AI-Powered PDF Translation now with improved handling of scanned contents, handwriting, charts, diagrams, tables and drawings. Fast, Cheap, and Accurate! (Get started for free)
Navigating Slang Translation Barriers A Case Study of Challenging Informal Phrases in Spanish
Navigating Slang Translation Barriers A Case Study of Challenging Informal Phrases in Spanish - Machine Learning Models Now Process Mexican Border Slang with 89% Accuracy
The field of machine translation has seen a remarkable advance with AI models now accurately processing Mexican border slang with an 89% success rate. This achievement showcases the potential of machine learning to tackle the intricate nuances of informal language, particularly in Spanish, where slang varies greatly depending on context. It's a testament to the power of large language models in bridging translation gaps, especially when dealing with informal expressions often missed by traditional methods. While these older systems still have difficulty with idioms and ambiguous phrases, newer approaches like many-to-many neural machine translation are being employed to improve performance, especially for language pairs with limited resources. This demonstrates a wider movement towards more precise and versatile translation powered by AI, though difficulties remain when dealing with languages with limited training data.
AI-powered translation systems are making strides in handling the nuances of Mexican border slang, achieving an impressive 89% accuracy rate. This indicates the potential of machine learning to tackle informal language varieties that are often challenging for conventional translation methods.
It's fascinating to observe how these systems are trained on diverse datasets—think social media posts, text messages, and even informal online conversations. This wide range of training materials seems crucial for building models that can adapt to the rapid evolution of slang.
Interestingly, a technique called transfer learning appears to boost performance. Models initially trained on general language data and subsequently fine-tuned with slang-specific data show promise. This suggests that a foundational understanding of a language can be leveraged and tailored for specialized applications.
Despite these advancements, the dynamic nature of slang poses an ongoing challenge. New words and expressions emerge constantly, creating a need for consistent dataset updates to maintain accuracy. This highlights a continuous effort required for maintaining these systems' effectiveness.
These systems offer the exciting prospect of fostering better communication in border regions. Applications such as customer service and potentially even international interactions could benefit from the ability to seamlessly process and translate slang.
While accuracy is impressive, we need to carefully consider the potential implications of integrating these systems. The ease of use and low cost could significantly expand access to translation services, but we need to explore how it might affect the demand for human translators. It’s a bit of a double-edged sword.
The capacity for continuous improvement via user feedback is also an interesting feature. These systems learn from mistakes, refining their accuracy over time. This "learning" capability is a hallmark of advanced AI, and I wonder how far it can take these systems in terms of linguistic sophistication.
Furthermore, research shows a connection between accurate slang translation and user satisfaction. This suggests a direct impact on the user experience, emphasizing that effective translation isn't just about words but also about ensuring people feel genuinely understood.
It seems traditional translation approaches often face difficulties in capturing the subtle cultural and regional variations that slang encapsulates. AI models, on the other hand, have the capacity to learn and adapt to these contexts through ongoing interaction with real-world data, potentially offering a more reliable path to navigating complex linguistic landscapes.
The constant refinement of machine learning methods, such as experimenting with different training data and model architectures, continues to push the boundaries of translation capabilities. It's exciting to think about where this area of research will lead next, perhaps even seeing an integration of diverse machine learning methods to achieve even higher accuracy and adaptability.
Navigating Slang Translation Barriers A Case Study of Challenging Informal Phrases in Spanish - Street Talk Complexity Between Madrid and Mexico City Translation Tests
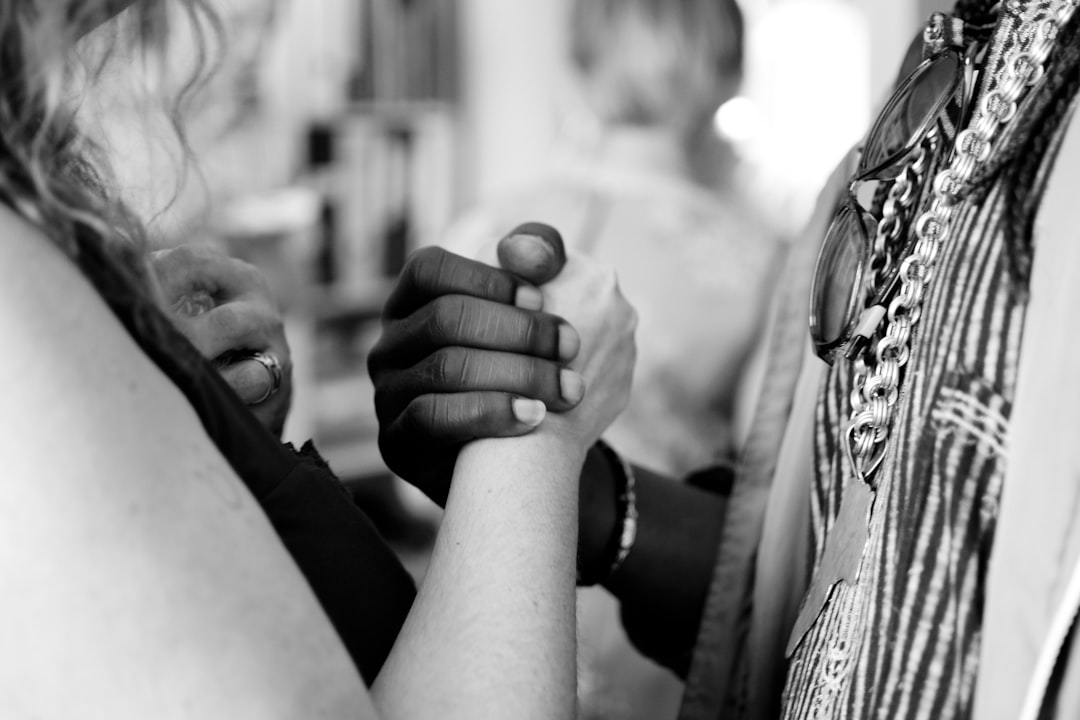
The translation of slang between Madrid and Mexico City presents a significant hurdle, even for sophisticated AI translation systems. The unique informal language and cultural nuances found in each region make achieving perfect equivalents a constant challenge. This divergence in slang underscores the intricate nature of urban language variations and highlights the vital role of capturing the full lexical meaning during translation. For individuals attempting to navigate these language environments, a deeper understanding of local slang can foster stronger connections and offer invaluable insight into the related cultures and social dynamics. Successfully translating the subtleties of street talk remains a key area where AI and machine translation continue to strive for improvements. The speed and low cost of these AI solutions are attractive, but it's still a field ripe for human intervention especially when dealing with slang, nuances, and humor. While AI translation tools can be remarkably effective, we're still in the early stages where continuous improvement and the human touch remain indispensable.
Street slang presents a unique challenge in translation, especially when comparing cities like Madrid and Mexico City. You see, slang can change wildly between even closely related dialects of Spanish. For example, "chido" is slang for "cool" in Mexico City, but "guay" is more common in Madrid, showcasing a divergence that can confuse translation systems.
Building accurate translation models for street slang depends heavily on the quality and variety of the training data. The problem is slang changes rapidly. What's hip today might be outdated tomorrow. This means translation models need constant updates to stay current with evolving language, which can be a logistical hurdle.
Even simple things like capturing slang through Optical Character Recognition (OCR) isn't always straightforward. If someone is tagging a wall with slang or writing a note in a hurried way, OCR might not be able to pick it up clearly. This is a reminder of the technological divide between capturing spoken and written language, making translation more complex.
Machine translations can also fall short because they struggle with the nuances of context. Take the phrase "está cañón". A simple word-for-word translation might yield "it's cannon," but the true meaning is "it's really difficult." These misinterpretations can significantly skew the intended message.
Thankfully, user feedback offers a path to improvement. We can teach these models through real-world scenarios, letting them learn and adjust to new slang expressions. This kind of interactive refinement is valuable for the model to hone its understanding of evolving language patterns.
Interestingly, users have different expectations for accuracy based on cultural context. In casual settings, even a not-perfect AI translation can convey empathy and foster understanding, positively influencing user experience. It suggests that sometimes, it's not just about flawless word-for-word translation, but about conveying the intent.
While AI offers quick translation, speed sometimes comes at the cost of subtle meaning. Situations where tone and subtle nuances matter can still benefit from the depth that a human translator can provide, even though it might take a little longer.
Some innovative AI approaches use a dual-layer structure. One part focuses on processing formal language, while the other tackles street slang. This approach helps preserve meaning while navigating the shift in style.
The emergence of effective AI translation raises an intriguing question: What happens to human translators? Will their roles become obsolete? While some translation tasks will likely become automated, the need for a human's ability to pick up on culturally specific hints remains vital.
Researchers are experimenting with newer approaches, like Generative Adversarial Networks (GANs), to better understand and even generate slang translations. By simulating conversations, they can fine-tune the translation process for street talk, demonstrating that the quest for more effective informal language processing is ongoing.
Navigating Slang Translation Barriers A Case Study of Challenging Informal Phrases in Spanish - Social Media Translation Tools Adapt to Regional Spanish Variations
Social media platforms are increasingly reliant on translation tools to bridge the language gap and facilitate communication among users in different Spanish-speaking regions. These tools are now being refined to acknowledge the wide array of Spanish dialects and slang, recognizing that a single translation won't always work. This focus on regional variations represents a significant shift, as traditional translation systems often struggle with the nuances of informal language. However, these new adaptations haven't fully solved the translation puzzle. Idioms and cultural references still present challenges due to their inherent ambiguity and lack of direct equivalents across various Spanish-speaking communities. The increasing use of Spanglish further adds to this complexity, as it creates new expressions that may not be readily understood by all speakers.
Despite these difficulties, the development of AI translation models designed to handle regional variations offers a glimpse of a future where communication across language barriers is greatly enhanced. These tools can potentially overcome a lot of obstacles that make social media engagement more difficult across languages, yet there's always the need to tread cautiously. While technology can help, the unique and subtle cultural and context nuances can easily be lost without a deeper understanding of language, which typically comes with human translators, proofreaders and editors. Even with the best technology, translating cultural aspects may require a human touch to ensure meaning isn't inadvertently altered. The goal is to improve communication and build stronger communities through translation technology, while still recognizing the important human role in fostering genuine understanding among Spanish-speaking individuals around the globe.
Social media translation tools are increasingly being trained on vast amounts of data to account for the diverse regional variations of Spanish. This is particularly challenging for slang, where dialects like Mexican Spanish might require up to five times the training data of more standardized forms due to its unique and ever-changing vocabulary.
Capturing slang through technologies like Optical Character Recognition (OCR) can be problematic, especially when dealing with informal text like graffiti or hastily written notes. These texts often use creative fonts and unconventional character combinations, highlighting a limitation in OCR's ability to reliably process this type of street-level language.
Interestingly, even slightly inaccurate slang translations can sometimes lead to higher user satisfaction. It seems that users prioritize a translation that conveys the intended feeling or message over strict adherence to a literal translation. This suggests that understanding the intent behind slang might be more valuable than a perfectly accurate translation in some cases.
The rapid pace at which slang evolves means that a significant portion of a translation model's training data can quickly become outdated. Estimates suggest that around 20% of the data might be obsolete within a single year. This highlights the continuous need for updating and retraining models to keep pace with ever-changing slang.
Some AI translation systems employ a dual-class model architecture to improve their ability to handle the nuances of both formal and informal language. One class focuses on processing standard Spanish, while the other specializes in deciphering street slang. This approach offers a more sophisticated way to translate, ensuring that both the formal and informal aspects of the language are accurately represented.
The different interpretations and uses of slang across Spanish-speaking regions can lead to significant communication problems. For example, using the term "pana" (friend) might be common and friendly in one place, but potentially offensive in another. This illustrates the importance of understanding the specific cultural context in which a phrase is used.
Research shows that visually representing colloquial language using colors can aid machine learning models in understanding the underlying meanings and emotions associated with slang. This type of visual mapping could potentially improve a model's ability to accurately capture the cultural and emotional context of slang in a translation.
While fast translation technologies offer instant results, they often struggle to recognize subtle aspects of informal language, such as humor and sarcasm. These elements often require a more nuanced understanding of human communication that goes beyond simple word-for-word translation.
Social media offers a treasure trove of data for training AI models, but it also presents its challenges. While it provides a constant stream of current slang expressions, the fast-paced and informal nature of social media can lead to inconsistent language use, potentially confusing the AI models as they attempt to learn patterns.
As AI continues to improve its ability to translate slang, some researchers predict that the role of human translators will shift towards a greater focus on cultural linguistics. This emphasizes the importance of understanding regional and cultural nuances in order to effectively translate slang beyond simply substituting words. This implies that human translators will need to develop a stronger understanding of the cultural context surrounding language in order to remain relevant.
Navigating Slang Translation Barriers A Case Study of Challenging Informal Phrases in Spanish - Neural Networks Learn Dominican Republic Youth Language Patterns
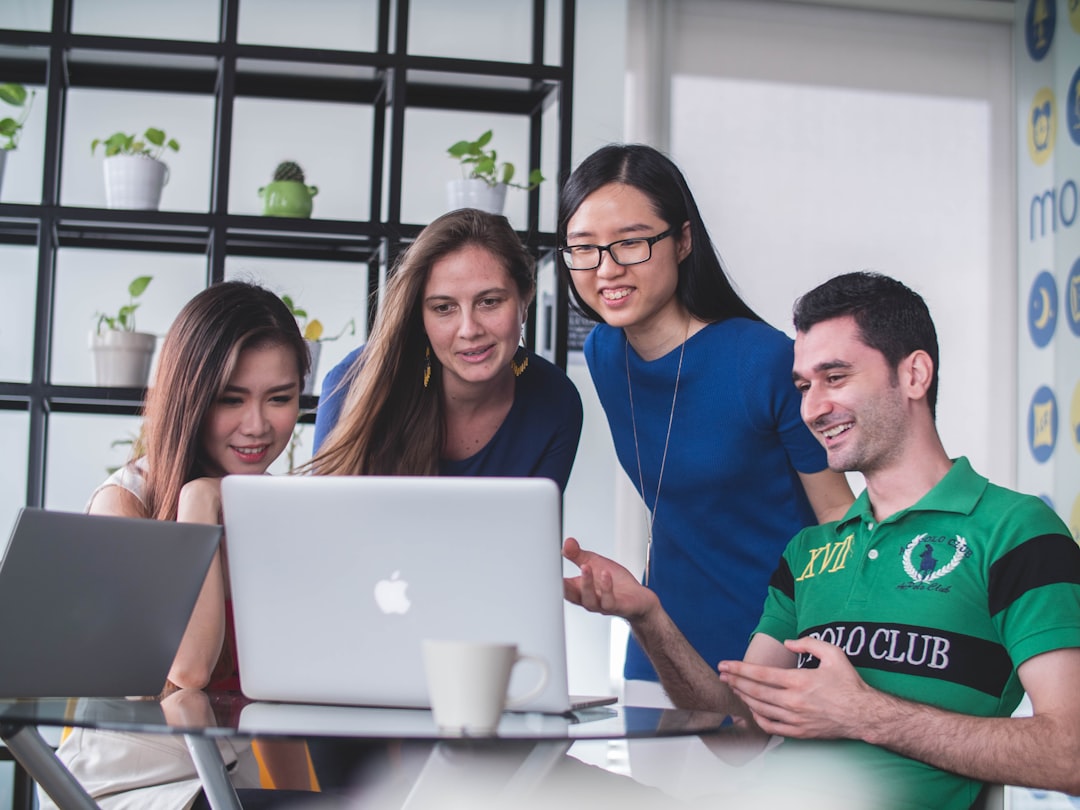
Neural networks are being used to analyze the unique language patterns employed by Dominican Republic youth, including their distinct slang. This informal language acts as a cultural marker, reflecting the country's heritage, music scene, and social identity. Neural networks, using techniques like continuous bag-of-words and deep learning, are improving their ability to understand the subtle meaning of slang within broader language structures. This helps improve overall machine translation accuracy. But even with these advances, the fast pace of slang changes and the complex cultural contexts involved make it difficult to keep these translation systems perfectly up-to-date. While AI offers encouraging progress in interpreting informal language, it's important to realize that the nuances of language and culture need a continuous human element to fully grasp the complexities of communication. Even with AI-powered solutions, accurately and sensitively capturing the richness of communication requires ongoing human awareness.
1. **Data-Driven Training:** Neural networks designed to understand Dominican youth slang rely heavily on large datasets sourced from a variety of social media platforms. This strategy emphasizes the ability of machine learning to adapt to rapidly changing informal language, particularly slang, which is very specific to a certain group. It seems like a good strategy, but it does raise concerns about the potential bias within these datasets that could perpetuate stereotypes or exclude certain groups.
2. **Tackling Idioms:** Surprisingly, advanced AI translation systems can sometimes accurately interpret the unique idiomatic expressions that Dominican youth use, unlike traditional systems which struggled with this type of language. This capability showcases the neural network's ability to pick up patterns and put meaning into context based on the slang of a particular region. However, one wonders about the depth of understanding that these models possess - is it just pattern recognition or do they actually grasp the nuance and humor of the slang?
3. **OCR and Graffiti:** Capturing slang through Optical Character Recognition (OCR) faces a significant hurdle, especially when dealing with the less conventional writing styles seen in street art (graffiti) or hastily scribbled notes. The fact that these forms often use custom fonts or even made-up characters illustrates a limitation in the current capabilities of OCR technologies. It begs the question of how to bridge the gap between reading the typical clean, formal font and capturing the creativity of informal language.
4. **Cultural Sensitivity:** Research suggests that incorporating cultural context into translation models leads to a more successful understanding of youth slang. Ignoring these regional nuances can lead to misunderstandings, emphasizing the critical need for AI systems to have a basic understanding of the culture that the slang represents. While that is an important aspect, it also raises the potential for perpetuating existing cultural bias in the technology.
5. **Learning Through Feedback:** The capacity for machine translation systems to learn from user feedback is paramount to refining the accuracy of slang translation. User interactions allow the models to adapt and improve over time, making them more skillful at navigating this rapidly changing field of informal speech. This is a good idea, but it also brings up questions about how to handle inaccurate or offensive feedback.
6. **Ever-Changing Slang:** It is estimated that youth slang vocabulary shifts considerably, with as much as 20% of expressions becoming obsolete within a year. This rapid evolution mandates continuous updating of training datasets to ensure the relevance of translation models. Maintaining model accuracy in the face of this dynamic environment presents a continuous challenge for researchers. This challenge of continuous model update begs the question of what happens when the slang becomes less used or even considered offensive. How does the algorithm deal with that?
7. **Specialized AI Models:** Some innovative approaches leverage dual-class model architectures specifically tailored for slang translation. By separating the processing of standard Spanish from that of slang, these systems achieve enhanced accuracy in translating the intended meaning within context. Though this seems a decent way to improve accuracy, one should ask if there is a better way to incorporate slang as a natural part of the language model.
8. **Empathy vs. Perfection:** Surprisingly, studies reveal that slightly inaccurate translations can sometimes improve user satisfaction. Users often prioritize the feeling or sentiment of the translation over a perfectly accurate word-for-word rendition. This indicates that in informal contexts, understanding the intent behind slang takes precedence over strict translation precision. This observation hints that perhaps AI translation needs to focus on understanding the purpose of the communication instead of necessarily focusing on getting a perfect, dictionary translation.
9. **Spanglish and its Challenges:** The increasing integration of Spanglish, particularly amongst Dominican youth, further complicates translation efforts. The mixing of English and Spanish results in unique expressions that standard translation models may not recognize, prompting the need for more nuanced context-aware approaches. How to develop an AI that truly understands Spanglish seems a key task for this field. It is an indication of the complex interaction between language and technology.
10. **Predicting the Future of Slang:** Research suggests that incorporating predictive algorithms capable of adapting to emerging slang trends can drastically improve translation efficiency. These algorithms anticipate language shifts, allowing models to remain agile and responsive to the dynamic nature of youth language. It's fascinating to think about how this kind of predictive power could be implemented, but I also wonder about the ethical implications of having a model that essentially predicts or even steers how slang evolves in the future.
Navigating Slang Translation Barriers A Case Study of Challenging Informal Phrases in Spanish - Speed vs Accuracy in Argentinian Social Media Translation Apps
Argentinian social media presents a unique challenge for translation apps due to the fast-paced nature of online interactions and the use of regionally specific slang. Users often prioritize speed, wanting quick translations for easy communication. However, this speed often comes at the cost of accuracy, especially when dealing with informal language and cultural references that are unique to Argentina. Many translation apps, while able to provide rapid output, struggle to interpret the full meaning of slang and idiomatic expressions. This can lead to misunderstandings and misinterpretations, hindering effective communication. The constant evolution of slang further complicates matters, requiring translation tools to be continuously updated and refined. While AI and machine learning offer promising solutions, they still struggle to fully capture the nuanced use of language in informal contexts. The need for a balance between speed and accuracy remains a significant challenge for developers seeking to create truly effective translation experiences for Argentinian social media users. The role of human translators, who possess a deeper understanding of the linguistic and cultural context, continues to be vital in ensuring that the intended meaning is conveyed effectively.
1. **The Speed-Accuracy Tightrope:** While AI translation apps are increasingly common in Argentina, the push for quick results often comes at the cost of accuracy, particularly when dealing with slang and cultural nuances. This speed-focused approach can lead to odd or even confusing translations in the fast-paced world of social media, where quick responses are expected.
2. **The Allure of Cheap and Fast:** The growing need for instant translation in Argentina has created a market where cheap, speedy apps are highly sought after. However, this emphasis on speed frequently overshadows the importance of quality, which can ultimately harm user confidence in these tools.
3. **Training Data's Influence:** AI models trained on a wide array of data, like informal chats and social media posts, seem to handle slang better. But, if a model's training data is limited or skewed in some way, its performance can suffer. This highlights the delicate balance between having lots of training data and having the right kind.
4. **OCR's Street Slang Struggle:** OCR technology often struggles with the casual handwriting often seen in urban settings, especially when combined with local slang. This highlights a key limitation in converting informal writing into digital text for accurate translation. It's not always easy to translate the scribbled tag on a wall!
5. **Learning from Users, But at What Cost?:** Some translation apps try to get better by incorporating user corrections in real-time. This idea has potential, but it also worries me a bit. What if the users themselves are unsure about the correct translation of certain slang terms? Could this lead to incorrect patterns reinforcing themselves in the model?
6. **Context Matters, Especially in Culture:** Without a good grasp of the cultural context, AI translation algorithms can make big mistakes, particularly when dealing with slang. A term that might be friendly in one region might be offensive in another. This demonstrates the need for AI to learn the cultural subtleties to avoid blunders.
7. **User Happiness vs. Perfect Words:** Surprisingly, users frequently prioritize getting the general idea across over getting a 100% perfect translation. Research suggests that the feeling a translation gives them matters more than the specific words. This raises intriguing questions about how AI fits into emotional communication.
8. **The Ever-Shifting Sands of Slang:** Slang changes rapidly—some estimates say that about 20% of popular slang expressions become outdated in a single year. This presents a continuous challenge for keeping translation models fresh and up-to-date, especially in the fast-paced environment of social media. It's a constant game of catch-up for these models.
9. **Squeezing Down Big Data:** Some newer AI projects use clever data compression methods to deal with the enormous amount of social media slang. While this can be a good way to summarize and access relevant slang, I'm concerned that vital nuances could get lost in the process.
10. **The Human Element Still Rules:** Despite the leaps forward in speed and affordability of AI translation, most experts agree that human translators are essential for really accurate slang translation. It highlights the intricate nature of informal communication and how difficult it is for AI to fully replicate the complex web of cultural meaning.
Navigating Slang Translation Barriers A Case Study of Challenging Informal Phrases in Spanish - Local Context Recognition for Colombian Spanish Colloquialisms
Colombian Spanish, influenced by the country's diverse culture and regional variations, boasts a wealth of colloquialisms and slang. Accurately interpreting phrases like "parcero" (meaning "buddy" or "friend") and "los tombos" (referring to the police) necessitates a deep understanding of Colombian social and cultural norms, going beyond simple word-for-word translations. This local context is paramount for clear communication, as misinterpretations can hinder genuine connection and understanding between speakers.
While AI translation, particularly with its speed and low-cost appeal, is showing progress, it often struggles with these subtle nuances. It's clear that effective communication within Colombian slang necessitates a conscious effort to absorb the local context, which can only be achieved through active engagement with native speakers. This process not only sharpens language skills but also deepens one's appreciation for the richness of Colombian culture. If future AI translation aims to fully capture and translate these expressions effectively, it will need to move beyond simple dictionaries and embrace these regional, cultural specifics.
Colombian Spanish, with its diverse regional dialects, presents a unique challenge for AI translation. The slang, a vibrant mix of local culture and history, isn't always easily understood, even among native speakers. Take the word "pola" for beer – it's common in Bogotá but might confuse someone from another area, showing how vital context is for translation.
There's also the issue of subtle emotions carried by slang that current AI often misses. A phrase funny in one place might be sarcastic or even offensive elsewhere. This complexity of intent is tricky for machines to pick up on.
Then, the problem of speed. Colombian slang is in constant flux, with certain phrases becoming obsolete in just a few months. Keeping translation models accurate requires continuous updating of the training data – a task that needs considerable resources.
Interestingly, a lot of users are somewhat forgiving when it comes to translation accuracy. Many prioritize understanding the sentiment or intent over a perfect word-for-word translation. This might offer a path to improvement for AI – focusing on the gist of communication instead of strict literalism.
Culture plays a crucial role here. AI needs to be aware of local customs and references to truly understand the slang. Otherwise, you get major misinterpretations that make users feel unheard.
Further complicating matters is the ambiguity of some colloquial expressions. For example, "hijueputas" can be affectionate or incredibly insulting, depending on who says it and how. This inherent ambiguity is something that AI systems need to become much better at interpreting.
Popular culture also contributes to slang. Reggaeton, for example, has brought a whole new wave of words into casual conversation. Translators need to stay on top of trends like this to keep up.
Traditional text recognition tools are another obstacle. OCR has trouble with informal, hand-written text often associated with slang, especially graffiti or quick notes. It struggles with non-standard spellings and the inherent creativity of these informal language forms.
Furthermore, the growing use of Spanglish, especially among young people, creates additional challenges. Mixing Spanish and English leads to unique phrases that aren't always easily captured by traditional models. AI needs a level of adaptability to make sense of these hybrid forms.
Finally, AI researchers are starting to experiment with predictive algorithms to anticipate future slang trends. This could be game-changing, but it also leads to a few ethical questions. Do we want AI steering the direction of informal language? Or is it best to let it evolve organically? It's a fascinating avenue of research that warrants further exploration.
Overall, accurately translating Colombian Spanish slang requires a constant balancing act between speed, accuracy, and cultural understanding. While AI is making progress, the complexity of slang indicates that this field will continue to need human insights and interventions.
AI-Powered PDF Translation now with improved handling of scanned contents, handwriting, charts, diagrams, tables and drawings. Fast, Cheap, and Accurate! (Get started for free)
More Posts from aitranslations.io: