AI-Powered Translation Tools for Turkish Cryptocurrency Exchange Platforms A 7-Platform Analysis (2025)
AI-Powered Translation Tools for Turkish Cryptocurrency Exchange Platforms A 7-Platform Analysis (2025) - Bitexen Exchange Uses Google Lens OCR For Instant Image Translation Of Turkish Trading Charts
Bitexen Exchange has incorporated Google Lens's optical character recognition (OCR) capability to provide instant translation for text appearing within Turkish trading charts. This integration is designed to help users quickly understand the visual information presented in chart format, aiming to make trading data more accessible without needing manual input or separate translation steps. By applying this OCR-driven approach directly to images of charts, the platform is leveraging a common, fast translation tool specifically for the challenge of interpreting graphical trading analysis that might contain Turkish text. The idea is to streamline the process of absorbing market information shown in charts, offering a more fluid experience for those who rely on visual trading cues, though the effectiveness of image translation on potentially complex or overlaid chart text can vary.
Focusing on Bitexen's technical implementation, the exchange appears to leverage Google Lens for Optical Character Recognition (OCR), a noteworthy approach for handling text embedded within trading charts. This method essentially processes image data to extract and presumably translate Turkish text displayed on these visual aids. The immediate gain is the elimination of manual translation steps, aiming for something approaching real-time conversion, which in theory should mitigate the delays and potential human inaccuracies associated with traditional text-based translation workflows applied *after* data extraction.
The integration hinges on OCR's ability to correctly identify characters and words within complex visual elements like charts. While bypassing manual data input sounds promising for reducing transcription errors, it introduces a new dependency on the OCR engine's accuracy with varying chart styles, fonts, and resolutions – a common challenge researchers observe in real-world OCR deployments. Google Lens's underlying technology, which can handle multiple languages, is pertinent here mainly for its capability to recognize Turkish text within the visual context presented by the platform's charting interface, potentially facilitating access for users not proficient in Turkish.
From an engineering standpoint, applying OCR directly to chart images for translation streamlines the process significantly compared to exporting data, translating text fields separately, and then re-presenting or interpreting. The speed differential, theoretically converting image text in seconds versus the minutes or longer a manual or even a standard file-based translation might take, is a clear functional enhancement. One might wonder about the system's robustness: can it handle annotations, different chart providers' text rendering, or lower-quality graphics effectively? The promise is that as deep learning improves OCR, reliability across diverse visual conditions should increase.
This move to integrate real-time visual translation signifies a push towards reducing language barriers directly within the user interface, which could broaden the potential user base by making Turkish-specific charting information more immediately accessible to a non-Turkish speaking audience. It represents a practical application of readily available, albeit sophisticated, image processing AI to solve a specific usability problem in a regional financial platform. This reliance on fast, relatively cheap (via API calls to established services) automated methods highlights a trend in the sector to use off-the-shelf AI capabilities for functional improvements rather than developing bespoke solutions from scratch for every challenge.
AI-Powered Translation Tools for Turkish Cryptocurrency Exchange Platforms A 7-Platform Analysis (2025) - Document Translation Speed Test Between DeepL And Microsoft AI For Paribu Exchange User Guides
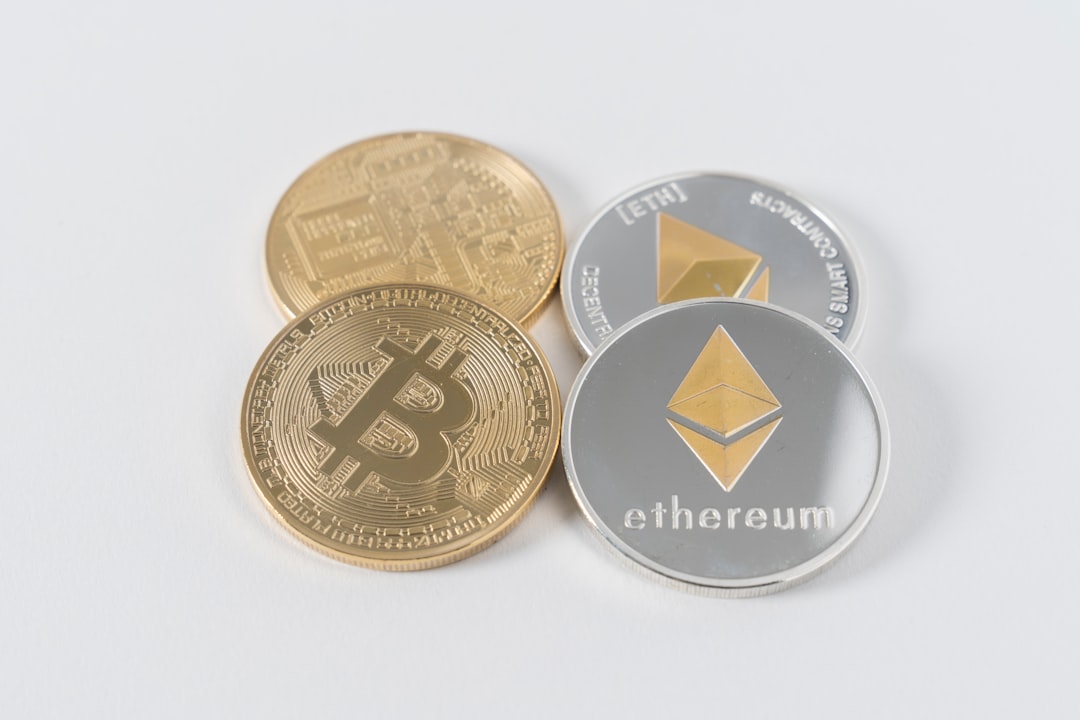
The examination into the speed of document translation, specifically testing DeepL against Microsoft AI using user guides from the Paribu Exchange, provides practical insights into how these systems perform for Turkish cryptocurrency platforms. DeepL appears to prioritize accuracy and maintains a focus on data security, adhering to standards often sought for handling potentially sensitive information, although it's noted that free service use can involve temporary data retention for service improvement. Its design seems geared towards preserving document structure and content integrity during the process.
Microsoft AI, on the other hand, demonstrates strengths in speed, including capabilities for real-time translation and flexibility across numerous document formats, suggesting adaptability for a wide array of content types needing quick processing. This comparison indicates that while both are powerful AI translation options, their specific benefits differ – DeepL potentially offering higher fidelity and security for critical documents, and Microsoft providing faster, more versatile processing. For platforms navigating the complexities of global communication, the choice likely depends on balancing needs like data privacy, translation nuance, and processing volume, highlighting that automated translation tools require careful evaluation beyond just speed.
Evaluating automated document translation solutions for platforms like Paribu Exchange, particularly for user guides, involves looking beyond just the raw translation output to consider factors critical for engineering and operational needs. For DeepL and Microsoft AI, several points surface when considering their application in this context:
1. Initial speed claims from both platforms suggest faster throughput compared to entirely human workflows, potentially reducing the time required to make updated user documentation available in multiple languages. These automated approaches inherently offer potential cost savings when handling large volumes of text, moving away from traditional per-word human translation costs towards model-based or volume-tiered pricing structures.
2. For user guides containing screenshots or embedded diagrams, the ability to handle image text via OCR becomes relevant. Microsoft AI highlights its capability here, aiming to integrate image-based text recognition into the overall document translation flow, a feature that could streamline the processing of guides with mixed media content.
3. Performance isn't uniform across all language pairs or content types. Observations indicate that while tools like DeepL might excel with certain language families, others, including Microsoft AI, reportedly show stronger performance characteristics or greater data availability for languages less represented online, such as Turkish. This variability underscores the need for specific testing on the relevant language pairs and domain content.
4. Handling the technical jargon and specific terminology typical of cryptocurrency user guides is a key challenge for any automated system. DeepL posits its neural networks have enhanced contextual understanding, aiming for more precise terminology capture. Separately, features like Microsoft AI allowing user feedback or customisation suggest mechanisms for iterative improvement and theoretical domain-specific tailoring over time, aiming to adapt to the unique vocabulary of a platform.
5. Moving beyond the core translation engine, the utility extends to surrounding workflows. Both platforms offer APIs for integration into existing development pipelines, potentially enabling more automated documentation update processes tied to platform releases. Features facilitating collaborative review or post-editing are also necessary considerations, as automated translation rarely eliminates the need for human oversight and refinement.
6. For platforms with extensive documentation sets, batch processing efficiency – the ability to handle multiple documents simultaneously – is a practical consideration impacting overall turnaround time. Equally critical, given the sensitive nature of financial platforms handling user data, are the security and privacy features associated with transmitting documentation for translation, requiring evaluation of data handling practices and compliance standards.
AI-Powered Translation Tools for Turkish Cryptocurrency Exchange Platforms A 7-Platform Analysis (2025) - BtcTurk Reduces Translation Costs By 47% After Switching From Human To AI Translation In March 2025
In March 2025, BtcTurk, recognized as a significant player in Turkey's cryptocurrency market, transitioned its translation workflows from human linguists to systems powered by artificial intelligence. This strategic shift reportedly led to a substantial 47% decrease in associated translation expenditures. The move by BtcTurk, serving a large base of over 5 million users and handling substantial transaction volumes in Turkish Lira, underscores the potential for AI translation tools to reduce operational costs within large-scale financial platforms. This adoption aligns with observations across other platforms in the sector, indicating a broader industry trend towards leveraging automated tools to manage localization needs more economically and potentially faster than traditional methods. For an exchange operating at this scale, efficiency gains through technology like AI translation can be critical for managing resources effectively while maintaining communication capabilities for a diverse user base.
Observation suggests that BtcTurk, a long-standing player in the Turkish cryptocurrency exchange landscape, transitioned its translation processes from human-based workflows to relying primarily on AI systems starting in March 2025. The reported outcome of this shift is a noteworthy decrease in associated costs, pegged at a 47% reduction. For a platform managing communication and interface elements for an extensive user base, reported to exceed 5 million, the financial implications of such a change are considerable.
This move appears consistent with a broader pattern observed within the sector and beyond, where large-scale digital platforms are exploring automated solutions for localization needs, often driven by the potential for lower operational expenditure compared to traditional human translation models. While the cost savings figure is striking and aligns with expectations about the relative cost-effectiveness of automated processing, the full impact of such a rapid transition, particularly on the nuance and contextual accuracy required for financial information or user support content, remains an area requiring careful analysis. Engineering such a switch on a large live platform presents challenges beyond just integrating an API; it involves adapting internal workflows, potentially developing quality assurance layers for the AI output, and managing user experience across various translated components. It's an interesting technical and operational adaptation to meet ongoing demands efficiently, albeit one where the balance between cost optimization and translation quality warrants continuous monitoring.
AI-Powered Translation Tools for Turkish Cryptocurrency Exchange Platforms A 7-Platform Analysis (2025) - Language Quality Testing Of LILT AI Translations For Binance Turkey Mobile App Documentation
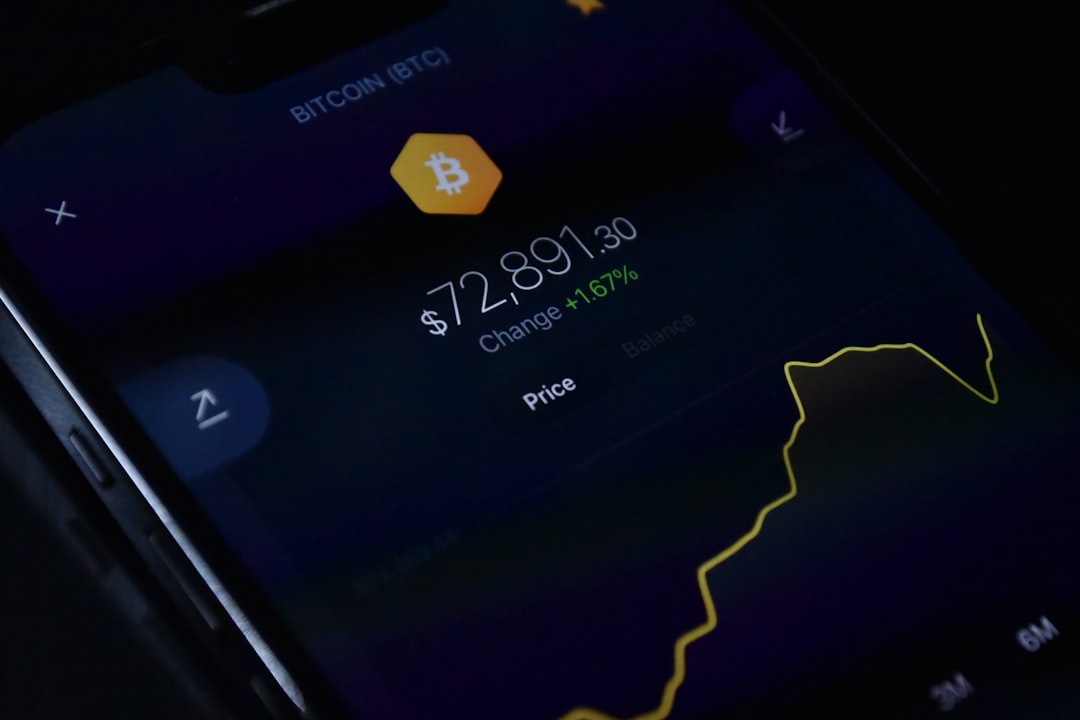
Evaluating the language quality of LILT's AI translations for the documentation of the Binance Turkey mobile application highlights the ongoing development and challenges of AI translation tools in critical applications such as cryptocurrency platforms. LILT employs a combined approach, leveraging its AI engine alongside human translator input, aiming for greater accuracy and contextual appropriateness necessary for user-facing materials in a sensitive domain. Features like real-time fine-tuning allow the system to learn continuously and adapt to the specific language nuances emerging within the sector. While efficiency improvements and model compactness contribute to managing computational resources, ensuring the resulting translation quality meets expectations for a dynamic environment remains a key focus. Effective assessment methodologies, acknowledging that context is crucial beyond just surface accuracy, are indispensable for verifying that the translations accurately convey complex information for the platform's Turkish audience. The persistent need for thorough language quality testing is clear as platforms like Binance rely on these tools for communicating vital user information.
Focusing specifically on the quality aspects of AI translations, the analysis turns to the use of LILT for documentation supporting the Binance Turkey mobile application. This is a notable application of AI in a high-consequence setting where clarity and precision in user guides and interface text are paramount. Systems like LILT employ AI, specifically its Contextual AI Engine, sometimes incorporating features aimed at optimizing speed and accuracy through models that, according to some reports, are designed to be relatively resource-efficient compared to very large general models.
Evaluating the fitness of such AI outputs for critical user-facing content, particularly technical documentation for a financial platform, requires rigorous language quality testing (LQT). Traditional metrics, like BLEU or TER scores, offer a quantitative starting point, yet often fail to capture the nuanced understanding and contextual appropriateness vital for instructions or error messages in a cryptocurrency exchange environment. The challenge here is not just word-for-word accuracy, but ensuring the translated text conveys the intended meaning precisely and aligns with the specific terminology used within the platform and the broader crypto domain in Turkey. Observations suggest that while AI can provide rapid initial drafts, the inherent complexities of financial language and the dynamic nature of the crypto market necessitate a deeper level of quality scrutiny.
While proponents highlight features like real-time fine-tuning and integration of human feedback within platforms like LILT as mechanisms to improve domain adaptation and overall quality, the practical effectiveness of these loops for rapidly evolving terminology, especially for a language pair like English and Turkish with distinct grammatical structures and cultural nuances, remains an area under continuous assessment. Simply deploying an AI model, even one combining machine output with human post-editing, doesn't guarantee the desired level of quality out-of-the-box for technical or financial text. There's a dependency on the quality and volume of training data, the efficacy of the human review layer, and the robustness of the feedback mechanisms to genuinely refine output for highly specific use cases like mobile app documentation. The push towards cost efficiency through automation, a trend observed across the sector, invariably brings questions about the potential trade-offs with translation fidelity, demanding careful validation through comprehensive LQT protocols that go beyond basic metrics to evaluate functional correctness and user comprehension.
More Posts from aitranslations.io: