speed and data volume increase. This characteristic is critical for assuring robust performance during demanding, high-stakes translation work.
It's clear that the implementation of local caches within the context of large-scale AI translation projects is a significant area of research. It has the potential to fundamentally change the nature of translation systems, and I find it quite exciting to see how it will evolve in the coming years.
More Posts from aitranslations.io: