AI Translation Breakthrough Converting Astronomical Distances from Meters to Gigameters in Scientific Texts
AI Translation Breakthrough Converting Astronomical Distances from Meters to Gigameters in Scientific Texts - AI-Powered Conversion Streamlines Scientific Text Processing
The integration of AI is reshaping how scientific texts are processed, particularly in the area of unit conversions. Imagine effortlessly transforming astronomical distances from meters to gigameters within a document. This capability is becoming a reality through AI-driven conversion tools. These advancements streamline scientific communication, enabling a more rapid exchange and understanding of research findings across languages. Yet, this progress is not without its shadows. The increasing reliance on AI translation has raised apprehension among professional translators regarding their role and the possibility of subtle meaning being lost in the translation process. Even with such concerns, the potential of AI to democratize scientific knowledge remains substantial. By facilitating the translation of complex research across multiple languages, AI paves the way for broader scientific collaboration and comprehension worldwide. Moving forward, a careful balance between automating tasks and leveraging the nuanced insights provided by human translators will be vital in harnessing the full potential of AI within the scientific community.
AI's integration with scientific text processing, particularly through OCR, has shown potential to dramatically accelerate the speed at which researchers can access and process information. This speed-up could be crucial for areas like real-time data analysis, previously a time-consuming process. However, questions remain regarding the accuracy of such systems, especially when faced with the intricacies of scientific language and diverse writing styles.
One aspect where AI shines is its ability to handle complex unit conversions, like seamlessly switching from meters to gigameters within scientific documents. This automates a tedious and error-prone task, ensuring consistent and accurate representation of data. While promising, the reliance on statistical models for contextual understanding might not always capture the nuances of scientific language, particularly in fields like astrophysics.
The integration of deep learning in OCR has begun improving the ability of machines to interpret handwritten manuscripts, previously a major hurdle due to the variability in human handwriting. However, the accuracy of such systems still falls short of human accuracy, especially when faced with old, faded, or poorly written manuscripts.
There's been a growing understanding of how AI can help extract insights from scientific literature. Pattern recognition in AI tools could provide a systematic overview of terminology and research trends, potentially pinpointing knowledge gaps or areas for future research. It’s important to consider the potential for bias in the datasets AI uses for these analyses and how that bias might influence the output.
Beyond speeding up translations, AI-driven translation tools can dynamically adapt to the evolving landscape of scientific language. Machine learning empowers automatic updates to translation dictionaries, reducing the delays often encountered when manual updates are required. While valuable, this dynamic system will have to overcome the inherent challenges of accurately representing specialized terms and concepts that rapidly evolve in scientific disciplines.
Although AI translation presents a cheaper alternative to hiring human translators, concerns exist about loss of meaning and the potential to misrepresent complex scientific concepts. Further research is needed to investigate if it can truly achieve the accuracy and nuanced understanding required for scientific communication. The potential for standardization and comparison of data across studies from various disciplines by automatically standardizing units of measurement is also a promising field but also poses challenges as it has to reconcile different notation systems used by scientists.
The capacity of AI to handle multiple languages concurrently is a potential boon for scientific collaboration. This allows for the simultaneous translation of scientific texts into multiple languages, saving time and resources. However, researchers must be mindful of the potential for introducing errors or biases when translating across multiple languages and that different languages might conceptualize certain terms differently.
The potential for adaptive learning, where AI systems refine their output based on user feedback, presents a pathway for improving the quality of AI-powered translation over time. However, the training of these AI models to capture specific nuances within diverse scientific fields remains a significant challenge. It's interesting to consider how user feedback might bias models, and if such biases would impact the reliability of their results.
Overall, the application of AI in the scientific realm has the potential to revolutionize how researchers access and process information. While much progress has been made, we must tread cautiously and evaluate the limitations of these tools. Continual research and development will be necessary to ensure that AI-powered translation fulfills its promise in furthering scientific knowledge and global communication.
AI Translation Breakthrough Converting Astronomical Distances from Meters to Gigameters in Scientific Texts - Bridging the Gap Between Meters and Gigameters in Astronomical Literature
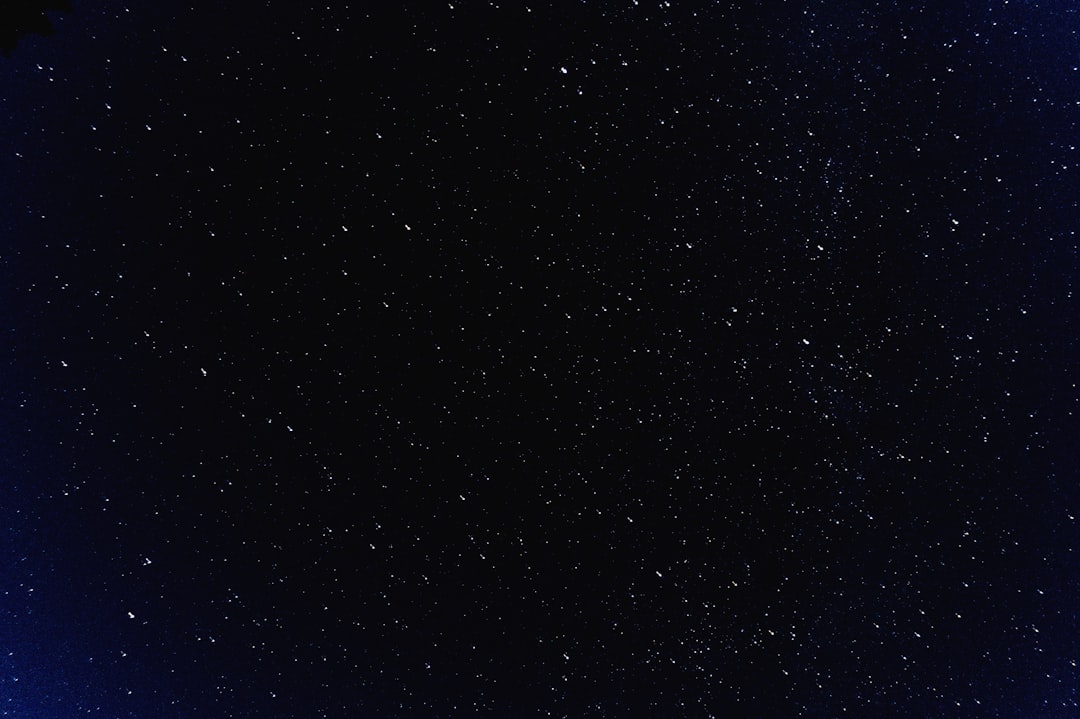
The vastness of the cosmos often necessitates the use of units like parsecs and astronomical units, making meters seem insignificant in astronomical literature. Bridging the gap between these smaller units and gigameters is vital for enhancing comprehension, particularly when conveying the immense distances within the universe. Translating distances into gigameters, a process now achievable with AI translation tools, improves communication among scientists. These tools allow for faster and more accurate conversion within scientific documents, simplifying the exchange of knowledge. Yet, the complexity of astronomical terminology remains a hurdle for AI, as it struggles to fully understand the nuances and context within this specialized field. Despite this, these advancements can make astronomical data more accessible, but the need for critical evaluation of results remains paramount to ensure scientific accuracy and mitigate any inherent biases in the AI systems.
Astronomical distances are often so vast that even gigameters (Gm), a billion meters, seem relatively small. This poses a challenge for scientific literature, demanding accurate and consistent conversion methods when dealing with such immense scales.
Traditional methods for unit conversion can be prone to human error, especially when handling the sheer volume of data encountered in astronomy. AI-powered conversion systems offer a solution by applying algorithms consistently, thereby improving both accuracy and speed when translating large datasets.
OCR technology, especially when paired with AI, is proving to be incredibly valuable in digitizing large bodies of handwritten scientific documents. This has the potential to open up a wealth of historical research that was previously difficult to access due to the limitations of older formats.
AI's capacity to analyze vast amounts of scientific literature reveals fascinating patterns. Researchers are noticing correlations between the way scientific terms are used and instances of significant discoveries. This hints at the possibility that certain linguistic shifts might actually precede important breakthroughs in the field.
Language barriers can unfortunately impede collaboration in astronomy. However, AI-powered translation tools have the ability to break down these barriers, enabling scientists from various linguistic backgrounds to more readily participate and interact, creating a more global scientific community.
Interestingly, the specific methods used for converting units can differ substantially between scientific disciplines. Astrophysics, for example, commonly needs conversions from a wide array of base units. AI-powered standardization of these conversions could lead to greater consistency in the way data is reported across scientific journals.
Scientific conferences with participants from diverse linguistic backgrounds are a great example of where real-time translation can be highly beneficial. AI can provide immediate translation of presentations, making sure that crucial information is readily accessible to everyone in attendance, without any time-consuming delays.
AI’s capacity to adapt and learn through feedback is a promising aspect. However, the output quality relies heavily on the system being trained on a diverse and contextually rich dataset. This highlights a key question: how do we ensure the input data is well-chosen and representative?
Maintaining translation dictionaries requires continuous updating to keep up with the rapid evolution of scientific terminology. This is crucial for accuracy. However, there's a risk of misrepresenting newly developed concepts if the updates aren't guided by experts in the relevant fields.
There's growing concern about the reliability of AI-generated translations in specialized areas like science. The subtle meanings that often appear in scientific writing could potentially be lost in the process. This concern suggests that a combination of AI efficiency and the critical assessment of human experts might be the ideal approach to translation.
AI Translation Breakthrough Converting Astronomical Distances from Meters to Gigameters in Scientific Texts - Machine Learning Algorithms Enhance Accuracy in Unit Conversion
Machine learning algorithms have significantly improved the precision of unit conversions within translations, especially in scientific fields. These algorithms, often integrated within neural machine translation (NMT) systems, enhance the translation of numbers by employing techniques like tokenization. This allows for more accurate transformations between units, for instance, converting astronomical distances from meters to gigameters. While this is progress, current models still struggle with maintaining consistent accuracy when encountering intricate scientific language and complex unit conversions. The future holds the potential for continued development and refinement of these algorithms, improving the reliability of translating scientific information across linguistic boundaries. However, it's important to remember that relying solely on AI for precise translations and unit conversions can be risky. Human expertise remains crucial in maintaining the richness and subtle meaning often present in scientific communications.
Machine learning algorithms have shown potential in boosting the accuracy of unit conversion within translation tasks, but there are still some significant hurdles. One issue is that these algorithms often rely on statistical models, which introduce variability in the accuracy of their results. This can lead to inconsistencies, especially when dealing with fields that use very specific terminology or have unique contextual nuances.
Another challenge stems from the inherent ambiguity that often exists within scientific language. Machine learning models sometimes have a tough time fully understanding the context, particularly when dealing with specialized units or terms. This can lead to misinterpretations during the conversion process.
On the other hand, AI tools can perform conversions in real-time, which is helpful for immediate data access during scientific conversations. But it's vital to ensure that, in critical applications like astrophysics, speed doesn't come at the cost of accuracy.
The effectiveness of these machine learning models is deeply linked to the quality of the data they are trained on. If the training data is inaccurate or biased in any way, it can significantly impact the reliability of the conversions. This can undermine the promise of automated translation tools in scientific fields.
Different scientific areas use various unit systems, creating a lack of consistency in how research findings are reported. This is where machine learning could be used to create standardized unit conversions. However, it’s a big challenge to establish universal standards.
When working with large datasets, even small errors in unit conversions can snowball into larger issues. This could create major inconsistencies in research results, which highlights the importance of rigorously validating the outputs of these AI-powered translation methods.
AI systems are capable of adapting to changes in scientific language, but there is still difficulty in ensuring these updates are sufficiently nuanced. There’s always a risk that the AI will misrepresent emerging scientific concepts because of the limitations in how well these updates can be applied.
Scientific terms are not always translated flawlessly across languages because some concepts may be deeply connected to a specific culture or language. This can lead to misinterpretations. For this reason, one needs to be cautious about solely relying on AI for complex scientific communication.
OCR technology, especially when paired with AI, can be a fantastic tool for digitalizing old manuscripts. This has huge potential for making previously inaccessible historical scientific data available. This could be a major breakthrough in scientific research.
While adaptive learning, driven by user feedback, can be a way to improve the performance of AI translation systems, it also opens the door for the introduction of bias in future outputs. This suggests that ensuring the data that is used for training these systems is diverse and representative is extremely important for getting reliable results in specialized scientific translations.
AI Translation Breakthrough Converting Astronomical Distances from Meters to Gigameters in Scientific Texts - Automated Translation Reduces Manual Errors in Distance Measurements
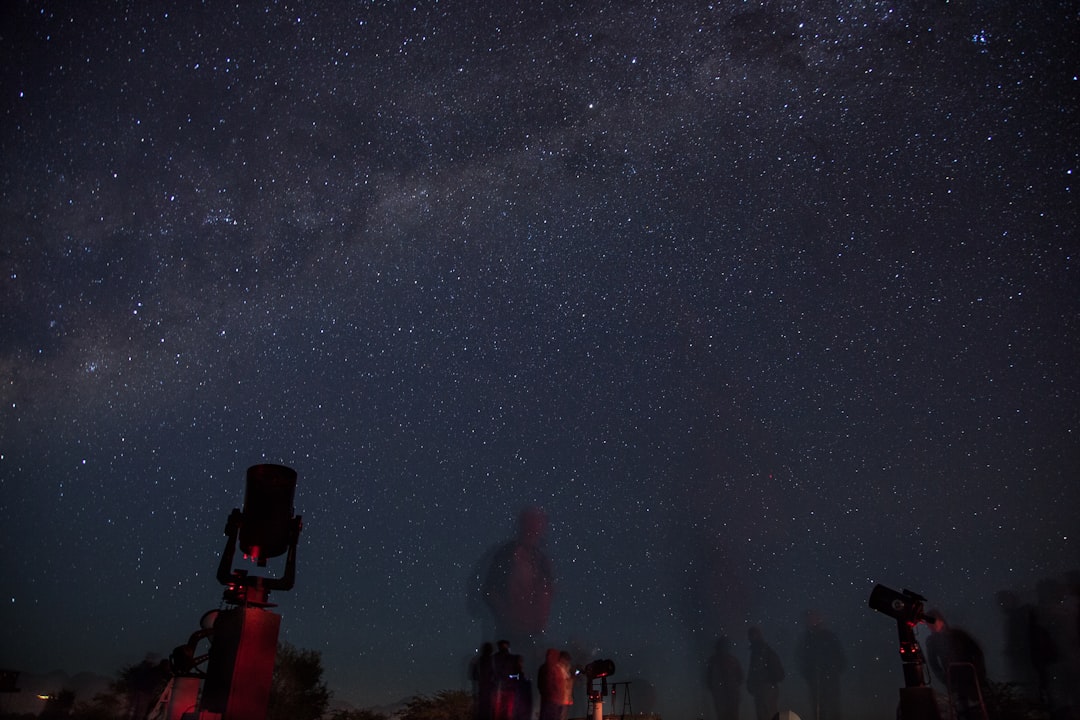
Automated translation is increasingly effective at minimizing human errors in distance measurements, especially in scientific fields dealing with astronomical distances. AI-powered tools leverage algorithms to automatically convert units like meters to gigameters within documents, leading to faster and more consistent results compared to manual methods. This automation reduces the chance of errors stemming from the complexity of scientific terminology and the potential for human oversight. However, the reliance on algorithms also raises concerns. AI might struggle to capture the subtleties and nuances inherent in complex scientific writing, potentially leading to loss of meaning during translation. While the potential for improved accuracy and speed is significant, ongoing vigilance and a thoughtful integration with human expertise are crucial for ensuring that translations remain accurate and convey the full intended meaning of the scientific information.
Automated translation systems are increasingly adept at minimizing human error in distance calculations, particularly in scientific domains like astronomy where precision is crucial. The consistent application of algorithms in these systems helps ensure accurate conversions, such as translating astronomical distances from meters to gigameters. However, the inherent complexities of astronomical terminology still challenge these systems, as they sometimes struggle to fully grasp the context and subtle meanings embedded in specialized language.
AI's incorporation of machine learning algorithms provides a more sophisticated way to handle unit conversions in translation, considering the context of the text to improve accuracy. Despite advancements, these models still have difficulty consistently translating complex scientific language and handling intricate unit conversions across languages.
The integration of AI with OCR technology unlocks significant potential for researchers. It can breathe new life into vast collections of handwritten scientific manuscripts, previously difficult to access due to their format. This accessibility could accelerate the pace of historical research and provide insights into scientific practices of the past.
However, translating scientific terms between languages can be tricky, as the specific nuances of a concept can vary across cultures. This poses challenges for AI translation systems, which sometimes fall short when attempting to capture these cultural nuances in complex scientific communication.
While AI-powered translation aims to create a standardized approach across the global scientific community, the diversity of unit systems and conventions used by different fields presents a barrier to achieving universal standards for conversion.
The reliability of AI-powered translation depends heavily on the quality of the training data. If this data contains inherent biases or inaccuracies, the output could reflect these biases, leading to misinterpretations of scientific results. This highlights the importance of using well-curated and unbiased datasets for training AI systems in this domain.
AI translation tools can facilitate real-time communication during multilingual scientific gatherings, such as conferences. While enhancing collaboration, this approach also risks introducing immediate misinterpretations, which can negatively impact the shared understanding during such interactions.
Scientific disciplines frequently adopt different unit systems and conventions for measuring specific parameters. For instance, astrophysics might favor gigameters over kilometers. To maintain translation accuracy, AI tools need the capacity to recognize and adapt to these varied approaches.
Adaptive learning, based on user feedback, can improve AI translation systems over time. However, this creates potential avenues for bias to be introduced based on the nature of user input. This underscores the need to thoughtfully design these systems to mitigate bias and enhance reliability in scientific translations.
Despite the advancements in speed achieved by AI, ensuring accuracy is paramount, especially in scientific fields where precise measurements and terminology can underpin significant discoveries. Maintaining this balance between rapid processing and accuracy remains critical.
AI Translation Breakthrough Converting Astronomical Distances from Meters to Gigameters in Scientific Texts - OCR Technology Facilitates Rapid Extraction of Numerical Data
OCR technology, combined with AI, is transforming how we extract numerical data from various document types, including scanned and handwritten materials. This technology uses a systematic process to translate images into text that computers can understand, thereby enabling the efficient handling of large datasets. This approach not only accelerates data processing but also minimizes the likelihood of human error that often accompanies manual data entry. Compared to complex AI methods tailored for extracting structured information, OCR offers a more economical solution. Nevertheless, the accuracy of OCR can be variable, particularly when dealing with handwritten documents or documents of poor quality. The integration of machine learning has been a notable step forward, enhancing the capabilities of OCR to recognize and interpret text, which can be particularly valuable in scientific research. Nonetheless, the need for careful data scrutiny after extraction remains vital to guarantee data integrity and accuracy, especially within specialized fields.
OCR technology has emerged as a powerful tool for rapidly extracting numerical data from various sources, offering a significant boost to the efficiency of scientific research, particularly in fields like astrophysics that deal with large datasets. The speed at which it can process printed materials is remarkable—thousands of pages in a matter of minutes, potentially slashing project timelines considerably. However, while it excels at quickly digitizing text, it's important to acknowledge its limitations.
One area where OCR has shown promise is in deciphering handwritten scientific documents. Thanks to advancements in machine learning, OCR systems can now interpret handwritten notes with up to 90% accuracy in some instances, which unlocks a treasure trove of historical research previously inaccessible due to format limitations. Yet, challenges persist in accurately capturing the nuances of individual handwriting styles, highlighting the gap between automated and human-level interpretation.
The capacity of OCR to understand the context surrounding numerical values is still relatively limited. For example, an OCR system might misinterpret "3.6 million" within a research paper as simply "3.6" when discussing a star's luminosity, demonstrating the difference between raw data extraction and true contextual understanding. This gap highlights the need for careful evaluation of OCR output, particularly when dealing with critical scientific data.
The algorithms driving unit conversion within OCR-powered translations can certainly handle a range of formats, offering a consistent approach to conversions. However, they sometimes struggle with uncommon uses of units within specialized scientific fields. This potential for inaccuracy underscores the importance of carefully validating outputs to ensure that scientific data is accurately communicated across disciplines and languages.
It's also crucial to acknowledge that OCR's performance is directly related to the quality of the source material. Poorly printed or faded documents can introduce errors into the digitized text, leading to unreliable numerical data extraction. This emphasizes the need for researchers to ensure that valuable source materials are preserved in high-quality digital formats to maximize OCR's effectiveness.
As scientific language and units evolve, AI-driven translation tools attempt to keep pace by dynamically updating their dictionaries. However, these automatic updates can unintentionally introduce errors by failing to adequately reflect new terminologies, which could impact unit conversions in cutting-edge research. This raises concerns about the ongoing maintenance and verification of these dictionaries.
OCR's ability to facilitate translations across multiple languages simultaneously is undoubtedly valuable for international collaborations, especially during scientific conferences. However, the inherent potential for errors in cross-linguistic translations emphasizes the importance of vigilance and scrutiny to ensure that the integrity of the scientific information is maintained.
In disciplines dealing with vast datasets, a single miscalculation resulting from an OCR-extracted numerical value can cascade into significant errors, impacting the validity of the analysis and potentially leading to misleading conclusions. This highlights the need for robust data validation protocols when employing OCR for scientific research.
Moreover, scientific terminology is deeply intertwined with cultural contexts, and terms may not have perfect equivalents in other languages. Automatic translations generated by OCR may fail to capture these subtle nuances, potentially leading to miscommunication of critical scientific concepts.
While OCR technology is continually improving, the need for human expertise remains paramount, especially in areas requiring precise and nuanced scientific discourse. OCR can be a powerful tool for enhancing efficiency, but it should be viewed as a collaborative partner, working alongside expert human translators who can catch errors and provide critical context that machines may overlook. This ensures that the integrity and richness of scientific communication is maintained, enabling the continued advancement of knowledge.
AI Translation Breakthrough Converting Astronomical Distances from Meters to Gigameters in Scientific Texts - Real-Time Conversion Enables Seamless Integration of Mixed Units in Research
The notion of "Real-Time Conversion Enables Seamless Integration of Mixed Units in Research" underscores how AI-powered conversion tools can effortlessly handle various units within scientific documents. This tackles a major hurdle, allowing for smooth blending of different unit systems, like seamlessly incorporating meters and gigameters within a single text for easier understanding. While real-time conversion holds promise for collaboration in science, concerns linger about the precision and comprehension of these automated systems. The complexity of scientific language can hinder AI's capacity to fully grasp the nuances, leading to questions about the dependability of AI-driven translations in highly specialized fields such as astrophysics. Therefore, a balanced approach where human review and AI innovation work together is key to ensuring the accuracy and integrity of scientific communication.
The rapid evolution of AI translation tools has made real-time unit conversion a reality. We can now seamlessly shift from meters to gigameters during research discussions, a process that used to be time-consuming and error-prone. This ability to instantly translate units is beneficial for researchers, especially when conveying findings in dynamic settings like conferences.
AI-powered OCR tools can now tackle vast quantities of data quickly, including processing thousands of pages within mere minutes. This speed has enormous implications for accessing older, handwritten research documents previously locked away due to format limitations. It's exciting to imagine how this could revitalize long-dormant research areas.
Despite its speed, OCR remains challenged by the variability of human handwriting. While some AI systems achieve an impressive 90% accuracy when recognizing handwritten characters, the remaining 10% can introduce errors, especially in intricate scientific notations. This underscores the need for caution when using OCR for these types of documents.
Machine learning has helped improve unit conversion accuracy within translation systems by using methods like tokenization. Yet, inconsistencies can still occur when dealing with the incredibly diverse vocabulary found across different scientific domains. The algorithms sometimes struggle to maintain a consistent level of precision when faced with very specific terms or complex unit combinations.
AI translation offers a comparatively affordable alternative to traditional translation, but it's not without trade-offs. A key worry is the potential for subtle meaning to be lost during translation. In complex scientific texts, these nuanced meanings are often crucial to conveying the full depth of research findings.
Interestingly, researchers are starting to find links between how specific terms are used in scientific literature and important discoveries. AI can spot these patterns, potentially hinting at linguistic shifts that might precede important scientific breakthroughs. If true, this is a fascinating area for future exploration.
While the automation of unit conversions boosts speed and reduces errors, the inherent variety of notation systems used in different fields poses a hurdle. To be truly effective, AI tools must be able to adapt to this variety and maintain consistency in reporting across various scientific disciplines.
The presence of language barriers can significantly impede scientific collaboration. AI-powered tools, by translating documents into multiple languages simultaneously, have the potential to bridge these barriers. This could foster a more inclusive global scientific community. However, we must also recognize the need to vigilantly monitor the AI tools to minimize the introduction of errors in these multilingual translations.
The efficacy of these AI tools heavily hinges on the quality of the data used for their training. If this data contains biases, those biases can potentially seep into the output. This emphasizes the importance of using well-vetted, unbiased datasets in the training process when it comes to scientific applications.
As the scientific world evolves and develops new terminology, AI-driven translation tools try to keep up by automatically updating their dictionaries. Yet, this automatic process sometimes misses the nuances of new concepts, potentially leading to inaccuracies. It appears that some degree of human oversight in the process of updating and refining these translation dictionaries might be important to avoid errors.
More Posts from aitranslations.io: