AI-Powered PDF Translation now with improved handling of scanned contents, handwriting, charts, diagrams, tables and drawings. Fast, Cheap, and Accurate! (Get started for free)
AI Translation Breakthroughs Analyzing JWorg's 1,084-Language Milestone in 2024
AI Translation Breakthroughs Analyzing JWorg's 1,084-Language Milestone in 2024 - JW.org's 1,084-Language Milestone Expands Global Reach
JW.org's recent achievement of offering content in 1,084 languages, including 100 sign languages, is a significant step in expanding its global reach. This makes it the world's most translated website, highlighting the organization's dedication to making religious information available to a wide audience. The site provides a diverse array of materials, encompassing articles, videos, and audio content, all focused on Bible study and related teachings. Dedicated teams translate and adapt the original English content, ensuring the message resonates with the cultural and linguistic nuances of each language. Interestingly, the effort also extends to developing tools like the JW Language app, which assists with language learning and communication. This focus on language access suggests a continued aim to foster inclusivity and accessibility across their global community. While the sheer number of languages might appear impressive, the speed and accuracy of the translation processes, especially given the complexity of different language structures, could potentially be a point of further inquiry and potential optimization.
JW.org's achievement of 1,084 languages is quite impressive, especially considering the rapid advancements in AI translation. It's fascinating how the website's translation efforts have grown, potentially leveraging some of these cheaper, faster AI-powered methods. Imagine the sheer volume of content—over 200,000 pages—translated and made available across so many language variations.
While the use of AI translation seems likely given the scale and pace, maintaining quality across such a diverse linguistic landscape must be a significant challenge. OCR probably plays a role, helping them to digitize older materials and speed up the input process. But with less commonly spoken languages, there's bound to be a reliance on smaller training datasets. It's likely this is where quality may suffer, potentially resulting in some translation quirks or inaccuracies. It would be interesting to see how JW.org manages the trade-off between leveraging AI's speed and ensuring translations are culturally appropriate and accurate.
The expansion into sign language is particularly noteworthy, suggesting a thoughtful consideration of accessibility beyond just spoken languages. I wonder how AI handles the nuances of sign languages – the visual component presents a different challenge compared to traditional language translation.
Their efforts to provide free Bible study programs in so many languages underscore the power of language technology in breaking down cultural barriers and promoting inclusivity. But even with AI speeding things up, it's a massive undertaking and one that probably requires both human translators and technology. The future of translation, with its increasing automation, will undoubtedly affect the roles and skills needed in this field. We might see a shift toward specialists who focus on refining and ensuring accuracy in AI-generated content, especially in specialized or culturally-sensitive materials.
AI Translation Breakthroughs Analyzing JWorg's 1,084-Language Milestone in 2024 - NLLB200 AI Model Tackles 200 Languages for Metax
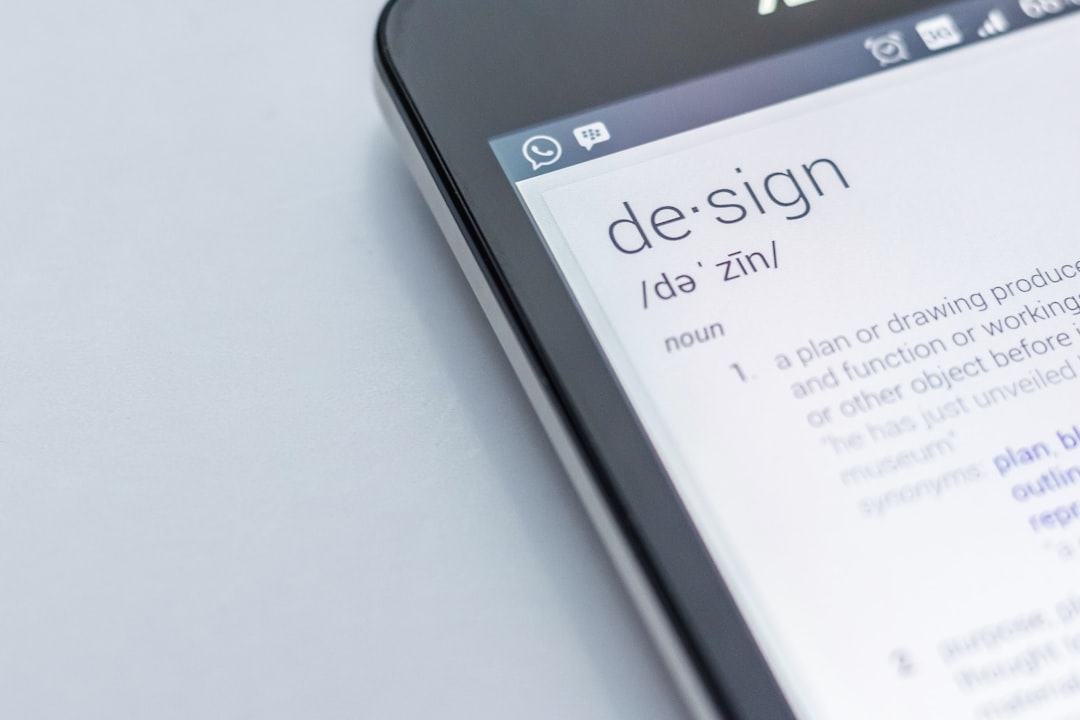
Meta's NLLB200 model represents a notable step forward in AI translation, aiming to bridge the gap for 200 languages. It's part of Meta's larger "No Language Left Behind" effort to make high-quality machine translation available for a wider range of languages, including those with limited digital resources. The model has been rigorously evaluated across these 200 languages, using a dataset specifically designed for this purpose, and Meta claims a significant 44% leap in translation quality compared to earlier models.
The model, a massive 545 billion parameter Mixture of Experts system, is built to be both accurate and efficient. Furthermore, making the model open-source offers developers and researchers greater access to these advanced translation capabilities. While this initiative potentially fosters inclusivity and cross-language communication, challenges persist in maintaining high-quality translations, particularly across diverse cultural contexts. Ensuring that translations are both accurate and culturally appropriate, especially for languages with smaller datasets, will continue to be a key focus in this rapidly evolving field. The speed at which AI translation can produce outputs will likely remain a major benefit but needs careful management to not compromise the essence and intention of the original message.
Meta's NLLB200 AI model is a significant development in the field of machine translation, tackling the ambitious goal of translating between 200 languages. This project, part of Meta's "No Language Left Behind" initiative, aims to bridge the gap for languages that are often overlooked by traditional translation systems. What's impressive is their focus on low-resource languages like Asturian, Luganda, and Urdu. These languages typically have limited datasets for training AI models, but NLLB200 seems to handle them surprisingly well.
They've achieved this through various techniques like unsupervised learning, which allows the AI model to learn language patterns without needing vast quantities of human-labeled data. It seems they've combined advanced transformer architectures with self-supervised training to boost translation quality and speed. This approach is especially promising because it allows the model to adapt quickly to new language pairs and different translation tasks.
One interesting aspect of NLLB200 is its emphasis on context. In my experience, AI translation often struggles with phrases that can have multiple meanings, leading to some strange mistranslations. NLLB200 appears to handle this issue better than some existing translation models.
I think OCR technologies play a role in feeding data to the NLLB200 model, too. This could be crucial in converting older documents or images into digital text for faster processing.
Furthermore, NLLB200 has a broader goal of translating not just the literal meaning but also the nuances of a language. Cultural references and idioms can be tricky for AI, but capturing those is important for effective translation. This highlights a potential challenge as well as an area where continued refinement is crucial.
While NLLB200 boasts a competitive translation speed, rivaling human translators in some cases, there's always a trade-off between speed and quality. Complex translations that involve cultural and contextual understanding will likely require human oversight for optimal results. Despite being a remarkable achievement, it's important to remember that AI translation technology still needs human monitoring to address subtleties and prevent unintended errors in more nuanced content. It'll be interesting to see how this model matures and helps us navigate the constantly evolving world of multilingual communication.
AI Translation Breakthroughs Analyzing JWorg's 1,084-Language Milestone in 2024 - Neural Machine Translation Enhances Accuracy Across Languages
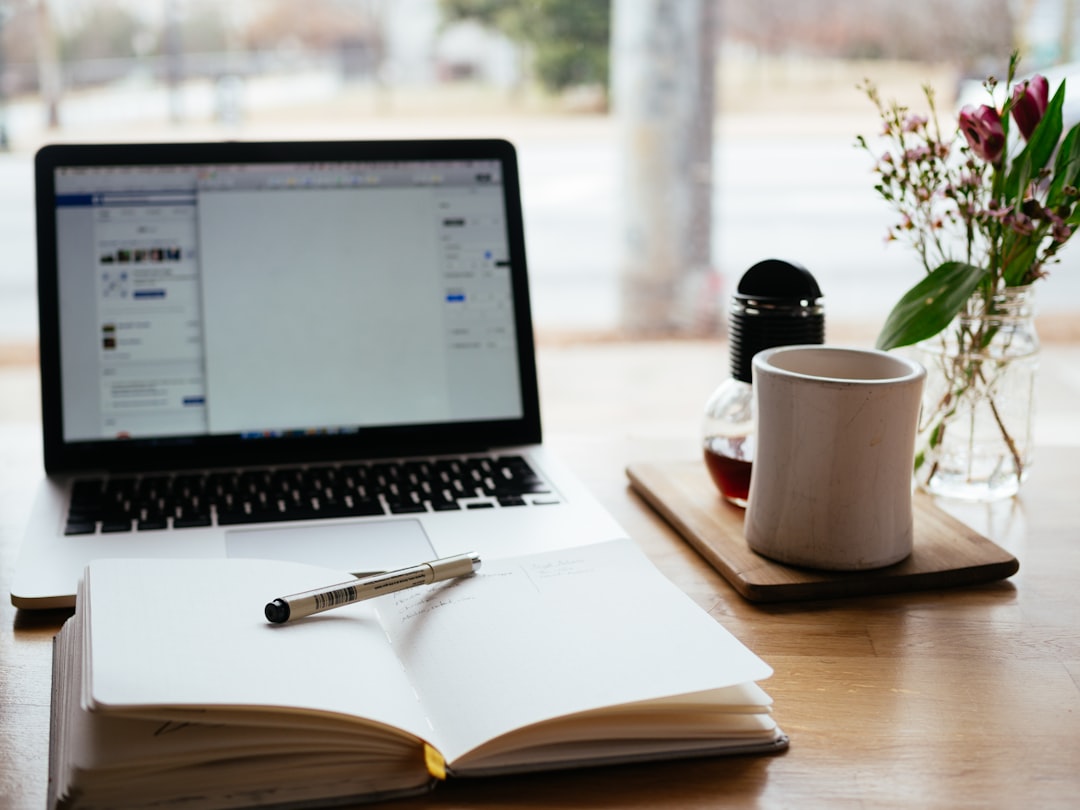
Neural machine translation (NMT) has significantly boosted the precision of translations across various languages. The field has seen rapid advancements, particularly in handling languages with limited digital resources. We've seen notable progress with models like the NLLB200, pushing the boundaries of AI translation by allowing accurate translations between 200 languages, a significant feat. This is especially useful for languages with smaller digital footprints, bringing the potential for improved translation quality to lesser-known languages. However, there's a constant need to ensure these powerful tools are used thoughtfully, respecting the nuances and cultural aspects that are embedded within languages. The need to strike a balance between speed and quality, while maintaining accuracy and cultural sensitivity across varied linguistic environments, remains a key challenge. It is a constant balancing act in the dynamic world of AI-driven language processing.
Neural machine translation (NMT) has demonstrably improved translation accuracy across a wide range of languages, significantly enhancing the overall quality of translated outputs. It's quite fascinating how these systems are getting better at handling the complexities of different languages. JW.org's 1,084-language milestone, achieved in 2024, highlights the potential of these technologies, though it also raises questions about the nuances of translation across such diverse linguistic landscapes.
One aspect that stands out is the ability of NMT to excel in certain language pairs, particularly those with closer linguistic relationships. For example, Spanish-Portuguese translation tends to be more accurate than, say, Romanian-Japanese, due to shared vocabulary and grammatical structures. This highlights the importance of understanding the underlying relationships between languages when designing and training these systems.
Another intriguing development is the increased emphasis on contextual understanding in NMT. Newer models utilize attention mechanisms to focus on the context of words and phrases within a sentence, leading to a marked improvement in the clarity and coherence of translations. This is a significant step forward from older statistical approaches.
Interestingly, some NMT models are now able to tackle low-resource languages more effectively by leveraging transfer learning. By applying insights gained from high-resource languages, researchers can potentially boost translation quality for languages with limited training data, bringing the benefits of AI translation to communities that previously had limited access.
While the speed of AI translation is undeniably advantageous, achieving optimal accuracy remains a challenge. Complex sentence structures, culturally-specific expressions, and nuanced meanings often require the human touch to ensure fidelity to the source content. This highlights the inherent trade-off between speed and precision in the translation process.
OCR, or Optical Character Recognition, plays a crucial role in the translation workflow, especially when dealing with older or digitized materials. OCR converts printed or handwritten text into digital format, paving the way for faster and more efficient translation pipelines. This technology is essential for preserving knowledge and making it accessible across languages.
Despite these advancements, the quality of AI-generated translations can fluctuate considerably depending on the language pair and the complexity of the text. It's important to dig deeper and explore why certain languages tend to see lower quality compared to others. There may be hidden factors at play related to language structure or available datasets.
Sign languages pose a particularly intriguing challenge for AI translation. Their visual-spatial nature presents a unique hurdle for models designed for spoken languages. Figuring out how to effectively translate sign language requires innovative approaches that capture the essential nuances of visual communication.
The structural differences between languages also present obstacles. Languages with divergent grammatical structures (like SOV vs. SVO) can produce awkward phrasing or lead to misinterpretations if not handled carefully by the translation system. This suggests a need for systems that are more adaptable to varying linguistic structures.
Idiomatic expressions continue to challenge NMT models. These culturally embedded phrases are difficult for AI to translate accurately. Developing models that can recognize and replace idiomatic expressions with equivalents in the target language is an ongoing research area.
Looking ahead, the future of translation may involve more collaborative human-AI partnerships. AI can provide initial translations, and human translators can step in to refine and ensure accuracy, especially for complex or sensitive materials. This hybrid model combines the strengths of both AI speed and human expertise, potentially leading to more reliable and culturally sensitive translation outcomes.
In conclusion, NMT has clearly demonstrated significant improvements in translation accuracy across diverse language groups. As these technologies mature, they hold tremendous promise for improving communication and understanding across cultures. However, ongoing research and development are necessary to address the remaining challenges, including nuanced language, cultural variations, and human oversight in critical situations.
AI Translation Breakthroughs Analyzing JWorg's 1,084-Language Milestone in 2024 - AI Translation Bridges Cultural Gaps in Global Communication
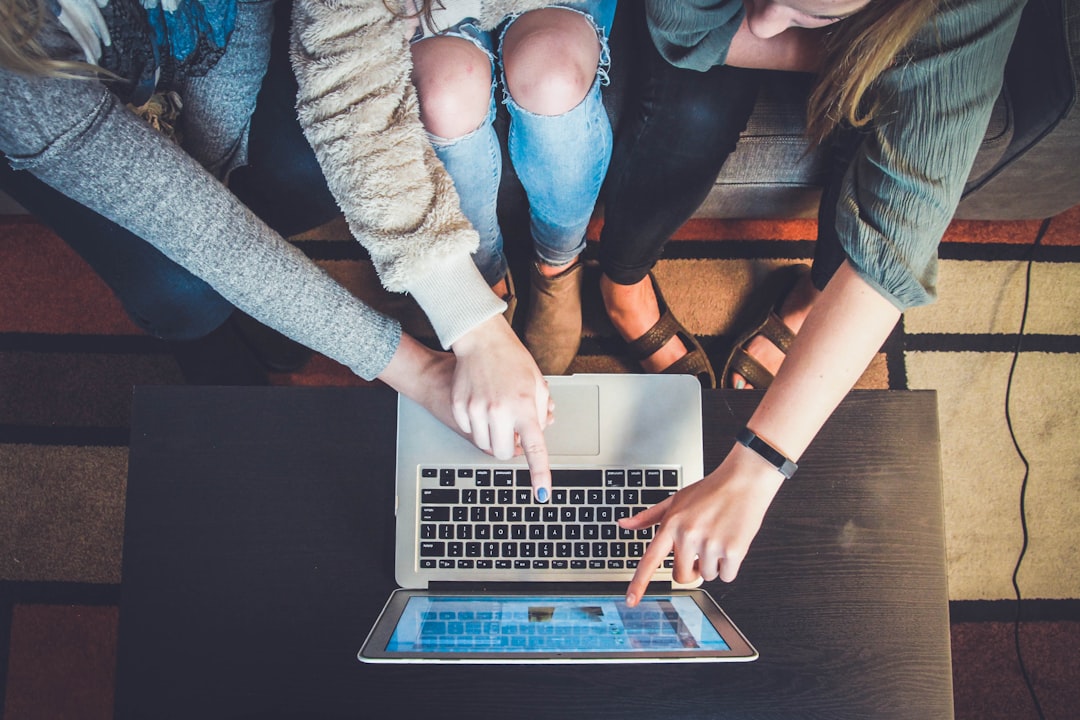
AI translation is rapidly becoming a key player in fostering communication across cultures. These technologies are evolving beyond simple word-for-word substitutions, increasingly capable of capturing the context and cultural subtleties of language. This represents a major shift from older translation methods that often struggled with these nuances, resulting in mistranslations and cultural insensitivity. The ability of AI to translate quickly and with increasing accuracy is a remarkable development. The drive towards broader language representation, evident in efforts like JW.org's achievement of 1,084 languages, emphasizes the power of AI in promoting global dialogue and connecting people from various backgrounds.
While the advancements in AI translation are encouraging, there are still hurdles to overcome. Maintaining translation quality for languages with limited digital resources is a challenge. Ensuring that AI-generated translations remain culturally sensitive and appropriate across diverse communities requires ongoing development. The path forward will likely involve a careful balance between the speed and efficiency of AI and the nuanced understanding that human translators bring to the table. Ultimately, the goal is to ensure that these technologies promote true understanding and connection, not just a superficial exchange of words.
AI translation tools have shown remarkable speed and scale, often outpacing human translators in terms of sheer volume. However, maintaining accuracy, especially when it comes to complex sentences or cultural nuances, remains a challenge. Models like NLLB200, trained on enormous datasets, are pushing the boundaries of AI translation, particularly for languages with fewer digital resources. This helps bridge the gap for languages previously overlooked, but it also brings up questions about the quality of translations when the training data is limited.
Interestingly, AI often struggles more with lesser-known languages, as they tend to have smaller training datasets. This makes you wonder how dependable these translations really are in those situations. OCR has become crucial in the whole translation process, making it possible to quickly convert scanned documents or images into usable text formats. This is invaluable when dealing with historical archives or other less accessible forms of information.
Advanced AI systems now employ attention mechanisms to better understand the context within sentences, rather than just processing words in isolation. This approach leads to more fluid and coherent translations, which is especially helpful for complex sentence structures. However, challenges remain when dealing with languages that have very different grammatical structures. The resulting translations can sometimes feel awkward or miss the original intent.
While AI translation is expanding access to information, it's important to remember that human oversight is still crucial, especially for content with cultural implications. We need to ensure the heart of the message isn't lost in the translation process. Sign languages, with their visual and spatial components, highlight the limitations of current AI translation systems, which primarily deal with spoken languages.
One unexpected benefit of AI translation is its application in education. Language learning apps often leverage AI to offer quick and relevant translations, potentially making language acquisition faster. It's fascinating that while AI translation models like NLLB200 show some improvement in handling cultural references and idioms, they still frequently struggle with subtleties. This suggests that nuanced communication remains a significant hurdle in the field. It's a reminder that, as impressive as AI translation is, there's still much to learn about how to effectively convey the richness of human language.
AI Translation Breakthroughs Analyzing JWorg's 1,084-Language Milestone in 2024 - Zero-Shot Translation Increases Flexibility in Language Pairs
Zero-shot translation offers a new level of adaptability in AI translation, allowing models to handle language pairs without needing specific training data for those pairs. This is particularly useful when dealing with languages that have limited digital resources, where traditional training methods often struggle. Instead of requiring separate training for every language combination, a single AI model can be trained to tackle a wide range of pairs. This approach can streamline the translation process, even for languages with complex structures.
While promising, zero-shot translation still presents some challenges. Accuracy and cultural appropriateness can be difficult to maintain, especially for languages with very different grammatical structures or cultural contexts. These systems, however, are core to how ambitious projects like JW.org's 1,000+ language effort can be approached. The ability to translate between numerous languages without needing vast training datasets for each pair is a boon, but it also underscores the need for ongoing quality checks and potential human intervention in critical situations. The future of translation may very well rely on a combination of powerful AI systems and human expertise to ensure that translations are both accurate and culturally sensitive.
Zero-shot translation is a fascinating development in AI that allows translation models to handle language pairs they haven't been specifically trained on. This means the model can leverage its existing knowledge to translate between languages without needing a huge amount of pre-existing training data specifically for that language pair. It's like giving the model a foundation in language structures and then allowing it to figure out how to bridge the gaps between different languages. This approach could potentially increase the flexibility of translation systems, allowing them to quickly adapt to new languages and language pairs as needed.
One benefit of this approach is the potential to speed up translation efforts. For many languages, especially less common ones, finding enough data to train traditional AI translation models is difficult. With zero-shot translation, we could potentially have a faster way to get basic translation capabilities up and running. It would be especially beneficial in situations where getting access to large parallel datasets for specific language pairs is challenging or costly.
Zero-shot models are often built with massive numbers of parameters, meaning they can capture complex relationships within languages. This enhanced understanding may enable them to better handle the nuances and variations between languages. However, the sheer complexity of these models also means they're demanding on computational resources.
Another interesting aspect is how zero-shot translation can be used in conjunction with transfer learning. If a model has been trained on a related language, it can potentially use that knowledge to bootstrap translation capabilities for a new language. Languages that share grammatical structures or vocabulary could benefit from this transfer process, allowing the system to adapt to new languages more efficiently.
These models also appear to be more adaptable across different domains. It seems they can switch between translating, for instance, formal documents, medical texts, or everyday conversations with less need for re-training. This type of adaptability could be especially useful in situations like JW.org where consistent messaging across diverse content types and languages is important.
While promising, there are still challenges with zero-shot translation. These models sometimes struggle to capture cultural references and idioms as accurately as human translators, which can be a problem for contexts where cultural sensitivity is vital. It's still a field of active research and development, and refining these models to better handle these nuances is a crucial area.
Additionally, zero-shot translation offers the intriguing possibility of integrating with OCR. OCR helps convert images of text into digital formats, which can then be used as inputs to the AI translation model. This allows access to historical documents or texts from images, expanding the range of what can be translated.
From a cost-efficiency perspective, zero-shot translation could be a more viable option for groups that can't afford to invest in the expensive and laborious process of creating enormous training datasets. However, we need to continue investigating whether the translation quality of zero-shot methods is sufficient for all use cases.
While it’s still an active research area, the ability of zero-shot translation to learn and improve from user feedback or interactions could pave the way for even better translation outcomes in the future. Imagine a translation model that automatically adapts over time based on how users are interacting with it, constantly refining its abilities.
Zero-shot translation presents an exciting possibility for enhancing flexibility and speed in language translation. As AI systems mature, understanding how to best leverage this new approach while mitigating its limitations will be essential. The intersection of zero-shot translation and translation challenges faced by organizations like JW.org highlights the potential to expand translation capabilities to a wider range of language pairs and content. The future of translation might involve a careful blend of these AI approaches and continued human expertise.
AI Translation Breakthroughs Analyzing JWorg's 1,084-Language Milestone in 2024 - Cultural Nuances Integration Improves AI Translation Authenticity
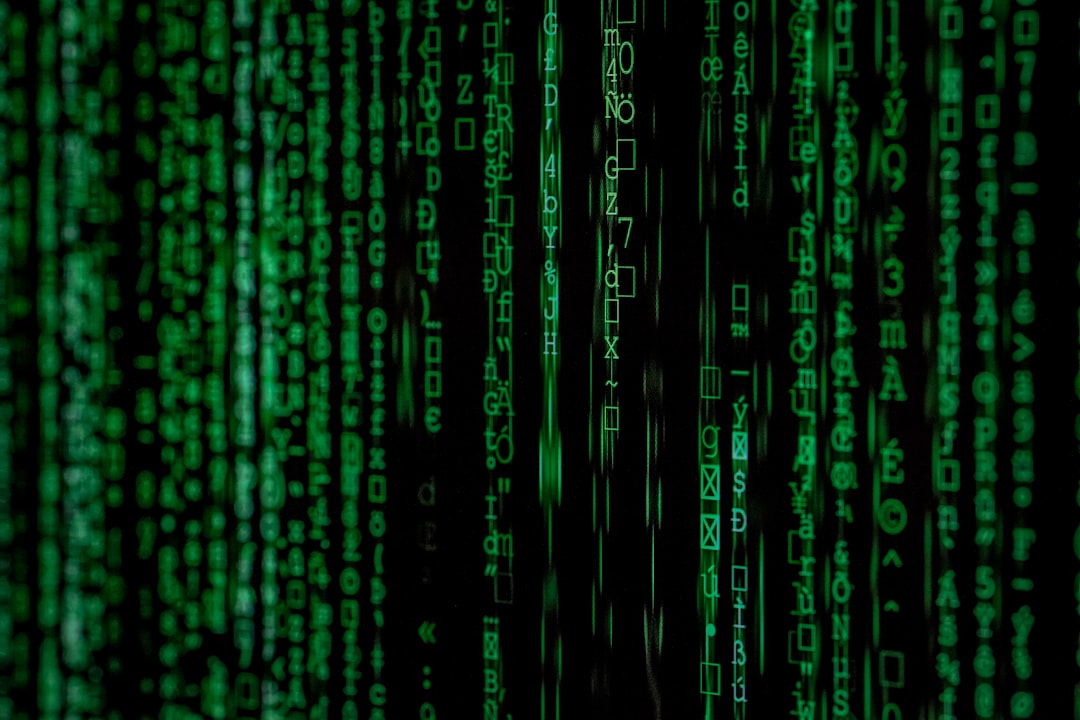
AI translation systems are increasingly incorporating cultural nuances, leading to more authentic and accurate translations. This shift away from basic word-for-word translations represents a significant improvement, as it acknowledges that language isn't just about individual words but also the context and culture surrounding them. The growing need for communication across cultures highlights the importance of understanding these nuances, helping to avoid misunderstandings and foster better connections.
However, AI still faces challenges, particularly when dealing with idioms and cultural expressions, or when translating languages with limited digital resources. These situations may require human intervention to ensure the translated content is truly faithful to the original message and culturally appropriate. Organizations like JW.org, striving to reach a global audience through translation, provide a good example of how important these cultural considerations have become. Their effort to adapt materials across a vast range of languages and cultures reflects a growing recognition of the need for translation to be not just fast and cheap, but also sensitive and accurate. Maintaining that balance is the next frontier for AI translation.
AI translation is making strides in capturing the essence of languages, not just through literal word swaps but also by incorporating cultural nuances. This is especially important in areas where misinterpretations due to cultural differences could be problematic. For instance, training AI on a diverse set of texts allows it to better understand and handle things like idiomatic expressions or cultural references that would otherwise lead to inaccurate translations.
We're seeing improvements in how AI maintains context throughout a piece of text. This ability to connect phrases and sentences is essential for conveying subtle meanings that might otherwise be lost in a straightforward word-for-word translation. This contextual awareness seems to contribute to the overall authenticity of the translated material.
However, challenges remain. Language structures vary widely, and simply translating between languages with drastically different grammatical structures can lead to clumsy phrasing. For example, languages that arrange words as subject-object-verb (SOV) compared to those that use subject-verb-object (SVO) may present unique obstacles to a translation model unless it's specifically designed to handle them.
One interesting approach to addressing some of these issues is "zero-shot" translation. It allows AI to translate between languages it hasn't been explicitly trained on. This is a huge benefit for languages with limited digital resources, where large training datasets may not be readily available.
The idea of "transfer learning" seems like a powerful tool, especially for lesser-known languages. AI models can learn from existing translations in related languages and apply those insights to improve the translation quality of similar, but less-documented, languages. It's like having a foundation of linguistic knowledge and then building on it for different language pairs.
OCR technology plays a supporting role, helping to make text available in a format AI can process. This is important when digitizing older documents and ensures that historically and culturally significant materials can be accessed and translated.
A crucial aspect is ensuring that the output of AI translation doesn't just maintain accuracy but also remains culturally relevant to the audience. This is vital for things like religious texts or sensitive subject matter, especially with the scale JW.org is working at.
The ability of AI to handle large volumes of text is crucial for projects like the JW.org initiative. With over 1,000 languages, AI can quickly translate large quantities of content, promoting speed and consistency across a vast range of language variations.
AI systems are also becoming more dynamic by incorporating feedback loops. As AI interacts with users, it can learn from their experiences and refine its translation abilities. This continuous learning means the models adapt to changing language trends and user preferences.
Despite all the advancements, it's important to recognize that human oversight is still necessary, especially when the translation involves subtle cultural cues or sensitive topics. AI is excellent at generating a foundational translation, but human expertise ensures that the final product captures the spirit and cultural sensitivity of the original message. It's a collaborative effort between AI speed and human nuance.
AI-Powered PDF Translation now with improved handling of scanned contents, handwriting, charts, diagrams, tables and drawings. Fast, Cheap, and Accurate! (Get started for free)
More Posts from aitranslations.io: