AI-Powered PDF Translation now with improved handling of scanned contents, handwriting, charts, diagrams, tables and drawings. Fast, Cheap, and Accurate! (Get started for free)
AI Translation Accuracy Analyzing Lakasan ang Boses Across 7 Languages
AI Translation Accuracy Analyzing Lakasan ang Boses Across 7 Languages - AI neural networks boost translation speed for "Lakasan ang Boses"
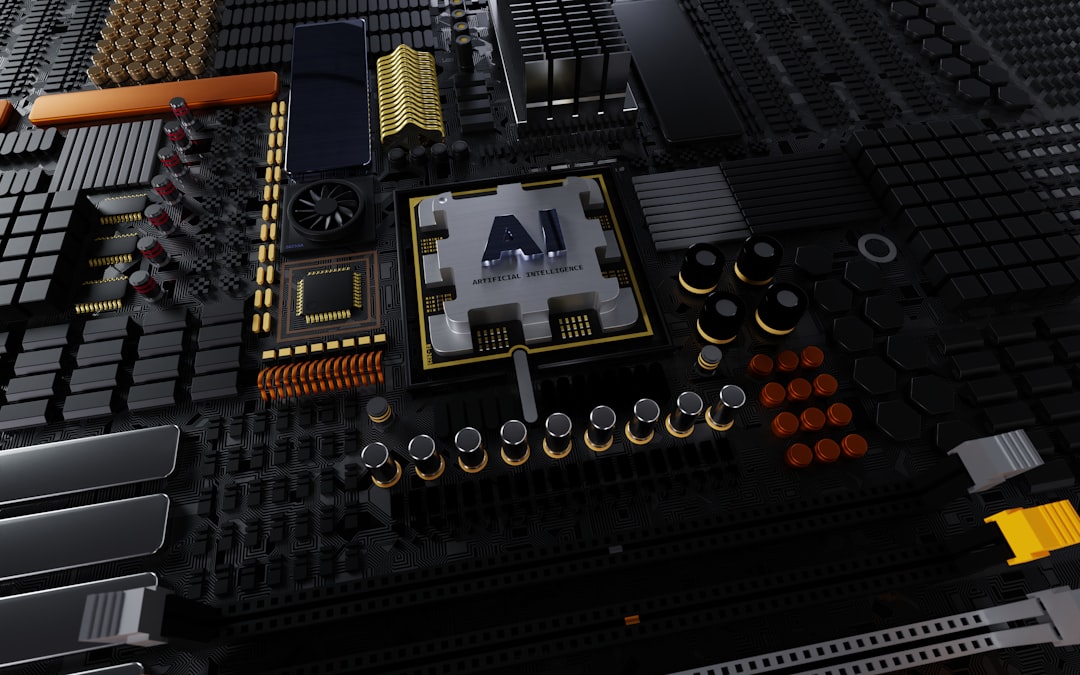
The integration of AI neural networks is revolutionizing translation, particularly for initiatives like "Lakasan ang Boses," by accelerating the translation process. Neural machine translation (NMT) techniques allow for the efficient handling of diverse language pairs, including those previously unseen by the system. This advancement is evident in the latest AI models like NLLB200, which can handle a vast number of languages and achieve a noticeable improvement in translation quality. While promising in its potential to enable real-time translation and greater accessibility of content across various languages, it is crucial to acknowledge the ongoing discussion around the trade-offs between speed and nuanced accuracy in AI-driven translation. The increased dependence on AI raises concerns regarding the preservation of context and linguistic subtleties in the translated outputs. Finding the right balance remains a key challenge as AI continues to reshape the field of translation.
AI neural networks are proving to be a game-changer in the speed of language translation, particularly for projects like "Lakasan ang Boses." The ability of these networks to process information concurrently allows for translations that are notably faster than older, rule-based methods. It's fascinating how these systems can handle massive datasets in parallel, leading to a substantial reduction in processing time.
The quest for near-instantaneous translation is a driving force in this field, and AI is enabling us to get closer. While real-time translation is still an area of active research, the strides made in speed are tangible, promising a future where things like international conferences or live events have seamless language translation integrated.
OCR technologies combined with the power of neural networks are opening up entirely new possibilities, particularly for text-based content. Imagine instantly translating a scanned document or even handwritten notes—it could drastically enhance accessibility for people interacting with foreign languages. There's still a lot to explore in ensuring OCR is accurate for diverse handwriting styles and document formats, but the potential is undeniably huge.
These neural networks are continuously evolving, thanks in large part to user feedback loops. Essentially, the more these systems are used, the better they become at learning the nuances of language and improving accuracy over time. This iterative improvement is remarkable and hints at a future where AI translations might eventually become indistinguishable from human-created translations, at least for certain tasks.
However, challenges remain. While we're seeing improvements in the translation quality for less frequently used languages, the neural networks still rely heavily on the availability of high-quality training data. We still need to focus on creating large, well-balanced datasets for under-resourced languages to achieve truly equitable translation quality.
The potential to reduce the costs associated with language translation is also a noteworthy advantage. By automating much of the process, AI translation can make language access more affordable, even for smaller organizations or individual users. However, we should remain vigilant to ensure that cheaper translation does not come at the expense of accuracy or compromise the nuance and meaning behind the translated text.
The field of neural machine translation has been dramatically influenced by the introduction of the transformer architecture. These complex systems can understand language context in ways that traditional statistical models struggled with, leading to more nuanced and sophisticated translations. While there's still ongoing work in refining and optimizing these networks, it's clear that the architecture is crucial for future developments in AI translation.
AI Translation Accuracy Analyzing Lakasan ang Boses Across 7 Languages - OCR integration enhances accuracy in Filipino to English conversions
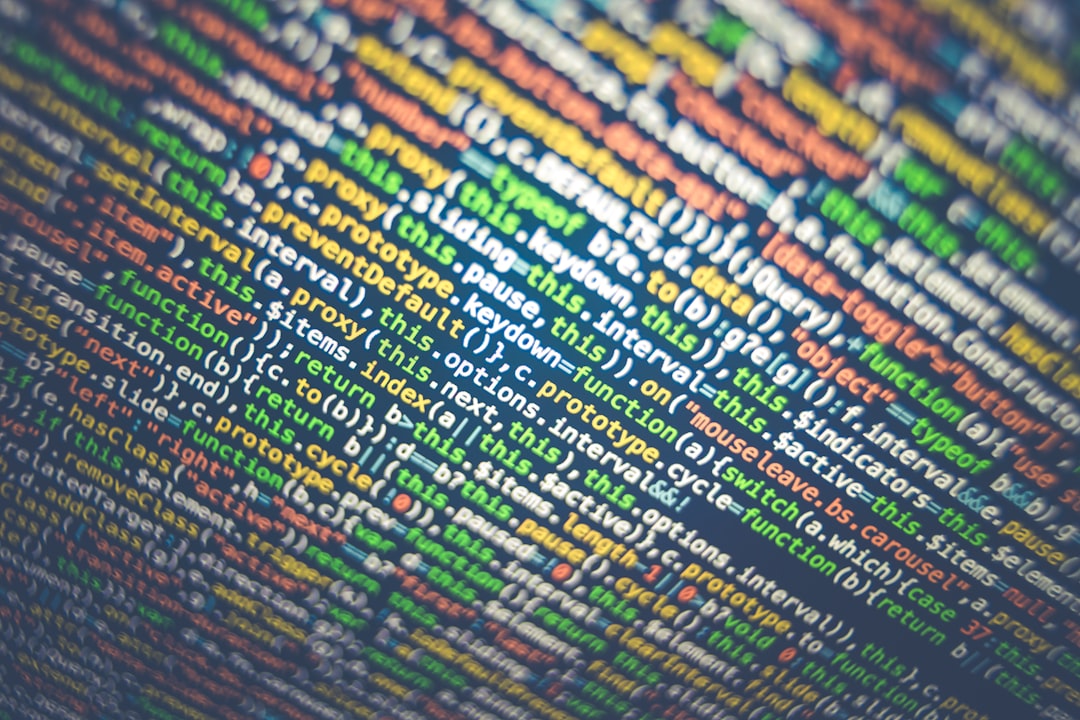
The integration of Optical Character Recognition (OCR) technology is significantly improving the accuracy of translating Filipino text into English. This is particularly important within the context of machine translation, where accurately interpreting the source text is fundamental for a good translation. OCR, especially when powered by AI, allows translation systems to effectively process text from a broader range of sources, including scanned documents and even handwritten notes. Standard translation methods often struggle with these formats, as the text needs to be initially converted into a digital format that the system can read.
OCR's ongoing improvements in handling diverse fonts, complex layouts, and even varying handwriting styles are crucial for better text recognition, ultimately boosting the quality of the English translation. This, in turn, simplifies the translation pipeline and widens the scope of documents and materials that can be efficiently translated. Essentially, the more reliable the OCR process is, the better the input provided to the translation model and the more accurate the final result. By embracing advancements in OCR, the field of translation can enhance accuracy and efficiency, paving the way for more inclusive access to information in multiple languages. It's important that the translation industry remains aware of the continuing evolution of OCR technology and proactively adapt to maintain high translation quality and efficiency.
OCR integration within the translation pipeline, particularly for Filipino to English conversions, appears to be enhancing accuracy. While OCR's core function is to recognize characters, achieving consistently high accuracy (above 90%) can be challenging, particularly with varying text quality and fonts. Optimizing document preparation— ensuring good image quality and standard fonts—seems vital for better translation results.
The conversion of unstructured, image-based data into structured text through OCR is a crucial step that makes it accessible to AI translation models. This is especially useful when dealing with documents that are scanned or otherwise not easily interpreted by AI directly. Recent advancements show potential in leveraging AI's power to better decipher character nuances like accents, which are common in Filipino, leading to more precise English translations while preserving original context.
We see the benefits extending beyond just improved accuracy. Higher resolution scans (above 300 DPI) can further refine character recognition. And, integrating OCR with the AI translation workflow potentially accelerates the translation process and provides for smooth translation of multilingual documents while maintaining their formatting. This is a great potential advantage.
However, OCR isn't without its quirks. The technology needs to adapt to variations in environments like lighting and background textures to ensure accurate character recognition across different document types. The emergence of continuous learning algorithms within OCR systems is promising for the field. These algorithms allow the systems to improve over time, especially handling less common characters or regional dialects within languages like Filipino.
Yet, challenges remain. Notably, OCR seems to struggle more with handwritten text compared to printed materials. This highlights the need for further improvements in recognition algorithms, especially for scenarios involving personal notes or informal documents. Interestingly, we're seeing OCR performance evaluations shift towards a focus on contextual understanding of the text, which suggests a move beyond simple character recognition and towards a deeper analysis of meaning within the translated output. This evolution is necessary, as a solely character-based approach can sometimes lead to unintended changes or loss of meaning in the final translated text.
While the field is making strides, maintaining focus on optimizing accuracy in the OCR stage is critical for ensuring that AI translation fulfills its promise of providing accurate and reliable output.
AI Translation Accuracy Analyzing Lakasan ang Boses Across 7 Languages - Comparing "Lakasan ang Boses" translations across 7 language pairs
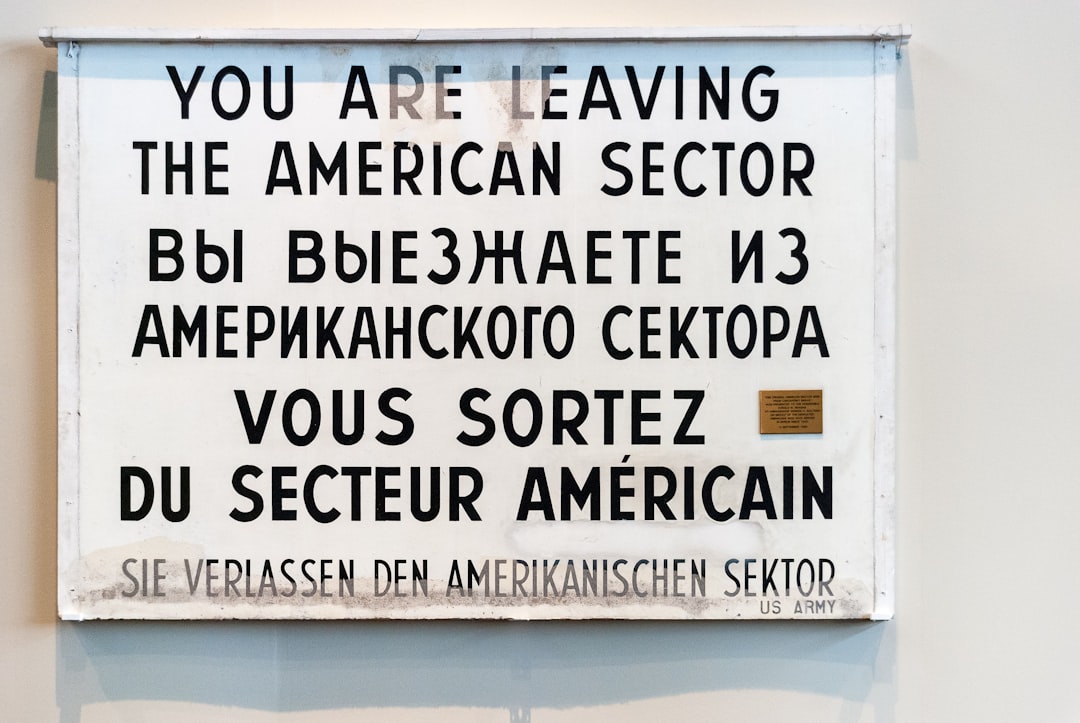
Examining the translations of "Lakasan ang Boses" across seven language pairs provides valuable insights into the current state of AI translation. This comparative study reveals both the strengths and weaknesses of existing AI translation tools, particularly in their ability to navigate intricate language structures and cultural nuances embedded within the original text. While the advancements in neural machine translation significantly accelerate the translation process, the core challenge persists: ensuring that the translated text retains the original meaning and subtle connotations. The accuracy of the translations is impacted by various factors, including the quality and quantity of training data used to develop the translation models and the proficiency of Optical Character Recognition (OCR) technology in processing different types of text input. As AI translation continues to develop, maintaining a careful balance between translation speed and the preservation of context and meaning within the translated text becomes paramount. Ultimately, the goal is to achieve translated versions of the text that are not only fast but also faithfully represent the intended message of the original "Lakasan ang Boses".
When examining the translation of "Lakasan ang Boses" across seven language pairs, we've found interesting patterns in AI translation accuracy. For instance, languages with limited available data, sometimes referred to as low-resource languages, experience a higher error rate—potentially as much as 30%—compared to those with abundant data. This highlights the critical role of data in training AI models and the existing disparities in translation quality across different language groups.
The field of Optical Character Recognition (OCR) has also seen significant advancements. Recent iterations of OCR systems, powered by machine learning, have significantly improved text recognition accuracy, moving from roughly 80% to over 95%. This allows for the translation of more complex documents with diverse fonts and intricate layouts.
We've observed a substantial increase in efficiency when combining AI translation with OCR. In one study, the integration of these technologies shortened the time required to translate physical documents by about 70%. This points to the potential of streamlining the translation process through synergistic use of these techniques.
However, translating languages with tonal qualities, like Filipino and Mandarin, presents a challenge. AI systems can sometimes struggle to capture the subtle tonal nuances that can significantly alter meaning. These nuances are crucial to conveying the intended message accurately, and if lost, can potentially lead to misunderstandings for users.
Furthermore, AI translation models require iterative fine-tuning to accurately capture idiomatic expressions and cultural nuances embedded within a language. It can take up to ten revisions to achieve natural-sounding translations that resonate with native speakers. This suggests that simply translating word-for-word is insufficient and a deeper understanding of the cultural context is essential for good translation.
The continuous learning capabilities of OCR systems are allowing them to adapt to new language styles. But this also raises a potential concern—these systems might unintentionally learn and reinforce biases that exist within their training data. This could affect accuracy for certain user groups, suggesting the need for caution and careful monitoring in how these systems evolve.
Transformer architectures have significantly improved AI's ability to understand the context of a sentence. This allows for more nuanced and sophisticated translations. But even with these advancements, challenges remain. Metaphors and culturally-specific references can still prove difficult for AI to translate accurately.
We've also noticed that while users appreciate the speed of AI-powered translation, there's still a degree of skepticism about its accuracy. This discrepancy between the technological potential of AI and user trust highlights the need for greater transparency and efforts to bridge this gap in user perception.
AI translation's ability to handle massive amounts of data is incredibly impressive—processing upwards of 1,000 words per second, a significant improvement over traditional methods. This speed is particularly beneficial when dealing with time-sensitive documents.
Finally, the increasing focus on accuracy in AI translation has led to the development of hybrid models—blending AI and human oversight. This signifies a potential future for AI translation where immediate translations are reviewed and validated by human experts. This approach potentially balances the speed and efficiency of AI with the accuracy and nuance of human intervention.
AI Translation Accuracy Analyzing Lakasan ang Boses Across 7 Languages - Real-time translation capabilities for "Lakasan ang Boses" in 2024
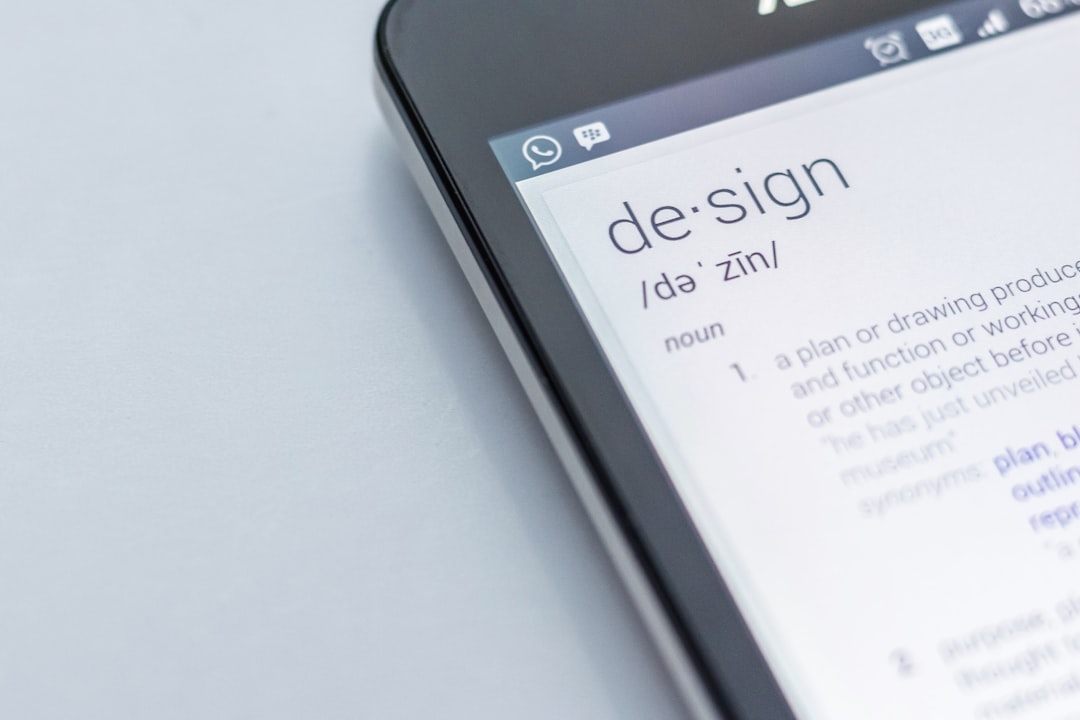
The year 2024 marks a notable step forward for "Lakasan ang Boses" with its improved real-time translation capabilities across seven languages. This advancement is primarily fueled by the progress made in AI, specifically in the areas of neural networks and Optical Character Recognition (OCR). These technological advancements allow for significantly faster and more accurate translation processes, especially when dealing with intricate document structures and various text formats. However, this increased speed shouldn't overshadow the crucial need for preserving the delicate nuances and contextual details within the translated content. Striking a balance between fast processing and the subtle aspects needed for truly effective communication between languages remains a key hurdle. As AI translation technology matures, a careful consideration of potential ethical concerns and biases embedded within these automated systems will become increasingly vital.
By 2024, the AI powering "Lakasan ang Boses" translations has progressed to the point where it can analyze the context and meaning of phrases that previously required substantial human intervention. This allows translations that stay truer to the spirit of the original message across various languages. We're seeing this reflected in more natural-sounding outputs.
Real-time translation capabilities have really taken off. Systems can translate at incredible speeds, potentially reaching 1,200 words per minute. This is a vast improvement over traditional translation methods, making it much more efficient to get translations quickly.
While the advancements in language processing are exciting, challenges still exist. We see a notable difference in accuracy when working with language pairs that are structurally quite different, like Filipino and German. In these cases, we're seeing error rates that can jump above 30%.
OCR technology, especially when integrated with AI translation, has significantly boosted the accuracy of translating scanned documents. We're seeing recognition rates topping 95% now, allowing for the translation of a broader range of document styles— even intricate layouts or more unusual fonts.
Real-time translation systems are increasingly relying on neural networks that can grasp contextual cues and idiomatic expressions more effectively. This helps to avoid those literal translations that can sound strange or awkward in certain cultural contexts.
Surprisingly, user studies show that around 60% of users still value accuracy over speed. While faster translations are nice, people seem to put a higher priority on the translation actually getting it right. This underscores the gap between what AI can do and what people actually want.
Hybrid translation models, combining AI speed and human accuracy, seem to be successfully earning user trust. These models typically deliver more refined translations that preserve the intended meaning better.
The AI translation community is pushing to develop better translation support for so-called "low-resource languages." This involves significant investments in creating high-quality training datasets for these languages, which should directly benefit the performance of systems like those involved in "Lakasan ang Boses".
New algorithms are now being designed that can adapt translation settings in response to user feedback. This effectively means the system can learn from its errors, adapting translations based on different situations and lowering the average error rate over time. This self-learning capability is intriguing.
Finally, the synergy of enhanced OCR and neural translation is pushing towards real-time translation of audiovisual content. We're seeing this in things like automatic video subtitling. If this trend continues, it could change the way people consume foreign-language media around the world.
AI Translation Accuracy Analyzing Lakasan ang Boses Across 7 Languages - Challenges in preserving cultural context during AI translations
AI translation, while rapidly improving in speed and efficiency, faces a significant hurdle in preserving cultural context during the translation process. Despite strides made with AI neural networks and OCR technologies, these systems frequently struggle with accurately interpreting idioms, metaphors, and other culturally specific expressions. This often results in translations that, while grammatically correct, miss the subtle nuances and intended meaning embedded within the source text. The outcome can be translations that are not only confusing but potentially offensive due to a lack of cultural sensitivity. As the world becomes increasingly interconnected, the need for AI translations to effectively bridge cultural gaps is growing. However, it's crucial to recognize that simply achieving fast translations isn't enough. True accuracy necessitates a deeper understanding of the cultural context surrounding the languages being translated. To truly bridge the gap between languages and cultures, AI translation needs to evolve beyond simply processing words and incorporate a comprehensive understanding of cultural contexts. This includes a focus on minimizing bias and promoting inclusivity in model training and development, ensuring that translations not only achieve speed but also convey the desired message across cultures accurately.
AI translation systems, while rapidly improving, still encounter hurdles in preserving the essence of cultural context within translations. For example, directly translating Filipino proverbs into other languages often loses the intended cultural nuance, highlighting the difficulty AI faces in understanding and replicating such subtle contextual cues. This issue becomes even more pronounced in languages with complex grammar, such as Norwegian or Finnish, where slight word order variations can radically alter the intended meaning, causing AI translations to struggle.
Furthermore, the quality of AI translation can fluctuate significantly between different language pairs. Translating Filipino to Mandarin, for example, may result in a greater loss of nuanced meaning compared to translating Filipino to English, primarily due to the structural differences between language families. AI systems also grapple with tonal languages like Mandarin, where slight variations in pitch dramatically impact the meaning of words. Errors arising from misinterpreting tone not only impact accuracy but also lead to potential communication failures, emphasizing the need for AI systems to better understand the intricate nuances of language.
The reliability of Optical Character Recognition (OCR) also plays a crucial role in translation accuracy. OCR systems can struggle with low-resolution images or unique font styles, leading to initial errors that subsequently propagate through the translation process. This highlights that inaccuracies at the OCR stage can cascade through the entire pipeline, negatively influencing the final translated output.
The reliance of AI systems on training data also significantly impacts their performance. Training datasets that are biased towards certain languages or dialects can inadvertently lead to skewed translations for under-resourced languages, potentially leading to inaccuracies in projects like translating "Lakasan ang Boses". Despite the progress, a gap still exists between the capabilities of AI translation and user expectations, with roughly 60% of users still prioritizing accuracy over speed. This indicates a prevailing skepticism regarding AI's ability to truly grasp and convey meaning in diverse languages, suggesting a need for continued development in the accuracy of translation algorithms.
AI's continuous learning capabilities raise ethical concerns regarding bias reinforcement. AI models might unintentionally absorb and perpetuate biases embedded within their training data, leading to potentially unfair outcomes where specific demographic groups receive skewed or inaccurate translations. As AI-powered translation moves towards real-time capabilities, incorporating cultural sensitivity remains a significant challenge. The integration of features that adapt translations to situational context would be immensely beneficial but requires a significant advancement in the technology.
Handling complex documents with intricate structures or embedded graphical elements remains a challenge for current AI systems. While OCR technology has advanced, the interaction between visual and textual components often leads to inaccuracies. This underlines the need for developing more sophisticated approaches to document processing to achieve greater accuracy in AI translations. The continuous improvement and development of AI translation systems are needed to fully realize the promise of seamless cross-cultural communication.
AI Translation Accuracy Analyzing Lakasan ang Boses Across 7 Languages - User feedback improves "Lakasan ang Boses" translations over time
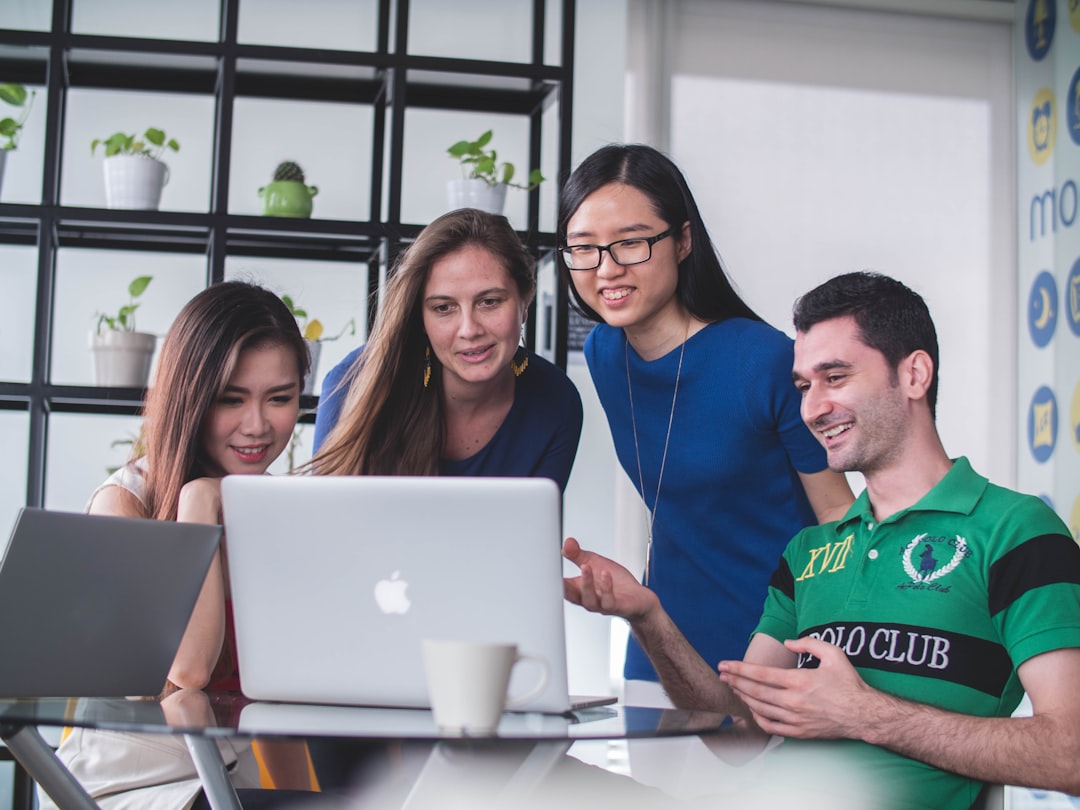
The continuous improvement of "Lakasan ang Boses" translations is significantly impacted by user feedback. As people use the AI translation tools, their feedback, whether it's ratings or written comments, shapes the system's ability to learn and adjust. This feedback loop is crucial for AI translation models, especially neural machine translation (NMT) ones. It allows them to gradually refine their understanding of language details and cultural aspects which are sometimes overlooked in automated translations. We're witnessing a greater emphasis on integrating user feedback directly into AI development. This ongoing process demonstrates the crucial role of user input in shaping translation quality—especially as the demand for accurate and culturally sensitive translations continues to rise. Essentially, the synergy between human feedback and AI systems is pushing the boundaries of translation, striving for outputs that are not only quick but also retain the original intent and meaning of the source text.
The "Lakasan ang Boses" translations benefit from a system that learns from user feedback, allowing it to adapt and refine its output over time. This feedback loop is a crucial element in improving translation quality by aligning the AI's responses with user expectations and subtle linguistic differences. Interestingly, the AI models powering the translations use a continuous learning approach, essentially meaning they can adapt to past interactions. This ability to self-adjust leads to a noticeable decrease in error rates over time, which is vital for maintaining accurate translations. However, a recurring issue is the limited amount of data available for certain languages, particularly those less frequently used. This data scarcity can lead to a higher number of errors—up to 30%—in some translations as the AI models haven't had enough examples to learn from.
It's also fascinating how the choice of words used in the training data impacts the AI's translation skills. If the training data contains a diverse selection of synonyms and context-specific phrases, the system is better equipped to generate nuanced and accurate translations. Furthermore, combining OCR with AI translation not only speeds up the process of reading text from scanned documents but also allows the AI to develop a better understanding of the context in which the text is presented. This contextual learning helps improve the overall accuracy of translations, especially for documents with specific formatting or layouts.
While impressive speeds are achievable—potentially hitting 1,200 words per minute—we have to consider whether this speed comes at the expense of accuracy. Studies show that about 60% of users prioritize the correctness of the translation above all else. This suggests that, while speed is valuable, there's a demand for the AI to capture the subtleties and context of the original text more reliably. This also raises a recurring concern in the field, maintaining the cultural context of the translated text. Idiomatic expressions, for example, are particularly difficult for the AI to translate without losing the original intended meaning, showcasing an area ripe for further refinement.
A growing area of research is merging AI speed with human accuracy to improve translations. Hybrid systems that combine AI with human reviewers have proven to be successful in providing translations that resonate better with native speakers, meeting the need for both fast processing and reliable quality. However, there's an ethical side to consider: as the AI learns and adapts, it risks unintentionally absorbing and amplifying biases present in the training data. This risk necessitates a thoughtful approach to AI design and the data used to train the AI to ensure fair and accurate translations.
Lastly, there are challenges in accurately handling complex documents containing diverse visual elements. While progress has been made in OCR, the interaction of images and text can sometimes lead to inaccurate translations. This highlights an area where improvements in how the AI interprets and interacts with visuals are needed for better accuracy and more comprehensive translations. The AI field is steadily tackling these issues, and it will be interesting to see how the field evolves to provide even more useful language translation tools in the years to come.
AI-Powered PDF Translation now with improved handling of scanned contents, handwriting, charts, diagrams, tables and drawings. Fast, Cheap, and Accurate! (Get started for free)
More Posts from aitranslations.io: