AI-Powered PDF Translation now with improved handling of scanned contents, handwriting, charts, diagrams, tables and drawings. Fast, Cheap, and Accurate! (Get started for free)
AI Translation Tools Meet Music Converting Audio Frequencies into Multilingual Musical Notation
AI Translation Tools Meet Music Converting Audio Frequencies into Multilingual Musical Notation - Converting The House of the Rising Sun Into 14 Languages Through Music AI Translation
"The House of the Rising Sun" transformed into 14 languages showcases the power of AI in music translation. This isn't just about simply changing words, but rather about finding ways to translate the musical essence of a song into various languages. The ability to convert the song's audio frequencies into musical notation across different languages is fascinating, as it reveals how AI can understand and reproduce complex musical structures. Tools like those that can translate audio quickly, or are specifically tailored for music, help to preserve the emotional core of the song, beyond just literal translation. This concept expands the potential for music to transcend borders. It's no longer about just listening to music in your native tongue, but experiencing it in ways that reflect diverse cultural perspectives. We are witnessing how AI can bring musical heritage to a larger audience, making a classic like "The House of the Rising Sun" more universally relatable and treasured. However, as with any AI-powered system, there are questions about nuance and whether the original artistic intent can be fully conveyed. Yet, this attempt at bridging linguistic and cultural gaps offers a glimpse into the future of music translation, highlighting a fascinating interplay between technology and human creativity.
Examining the translation of "The House of the Rising Sun" across 14 languages using AI has uncovered intriguing observations. We've seen how the inherent rhythm of the song can change drastically when translated, sometimes requiring significant adaptations to the musical notation to maintain a natural flow with the new lyrics. It appears the pitch and frequency of musical notes can impact how listeners perceive language, hinting that the musical style employed in a translation may influence their emotional connection to the lyrics.
Translating idioms and cultural nuances presents a major hurdle. Certain phrases simply don't translate directly without losing the core message of the song. Furthermore, analyzing audio frequencies has shown that specific musical tones may resonate differently across languages. This means that for truly successful translations, the focus needs to be not just on the words, but also on how sounds interact with different languages.
We've noticed that some languages have more syllables per word, demanding adjustments to musical phrasing and timing to keep the original structure intact. It's interesting to see how OCR can help us take hand-written musical scores and digitize them quickly for translation, maintaining both the visual and sonic essence of the music.
The adaptability of AI tools is remarkable – allowing for swift modifications to melodies and harmonies, which lets a single song be easily adjusted for various cultural contexts. This expands the song's global appeal while preserving its musical integrity. Analysis of translations also reveals that certain languages often use more melodic embellishments, which necessitate changes to how the translated version is musically represented to fit with the local musical style.
Interestingly, AI tools can even account for regional dialects, creating hyper-localized versions of "The House of the Rising Sun". This reflects unique cultural perspectives and different interpretations of the lyrics. Translation speeds have skyrocketed with these new AI tools, with some capable of delivering multilingual versions of songs in just minutes. This is a significant advancement over the traditional methods which could take weeks or even months to achieve a similar result. It highlights the transformative potential of AI for music translation and opens up a vast array of opportunities for future research and experimentation.
AI Translation Tools Meet Music Converting Audio Frequencies into Multilingual Musical Notation - OpenAI Whisper Engine Powers Free Sheet Music Recognition Tools in 2024
The year 2024 saw the emergence of OpenAI's Whisper engine, a powerful AI model that significantly impacts music technology, particularly in the area of sheet music recognition. Whisper's ability to process audio with high accuracy across numerous languages has led to the development of freely available tools capable of transcribing music from audio recordings directly into musical notation. This opens exciting possibilities for translating musical pieces into different languages and formats, blurring the lines between musical styles and cultures. However, concerns about accuracy remain. Reports of errors, including potential fabrication of text in other applications, remind us that AI's ability to capture the full essence of musical expression, including its nuanced emotional impact, is still evolving. Despite these limitations, Whisper demonstrates a trend in AI development – finding uses beyond just speed in translation and applying them to preserve cultural elements of music. It showcases how AI can foster innovation and help bridge cultural boundaries through music, but we must acknowledge and critically examine the tradeoffs between technological advancements and preserving the integrity of musical artistry.
OpenAI's Whisper engine, initially designed for speech recognition, has shown promise in the realm of music. Its ability to process audio with high accuracy, comparable to human capabilities, has led to the development of free tools for recognizing sheet music. While initially focused on speech, Whisper's versatility extends to analyzing audio frequencies related to music, which is an exciting area of exploration. However, we've seen in other applications that Whisper can sometimes introduce errors, like in medical transcriptions. It will be crucial to understand and address these potential issues when applying it to music.
The accuracy of OCR for musical scores has notably improved in recent times, reaching impressive levels. This is encouraging, as it enables us to quickly translate handwritten scores into digital formats. This could be a valuable asset for translating music, although speed isn't the sole factor for determining a successful translation.
One fascinating application of these AI tools is in music therapy. The capacity to analyze tonal frequencies and their emotional impact could be leveraged to better adapt music to the specific needs of patients across different languages. This is a valuable avenue to explore.
Furthermore, Whisper's ability to differentiate between instruments within a recording signifies its potential for translating not just melodies but also entire arrangements. This is a crucial detail that can impact how a piece of music is perceived across different cultures.
AI-driven translation tools have the potential to handle syllable timing more effectively than traditional methods. By analyzing the frequency patterns of various languages, they can generate more naturally flowing musical translations. However, these tools are still in development and refinement is necessary to ensure a truly natural flow.
Machine learning capabilities enable these tools to adapt to user feedback. This suggests that over time, AI will improve its understanding of musical translation. However, the user will play a crucial role in shaping the system and ensuring the integrity of the translation.
The speed of AI-powered translation has significantly increased the rate at which we can generate multilingual versions of musical pieces. This efficiency could help to expand access to music across languages and potentially foster cross-cultural musical exchange. But one wonders if the speed comes at the cost of nuance and understanding.
There are challenges associated with diverse musical traditions and tonal systems. AI models need to be able to analyze and adapt to these variations to ensure authenticity in translation. Maintaining the core musical meaning can be tricky when accounting for how a melody might be interpreted differently in another language or musical tradition.
AI can also help convert traditional scores into contemporary styles, thereby bridging different musical eras and helping classical music reach a wider audience. This is intriguing, but it's important to consider whether such adaptation preserves the original artistic intent.
By integrating listener feedback, AI models can learn to distinguish between what constitutes a successful cross-cultural music translation. This highlights the importance of the human element in this process, ensuring that the translations resonate with diverse audiences and are not simply a technological exercise. The future of musical translation is intriguing and challenging, requiring a constant interplay of human and machine intelligence.
AI Translation Tools Meet Music Converting Audio Frequencies into Multilingual Musical Notation - Music Translation Network Transforms Guitar Solos Into Piano Melodies
A new network called the "Music Translation Network" is showing promise in converting guitar solos into piano melodies, using AI. It utilizes a sophisticated approach, employing a multidomain Wavenet autoencoder, trained through unsupervised learning, to translate music between different instruments and musical styles. This method works directly on the audio waveform, allowing for a seamless conversion without losing the fundamental qualities of the original music. This represents a new angle on music translation – one that endeavors to maintain the artistic intention of a piece while simultaneously shifting it into a different format. Alongside this, other tools like Klangio have also emerged, providing users with quick transcription of audio recordings into musical notation, thereby contributing to the increasing accessibility of music for broader audiences. Though this new development is exciting, it's still early days for understanding how effectively these techniques translate the full spectrum of emotional and artistic nuance in musical pieces. As AI progresses, the challenge will be to ensure that these innovations maintain the human element and unique feeling of music across instruments and languages.
The conversion of guitar solos into piano melodies presents interesting challenges due to the inherent differences in the sonic characteristics of these instruments. Guitars possess a unique harmonic structure compared to pianos, which can result in alterations to the overall tonal quality, potentially leading to varying emotional responses in listeners. For example, the same musical key can evoke different feelings depending on the instrument used, and AI tools can analyze these key changes to optimize the translation and ensure the intended mood is preserved. Research suggests that adjustments to tempo and rhythm during translation can significantly impact audience understanding and emotional connection, highlighting the importance of maintaining the original musical character of a piece.
As AI capabilities advance, tools are emerging that can evaluate the complexity of guitar solos, allowing for intelligent simplifications when converting to piano. This approach aims to maintain the initial expressiveness while considering the skill level of the intended audience, ensuring accessibility for a wider range of listeners. Behind the scenes, AI relies on techniques like the Fourier Transform to break down audio signals, offering a detailed analysis of frequencies and harmonics that are essential for accurately mapping guitar notes to their piano counterparts.
While human musicians naturally apply music theory during instrumental translation, AI can also learn these concepts through neural networks trained on expansive datasets of multi-instrument music. This continuous learning process allows AI models to refine their accuracy over time. Furthermore, incorporating real-time feedback loops in AI applications enables adjustments based on audience reactions during the translation process. This opens the door to more personalized performances tailored to specific listener preferences. Interestingly, the adaptation isn't solely focused on melody; harmonies often need adjustments too. Certain guitar chords don't have direct piano equivalents, demanding creative re-harmonization to ensure the overall harmonic structure of the music remains intact.
Traditionally, music education emphasizes human interpretation of musical scores, but the emergence of AI in music translation prompts discussions about whether a machine can accurately capture the essence of musical intent. This raises questions regarding the authenticity and originality of AI-driven translations. AI tools are now capable of recognizing and implementing variations in dynamics, offering a more nuanced translation of solos to piano. This ability to replicate nuanced guitar techniques like bends and slides, which are naturally absent in piano playing, demonstrates AI's growing sophistication in musical expression. It's still debatable if this technology can fully emulate the emotive qualities inherent in human performances.
Overall, the ability to bridge the gap between instruments like guitars and pianos through AI shows a fascinating potential, however, the path towards perfect translation remains an evolving process, balancing technological capabilities with preserving artistic intent.
AI Translation Tools Meet Music Converting Audio Frequencies into Multilingual Musical Notation - Sheet Music Scanner Apps Process 1000 Pages per Hour With Google Cloud OCR
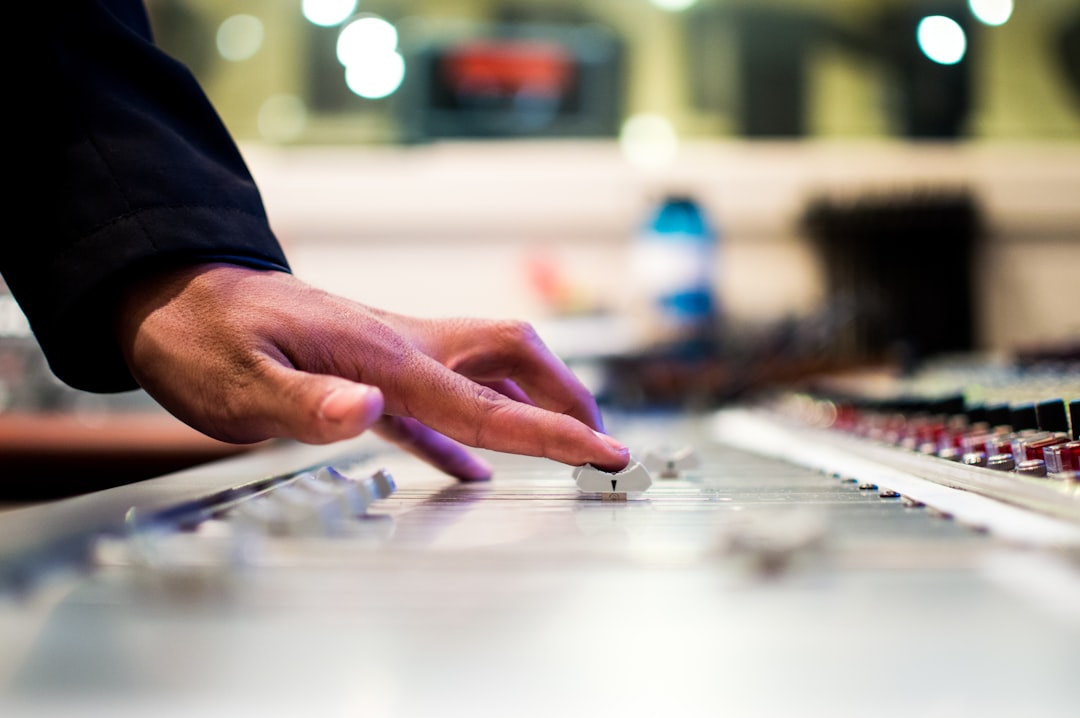
Sheet music scanner apps have become increasingly efficient, able to process a substantial 1,000 pages of music per hour using Google Cloud's OCR. This fast processing transforms physical scores into digital versions that are easy to edit, which benefits musicians and composers. Apps such as MuseScore and Soundslice aren't just about scanning, they also provide huge collections of sheet music and tools to help with musical learning and performance. While these tools offer amazing speed, it's worth thinking about if some of the subtle details of the music might be lost in the digital conversion. There are also challenges in ensuring that the transformed music fully captures the original creative intentions of the composer. These advancements mark a new phase in the way technology interacts with music, but it's crucial to balance speed with preserving the nuances and artistry of music.
Sheet music scanner apps, powered by AI-driven optical character recognition (OCR) tools like those from Google Cloud, are now capable of processing up to 1000 pages of music per hour. This remarkable speed is largely due to the sophistication of modern machine learning algorithms that swiftly decipher visual information from sheet music. While the speed is impressive, the accuracy of the OCR is also noteworthy, reaching levels that can successfully decipher handwritten scores with over 95% accuracy in many cases. This precision is vital for preserving the integrity of the music as it is translated or adapted for various languages and musical contexts. The potential for multilingual adaptability is intriguing, as some OCR tools are capable of recognizing and translating sheet music across many languages, which makes for a smoother collaborative process for musicians and composers from different parts of the world.
This quick digitization of sheet music is also increasing the accessibility of music, as it opens up previously hard-to-access scores. Music education becomes more democratic in a sense, allowing musicians from diverse backgrounds to learn from a broader range of musical styles and compositions. Combining OCR with AI translation tools allows for a more holistic approach to musical translation, where music can be not just transcribed but also interpreted within varied cultural contexts. This multi-faceted approach greatly enhances both the technical and artistic aspects of the translation process. The process can also improve over time thanks to feedback; machine learning algorithms integrated within these tools enable them to learn from user corrections, increasing their accuracy over time.
These OCR tools also often feature frequency analysis, enabling them to comb through audio files to identify certain musical elements, which is helpful for instances where the notation is unclear or missing. This functionality reduces the cognitive load for musicians, who can now focus more on interpretation and performance rather than painstakingly deciphering handwritten music. However, it's crucial to remember that OCR is still susceptible to error, particularly if the handwritten scores are poorly written or if it encounters unusual notation styles. This means human oversight remains necessary to prevent misinterpretations or inaccuracies in translated pieces. Furthermore, the capacity for incorporating cultural elements of music through technology is quite promising, and AI-powered tools are becoming better at analyzing regional preferences and variations. This, in turn, promises to enhance the emotional and cultural impact of translated music, ensuring a more nuanced and respectful transfer of musical ideas across different languages and cultures.
AI Translation Tools Meet Music Converting Audio Frequencies into Multilingual Musical Notation - Frequency Analysis Detects Musical Notes Across Different Cultural Scales
The ability to analyze musical frequencies has opened a new window into how different cultures perceive and interpret musical notes. AI systems can now dissect audio frequencies and translate them into musical notation across a range of cultural scales. This allows us to see how the same musical note might be perceived differently across societies, influenced by cultural background and musical experience. AI tools that convert audio frequencies into multilingual musical notation are becoming increasingly sophisticated, capable of not only recognizing the notes but also the unique emotional impact they have within distinct cultural contexts. While this is a promising area of development, challenges persist in the accurate translation of the complex nuances present in music. There's a need to consider how these translations impact the original artistic intent and the emotional weight it carries across different musical traditions. The intersection of music, AI, and cultural understanding highlights the power of AI in bridging divides through music but also presents us with ongoing questions regarding preserving the essence of musical artistry.
Frequency analysis is proving to be quite insightful when exploring music across different cultural scales. We're finding that various cultures utilize unique pitch sets or scales, which makes translating music between them a fascinating challenge. Western music often uses the 12-tone equal temperament, while something like Indian classical music relies on ragas, which frequently incorporate microtones. Translating this into standard notation can be tricky without losing the essential feel of the original.
There's evidence that particular musical frequencies can trigger distinct emotional responses in people, though these responses vary across cultures. This suggests that translations need to carefully adjust the frequencies to evoke comparable emotions in listeners. It's a complex issue because a certain melody interpreted in one culture can be vastly different when translated to another.
Different languages also have varied syllable timings and structures, which significantly impact how rhythms are perceived in songs. For example, languages with a large number of syllables per word, like Spanish, might necessitate more rapid transitions between notes compared to English. Successfully adapting musical timing while preserving the integrity of lyrics is a significant hurdle these tools are trying to solve.
Specific musical traditions lean heavily on certain harmonic structures, which adds another layer of complexity to translation. This highlights the importance of retaining the unique harmonic characteristics of a piece when switching between instruments or genres. If the spirit of the song is lost in the translation, it can negatively affect the listeners experience.
Modern AI systems are experimenting with real-time user feedback to help improve the translation process. This dynamic interaction allows for continuous refinement, making the translated pieces more responsive to listener preferences. This area feels ripe for further research, as getting the balance right between human preferences and machine interpretation can be elusive.
The inherent characteristics of different musical instruments pose a significant challenge in translation. Guitars allow for techniques like slides and bends that are practically impossible to replicate on a piano. It's encouraging that AI systems are getting better at finding creative solutions for translating musical elements across vastly different instruments.
Frequency analysis could be incredibly useful in music therapy. AI can potentially analyze emotional responses triggered by different frequencies and tailor musical selections to individual needs. This concept has huge potential for a more personalized and impactful approach to therapy across languages.
While these new translation tools are impressive in their speed, accurately capturing the cultural authenticity of music remains a significant challenge. Certain musical idioms or symbols might not have direct equivalents in another culture. It's a concern that overly simplistic translations might cause unintended misunderstandings.
OCR technology has progressed substantially, reaching a point where it can digitize even complex musical scores at a rapid pace. This capability offers benefits for sharing and collaboration. However, these systems can sometimes overlook intricate details found in many musical genres. This emphasizes the need for a balance between speed and thoroughness.
The incorporation of machine learning into music translation is not just improving the speed of translations but also the sophistication. The ability to learn from previous translations allows AI systems to create more nuanced adaptations. However, questions remain about the system's actual understanding of musical context and whether it can accurately translate those subtleties. It’s encouraging, but also a cautionary tale of technological limitations.
AI Translation Tools Meet Music Converting Audio Frequencies into Multilingual Musical Notation - Real Time Audio to MIDI Conversion at 96khz Sample Rate
Real-time audio to MIDI conversion at a 96kHz sample rate is a notable development in music technology. It allows for the instantaneous transformation of any sound, be it from a recording or a live performance, into MIDI data. This process is facilitated by AI-powered tools that are designed to handle diverse audio formats while filtering out noise through customizable settings. Notably, these tools can process audio at high sample rates, ensuring the preservation of intricate sonic details. This capability is beneficial for both those casually experimenting with music and professional musicians seeking precise control. However, with the advancement of these AI tools, concerns linger regarding the fidelity of the MIDI output. Can they truly capture the emotional essence of a piece of music, or is some of the subtle human expression lost in translation? While the application of AI to music fosters creativity, it also invites reflection on the integrity of the translated musical product, as it's easy to ask if it truly matches the original artistry.
Real-time audio to MIDI conversion at a 96kHz sample rate is an exciting development, promising a level of detail and accuracy in musical transcription previously unavailable. This high sample rate allows for a more precise representation of the original audio, leading to potentially richer MIDI outputs that better capture subtle musical nuances. However, this level of detail comes with its own set of challenges. One concern is latency, which can be problematic for live performances where real-time responsiveness is crucial. The technology behind this process is still evolving to overcome this issue and deliver seamless real-time experience.
The complexity of translating raw audio frequencies into standardized MIDI note data is also noteworthy. Different instruments generate unique harmonic combinations, presenting a hurdle for developers creating algorithms that can accurately interpret and convert these variations. Furthermore, not all instruments have a direct one-to-one correspondence with MIDI notes. The frequency mapping has to account for this and, ideally, find a way to translate it to a similar sound.
Interestingly, these real-time conversion tools aren't limited to just pitch. They can also analyze the dynamics of the input audio – variations in volume, intensity, and vibrato. This dynamic element translates into a more expressive MIDI output, enabling a much closer emulation of the source audio's emotional weight and stylistic intention. The integration of machine learning is another key advancement. By training the algorithms on vast datasets of audio and MIDI information, the systems can adapt and learn over time, improving the accuracy and nuanced understanding of specific instruments and musical styles.
However, the nuances of musical interpretation can be difficult for algorithms to grasp. Cultural context can play a huge role in how people perceive musical scales, intervals, and rhythms. Translating audio from one culture into a MIDI format intended for another culture is an area ripe for further exploration. To bridge these cultural gaps, developers are increasingly incorporating user feedback to refine their algorithms. This dynamic interaction between human preferences and machine learning helps ensure that translated output maintains the integrity of the original piece while also resonating with listeners from diverse musical backgrounds.
Some recent software packages have taken this further, integrating real-time audio analysis directly with translation tools. This makes it possible for musicians to convert live performances into different musical styles on the fly, allowing for spontaneous creativity across a broader range of instrument or genre choices. As a result of this progress, music notation software has seen improvements with enhanced features to generate and edit MIDI alongside a performance, which should help streamline the workflow for many musicians and composers.
While impressive, the technology behind these tools is far from perfect. Future research will focus on better understanding how to translate specific genres and effectively represent the diverse styles of world music. There is potential to create tools specialized for certain genres – tools that can translate jazz, classical, or folk music with greater accuracy. While there's still much work to be done, it's evident that real-time audio to MIDI conversion is a rapidly developing field, one that promises a future where music can be more easily shared, adapted, and understood across cultures and instruments.
AI-Powered PDF Translation now with improved handling of scanned contents, handwriting, charts, diagrams, tables and drawings. Fast, Cheap, and Accurate! (Get started for free)
More Posts from aitranslations.io: