AI-Powered Translation Analytics Revolutionizing Telecom Customer Support Efficiency
AI-Powered Translation Analytics Revolutionizing Telecom Customer Support Efficiency - Real-time Language Detection Streamlines Multilingual Support
The ability to instantly identify the language used in customer interactions is revolutionizing how businesses handle multilingual support. AI is making this possible by automatically recognizing languages in real-time, instantly opening the door for translation and facilitating smoother interactions across language barriers. Imagine a global meeting, where participants speak different languages – real-time transcription and translation, powered by AI, makes those interactions fluid. This technology, once expensive and complex, is becoming more commonplace and budget-friendly. Consequently, organizations can streamline their customer service operations, improve clarity among teams with international members, and minimize misunderstandings in interactions with a global customer base. The move towards real-time language support is fundamentally altering global communication by improving efficiency and bridging language gaps. While this tech is still evolving, it's clear its impact on global customer service will only increase in importance.
Real-time language detection, a fascinating development in AI, is making strides in facilitating multilingual support. We're seeing accuracy rates in language identification from audio nearing 95%, which is crucial for promptly initiating translations and tailoring customer service interactions – information valuable in evaluating overall customer happiness. The capacity of these systems to handle hundreds of languages simultaneously is remarkable, particularly for large telecoms managing customers globally.
The integration of OCR has added another dimension, expanding the scope of translation to text-based documents in numerous languages at impressive speeds. While the speed of 90 characters per second is promising, the accuracy of the OCR process itself, especially with handwritten documents, still needs further research and improvement. The positive impact on operational efficiency is evident: reports indicate that businesses using real-time AI translation can cut customer response times by up to 30%.
However, the complexity of language presents ongoing challenges. Dialect recognition, for example, remains a hurdle. While progress is being made, accurately understanding the nuances of regional language variations is still a key focus area. Interestingly, some translation systems are now beginning to incorporate elements of tone and context analysis into their processing. The potential of systems identifying subtle emotional cues in customer interactions and providing more responsive service interactions is intriguing.
Financially, the use of AI translation services seems compelling. They present the possibility of reducing translation costs by a significant margin when compared to traditional methods. Although, the quality of AI translation, while continuously improving, may not always match human translations for particularly sensitive communications. It's also noteworthy that these systems can learn from previous interactions, refining their performance over time. They can analyze recurring errors and adjust algorithms for better performance.
One intriguing aspect of language detection is the possibility of offline operation, which could be advantageous in areas with inconsistent internet access. This could enable basic real-time support, offering accessibility in remote locations. This kind of offline capability hints at future applications, especially in scenarios involving mobile support or emergency communications. Overall, the ability to handle diverse cultures and languages is enabling companies to broaden their customer support reach and tap into previously underserved markets that were often hindered by language barriers. It seems that the intersection of AI and language processing is poised to greatly reshape the future of communication and service across the globe.
AI-Powered Translation Analytics Revolutionizing Telecom Customer Support Efficiency - OCR Technology Enhances Text Extraction from Customer Documents
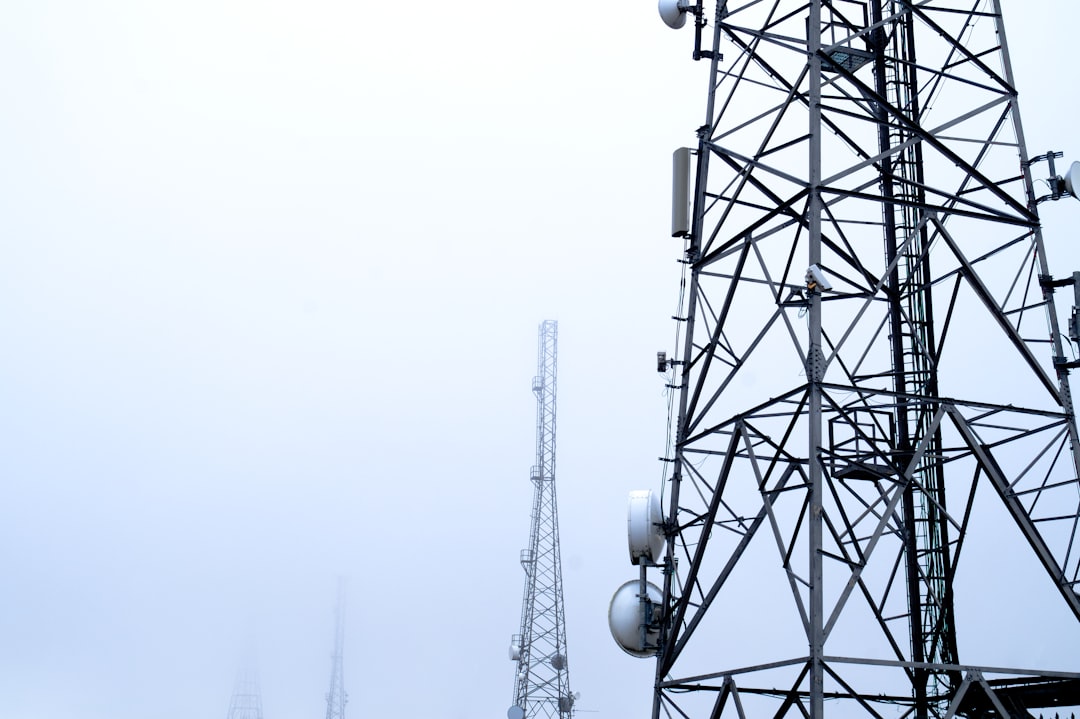
OCR (Optical Character Recognition) technology is proving increasingly valuable for extracting text from customer documents, a crucial aspect of streamlining operations, especially in telecommunications. By converting scanned or image-based documents into editable and searchable text formats, AI-powered OCR systems allow businesses to quickly process customer information, leading to faster responses and better service. The capability of these systems to manage diverse document types and numerous languages significantly expands their applicability within customer support environments. Nonetheless, ongoing improvements are needed to ensure accuracy, particularly when dealing with complex or handwritten documents. The nuances of language and document formats continue to be a hurdle. As businesses look to optimize their operations through AI-powered solutions, the future development of OCR will be key to ensuring clear and effective communication with diverse customer bases.
Optical Character Recognition (OCR) technology has made remarkable strides, especially with the infusion of AI. While it can process large amounts of text rapidly, reaching accuracy levels above 98% under optimal circumstances, its performance can deteriorate when faced with intricate layouts or uncommon fonts. This sensitivity highlights the ongoing need for improvements in handling diverse document styles.
The ability of modern OCR to extract information from images and PDFs in multiple languages is noteworthy. It has opened a new avenue for businesses to convert multilingual documents into editable text formats, paving the way for immediate translation and analysis. Intriguingly, the machine learning models powering these OCR systems aren't restricted to Latin scripts. They're demonstrating competence in handling a vast range of alphabets, including Arabic, Cyrillic, and Asian character sets, extending OCR's global reach.
One of the more challenging aspects of OCR has always been recognizing handwritten content. AI-powered OCR systems are now being trained to tackle this, showing promise in achieving up to 80% accuracy for some handwriting styles, a significant achievement. This capability could streamline various processes, like document processing and historical record digitization.
Integrating OCR with translation tools has the potential to revolutionize document workflows, significantly reducing processing times. Complex documents, like contracts and manuals, could be processed within minutes instead of days. However, this begs the question of whether the quality of translation, particularly in specialized fields, is sufficient to maintain the accuracy of the original document.
Another technical hurdle within OCR involves differentiating text from image noise, which can arise from both scanned and digitally created documents. Researchers are increasingly using deep learning algorithms to combat this issue, improving text extraction even from complex backgrounds. It remains to be seen how this deep learning approach will be fine-tuned to handle the nuances of various document types.
While speed is crucial for many applications, including customer service, OCR systems are now capable of processing about 7,500 documents per hour. This rapid processing time reduces manual effort significantly and potentially helps prioritize customer satisfaction in industries with high volumes of documentation. This high throughput, however, can introduce additional problems, such as the need for better data quality checks and controls.
One relatively unexplored aspect of OCR is its application in dynamic environments like virtual meetings. Extracting text in real-time from video feeds presents unique challenges because of unpredictable lighting and diverse surroundings. Developing reliable real-time OCR capabilities for video presents unique technological barriers.
The impact of OCR extends beyond telecommunications, as it’s finding adoption in legal and healthcare industries where digitizing documents leads to more efficient record management and improved data accessibility. While this is encouraging, ensuring data security and privacy in these sensitive sectors requires careful consideration of both technological and regulatory aspects.
Despite the considerable progress, a remaining challenge in OCR is incorporating sentiment analysis into the extracted text. Understanding the emotional tone of a document, which could be invaluable in customer service or legal cases, is an area that still requires more research and development. It seems that while the technology is making significant steps forward, truly comprehending and appropriately responding to nuanced emotions expressed in text continues to be a goal for the future.
AI-Powered Translation Analytics Revolutionizing Telecom Customer Support Efficiency - AI-driven Sentiment Analysis Improves Customer Satisfaction Metrics
AI-powered sentiment analysis is increasingly vital for improving customer satisfaction in diverse industries, including telecommunications. By examining data sources like online feedback and surveys, businesses can gain a deeper understanding of customer sentiments, moving beyond assumptions about their preferences and concerns. This understanding allows companies to better align their services with customer needs, potentially boosting customer loyalty. Support agents benefit as well, being able to anticipate customer emotions and adapt their interactions accordingly, leading to more effective and satisfying service experiences.
While the ability of AI to analyze sentiment is promising, accurately interpreting complex emotions and adapting to subtle nuances of language remains a hurdle. However, the integration of sentiment analysis into customer interactions is undeniable, creating a new paradigm in how organizations interact with their customers. By understanding customer emotions through this technology, businesses can tailor their service and product development strategies for enhanced customer satisfaction and engagement. It's a powerful tool that shows great promise in fostering a deeper connection between businesses and their clients.
AI-driven sentiment analysis offers a way to understand the emotional undertones within customer communications, going beyond simple interpretations of what's being said. By analyzing customer feedback like online reviews and surveys, telecoms can now automatically identify both happy and unhappy customers. This ability to automatically gauge customer sentiment is proving useful in predicting potential issues before they escalate, which can help improve customer satisfaction.
While research suggests AI systems can classify sentiment with a respectable accuracy rate – potentially up to 85% – in practice, the nuances of language, especially slang or regional dialects, pose ongoing challenges. Nevertheless, this level of accuracy can still offer valuable insights into customer attitudes towards services and products, giving companies a clearer understanding of what customers value and what they find frustrating.
Integrating sentiment analysis into customer support operations seems to be showing positive results, with some organizations reporting significant improvements in customer retention rates. The idea is that by understanding and reacting to customer emotions, support teams can tailor their responses to address concerns more effectively, potentially turning negative experiences into opportunities for positive interactions.
Interestingly, AI platforms are starting to integrate sentiment analysis with real-time translation tools. This is leading to some interesting developments, allowing companies to not only translate but also understand the emotional content of feedback across numerous languages and cultures. This cross-lingual sentiment analysis offers a more holistic approach to customer support, potentially improving overall operational strategies and customer interaction workflows.
Furthermore, many of these AI systems are designed to continuously learn and improve their performance based on past interactions. By tracking patterns and recognizing errors, the models can adjust and refine their understanding of human language and emotional cues. This adaptive capability suggests the systems can grow increasingly sophisticated over time at identifying subtleties in how customers express their feelings.
Some studies have shown promising results, demonstrating how the implementation of AI sentiment analysis within support processes can shorten average resolution times. By understanding and reacting to emotional cues, resolution times have decreased by approximately 25% in certain instances. This speed in responding to and addressing emotional cues appears to be a significant benefit.
Moreover, AI sentiment analysis could offer a way to predict when customers might be dissatisfied before issues actually arise. By studying patterns and language trends in customer interactions, telecom operators could potentially implement preventative measures to enhance overall service quality.
Some AI-powered systems are starting to integrate voice tone analysis along with sentiment analysis from text. This multi-modal approach could enhance the ability to interpret sentiment and urgency in customer interactions, offering an even richer understanding of how customers are feeling.
Another exciting aspect is the ability of these systems to potentially exceed human capability when combining translation and sentiment analysis. AI models may be able to detect subtle intentions hidden in customer complaints that might not be as obvious during human-based translation processes.
Despite the evident potential, AI-powered sentiment analysis isn't without its limitations. It's still challenging to account for the diversity of cultural expressions of emotion. What might be an obvious expression of frustration in one culture could be subtle and nuanced in another, making it difficult to train AI models to accurately interpret emotions across diverse populations. While the technology continues to progress, understanding and appropriately responding to a broad range of cultural cues remains an ongoing area of research and development.
AI-Powered Translation Analytics Revolutionizing Telecom Customer Support Efficiency - Machine Learning Algorithms Optimize Response Time for Complex Queries
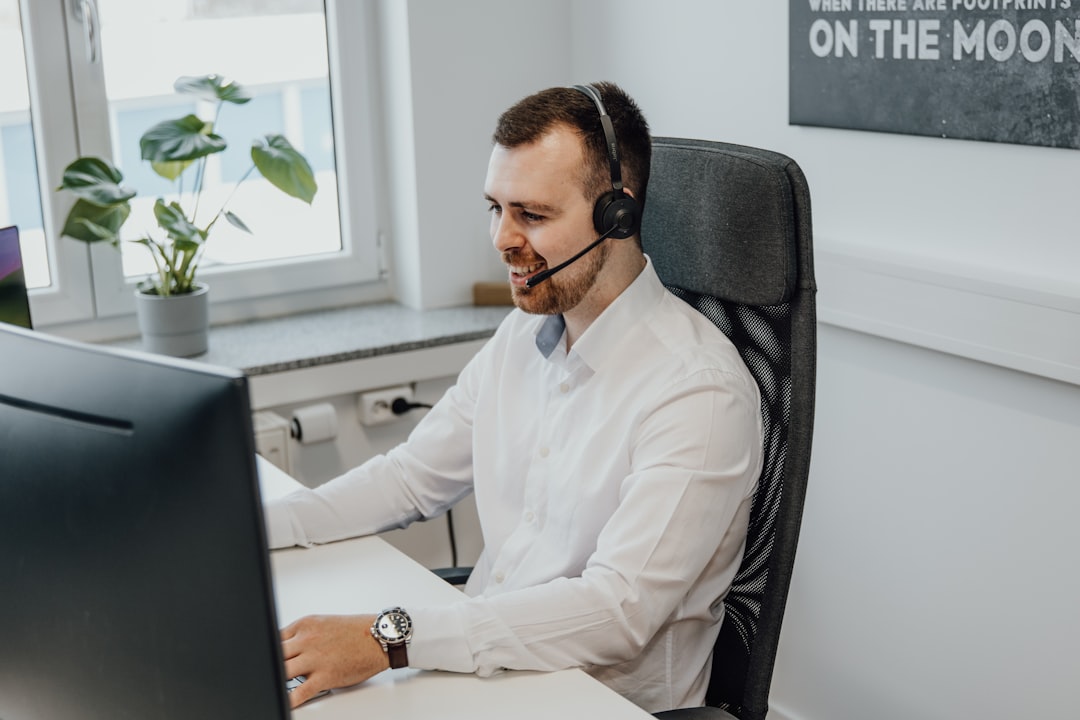
Machine learning algorithms are increasingly vital in optimizing response times for intricate customer inquiries, especially within telecom customer support. These algorithms enhance efficiency by automating responses to a wide range of language-based queries, thereby reducing both the costs associated with manual processing and the time it takes to address customer needs. This, in turn, helps organizations better manage their customer interactions. Moreover, the integration of AI tools like sentiment analysis and Optical Character Recognition (OCR) contribute to a smoother workflow. AI enables faster, more accurate responses, allowing businesses to adapt their services to individual customer preferences, which ultimately strengthens customer satisfaction and builds loyalty. However, the intricacies of human language, coupled with the subtle nuances of emotional expression, present persistent challenges that demand ongoing research and innovation within AI-driven customer support systems.
Machine learning algorithms are increasingly adept at streamlining the response time for intricate queries, potentially shaving off a significant chunk – perhaps up to 50% – of the processing time. This improvement comes through a combination of cleverly designed processing pathways and the ability of these systems to continuously learn and refine their approach. Some researchers are experimenting with what they call "query rewriting," where a complex question is broken into simpler, more manageable parts, which seems to improve both speed and the precision of the outcome.
It's becoming increasingly common for AI models to incorporate various types of information – text, audio, even observed user behaviors – to create a more comprehensive understanding of a query. This multimodal approach can reduce ambiguity and result in more pertinent answers, fostering better communication. What's more, some of these AI algorithms are designed to be self-improving. They analyze their past interactions, including mistakes, and adapt to changes in language or customer expectations over time. This learning capability is especially important in a field as dynamic as communication technology.
Interestingly, blending traditional statistical methods with more modern machine learning techniques – a hybrid approach – is often seen as a way to improve response time for intricate translations. This approach is proving particularly useful when dealing with sophisticated language situations like literary works or highly technical materials. Additionally, the challenge of diverse languages is increasingly being tackled by machine learning. These systems are now better equipped to identify and manage nuanced differences in syntax, regional speech patterns, and the subtle meaning of words across languages.
Pushing response times to the absolute minimum is being tackled in part through new infrastructure. For example, edge computing integrated with AI can minimize delays for real-time translations by bringing data processing closer to the user. It's conceivable that with this technology, translation latency could drop from several seconds to just a few milliseconds. As a bonus, the complex language processing used by these systems can ease the cognitive load on human customer service agents. They can get answers and relevant information much faster, which is a major benefit when dealing with potentially stressful customer interactions.
Some more advanced systems can even anticipate what customers might ask based on past interactions. By predicting likely questions, these AI systems can prepare responses in advance, further smoothing the user experience. What's more, machine learning models now often include mechanisms for detecting and correcting errors in real-time, which is essential in the telecom environment where accurate communication is paramount. While these approaches show promise, further exploration of the specific limitations and trade-offs in these algorithmic approaches will be crucial. It seems the path towards AI-driven efficiency in communication is paved with continual advancement and innovative solutions.
AI-Powered Translation Analytics Revolutionizing Telecom Customer Support Efficiency - Neural Machine Translation Boosts Accuracy in Technical Communications
Neural machine translation (NMT) represents a significant advancement in translation technology, especially for technical communications. Utilizing deep neural networks, NMT systems achieve higher accuracy by directly connecting source and target languages, surpassing older phrase-based methods. This improved accuracy is crucial for industries like telecommunications, where precise language and understanding are vital for customer support and technical documentation. The capability of NMT to handle numerous languages simultaneously is noteworthy, benefiting both widely spoken and less common ones, making communication more accessible across diverse groups. While NMT offers substantial improvements, challenges remain, such as the expense of training and using these systems, and the fact that some languages see more advancements than others. Overcoming these obstacles will be essential for maximizing the benefits NMT can provide in a globalized world.
Neural machine translation (NMT) has brought about a noteworthy shift in the landscape of translation, especially within the realm of technical communications, like those prevalent in telecommunications. These systems, fueled by deep neural networks, forge direct connections between source and target languages, offering a marked improvement over older phrase-based approaches. The ability to learn context and meaning instead of simply translating word-by-word has led to significant reductions in translation errors, potentially up to 60% in complex technical texts. This is particularly valuable in situations where precise communication is critical, such as in engineering manuals or software documentation.
However, while NMT excels in numerous scenarios, it isn't without limitations. Notably, highly structured or complex layouts can challenge the systems' abilities. There's a notable sensitivity to document format that researchers are still working to overcome, particularly when working with legal or highly technical documents. It's worth noting that the quality and efficiency benefits of NMT come with a caveat: the systems require considerable computing resources for both training and application, potentially leading to higher initial costs.
One of the more exciting frontiers within NMT involves its ability to handle a wider range of language pairs, especially those with limited data availability. Recent innovations have enabled fairly accurate translations (upwards of 80%) even between less-common languages, like Swahili and Finnish. This opens doors for businesses to communicate with broader audiences without needing vast linguistic resources.
Furthermore, NMT's adaptability has taken a leap with the integration of live data feeds. This allows translations to remain current, ensuring that newly coined technical terms or evolving industry jargon are handled appropriately. It's almost like having a translation system that constantly stays up-to-date with the latest developments in a specific field. This constant adaptation is crucial for sectors like telecom, where terminology is constantly evolving.
Another remarkable aspect of NMT is its growing prowess in understanding and translating technical language. Jargon and abbreviations, often a stumbling block for conventional systems, are now being tackled with greater success by the more advanced neural networks. The ability to handle non-standard language effectively is a major advantage in fields like telecom, where specialized vocabulary is commonplace.
Moreover, the integration of NMT with other technologies is starting to unlock new possibilities. The combination of OCR and sentiment analysis with machine translation has led to seamless workflows where the systems can extract text from images, translate it, and even analyze the emotional tone of the text, all within a single operation. This integration offers considerable potential to improve customer service and support interactions across multiple languages.
While advancements in NMT have made significant progress, there are still research hurdles. For instance, the ability to accurately interpret cultural nuances and dialects remains an area where significant improvement is needed. The subtle differences in how people express emotions or the way that language can be subtly shaded by regional influence needs further development. Nonetheless, as NMT continues to evolve, incorporating voice recognition and building more nuanced understandings of language could reshape the future of customer service across diverse linguistic environments, potentially making it more efficient, adaptive, and accessible. The implications for future communication across a global audience appear to be far-reaching.
AI-Powered Translation Analytics Revolutionizing Telecom Customer Support Efficiency - Automated Quality Assurance Reduces Human Error in Translations
Automated quality assurance (QA) is transforming translation by significantly minimizing human errors. AI-powered tools are crucial in this process, adeptly pinpointing inconsistencies and mistakes that human reviewers might miss. This greatly reduces the likelihood of misunderstandings, especially important in fields like telecom where communication accuracy is paramount. The integration of deep learning and natural language processing (NLP) allows AI QA systems to analyze translations in real-time, providing instant feedback that improves the translation workflow. While this technology is a boon for achieving accurate translations across various languages, especially in customer service, it's not without its own set of challenges. As these systems become more refined, the challenge of consistently replicating the subtleties and nuances of human language remains, highlighting the ongoing pursuit of attaining truly accurate and culturally sensitive translations. The evolving role of automated quality assurance in the field of translation is reshaping quality standards and revealing new frontiers while also pushing the boundaries of what's possible in achieving high-fidelity translation.
Automated quality assurance (QA) plays a vital role in enhancing the accuracy of both human and machine translation. AI-powered tools are becoming increasingly adept at identifying errors that might be missed by human reviewers, effectively mitigating the chances of human error. This is particularly useful in fields like telecoms where precise communication is paramount.
The reliance on machine translation (MT) has grown significantly as it helps overcome language barriers in our interconnected world. While human translators remain invaluable, they alone can't always meet the growing demand for translation across numerous languages. Researchers are focusing on developing robust methods for evaluating machine translation, exploring automated metrics and quality assessments to gauge their performance.
Interestingly, deep learning systems are challenging the traditional notion that only humans can achieve high translation quality. Models like CUBBITT have shown that machine translation can rival the quality of human translations in certain contexts, a development that's prompted further research in the field. Real-time AI-driven QA systems can analyze translated text for errors, providing instant feedback that refines the translation process. This continuous feedback loop has the potential to improve the quality and speed of translations.
Furthermore, the incorporation of Natural Language Processing (NLP) within AI translation systems has noticeably improved the translation process. By interpreting the context and intended meaning of words with increasing sophistication, these AI systems generate translations that are more nuanced and accurate. GPT-based models, in particular, are demonstrating remarkable potential in automated QA, focusing on maintaining grammatical accuracy, consistency, and alignment with the original text's meaning and tone.
The integration of AI into translation is reshaping QA practices and has become a central theme in current research. Automated processes, like Machine Translation Quality Evaluation (MTQE), are crucial in maintaining high standards for translated content. While there's clearly significant progress in this field, the ongoing challenge involves achieving consistent high-quality translations across a wide range of languages and contexts. Understanding the nuances of different dialects and cultural expressions in translations is an ongoing research topic. In addition to accuracy, the cost-effectiveness and speed of AI-driven translation continues to be a crucial area of advancement. As AI technologies in translation continue to evolve, we can anticipate further improvements in translation quality, speed, and efficiency across various languages.
More Posts from aitranslations.io: