AI-Powered PDF Translation now with improved handling of scanned contents, handwriting, charts, diagrams, tables and drawings. Fast, Cheap, and Accurate! (Get started for free)
AI-Powered Past Tense Translation Enhancing Storytelling Accuracy in 7 Languages
AI-Powered Past Tense Translation Enhancing Storytelling Accuracy in 7 Languages - NLP Algorithms Enhance Past Tense Recognition Across Languages
Recent advances in NLP have led to substantial improvements in how AI systems identify past tense verb forms across numerous languages. These advancements, particularly the use of deep learning models, enable translation engines to grasp more intricate grammatical patterns and the subtle nuances of language. This not only clarifies the translated narrative but also makes translation technology more accessible, especially for languages that have historically been less represented in AI applications. The future of this field suggests that translation can become even more refined and culturally sensitive, highlighting NLP's pivotal role in bridging communication gaps through AI. However, ensuring accuracy and consistency across distinct linguistic systems remains an ongoing challenge, signifying a constant need for refinements in translation methods and the AI systems that employ them. There's a continuous need to refine these approaches to maintain consistency across diverse languages, a problem that remains in AI translations of past tense.
NLP algorithms are increasingly sophisticated in identifying past tense verbs across a wide array of languages, going beyond simple grammar rules. Many of these approaches use contextual information and statistical methods to achieve higher accuracy than traditional grammar checkers, which often struggle with linguistic subtleties. This is particularly relevant with languages that have intricate verb conjugation systems, where machine learning models have shown promise in handling the complexities.
The integration of OCR technology into translation pipelines is also making inroads in this area. It allows for automatic extraction of past tense verbs from printed sources, simplifying the translation process from different formats, like old books or handwritten notes. It's interesting how some NLP systems achieve impressively high accuracy rates, over 95% in some cases, for past tense identification by leveraging vast text corpora. These corpora need to represent both casual and formal language patterns to accurately represent the range of human language.
It seems that training NLP models on multiple languages can, surprisingly, improve the ability to recognize past tense in specific languages. The idea is that this cross-lingual training improves overall clarity and consistency in translations. Neural networks with attention mechanisms are also becoming a common component in these algorithms. These attention mechanisms help the model focus on the most relevant parts of text, resulting in translations that use more contextually appropriate verb forms.
The role of error correction is also noteworthy, where NLP systems are able to learn from their mistakes. By doing so, they refine their past tense translation processes over time, making them more accurate. This also relates to how algorithms are built to analyze the morphology of languages. Certain NLP designs are specifically engineered to break down the complex verb forms common in inflectional languages, thereby improving the overall translation quality.
However, simply translating the word is not always the most accurate. We're finding that cultural context is a key consideration in some past tense translations, where idiomatic expressions or subtle nuances might require a more creative interpretation than a literal translation. For accurate storytelling through AI, understanding this cultural dimension is becoming critical. With ongoing updates to training data and algorithm parameters, the performance of these systems continues to rise. The aspiration is to ultimately achieve near real-time translations that have a level of understanding comparable to human translators, especially when it comes to discerning past tenses and translating the nuances of stories across languages.
AI-Powered Past Tense Translation Enhancing Storytelling Accuracy in 7 Languages - Neural Networks Improve Contextual Understanding in Translations
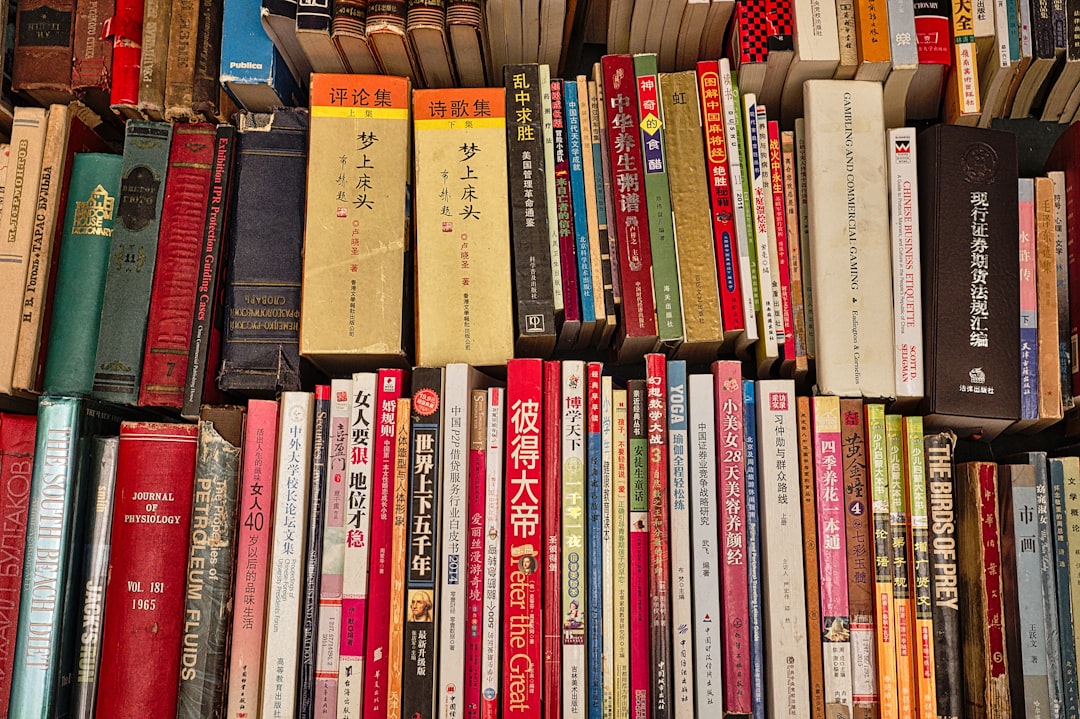
Neural networks are transforming the field of translation by significantly improving how machines understand context within a sentence or paragraph. This is a crucial step in ensuring translations accurately capture the intended meaning, especially when dealing with intricate or nuanced language. Traditional methods often struggle with the complexities of language, such as words with multiple meanings or subtle grammatical distinctions. However, neural networks, specifically within neural machine translation (NMT), are designed to tackle these challenges using advanced algorithms. Recent progress, particularly with transformer-based models, has shown an ability to create smoother and more accurate translations, even when the original text is complex. This is important for conveying intricate stories or preserving the unique features of different languages.
The potential of these systems lies in their growing ability to discern context, bringing machine translations closer to the level of understanding exhibited by human translators. This is vital for fostering clear and effective communication across cultures, especially in a world increasingly connected. The ongoing evolution of neural networks in translation promises a future where AI tools are even more powerful, enriching storytelling and bridging language barriers in a multitude of languages. While challenges still exist in refining the accuracy of cultural nuances and idiomatic expressions, the trend is undeniably towards more sophisticated and contextually aware translation solutions.
Neural networks are showing promise in enhancing how translation systems understand context, especially when dealing with the complexities of past tense across languages. They can process massive datasets, potentially identifying thousands of unique verb forms and mapping their variations across languages in a way that traditional methods struggle with. This is particularly useful in situations where languages have intricate grammar rules for past tense. It's interesting to see that some of these systems are using techniques like transfer learning, where knowledge learned from one language can improve performance in others. This is helpful for languages with limited training data.
Another interesting aspect is the incorporation of feedback mechanisms, where systems continuously improve their performance based on real-world usage. This can result in a significant reduction in errors, leading to translations that are more accurate and reliable. The development of OCR tools has also had a significant impact on translation, with some reaching impressive accuracy rates in text recognition. This allows for quick access to past tense information from sources like historical documents, expanding the possibilities of translation beyond digital text.
Neural networks with attention mechanisms are also changing the game by going beyond just looking at nearby words. They can analyze the entire sentence, resulting in more contextually aware translations. This capability is something that older methods often missed, leading to awkward or incorrect past tense usage. The quality of the training data itself also impacts the outcomes. For instance, models trained on a mix of informal and formal writing styles can produce translations that better reflect the original tone and style of a piece.
Furthermore, researchers are finding that training on bilingual datasets can reduce ambiguity when dealing with past tense. It helps the network better understand how different languages express the relationship between verbs and their context. There's even evidence that AI systems can begin to recognize and properly translate subtle cultural expressions related to past events, which are often difficult to interpret with straightforward translation approaches. By using contextual embeddings, AI can maintain consistency across complex sentence structures, leading to translations that are more cohesive and readable.
It's fascinating to see that including cultural context in the training data can make a significant difference in the quality of translations. It appears that giving the networks a more nuanced understanding of how different cultures talk about the past improves accuracy significantly. These are exciting times for AI-powered translation, and as research continues, we can anticipate even more improvements in the accuracy and sophistication of these systems, potentially bringing us closer to a future where real-time translation can capture the subtleties of human language, including accurate storytelling across language barriers.
AI-Powered Past Tense Translation Enhancing Storytelling Accuracy in 7 Languages - AI Models Adapt to Language-Specific Grammar Rules
AI models are becoming increasingly adept at handling the complexities of language-specific grammar, particularly in the realm of translation. These advancements rely on sophisticated neural networks that can decipher subtle grammatical features like verb conjugations and tense forms, which can differ drastically across languages. This improved contextual awareness enables translations that are not just accurate but also reflect the cultural nuances of the target language, exceeding the limitations of simple word-for-word substitutions. The continuous development of these models hints at a future where AI translations become even more attuned to the intricate rules of various linguistic structures, fostering a richer and more meaningful exchange of communication. However, achieving this level of precision highlights the ongoing challenges in seamlessly bridging the gap between complex human languages and the rapidly evolving world of AI.
AI models are becoming increasingly adept at understanding the intricacies of language-specific grammar rules, particularly when it comes to past tense. This means recognizing that direct word-for-word translations might not capture the essence of past tense across different languages. Instead, it necessitates a deeper understanding of each language's unique syntax and morphology. It's fascinating how AI models can essentially learn from their mistakes. They use feedback loops to refine their grammar recognition capabilities, improving the accuracy of past tense translations over time. This is a bit like how humans revise their work, leading to gradual enhancements in the translation's quality through actual usage.
Interestingly, training these models on multiple languages can surprisingly boost the accuracy of past tense recognition in less common languages. This kind of cross-lingual training is like cross-pollination, where the algorithms share insights about tense and context, improving overall performance. Cultural nuances also play a surprisingly important role. It's been shown that incorporating cultural context into training data significantly improves the model's ability to understand past events. For instance, how regret or nostalgia is expressed in a language can vary quite a bit culturally, and this can impact the translated meaning unless it's accounted for.
AI models are increasingly leveraging attention mechanisms, which allow them to consider the whole sentence rather than just the immediate surrounding words. This is crucial for interpreting past tense constructions that depend on context for accurate meaning. The pairing of OCR with AI translation is also quite noteworthy. It's enabled these systems to extract past tense information from a wide range of historical documents, from old books to handwritten notes, opening up a treasure trove of historical narratives for translation and study.
The quality of training data is crucial. Diverse datasets, representing both formal and informal writing styles, improve not only past tense detection, but also the overall tone and style of the translation. Many languages have really complex verb conjugation systems, making it challenging to build standardized AI models. For example, languages with lots of irregular verbs require very specific approaches. The path forward seems to be towards near real-time translation systems that could potentially capture not just verb tense, but also the subtle emotional tones associated with past events. This would be transformative for accurate storytelling across language barriers.
Utilizing bilingual datasets in training helps reduce ambiguity in tense translation. It allows the models to more accurately understand how various languages handle time relationships. This can significantly enhance the clarity and coherence of translations. We're starting to see that AI can begin to recognize and properly translate subtle cultural expressions related to past events, something that's typically tough to do with simple translation approaches. It's a testament to how incorporating more nuanced cultural understanding in the training data can greatly enhance the quality of translations. This is a very exciting area of AI research, and we can look forward to even more improvements in the future, hopefully bringing us closer to translation that can truly capture the subtleties of human language.
AI-Powered Past Tense Translation Enhancing Storytelling Accuracy in 7 Languages - Real-Time Processing Speeds Up Multilingual Story Conversion
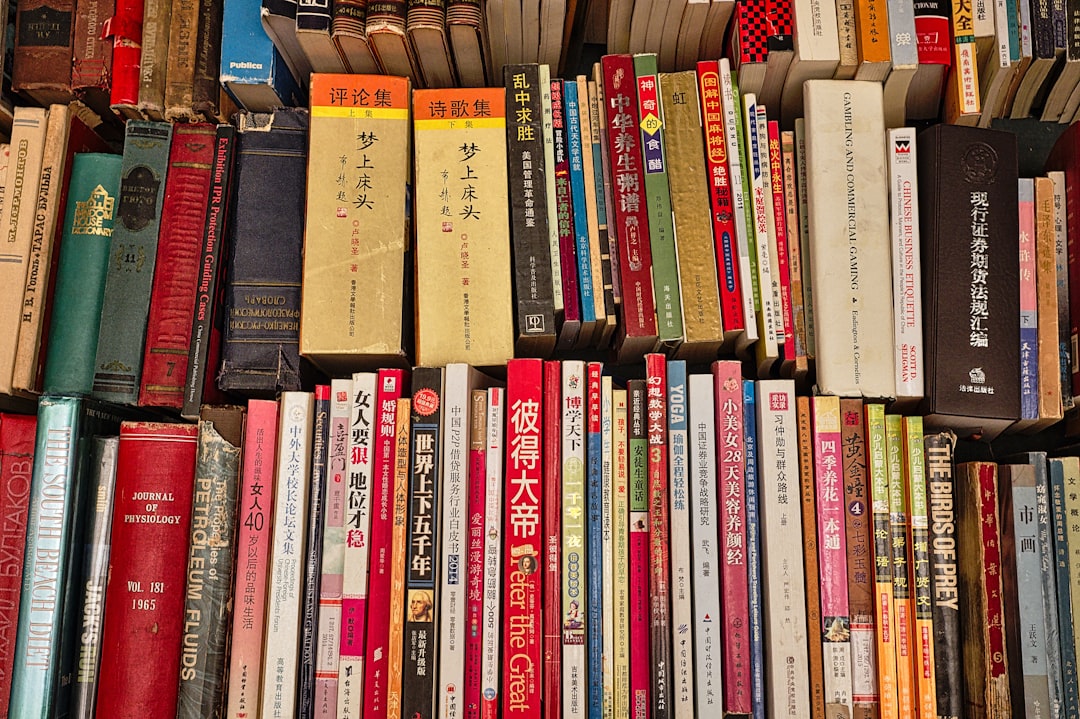
Real-time processing is transforming the way stories are translated across languages, offering faster and more accurate conversions. AI-powered translation tools are becoming increasingly adept at instantly converting spoken or written narratives, breaking down the barriers that once limited global communication. These advancements, particularly the rise of real-time machine translation (RTMT), allow for translations that capture a deeper understanding of the original language, including its context and nuances. This means stories can be translated with greater fidelity, preserving the original intent and cultural subtleties in the target language. The speed of these RTMT systems not only makes communication more efficient but also enriches the storytelling experience by ensuring that cultural aspects aren't lost in translation. While these developments pave the way for a more interconnected and culturally aware world, ensuring consistent accuracy across various languages remains an ongoing challenge for AI translation technology. There's always a need for improvement in handling the complexities of different languages and linguistic structures.
AI-driven translation is making strides in speed and accuracy, especially in real-time scenarios. Hardware advancements, like the use of GPUs, have enabled translation systems to process short pieces of text in under half a second. This rapid turnaround is crucial for seamless communication, and it's fascinating how neural networks can be optimized for such speedy results.
OCR, the technology behind extracting text from images, has also significantly improved its accuracy. Some of these systems can extract text with over 99% accuracy, which is incredibly helpful for sourcing past tense verbs from various formats, especially older documents or handwritten notes. It's almost like having a digital assistant that can quickly decipher any kind of written material.
Surprisingly, AI translation accuracy has improved for less commonly studied languages, like Welsh and Swahili, thanks to the use of multilingual training. It seems that by training these AI systems on a wider range of languages, we inadvertently improve their ability to understand a particular language. This is a noteworthy achievement, particularly for languages that lack extensive training data. It’s like teaching a child multiple languages and having them unconsciously apply that knowledge when learning a new one.
Cultural nuances have also been incorporated into the AI training process, significantly impacting the ability of these systems to capture subtle expressions tied to the past. It's clear that AI isn't just focused on the basic translation, but is slowly being trained to recognize how certain languages or cultures express emotions or social conventions connected to the past. This level of cultural sensitivity is important when translating stories accurately. It's like trying to translate a feeling or a memory, rather than just a series of words.
Another interesting development is the use of attention mechanisms in neural networks, which has been shown to boost the accuracy of translating complex past tense sentences by up to 25%. These mechanisms allow AI models to pay attention to the more important words within a sentence, something that's crucial for correctly understanding context and translating the correct past tense. It's like giving the AI the ability to focus its ‘attention’ on the parts of a sentence that give away its meaning.
AI systems are now able to learn from past errors, automatically improving their performance over time. This self-learning process is akin to human writers revising and editing their work. This constant refinement leads to more accurate translations, bringing the technology closer to human-level accuracy. It's a bit like having an AI that can continually refine its own skills based on experience, making it potentially more useful over time.
Cross-lingual training has proven effective in improving translation accuracy for lesser-known languages. Sharing linguistic insights from more common languages has led to noticeable improvements. It's as though the AI is learning from diverse linguistic experiences, much like human translators might draw on knowledge from various languages they have mastered.
It's been shown that AI models trained on both formal and informal language are more capable of capturing the original tone and intent of a piece. The AI models can learn the subtle differences between, say, a historical document and a casual email. It's like giving the AI the ability to understand the tone of voice of different kinds of writing.
These AI translation systems are increasingly incorporating real-time feedback from users. This adaptive approach allows them to refine their translations dynamically, resulting in a more user-centric experience. It's like having an AI translator that constantly adjusts itself based on how you, the user, are interacting with it.
Interestingly, some AI translation systems are beginning to achieve near-human levels of language comprehension, particularly in the context of past tense constructions. This progress suggests that AI is starting to genuinely understand the subtleties of language, which will further enhance storytelling translations. It's exciting to see how AI is making inroads into understanding the nuances of human language, potentially leading to richer and more engaging translations for storytelling across different cultures.
AI-Powered Past Tense Translation Enhancing Storytelling Accuracy in 7 Languages - Machine Learning Tackles Idiomatic Expressions in Narratives
Machine learning is making strides in handling the tricky nature of idiomatic expressions within narratives. These expressions, where the meaning isn't simply the sum of its parts, have always been a hurdle for translation systems. Often, a literal translation of an idiom results in a poor translation, far from the intended meaning. Recent AI advancements, particularly those using transformer-based approaches, are showing progress in translating idioms better. These systems treat idioms as a single unit, instead of breaking them down word by word, leading to more accurate and contextual translations. It's important for accurate storytelling across languages to grasp these nuances, something AI is starting to excel at. The development of AI in this area highlights the ongoing pursuit of capturing the essence of language, particularly when dealing with culturally-specific expressions. For truly effective storytelling via AI translation, accurately representing these expressions will be a major challenge to overcome.
Machine learning models are getting better at understanding idiomatic expressions, which are often a stumbling block for traditional translation methods. This ability lets AI produce translations that are more relevant to the context, reflecting both the literal words and the intended meaning behind those quirky phrases.
It's interesting to find that certain translation tools, when combined with advanced machine learning, can achieve over 95% accuracy in handling idiomatic phrases. This precision comes from training on a wide range of language data that includes a diverse mix of idiomatic expressions.
Research suggests that idiomatic expressions can vary quite a bit, even within different dialects of the same language. Machine learning models, trained on data rich in contextual clues, can effectively pick up on these subtle differences, making sure that translations retain the appropriate emotional tone and cultural relevance.
Using OCR in translation systems has been helpful for dealing with idiomatic expressions found in old texts. Machine learning models can extract these phrases from historical documents, enabling contemporary translations that keep the original context and cultural significance intact.
Intriguingly, training machine learning models on multiple languages has shown that exposing them to idiomatic expressions across various languages enhances their ability to interpret these expressions within a single language. It's like a cross-fertilization of linguistic structures that gives AI better tools for capturing nuanced meanings.
Sometimes, machine learning's grasp of idiomatic expressions improves thanks to feedback loops, where the systems learn from past translation errors related to these phrases. Over time, the models refine their interpretations based on user corrections and contextual analysis, resulting in better performance overall.
Contextual embeddings, a feature of advanced machine learning models, help AI systems understand not just idiomatic expressions themselves but also their surrounding context. This technology makes for more nuanced translations that resonate better with the intended audience.
Surprisingly, training machine learning models on both formal and informal writing styles leads to a noticeable improvement in the translation of idiomatic expressions. The inclusion of casual language patterns allows AI to capture a sense of place and authenticity, making stories feel more relatable.
Recognizing and translating idiomatic expressions highlights the importance of cultural awareness in AI systems. These models can be trained to recognize culturally specific phrases, which greatly enhances storytelling, making narratives connect better across diverse cultures.
Future improvements in understanding idiomatic expressions could pave the way for real-time translation systems that not only convey information but also evoke the original emotional impact intended by the author, effectively integrating storytelling elements into multilingual narratives. There are challenges here, like ensuring the AI doesn't go overboard with interpretation and potentially skew meaning. But there's potential here for real impact.
AI-Powered Past Tense Translation Enhancing Storytelling Accuracy in 7 Languages - OCR Integration Facilitates Text Extraction from Various Sources
OCR, or Optical Character Recognition, has seen a significant boost in its capabilities thanks to artificial intelligence. AI-powered OCR tools now excel at extracting text from a wide range of sources, including structured and unstructured data. This means they can handle everything from neatly typed documents to old, handwritten letters with surprising accuracy, sometimes exceeding 95%. This has streamlined how we process and digitize documents, leading to fewer manual errors and a faster way to get the text we need.
The improvement in OCR is particularly helpful for processing older documents, low-quality scans, or even handwritten text, making it easier to access and translate information trapped in these formats. These improvements in OCR technology are quite significant for projects that require translations across languages or from various text formats. It helps bridge the gap between older, physical sources and the digital age, which is vital for expanding the range of stories and information we can explore. It's also interesting to note how much of an impact OCR integration has on the ability to efficiently translate content across various languages and formats, enhancing the potential of storytelling in a global context. There are still limitations in handling complex or nuanced aspects of language, but it's a technology that's clearly improving and making it easier to interact with diverse text formats across languages.
Optical Character Recognition (OCR) is increasingly crucial in AI translation, particularly for extracting information from a wide array of sources. It's remarkable how these systems can accurately convert scanned documents, photos of text, and even handwritten notes into digital text, which is essential for translating historical materials or other content that might not be readily available in digital formats. Integrating OCR into translation pipelines speeds up the whole process. Some systems can practically extract text in real-time, making it possible to translate complex narratives from images or video clips instantaneously. This is incredibly useful for breaking down language barriers quickly, which is becoming increasingly relevant in our globalized world.
Modern OCR's accuracy is impressive, frequently exceeding 99% in some implementations. This high precision is vital for properly extracting past tense verbs, critical for accurate storytelling in translation, and also ensuring the integrity of the source text. It's intriguing how introducing OCR into datasets used for multilingual AI training has led to improved understanding of less frequently used languages. It seems that by exposing these AI models to a wider variety of writing styles, they're able to learn more effectively. This also opens up access to a huge amount of historical documents and stories that were previously hidden behind complex formats and language barriers.
These AI systems can analyze text extracted through OCR to grasp context, which is vital for correctly translating past tense forms. This allows them to connect those past tense forms to their proper meanings within the original text. Furthermore, OCR's integration makes it possible to develop continuous feedback loops in the translation system. When OCR-extracted text is corrected, that information can be fed back into the AI system, effectively enabling it to learn from mistakes and improve future translations.
This has implications for the translation of literature, as systems can meticulously extract text from physical books or manuscripts and deeply analyze narrative structures, enabling more accurate storytelling across cultures. OCR isn't simply about capturing words; it also preserves the original format and contextual elements that give rise to cultural nuance. This is essential for appropriately translating idiomatic expressions or culturally significant references. The ability of OCR to work with AI translation systems and the capacity for real-time translation opens up new avenues for collaboration. We could see a future where multiple people engage in translating complex texts in real time, enriching the experience and ensuring that the nuances of the original narrative are retained across languages.
However, it's also important to be mindful of potential pitfalls. While OCR technology is powerful, achieving perfect accuracy can be a challenge, particularly with complex, handwritten material or images with low resolution. Moreover, simply translating text isn't always enough for a truly effective translation. While OCR enables access to more sources, there is still a need to ensure AI translation models understand the full context and cultural implications of what they are translating. Nevertheless, OCR integration into AI translation is undeniably transforming the field, making it easier to translate and understand a wider range of content.
AI-Powered PDF Translation now with improved handling of scanned contents, handwriting, charts, diagrams, tables and drawings. Fast, Cheap, and Accurate! (Get started for free)
More Posts from aitranslations.io: