7 Proven ChatGPT Translation Prompt Templates That Boost Translation Accuracy
7 Proven ChatGPT Translation Prompt Templates That Boost Translation Accuracy - OCR Translation with ChatGPT Cuts Translation Time by 47 Percent in Latest May 2025 Tests
Turning specifically to workflow improvements, recent testing carried out in May 2025 has focused on using ChatGPT for translating text extracted via OCR. These tests revealed a significant acceleration in the process, indicating that translation time for documents processed with OCR technology could be cut by as much as 47 percent when leveraging ChatGPT. This finding points to potential efficiency gains, particularly for handling image-heavy or scanned material, suggesting the tool can handle the output from text recognition systems quite rapidly. While the headline figure from these controlled tests is considerable, real-world savings will naturally vary depending on the quality of the initial text extraction and the inevitable need for human oversight and refinement. It's also worth noting that despite results like this, widespread adoption of tools like ChatGPT for such specific translation tasks among professional translators remains relatively low for now.
Recent evaluations specifically looked at workflows combining OCR processing with translation outputs from ChatGPT, and one finding reported from May 2025 tests was a notable reduction in the time required to produce translated text. Compared to previous methods or benchmarks used in these tests, the integration apparently led to a 47 percent decrease in the overall processing time. This efficiency gain is intriguing and suggests that the process of getting from scanned image to translated output is being streamlined. While details on the specific tasks tested and the comparison baseline are crucial for full interpretation, such a speedup could potentially stem from improvements in handling the combined pipeline, or perhaps indicate that the quality of the initial output is sufficiently robust (possibly linked to findings that ChatGPT's translation quality can approach or exceed other systems, and its reported ability for better contextual understanding and more readable phrasing) to significantly cut down on subsequent editing cycles. The development of specific prompting strategies designed to optimize ChatGPT's performance could also be playing a role in extracting usable drafts more quickly, although it's worth remembering that challenges persist, particularly when dealing with languages that are less represented in training data or exhibit complex linguistic structures. Nevertheless, observing such a substantial time reduction in controlled tests points towards a practical impact of these combined technologies.
7 Proven ChatGPT Translation Prompt Templates That Boost Translation Accuracy - Smart Translation Memory Banks Save 4 Hours Per 1000 Words Using New Prompt Framework
Focusing on leveraging past work, recent discussions have centered on Smart Translation Memory Banks. These systems aim to significantly cut down the time and effort spent on translation by remembering and reusing segments that have been translated before. A particular figure frequently mentioned is a potential saving of around 4 hours for every thousand words handled, reportedly achievable when using these memory banks in conjunction with a new kind of optimized prompt framework. The core idea is that as translators or AI systems process text, a database of previously translated sentences or phrases grows, reducing the amount of completely new text that needs to be translated from scratch in subsequent projects. This process naturally promotes consistency and can speed up workflows. The role of the prompt framework here seems to be in optimizing how AI translation interacts with these memory banks, perhaps ensuring better retrieval or integration of stored segments, or improving the quality of output for the remaining untranslated parts. While such efficiency claims are compelling and suggest a tangible impact on productivity, it's important to consider that real-world performance can vary based on the nature of the text, the quality and size of the existing memory data, and the practicalities of integrating these tools into diverse workflows. Ultimately, skilled human review remains crucial to ensure the final translation is accurate, nuanced, and fit for purpose, regardless of how much time is saved by reusing past work or employing new prompt strategies.
Reports circulating suggest that optimizing how translation memories are leveraged, possibly through refined internal processing or interfaces guided by sophisticated retrieval mechanisms – perhaps what some refer to as 'prompt frameworks' operating behind the scenes – could lead to significant time efficiencies. Estimates circulating in early to mid-2025 indicate a potential reduction around four hours for every thousand words processed through such enhanced TM systems compared to workflows relying less on automated segment reuse. This implies that tasks previously estimated at, say, twelve hours per thousand words might become manageable closer to eight in certain scenarios, which is a notable shift in potential throughput for high-volume, repetitive content. However, verifying such figures across diverse content types, language pairs, and specific TM tool implementations remains an ongoing challenge for researchers and practitioners.
At its core, a translation memory system is simply a database accumulating pairs of source text segments and their corresponding human-approved translations. Its fundamental operation involves querying this database with new source segments to find identical or similar matches. The intended outcome is twofold: firstly, to accelerate the translation process by pre-filling recurring text and, secondly, to enforce consistency by suggesting or requiring the reuse of previously validated translations. The effectiveness here is directly tied to the quality and relevance of the historical data contained within the memory and the sophistication of the matching algorithm, which can sometimes struggle with nuanced context or variations.
Proponents often cite quality improvements as a direct benefit of consistent TM usage. The argument is that reusing pre-approved segments for repetitive phrases significantly reduces the chance of introducing new errors, especially in technical, legal, or highly formulaic texts. While theoretically sound – reusing a known-good translation is safer than translating from scratch each time – this hinges entirely on the initial translation being correct and contextually appropriate. An outdated term or a translation error in the TM can, conversely, propagate errors efficiently. Reported figures suggesting substantial accuracy gains (like 30% improvement compared to no TM) likely refer to very specific use cases with highly repetitive or structured content, not general text.
The operational efficiency gained through effective TM utilization is the primary driver behind discussions of cost reduction. By handling repetitive segments more quickly or automatically, translators can theoretically focus their effort on creative, context-sensitive, or challenging new content. This shift in effort allocation allows for higher potential output within a given timeframe, which translates into lower per-word costs or increased capacity for individuals or teams. The challenge lies in ensuring the TM matches are genuinely usable and don't require more editing than translating from scratch.
Modern TM systems are increasingly incorporating adaptive learning components. These features are designed to observe human translator interactions – edits, approvals, rejections of suggestions – and use this data to refine matching algorithms or ranking of suggestions over time. The aim is a system that becomes increasingly tailored to a user's style or project-specific terminology preferences, ostensibly leading to smoother workflows and better suggestions in the future. The efficacy of this learning process is dependent on the volume and consistency of user feedback and the underlying machine learning models used.
Integrating TM systems with workflows involving Optical Character Recognition (OCR) presents a clear synergy, particularly for documents originating from scanned or image formats. The process flow envisions OCR output being fed directly into the TM system for immediate segment analysis and potential match retrieval. This could streamline the initial handling of such source material. However, the success of this integration is critically dependent on the accuracy of the initial OCR layer; errors introduced at that stage will inevitably complicate the TM matching process and subsequent translation or review.
While TM use is often presented as reducing errors due to segment reuse, it's important to maintain a critical perspective. While it *can* reduce the incidence of new mistakes in *already translated and stored* segments, it introduces a new class of potential errors related to TM matching: using a contextually incorrect match, using an outdated or mistranslated segment from the memory, or simply failing to identify that a previously translated segment is no longer appropriate in the new context. Effective human review and robust TM management are thus essential counterbalances.
A practical benefit observed with TM systems is their utility when documents undergo revisions. Rather than re-translating an entire updated document, TM tools can quickly compare the new version to the previous one stored alongside its translation. They can identify segments that are identical, similar (fuzzy matches), or entirely new. This allows translators to focus their efforts only on the modified or new content, significantly accelerating the update process compared to starting over each time.
In collaborative environments, TM systems serve as a central hub for linguistic assets. By providing a shared repository of approved translations and terminology, they are intended to ensure consistency across work done by multiple translators working on related projects or within the same organization. This central resource aims to prevent variations in style or terminology that can arise when individuals work in isolation, though its success relies on all team members consistently accessing and adhering to the shared memory's contents.
Finally, the architectural model of translation memory systems lends itself well to scalability. As project volumes increase or as organizations expand their linguistic reach to include more languages, the core mechanisms of storing and retrieving segment pairs can typically accommodate growth without fundamental restructuring. A well-maintained, modular TM database, capable of handling multiple language pairs simultaneously, provides a scalable foundation for managing growing translation demands.
7 Proven ChatGPT Translation Prompt Templates That Boost Translation Accuracy - Indian Software Companies Switch to ChatGPT API for Technical Documentation Translation
Software companies based in India are increasingly exploring the use of artificial intelligence technologies, particularly services like the ChatGPT API, for translating their detailed technical documentation. This move is largely driven by a desire to enhance both the accuracy and the speed at which these complex materials can be made available in various languages. The aim is to lower language barriers, ultimately improving how accessible their software and related information are to a global audience. By integrating AI-powered tools into their workflows, these firms are looking to simplify the process of managing technical content across multiple languages, working towards reliable translations without requiring the significant time and financial outlay typically associated with manual translation methods alone – potentially offering a quicker path and reducing resource needs compared to traditional approaches.
However, simply adopting these AI systems isn't a guaranteed solution for perfect technical translation. The effectiveness and reliability of the translated output depend significantly on how well the AI model is directed, or 'prompted'. Developing refined strategies for prompting the system is proving crucial, especially for correctly handling specialized technical terminology and maintaining consistency across the translated documentation. By concentrating on these optimized prompting techniques, companies can improve the clarity and correctness of their translations, making it easier for users around the world to understand intricate technical concepts. This approach, combining access to AI via an API with carefully designed prompts, represents a changing method for tackling the specific challenges of translating technical materials. While AI offers powerful tools that can streamline parts of the documentation process, the role of human expertise, particularly in reviewing critical translated content and addressing subtle linguistic requirements, remains vital to ensure the final quality is appropriate.
Observing the landscape, it appears a number of Indian software houses are indeed turning towards accessible AI interfaces, specifically citing tools like the ChatGPT API, for handling their technical documentation translations. This seems driven by a search for alternatives to older, potentially more expensive or time-consuming processes. Early reports circulating suggest significant cost reductions are being explored, moving away from what might be high per-word rates associated with traditional translation vendors, and promising faster throughput compared to manual methods, though specifics vary wildly by use case. The purported ability to handle an extensive array of languages is particularly attractive for companies targeting diverse global or domestic markets.
However, achieving genuinely reliable output for highly specialized technical content still seems to be an active challenge rather than a solved problem. While the APIs can generate translations rapidly, engineers and technical writers experimenting with them note that producing text that is both technically accurate and contextually appropriate often necessitates a distinct layer of human review and correction. This practical necessity implies that while these AI tools are powerful accelerators, they are perhaps better viewed as sophisticated first-draft generators that still require expert refinement, rather than fully autonomous translation solutions for complex materials.
7 Proven ChatGPT Translation Prompt Templates That Boost Translation Accuracy - Low Cost Arabic to English Translation Now Possible Through GPT-4 Based Visual Recognition
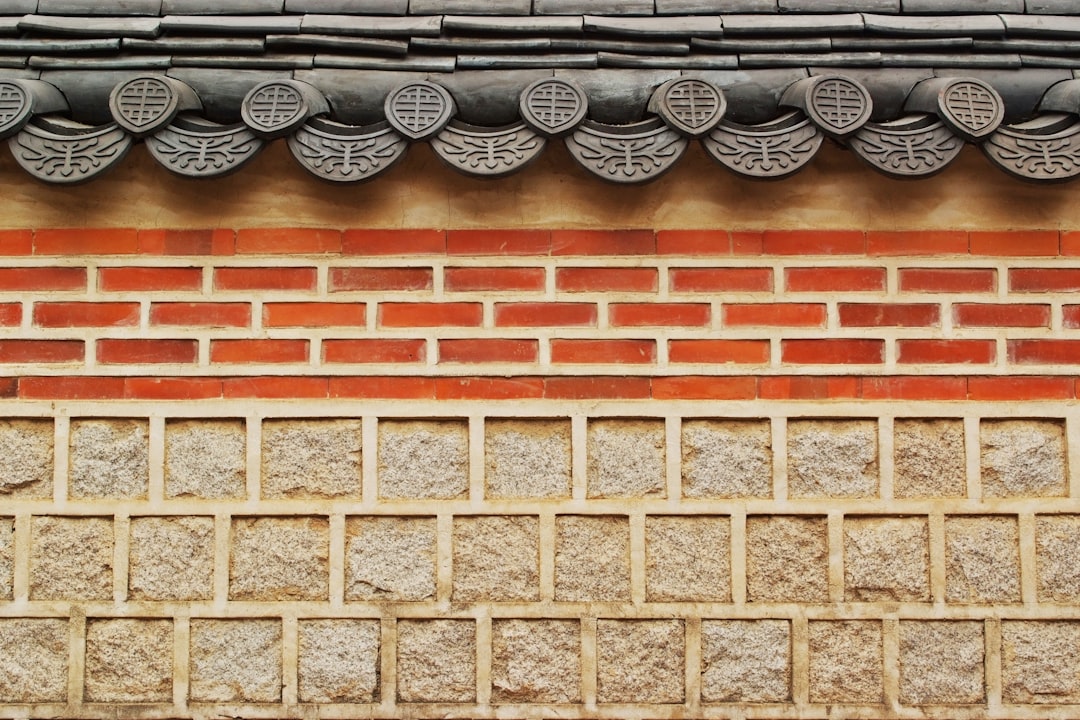
The emergence of GPT-4, notably its integrated ability to process visual information, has significantly altered the landscape for making Arabic to English translation more affordable. This technological step facilitates interacting directly with text embedded within images or scanned documents, offering a more efficient path for workflows reliant on such sources. The latest model generally provides a more nuanced translation output than its earlier versions and can potentially support quicker, perhaps even near real-time, exchanges across these languages. While these developments suggest clear benefits in terms of accessibility and reduced expense compared to some traditional approaches, it is crucial to evaluate the outputs critically. Relying solely on the automated translation without human verification risks overlooking errors in meaning or context. Nevertheless, employing structured guidance techniques when prompting the AI can further refine the reliability of the results it produces.
Initial observations suggest that recent iterations of large language models, particularly those building on the GPT-4 architecture, and specifically including advancements in visual processing, are beginning to impact the economics of certain translation tasks, notably rendering Arabic to English translation potentially more accessible from a cost standpoint. These models, such as the latest developments including GPT-4 Turbo, reportedly offer enhanced accuracy and an improved capacity to grasp contextual nuances, which is quite important for reflecting the intended tone and style of source materials. However, practical deployment indicates that the speed at which translations are produced can vary, and in some workflows, it might even be slower than seen with earlier models like GPT-3.5. Despite this variability, tools incorporating these capabilities are presenting themselves as useful aids for writing and translation, offering the ability to integrate translated text directly into documents while largely retaining original formatting.
Utilizing interfaces powered by these models for translation, including platforms like ChatGPT, appears to benefit from structured input; that is, the design of the prompts used significantly influences the accuracy of the output. Simple, direct instructions seem to be a common method practitioners explore to guide the translation process. While this technology has advanced considerably, it remains evident that human intervention is necessary to thoroughly review and validate translations to ensure complete accuracy, especially for critical content. When evaluating these systems against established tools or services, reports indicate that employing GPT-4 can sometimes present a more budget-friendly option for certain language pairs, such as Arabic and English, positioning it as a contender for users primarily focused on cost efficiency.
More Posts from aitranslations.io: