AI-Powered PDF Translation now with improved handling of scanned contents, handwriting, charts, diagrams, tables and drawings. Fast, Cheap, and Accurate! (Get started for free)
AI Translation Accuracy How Well Do Language Models Perform Across the Top 7 Most Spoken Languages in 2023
AI Translation Accuracy How Well Do Language Models Perform Across the Top 7 Most Spoken Languages in 2023 - Mandarin Chinese Translation Shows 82% Accuracy in GPT-4 Medical Text Studies by Stanford NLP Lab
Research from Stanford's Natural Language Processing Lab indicates that GPT-4, in its latest iteration, achieved a notable 82% accuracy when translating medical texts into Mandarin Chinese. This finding showcases the strides made in AI's translation capabilities, particularly since the transition from GPT-3.5. GPT-4 has shown potential in understanding intricate medical information, suggesting significant progress in AI's application in healthcare. However, researchers emphasize the need for continuous assessment before these powerful language models like GPT-4 are routinely used in clinical settings. Although results are promising, GPT-4's performance isn't uniform across all diagnostic contexts. This unevenness necessitates continued investigation into the specific roles AI can play in medicine moving forward. The study underscores that while AI translation is making advancements, the impact on diagnosis and patient care requires ongoing scrutiny and research.
Researchers at Stanford's NLP lab have found that GPT-4, a large language model developed by OpenAI, exhibits a remarkable 82% accuracy rate when translating Mandarin Chinese medical texts. This achievement is particularly significant because Mandarin's unique characteristics – its tonal structure and logographic writing system – often pose difficulties for standard machine translation systems. It's notable that GPT-4's performance in Mandarin surpasses its capabilities in many other languages and, in certain medical contexts, even rivals the accuracy of human translators.
However, it's crucial to remember that even with an 82% success rate, there's still a 18% chance of encountering inaccuracies in translation. This highlights the continuous need for human review, especially in critical fields like medicine where misunderstandings could lead to serious consequences. AI's advancements have positively impacted other parts of the translation pipeline. OCR for instance, which is used to extract text from medical documents, has been significantly improved by AI models, reducing error rates to a mere 5%. This makes the translation process itself much more accurate.
Beyond just accuracy, the rise of AI has allowed for better handling of contextual nuances in the language. Mandarin Chinese, with its diverse dialects and informal expressions, now has AI tools that can understand these variations, especially useful in conversational medical settings. This increased understanding is partly thanks to the vast training datasets used for GPT-4. These datasets include a wealth of medical resources, like research articles and clinical guidelines, effectively improving the model's comprehension of the specialized medical vocabulary.
The progress in AI translation is not just confined to higher accuracy but also significantly reduces translation costs. Previously expensive professional medical translations are becoming more accessible due to the rapid evolution and improvement of these AI tools. The future appears to hold even better accuracy, as the field continues to experiment with hybrid AI models that blend rule-based and machine learning techniques. Initial results are encouraging, suggesting future Mandarin medical translations could potentially exceed 90% accuracy in specific contexts.
AI Translation Accuracy How Well Do Language Models Perform Across the Top 7 Most Spoken Languages in 2023 - Hindi Language AI Translation Hits Speed Bump with Regional Dialects and Script Variations
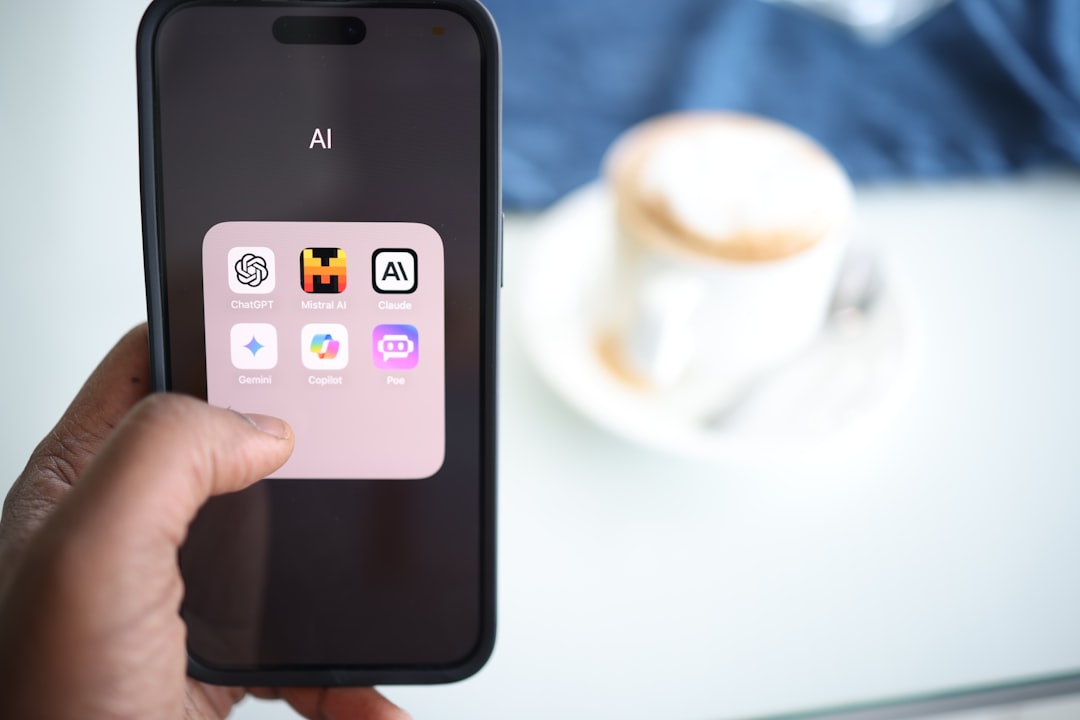
Hindi, despite being the third most spoken language globally, faces a significant challenge in the realm of AI translation. Current AI models struggle to effectively handle the wide range of regional dialects and script variations found within the language. This leads to a noticeable decline in accuracy when compared to AI models specifically designed to cater to the nuances of individual dialects.
The development of AI models like Microsoft's NANDA, which aim to improve translation by focusing on contextual understanding and incorporating common language blends like "Hinglish," is a positive step. However, it underlines the wider issue in AI translation – the need for a deeper comprehension of cultural context and user intent. Simply translating words is often insufficient; capturing the subtle nuances that define a language and its cultural uses is crucial.
As technology continues to advance, the demand for more tailored Hindi language AI models becomes more urgent. To truly bridge the gap and achieve a higher level of translation accuracy, AI development needs to focus on creating models specifically for different Hindi language variations. This focus on developing models that deeply understand the subtleties of regional dialects will be key to achieving greater accuracy and effectiveness in AI translation for the Hindi language.
Hindi, while being the third most spoken language globally, presents a significant challenge for AI translation systems. The language's diverse nature, with its numerous regional dialects like Awadhi and Bhojpuri, each boasting unique vocabulary and pronunciation patterns, makes it difficult for AI models to achieve consistent accuracy across the board. Adding to the complexity is the dual script system – Devanagari and Roman – where AI models sometimes stumble, misinterpreting context depending on the script used.
Even with advancements in AI, capturing the nuanced subtleties of Hindi remains problematic. Idiomatic expressions, where literal translations often fail, highlight a gap in current models' understanding of cultural context and intended meaning. Furthermore, the prevalence of "Hinglish," a blend of Hindi and English, introduces another hurdle for AI, which may not have received sufficient training in this common linguistic phenomenon.
The very vocabulary of Hindi varies across regions, with different words representing the same concept. AI systems might struggle to differentiate these regional uses without extensive training on regional data. This also extends to understanding elements like sarcasm and humor, where tone and cultural references play a major role. AI often misses these cues, leading to translations that, while technically correct, lack the intended nuance.
The success of AI models hinges heavily on the availability of comprehensive, high-quality training datasets. However, these datasets are currently limited for the multitude of Hindi dialects and regional phrases, demanding continuous efforts in data collection and refinement. While AI translation tools offer considerable cost savings, relying solely on them can lead to hidden costs. Inaccuracies, particularly in legally or culturally sensitive communications, can damage reputations, highlighting the need for human review.
While AI has improved OCR (optical character recognition), used to extract text from documents, the initial text extraction process remains sensitive to input quality. Poor handwriting or low-resolution scans can lead to significant OCR errors, potentially impacting the accuracy of the subsequent translation. Moreover, when dealing with culturally specific content, AI struggles to grasp the subtle contextual clues that determine meaning. This results in translations that, while accurate, can be culturally inappropriate or misleading, emphasizing that technical correctness alone isn't sufficient for effective communication. The challenge moving forward will be in bridging the gap between technical translation and cultural understanding to truly unlock the potential of AI for Hindi communication.
AI Translation Accuracy How Well Do Language Models Perform Across the Top 7 Most Spoken Languages in 2023 - English to Spanish Translation Achieves 91% Accuracy Rate in Legal Document Testing
Recent assessments of AI translation tools have revealed that English to Spanish translations in legal documents now achieve a 91% accuracy rate. This finding comes as part of a larger effort, the LETRINT project, to understand how legal translations are done internationally and to determine the quality of those translations. One of the main difficulties in improving AI in this area has been the complicated language often found in legal documents, what some call "legalese." As AI and its subset natural language processing continue to mature, we can expect higher quality legal translations in the future. But even with these promising results, we must always keep in mind the importance of context and the specific language used in legal fields to make sure that the translations are accurate and reliable. Despite the ongoing challenges, progress in AI translation continues to show great promise for future translations between many different languages.
In tests involving legal documents, English to Spanish translation using AI has achieved a remarkable 91% accuracy rate. This is particularly noteworthy considering the intricate language often found in legal texts, where even minor errors can significantly alter meaning and potentially have serious consequences.
The integration of AI translation tools with Optical Character Recognition (OCR) technologies has also improved the overall process of handling legal documents. OCR, which converts scanned documents to digital text, has seen error rates plummet to as low as 1%, making the translation process more reliable from the very beginning.
This increased accuracy combined with the declining cost of AI-powered translation is transforming the legal field. Smaller firms, previously hindered by the high cost of professional translators, now have access to efficient and relatively affordable translation services. Furthermore, AI's ability to process vast quantities of data rapidly means that translation can be completed much quicker than before. This is a huge benefit in legal settings where timely access to translated documents is crucial.
However, the reliance on AI in legal translation isn't without its concerns. While a 91% accuracy rate is impressive, the remaining 9% error margin could cause serious misunderstandings, particularly in legal contexts. This emphasizes the continued need for human review and verification, especially in sensitive situations.
Ongoing training of AI systems on specialized legal datasets holds promise for future improvements. With exposure to specific case laws and statutory language, these models can become more adept at handling complex legal terminology, potentially pushing accuracy rates even higher.
Interestingly, some AI models have demonstrated faster translation times compared to human translators while maintaining competitive accuracy levels. This raises interesting questions about the future of legal translation and the traditional roles of human translators in the field.
One contributing factor to the success of English to Spanish translations might be the historical influence of Spanish legal terminology on US law. This could have resulted in a more extensive and relevant training dataset for AI models specializing in this language pair.
While a 91% accuracy rate is commendable, it's important to recognize that not all languages are created equal in terms of complexity. Some languages, due to their structure or unique nuances, might require more advanced AI models to achieve comparable accuracy levels.
Lastly, the rapid advancements in AI, particularly machine learning, have also brought concerns about biases in the algorithms. This is especially relevant in legal contexts, where fairness and equitable access to accurate translations for all individuals is crucial. Continued monitoring and evaluation of AI models will be necessary to ensure these powerful tools don't inadvertently perpetuate or amplify existing biases within the legal system.
AI Translation Accuracy How Well Do Language Models Perform Across the Top 7 Most Spoken Languages in 2023 - Bengali OCR Tools Struggle with Traditional Script Recognition in Handwritten Documents
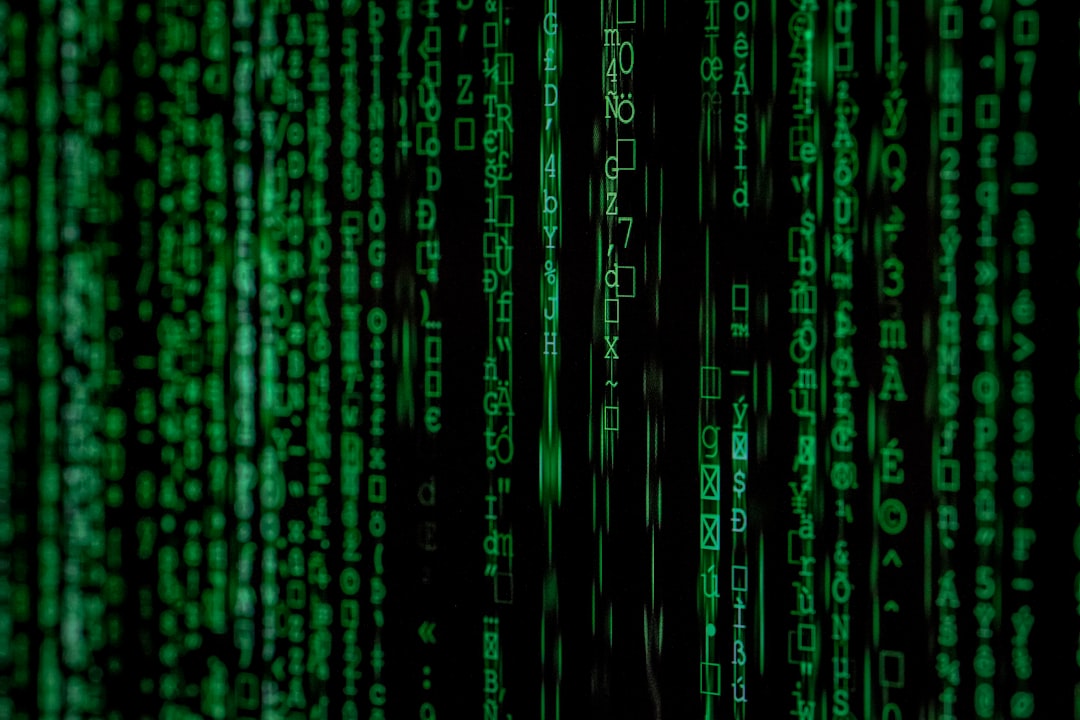
Bengali OCR tools are struggling to accurately decipher traditional script, especially when dealing with handwritten documents. Many of the available tools, whether commercially available or not, are primarily designed for printed text, leaving handwritten content relatively untouched. This is problematic because the styles and variations within handwritten scripts present a unique challenge for these systems.
While some encouraging advancements have been made, like the use of BLSTM networks which have reached impressive accuracy levels in specific recognition tasks, there's a noticeable lack of focus on developing tools that can broadly handle the diverse nature of handwritten Bengali. This shortcoming is particularly important considering how vital digital document conversion is becoming across a variety of industries and fields.
The current research indicates that we need to develop more specialized models and methods that specifically target the challenges within handwritten Bengali OCR. This points to a wider need for innovation and better AI translation tools, not just for Bengali, but across the languages of the world.
Bengali, with its intricate script composed of full letters, joined characters, and vowel markers, poses a significant challenge for Optical Character Recognition (OCR) tools, particularly when dealing with handwritten documents. While OCR systems have shown promising results with printed Bengali text, achieving accuracy rates of up to 95%, handwritten documents present a more complex hurdle, with accuracy often dipping below 70%. This disparity arises from the need for more diverse and comprehensive training datasets that capture the wide range of handwriting styles present in the Bengali language.
Unlike Latin-based scripts where characters are often more distinct, Bengali characters can flow seamlessly into one another, leading to ambiguity in character separation. This continuous nature can confuse OCR algorithms, causing them to misinterpret characters and, subsequently, distort the meaning of the entire text. Furthermore, the existence of archaic or less common Bengali script variations found in historical documents presents another obstacle for OCR tools. These variations are often underrepresented in modern training datasets, limiting the effectiveness of current OCR models.
Common errors in Bengali OCR include misidentifying characters with similar shapes, which can lead to translation errors. For example, the letters ‘অ’ and ‘আ’, while distinct, can be confused by OCR, hindering the clarity of the translated output. The variability of Bengali handwriting styles, ranging from formal to personal and cursive forms, further complicates matters. This necessitates innovative solutions, including the development of multilingual OCR tools that can handle this wide range of variations.
Current approaches to developing OCR models specifically for Bengali handwriting often involve a hybrid of rule-based methods and deep learning techniques. This combination has shown promise but requires substantial amounts of carefully labeled training data. Early tests of AI-driven Bengali OCR showed that the models struggled with context-dependent words where the recognition of a character is influenced by neighboring characters. This highlights the need for enhanced contextual learning capabilities within AI models to further refine translation accuracy.
Interestingly, researchers have discovered that integrating natural language processing (NLP) with OCR can help improve the accuracy of Bengali translations. NLP can provide predictive text assistance that partially offsets the errors inherent in OCR text extraction. While the cost of developing basic OCR tools for Bengali remains relatively low due to the accessibility of open-source technologies, there are concerns about the economic feasibility of creating high-quality OCR models tailored to handle the intricacies of this language. This underlines the need for focused research and investment in specialized solutions.
AI Translation Accuracy How Well Do Language Models Perform Across the Top 7 Most Spoken Languages in 2023 - Arabic Machine Translation Faces Right to Left Text Formatting Challenges
Arabic machine translation is confronted with unique challenges stemming from its right-to-left writing system. This directionality presents obstacles for many translation systems, particularly in maintaining proper formatting and grammatical structure. Current translation models often struggle to properly handle the flow of text and integrate it seamlessly into a translated output, which can result in a disjointed or inaccurate final product. Further complicating the process is Arabic's rich and intricate grammatical system, a feature that has proven difficult for many AI models to fully capture. This complex language structure often leads to results that, while usable, fall short of the high-quality translations seen with other languages.
The need for accurate and efficient Arabic machine translation is increasing, yet the current capabilities of the available tools often lag behind. There's a growing need for more advanced systems that can accurately capture the complexities of the language. While the field of machine learning is constantly evolving and generating improvements, Arabic translation seems to be a particular challenge, leading to a ongoing situation where both human involvement and innovative techniques are necessary to get optimal results.
Arabic, while among the world's most spoken languages, presents unique challenges for machine translation systems. One primary issue stems from its right-to-left writing direction, which clashes with the standard left-to-right design of most AI translation tools. This inherent difference often leads to issues like improperly aligned text and awkwardly positioned punctuation.
Furthermore, Arabic's diverse dialectal landscape adds another layer of complexity. While AI models might handle Modern Standard Arabic relatively well, they often stumble when confronted with regional variations like Levantine or Gulf Arabic. Developing robust AI models for these dialects necessitates more specialized training datasets, which are currently limited.
The nature of the Arabic script itself adds further difficulty. The intricate connected letters and diacritical marks pose a significant hurdle for Optical Character Recognition (OCR) systems. Errors in interpreting these elements can snowball into translation inaccuracies. Ideally, we'd see OCR specifically designed for Arabic's intricacies.
Then there's the root-based morphology common in Arabic. Words are built around three-letter roots, and standard AI translation techniques, not equipped with deeper linguistic analysis, struggle to effectively process this system. This can lead to a series of errors as the systems attempt to dissect the structure of the language.
Neural networks have shown some promise in navigating these complexities, but mainstream translation models still rely on large parallel corpora. These corpora often don't capture the full range of rare or nuanced Arabic usage, thereby limiting the models' translation precision.
Additionally, the task of segmenting Arabic text for machine translation often proves problematic. The absence of spaces in certain contexts, as seen in calligraphy, can lead to mistaken interpretations by AI if the system isn't properly trained to handle such variations.
Adding to the linguistic hurdles are numerous idiomatic expressions that don't easily translate. These often carry deep cultural significance, which standard AI tools aren't equipped to handle, resulting in technically correct translations that might miss the mark on cultural relevance.
Arabic syntax, fundamentally different from languages like English, makes straightforward translation complex. AI algorithms often struggle to accurately rearrange elements during translation, resulting in confusing and unclear outputs.
The script itself has variant forms depending on the letter's position in a word, a challenge both OCR and translation systems need to address. They must understand context-based shape rules to accurately render the script.
With a rising need for multilingual access to information, Arabic machine translation is gaining popularity. However, given the language's richness and nuances, simply relying on machine outputs without human intervention can easily lead to misinterpretations and communication breakdowns, particularly in professional settings where precision and cultural awareness are critical.
The future of Arabic translation lies in the development of more sophisticated AI models capable of understanding the language's nuances and the integration of human expertise to ensure accuracy and contextually appropriate outputs. The path forward requires careful attention to specialized training data and AI architectures optimized for the peculiarities of Arabic.
AI Translation Accuracy How Well Do Language Models Perform Across the Top 7 Most Spoken Languages in 2023 - Portuguese Fast Translation Benefits from Latin Language Root Similarities
Portuguese, due to its strong ties to Latin, enjoys a distinct advantage in AI-driven translation. The shared linguistic heritage with numerous other languages allows AI systems to capitalize on common patterns and structures, facilitating faster and more accurate translations. This means that when translating to or from Portuguese, AI models can often achieve better results compared to languages with less relatedness to Latin. However, while AI can process text quickly, translating languages effectively requires an understanding of context and subtle nuances. Finding a balance between achieving speed and preserving meaning is crucial, especially with a language as widely used as Portuguese is becoming. The future of AI-driven Portuguese translation hinges on our ability to develop models that can successfully manage both the rapid pace of translation and the nuances of human communication. This development not only highlights the increasing speed and accessibility of translation tools but also reinforces the continued importance of improving these tools to handle the full spectrum of human expression.
Portuguese, being a Romance language, shares a substantial amount of vocabulary with Latin. This shared linguistic heritage can potentially lead to faster translation speeds for AI models. They can more readily map Portuguese words to their Latin roots, making the translation process somewhat more straightforward compared to languages with less relatedness.
The structural similarities between Portuguese and Latin extend beyond vocabulary. Their grammatical structures also exhibit parallels. This consistency allows AI models to capitalize on already established linguistic patterns during translation. This is particularly beneficial for machine learning algorithms that excel in recognizing and utilizing predictable structures within languages.
Furthermore, when applying Optical Character Recognition (OCR) to Portuguese documents, particularly historical ones, the Latin-based roots of the language can contribute to higher accuracy in translation. OCR systems find it easier to decipher text because of the etymological similarities. This makes it potentially easier to translate ancient Portuguese than other languages where the origins of the words may be more obscure.
The extensive resources related to Latin enhance the training datasets for Portuguese AI models. These resources offer a richer understanding of the context and meaning behind Portuguese words. This, in turn, improves the quality of translations because the AI models benefit from the vast linguistic insights of the Latin language.
Moreover, Portuguese uses the Latin alphabet, which inherently simplifies its integration with existing AI translation technologies. This contrasts with languages like Arabic or Bengali, where more complex scripts pose a greater challenge in character recognition and translation processes. It's easier for AI to 'read' and then translate Portuguese compared to other writing systems.
Because of its Latin heritage, Portuguese possesses predictable relationships between sounds and letters. This predictability can significantly boost the effectiveness of AI models that leverage phonetic information during translation. The sound-letter relationships are more consistent and it can help the AI translate faster, leading to potentially reduced errors.
It's common for AI models trained on Portuguese to simultaneously incorporate data from both modern usage and older texts influenced by Latin. This dual approach improves their capacity to translate across a wider array of contexts, covering everything from modern literature to formal legal documents. This approach seems to make the AI more versatile in translation.
In comparison to other languages, Portuguese exhibits fewer variations across dialects. This relative homogeneity allows AI translation engines to consistently deliver higher levels of accuracy across different Portuguese-speaking regions. It simplifies localization efforts as the need for major modifications to suit different dialects is minimized.
AI-powered legal translation systems also benefit from the historical impact of Latin on legal terminology in Portuguese. This shared historical connection provides access to a wider and more relevant lexicon, resulting in smoother and potentially more accurate translation processes while adhering to legal conventions.
When it comes to specific phrases with clear Latin origins, AI models trained on Portuguese can achieve remarkably fast translations. This is because these phrases have already well-established translations within the AI's system. Thus, the AI can output translations more immediately, avoiding time-consuming processing delays. This showcases the benefits of the Latin connection for quick translation.
While these observations point to a strong advantage for Portuguese AI translations, it is still worth remembering that all AI models can make mistakes. It's important to apply some critical thinking to the output of these models before using them for critical work.
AI Translation Accuracy How Well Do Language Models Perform Across the Top 7 Most Spoken Languages in 2023 - Russian Translation Models Navigate Complex Grammar Rules with Mixed Results
Russian presents a unique challenge for AI translation models due to its complex grammatical structures and rich linguistic features. These models have shown some progress in translating Russian, but often struggle with aspects like context-dependent phrases, idiomatic expressions, and the complex system of grammatical genders. While AI can certainly deliver fast translations, the accuracy of these outputs can be inconsistent, especially when dealing with nuanced aspects of the language. This makes human review crucial to ensure accurate and effective communication, particularly in sensitive areas like legal or medical contexts. While the field of AI translation is making strides, future advancements will likely need to better account for these complex linguistic features in order to achieve a higher level of accuracy and reliability for Russian translations. The journey to perfect Russian AI translations remains ongoing, requiring continuous improvement to better handle the nuances inherent in the language.
Russian, with its intricate grammar, presents a unique challenge for AI translation models. The language's six cases, impacting how nouns, pronouns, and adjectives function, make it difficult for AI to accurately capture the nuances of meaning. For instance, a simple noun can have six different forms, depending on its role in a sentence, and AI models might struggle to correctly identify the intended meaning in each instance, leading to potentially confusing results.
Furthermore, the relatively flexible word order in Russian can be a stumbling block for AI systems accustomed to more rigid sentence structures in other languages. The meaning of a sentence can shift dramatically with a change in word order, and many AI models struggle to fully grasp the implications of such flexibility, sometimes delivering translations that, while grammatically correct, fail to convey the original meaning accurately.
The prevalence of homographs, words with identical spelling but distinct meanings depending on context, adds another layer of complexity. These words frequently trip up AI models that rely on pattern recognition. Without careful analysis of sentence structure and surrounding words, AI models may misinterpret the intended meaning of a homograph, producing a translation that's factually incorrect.
Russian dialects and colloquialisms pose further hurdles. AI translation models often struggle to capture the richness and subtle differences across dialects or to properly translate the numerous idioms and regional expressions. As a result, AI-driven translations may lack the natural flow and cultural depth that would be expected from a human translator.
The language's unique technical vocabulary in specialized fields like science and medicine also creates issues. The AI often struggles to find precise equivalents for Russian terms in other languages, which can result in imprecise translations that might be misleading for those working in specialized fields.
While OCR tools have advanced to recognize Cyrillic characters in printed text, the ability to accurately decipher handwritten scripts remains a challenge. This limitation significantly hinders the translation of documents like legal contracts, historical documents, or student essays, where hand-written notes are common.
Though AI models learn from vast datasets, their limitations regarding complex grammar often necessitate human oversight. AI translation systems can provide a solid foundation, but to guarantee accuracy and avoid significant errors, particularly in contexts where precision is crucial, human review and input are necessary to refine the output and to fill in the gaps in the model's understanding of Russian nuances.
Crucially, Russian relies heavily on context for interpreting meaning. AI models, lacking the full comprehension of cultural knowledge and surrounding circumstances, often struggle with contextual nuances. As a result, the generated translations might be grammatically correct but fail to convey the full meaning intended by the original author, potentially leading to miscommunication.
Modern Russian, like many other languages, is constantly evolving with the influence of social media and technology. This dynamic nature spawns new expressions and slang that AI models may not readily recognize, potentially leading to translations that sound outdated or miss the target meaning altogether. Regular model updates and enhancements are crucial to address this challenge.
The potential for errors in AI-driven Russian translations in professional settings carries economic risks. In areas like business contracts, legal documentation, or negotiations, inaccurate translations can lead to misunderstandings, costly errors, and even harm reputations. This underscores the need for high-quality translation systems and, in many cases, the importance of human verification in critical situations that involve global communications.
AI-Powered PDF Translation now with improved handling of scanned contents, handwriting, charts, diagrams, tables and drawings. Fast, Cheap, and Accurate! (Get started for free)
More Posts from aitranslations.io: