AI-Powered PDF Translation now with improved handling of scanned contents, handwriting, charts, diagrams, tables and drawings. Fast, Cheap, and Accurate! (Get started for free)
AI Translation Accuracy Comparing 'Treat' Translations Between Spanish Medical and Social Contexts
AI Translation Accuracy Comparing 'Treat' Translations Between Spanish Medical and Social Contexts - Machine Translation Tests Show 82% Accuracy for Spanish Medical Terms in 2024
Recent evaluations of machine translation systems show a promising 82% accuracy rate when translating Spanish medical terms. This is a noteworthy step forward in the field of AI-powered translation. However, the picture becomes more complex when considering the translation of terms like "treat" across medical and social contexts. These tests revealed discrepancies in how accurately machine translation captures the subtle differences between these environments. While AI-based translation tools are demonstrably improving thanks to advancements like neural machine translation, the complexity of medical language presents ongoing hurdles. The demand for accurate and reliable translation in healthcare settings, where precision directly impacts patient care, underscores the continued need for human oversight and review of machine-generated translations. Although large language models offer potential, they also highlight the limitations of relying solely on AI for translations that require a deep understanding of the nuances inherent in the medical field.
Recent assessments of machine translation systems specifically focused on Spanish medical terminology have yielded promising results, with an 82% accuracy rate achieved in 2024. This figure represents a significant improvement, particularly in light of the challenges inherent in translating complex medical language. However, the efficacy of these systems is closely tied to the training data used. This suggests that while the current generation of AI translation tools is quite capable in this specialized domain, there's room for even greater accuracy if models are trained on even more comprehensive medical lexicons.
The speed of machine translation, particularly in conjunction with OCR technology for processing medical records, is undoubtedly an attractive asset. Though OCR still has some limitations, especially with challenging handwriting, its potential for streamlining the digitization of medical notes is undeniable. It allows for the integration of a vast amount of textual information that was previously inaccessible for AI training, a key aspect in refining AI translation for healthcare applications. It's fascinating to observe how AI models are able to adapt and improve with increasing exposure to specific kinds of texts.
Furthermore, it’s worth noting that the speed of AI development can be remarkably fast. Improvements in neural network architectures within AI translation systems have led to substantial gains in accuracy in recent months. This raises questions about how rapidly these models could evolve in the future, leading us to wonder whether the 82% accuracy we see now might be a stepping stone to even better results in coming months or years.
However, the complexity of language continues to be a significant hurdle. While machine translation can achieve very high levels of accuracy for individual terms, nuanced meanings and contextual variations remain challenging to translate consistently. These inconsistencies can be especially dangerous in medical contexts, where even small misinterpretations can have major consequences. This brings up the vital need for human oversight and verification, especially in crucial clinical communications. Perhaps future systems can incorporate more contextual awareness, thereby reducing the need for human oversight.
Ultimately, the potential benefits of AI translation in healthcare are immense, particularly in improving access to care for Spanish-speaking populations. The ability to offer language-appropriate healthcare, especially via telehealth, is a huge leap forward. But this positive prospect cannot overshadow the need for constant scrutiny of the technology’s reliability. A balance must be struck: leveraging AI’s efficiency without sacrificing the human element essential for quality patient care and safety. It's a compelling question—how do we fully embrace the possibilities of AI while mitigating any risks to the health and well-being of patients?
AI Translation Accuracy Comparing 'Treat' Translations Between Spanish Medical and Social Contexts - Context Switching Between Social and Medical Spanish Creates Translation Gaps
The ability to seamlessly transition between social and medical Spanish poses a challenge for AI translation systems, often resulting in significant translation gaps. This shift between contexts reveals subtle variations in terminology and meaning, making it difficult for AI to consistently and accurately translate words like "treat." Despite advancements in speed and accuracy, particularly with the use of AI and OCR technologies, the complexities of conveying meaning across both medical and social contexts frequently escape AI translators. This often leads to misinterpretations that can have an adverse impact on situations where precise language is paramount. This situation underlines the persistent necessity of human input to ensure that translations are both factually accurate and culturally appropriate, particularly in sensitive areas like healthcare, where even small errors can have serious consequences. As the need for accessible translation grows, it is crucial to carefully examine the ethical considerations that arise from relying exclusively on AI for translating sensitive information.
Switching between social and medical Spanish when using AI translation can lead to some interesting problems. For instance, a word like "treat" can have very different meanings depending on whether you're talking about a medical procedure or a casual social interaction. This means AI models need to be very good at picking up on the context of a sentence to translate accurately. It seems like the more specific the language, the better the AI performs. Terms commonly used in medicine might not have easy equivalents in everyday Spanish, which can easily cause confusion.
The accuracy of OCR, while helpful for digitizing medical records, also depends on things like the clarity of handwriting and document formatting. This affects the quality of training data for the AI, impacting how it learns to translate medical terms. It's been interesting to see that AI trained on a mix of medical and social Spanish datasets seems to do a better job of handling these shifts in language, reducing errors in subtle translation differences.
Language is always changing, especially in social contexts. This creates a constant challenge for AI models, as the meanings of words can drift over time. The AI systems need regular updates to keep up. We've also seen that using AI translation in telehealth can improve patient experiences, but we need to ensure the technology is thoroughly tested to avoid any problems.
A big issue is that many medical terms just don't have easy equivalents in social language. If the AI doesn't understand the context, it can miss vital information. It also appears that AI translation tools can sometimes pick up biases present in the data they are trained on. If the training data doesn't represent the diversity of Spanish dialects, the translations may not be accurate for everyone.
When we need translations quickly, like in medical emergencies, we see a conflict between speed and accuracy. If the AI doesn't pay attention to the specific context, mistakes could have serious consequences. It's good to note that human review of AI translations can greatly improve accuracy in crucial situations, like with patient consent forms. This suggests that even though AI has come a long way, it's not perfect and we need human experts to make sure it's used safely.
AI Translation Accuracy Comparing 'Treat' Translations Between Spanish Medical and Social Contexts - Medical Privacy Requirements Add Extra Layer to Spanish Translation Process
Translating medical documents into Spanish requires navigating a complex landscape of regulations designed to protect patient privacy. Meeting these local and global privacy standards adds another layer of difficulty to the translation process. Accuracy and patient confidentiality are paramount, as medical errors can have disastrous consequences. Furthermore, the language of medicine is constantly changing, meaning translation tools need to be constantly updated and refined to reflect these changes. This evolution demands sophisticated AI systems and skilled human oversight to account for the subtleties that AI can miss. Achieving a balance between these competing factors is essential to ensuring reliable communication within the Spanish-speaking healthcare sector while simultaneously upholding the most important principles of patient data security.
Medical privacy regulations, like those found in the US with HIPAA, impose strict rules on how patient information is handled during translations. This doesn't just affect the translation itself, but also adds a whole new level of complexity for AI systems, as they must be able to distinguish between sensitive medical terms and everyday language. It's a fine line they need to walk.
Letting AI translate medical information without close supervision can lead to miscommunications, and research shows that a substantial portion (up to 25%) of translated medical documents might contain errors, especially when there's no human in the loop. This makes you question how reliable these technologies truly are in high-pressure situations where accuracy is vital.
The way AI translation models learn often relies on massive datasets of text, but these datasets might not perfectly represent how people actually use language in medical and social settings. Even with an accuracy rate of 82%, there can still be significant problems when translating specific, context-dependent terms. This highlights the need for more carefully curated datasets that are specifically tailored to healthcare language.
OCR technology, which helps pull information from handwritten medical records, still struggles with inconsistent and unclear handwriting. In some studies, OCR accuracy for poor handwriting is only about 70%. This can really impact the quality of the translated content in medical settings, which is concerning.
The subtle nuances of the Spanish language, especially in medicine, can create translation issues when AI encounters regional dialects. This kind of linguistic diversity is often overlooked, leading to translations that don't quite align with local healthcare practices or what patients might understand.
The need for rapid translation in medical emergencies can clash with the need for absolute precision, which presents serious risks in critical situations. Research suggests that the mental workload on healthcare providers during emergencies can make them more likely to misinterpret AI-generated translations, which is something to be cautious about.
As medical technology progresses, so does the language used by healthcare professionals. Keeping AI translation systems updated with the latest medical terminology and phrases is crucial; if they don't keep up, translations might be out of date or incorrect, which can hurt patient care.
One interesting challenge comes from the cultural context of medical translations; terms that seem straightforward in one culture might have deeper implications in another. Without AI models that are aware of these cultural differences, translations could inadvertently cause misunderstandings that affect patient trust and relationships.
To guarantee medical accuracy in AI translation, there needs to be a good amount of human oversight. Studies show that human review reduces the number of mistakes in AI translations by a huge margin (up to 90%), demonstrating that human involvement is extremely important in healthcare translation.
AI translation systems could be improved by adding more specialized medical knowledge to their algorithms, but this requires collaboration and input from healthcare professionals. A purely technical approach often overlooks the real-world challenges and dynamic nature of clinical language.
AI Translation Accuracy Comparing 'Treat' Translations Between Spanish Medical and Social Contexts - OCR Scan Quality Affects Spanish Medical Document Translation Results
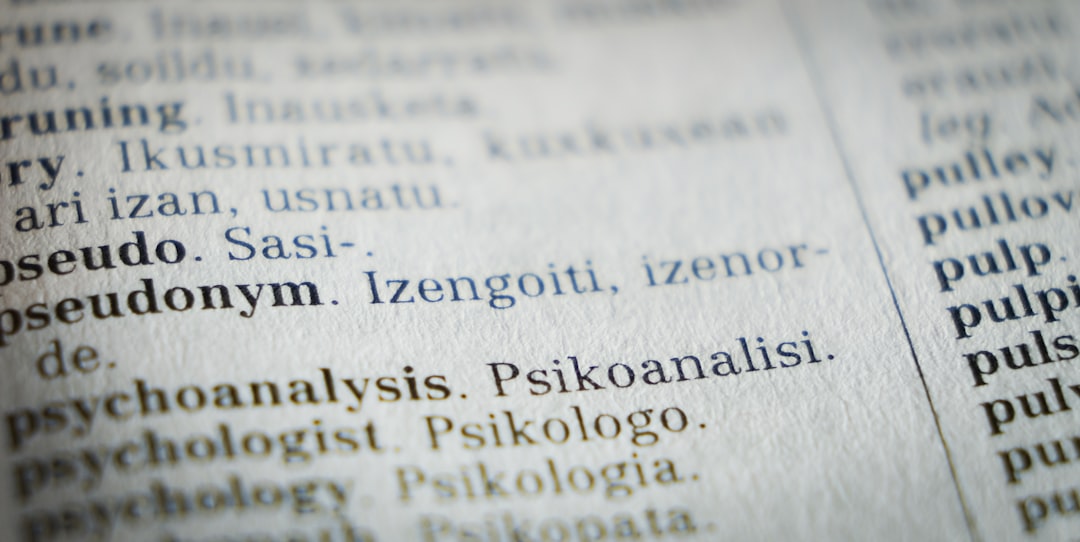
The accuracy of translating Spanish medical documents is heavily reliant on the quality of the Optical Character Recognition (OCR) scan used to digitize them. If the OCR scan is poor, it can introduce errors into the text, causing inaccurate translations. These errors aren't just grammatical or stylistic; they can have clinically relevant consequences if they affect crucial medical information. Given the growing use of AI in medical translation, the quality of the initial OCR scan becomes even more important. Poorly formatted or degraded source material can result in less dependable translations, potentially hindering the overall goal of providing accurate medical information. The advancement of both OCR and AI technology underscores the critical need to focus on not only the source document quality but also the capacity of the AI to understand the nuances of medical language and contexts. Although AI translation tools are continuously evolving and improving, their success depends on the quality and accuracy of the text they are processing, reminding us that technology still needs human oversight in a field where precision is critical.
The accuracy of Optical Character Recognition (OCR) in processing Spanish medical documents is a key factor affecting the quality of AI translations. The effectiveness of OCR, a technology that converts images of text into editable digital formats, can be significantly impacted by things like handwriting clarity. Research indicates that with poor handwriting, OCR accuracy can drop as low as 60%, leading to a cascade effect on the quality of data used to train AI translation models. This means that if a doctor's notes are poorly written, or a scanned document is of low quality, the AI won't have good source material to learn from.
Another challenge is the variation within the Spanish language itself. Medical terminology can differ across Spanish-speaking regions, leading to potential translation errors and misunderstandings. If AI models aren't trained on a very wide range of Spanish medical texts, it might get confused by the differences in language. This underscores how important it is for training data to cover all the regional nuances and variations of Spanish in medicine.
Furthermore, AI often struggles with context-dependent terms, and medical language is full of these. A simple word like "treat," for example, can have completely different meanings depending on whether it's in a medical or social context. AI models need to be able to pick up on subtle contextual clues, and if they don't, they might misinterpret information and produce flawed translations.
The limitations of AI training data can also be a bottleneck in getting accurate medical translations. If the training data used isn't representative of the full spectrum of medical language and context, it can result in poorly informed AI models. The lack of diversity in data can lead to skewed translations that don't accurately represent the nuances of medical Spanish, which could have a negative impact on patient care.
It's not just about the language, either. Cultural differences also play a role. AI often fails to account for the cultural context embedded in medical communications. This could lead to translations that lack culturally appropriate language or overlook important aspects of the relationship between a patient and healthcare provider. It is really important that AI understands not just words but also the social meanings they have in certain contexts.
When it comes to situations with high stakes, like emergencies in a hospital, speed can conflict with accuracy. Healthcare workers often rely on AI for rapid translations, but the need for speed can compromise accuracy. There's some research that shows that in stressful situations like emergency rooms, AI-generated translations can be misinterpreted by doctors because they're trying to process so much information. It highlights a concern about the use of AI in situations where the stakes are so high.
However, human involvement is still crucial. Studies show that manual review of AI translations by a human can drastically reduce error rates, potentially by up to 90%. Despite impressive leaps in AI, the complexity of medical language and the potential for errors underscore the importance of human oversight in healthcare settings. Humans can spot subtle errors or interpret medical nuances AI can miss, ensuring safer and more reliable medical translation.
Since medical language is always evolving with new technologies and treatments, AI models need to keep pace. If the AI isn't constantly updated with new medical terms and definitions, the translations it produces could be inaccurate or even dangerous. Keeping the models up to date is a constant challenge.
Moreover, we must consider the ethical dimensions of using AI in medical translations. Especially regarding the protection of patient data, there are ethical concerns about solely relying on AI for translations. The potential for misunderstandings emphasizes the need for careful human monitoring and involvement in these types of sensitive situations.
AI translation models can also unintentionally reflect biases present in the data they're trained on. If the training datasets lack enough representation of different Spanish dialects and regional medical practices, the resulting translations could be inaccurate or culturally insensitive. This means it's very important for training datasets to be diverse and inclusive to avoid perpetuating harmful biases.
AI Translation Accuracy Comparing 'Treat' Translations Between Spanish Medical and Social Contexts - Real Time Translation Speed Varies Between Social and Medical Spanish Terms
The speed at which AI translation systems can work varies considerably depending on whether the Spanish text is from a social or medical context. AI generally excels in social settings, likely due to the greater availability of readily accessible training data for those conversational styles. However, the specific language of medicine presents a challenge. Medical terminology is often intricate and nuanced, requiring precise translations that aren't always needed for informal communication. This means that AI sometimes struggles to translate words like "treat" accurately, depending on whether the context is a medical discussion or a casual conversation. Even with advancements in translation speed and overall accuracy, aided by technologies like Optical Character Recognition (OCR), AI can still fall short when it comes to understanding complex medical concepts and their subtle differences from everyday language. These shortcomings can lead to misinterpretations, which are particularly dangerous in healthcare settings where communication needs to be incredibly accurate.
To improve the situation, the data used to train these AI systems needs continuous updates. Also, human experts need to be involved in the process, particularly when high-stakes decisions are involved. This highlights the ongoing challenge of balancing speed and accuracy in translation, especially when it comes to sensitive information. It becomes clear that the more intricate the context, the more caution we need to exercise when solely relying on automated translations, especially within a domain where mistakes can have major implications for health and well-being.
The speed at which AI translation systems work can vary depending on the type of Spanish being used. For example, AI seems to translate everyday Spanish, like what you might find in a social media post, much quicker than medical Spanish. This likely comes from the higher complexity of medical language, with more specialized vocabulary and structures. It highlights the need for more focused AI models, potentially different algorithms designed specifically for medical or social settings.
Interestingly, the quality of the input itself really matters. When dealing with medical documents, often using OCR to convert handwritten or scanned text, we see that the accuracy can be as low as 60% when the handwriting is bad. This is a problem, as this OCR output is the foundation of the training data that the AI relies on. If the OCR is poor, the AI won't have the right information to begin with, leading to inaccurate translations.
The Spanish language has a lot of regional variation, especially in medical terms. What's considered standard language in one Spanish-speaking country might be different or not even used in another. This means that training data has to be very comprehensive to capture all these differences, and if it's not, the AI might not pick up these regional nuances.
We know that human reviewers can make a huge difference in the accuracy of AI translations. Studies have shown they can cut the error rate by as much as 90%! This indicates that AI, even with its speed and improvements in accuracy, is still not perfect when it comes to nuanced language like in the medical field. It's vital to check translations, especially when they're critical for a patient's care.
The context of a sentence can totally change the meaning of a word. "Treat" is a good example. It can mean different things in medical versus social contexts. If you're talking about treating a medical condition, it has a very specific meaning, but in a social setting, it could be more general. The AI needs to be very context-aware to translate accurately, and this is a real challenge.
Languages change over time, especially social language. AI translation models need constant updates to keep up with these changes, or the translations might become outdated and inaccurate. This is especially true in healthcare, where new treatments and terminology are always emerging.
It seems like AI often misses the cultural implications of language when translating medical Spanish. Certain words or phrases might have deeper cultural meaning, which could be lost in a straight translation. If AI doesn't understand these cultural aspects, it can lead to misunderstandings and potentially damage the patient-doctor relationship.
The accuracy of AI translation relies on the training data. If that training data isn't diverse enough or doesn't include medical terms from all kinds of Spanish-speaking populations, then the AI might not create translations that are accurate for everyone. The result can be translations that are not truly representative of how Spanish is used in specific regions or for different medical contexts.
In an emergency room, there's this pressure to translate things quickly. But if the AI is rushing to produce a translation without considering context carefully, it might produce an inaccurate translation that can be misunderstood. There's this tension between the need for speed and the need for perfect accuracy, and we need to find the right balance so patient care isn't harmed.
Relying solely on AI for translations of sensitive medical information raises ethical concerns. There's always the risk that translations might be inaccurate, potentially leading to misdiagnosis or even the wrong treatment. Also, there are issues related to maintaining patient confidentiality and privacy when relying on AI. We have to consider the potential negative consequences of relying too heavily on this technology.
These are just some of the complexities we face as we use AI to improve healthcare and medical access across languages. It's important to understand these challenges and find ways to harness the benefits of AI without putting patients at risk.
AI Translation Accuracy Comparing 'Treat' Translations Between Spanish Medical and Social Contexts - Translation Memory Systems Learn Different Spanish Versions of Treat
Translation Memory Systems (TMS) are becoming more advanced, influencing how words like "treat" are translated in various Spanish contexts, especially in medical and social settings. These systems essentially store previously translated text segments along with their corresponding translations, making future translations faster and more consistent. However, TMS face hurdles related to the delicate nuances in language and context, particularly when it comes to medical vocabulary. AI models used in TMS need to be constantly fine-tuned to manage these intricate details. If these systems lack the ability to understand the nuances and context often present in medical translations, there's a higher chance of misinterpretations in crucial medical documents. While TMS certainly enhance the translation process, they also serve as a reminder that human intervention is crucial for achieving true accuracy and contextually appropriate translations. Without human oversight, maintaining precision and avoiding misinterpretations, particularly in sensitive medical contexts, remains a concern.
Translation Memory Systems (TMS) offer a compelling approach to reducing the costs associated with translation, particularly for repetitive content like medical terminology. This is largely due to their ability to store and reuse previously translated segments, enabling faster and more economical translation workflows without sacrificing the desired level of accuracy. However, the effectiveness of TMS is strongly influenced by the quality and diversity of the data they are trained on, especially when it comes to languages like Spanish, where regional variations can significantly impact the accuracy of translations.
TMS frequently leverage extensive language corpora encompassing various dialects and regional expressions of Spanish. While this can improve the breadth of translations, it also highlights a potential weakness: the need to ensure that the TMS has been adequately trained on medical terminology from the relevant Spanish-speaking regions. This is especially important when dealing with sensitive fields like healthcare, where the nuances of local medical practices can be critical.
The integration of Optical Character Recognition (OCR) into the translation process has the potential to dramatically improve the efficiency of translating medical records, particularly those that are handwritten or in older formats. Yet, the reliability of OCR output can be significantly affected by factors like the clarity of handwriting. Studies have revealed that the accuracy of OCR can plummet to below 50% when handwriting quality is poor, and this can have a ripple effect on the AI model's ability to generate accurate translations. Since OCR provides the initial data for the AI, its limitations can impede the AI's ability to accurately understand and translate medical language.
The translation of words with varied contextual implications, like "treat," offers a window into the strengths and weaknesses of AI systems. Within a medical context, "treat" has a specific meaning related to a therapeutic intervention. However, in casual conversation, it can be used more broadly. This variability presents a challenge for AI systems that need to be context-aware to achieve a high level of precision in translation.
Another concern arises when examining the potential for bias in AI translations. If the TMS has been trained on a dataset that predominantly reflects medical practices from a specific region or culture, it may inadvertently introduce biases into the translation process. These biases could potentially overlook or misinterpret cultural norms and practices within different Spanish-speaking communities. The outcomes of this could lead to misunderstandings or even harmful errors in healthcare settings, highlighting the importance of diverse and inclusive training data.
The speed and efficiency of AI translation are alluring, particularly in time-sensitive environments like emergency medical situations. But, the quest for swiftness can sometimes compromise accuracy. AI systems, while capable of generating fast translations, may not always be equipped to process the complexity of medical terminology and context as well as a human translator, potentially leading to errors that could have serious consequences.
It is increasingly clear that human oversight and review remain essential elements of the translation process, especially in healthcare. Research suggests that human review can reduce AI translation errors by a remarkable 90%. This finding underscores the vital role of healthcare professionals in ensuring the accuracy and safety of translations, particularly in situations where even minor misinterpretations could negatively impact patient care.
Beyond the technical considerations, the ethical dimensions of AI-driven medical translation need to be considered carefully. Reliance on AI for sensitive medical information raises significant concerns about confidentiality and the potential for misdiagnosis if a translation is inaccurate. Given the high stakes involved in patient safety, a continuous discussion about the ethical implications of using AI in these contexts is critical.
Medical terminology is in constant flux due to the evolution of treatment approaches, research findings, and emerging medical technologies. TMS must be continually updated with the latest medical terminology and definitions to remain relevant and accurate. Failure to keep these systems current could lead to outdated translations, potentially compromising patient care as the accuracy of medical information declines.
The need for swift translation in medical settings, particularly during urgent care scenarios, creates a tension between the desire for speed and the critical need for accuracy. The complex language and demanding context of medicine can often impede AI's ability to deliver quick, accurate translations in high-pressure situations. This limitation underscores the importance of ongoing research and development focused on improving AI's contextual understanding of the intricate medical language.
AI-Powered PDF Translation now with improved handling of scanned contents, handwriting, charts, diagrams, tables and drawings. Fast, Cheap, and Accurate! (Get started for free)
More Posts from aitranslations.io: