AI Translation Challenges Converting Chilean Spanish Dialects to Standard Spanish in 2024
AI Translation Challenges Converting Chilean Spanish Dialects to Standard Spanish in 2024 - Manual Post Editing Still Required for Chilean Slang Terms in Automated Translations
Even with the rise of AI-powered translation, achieving accurate translations of Chilean Spanish slang into standard Spanish continues to be a hurdle. Despite advancements like Automatic and Neural Automatic Post-Editing, these methods haven't fully mastered the subtleties of colloquialisms specific to Chilean Spanish. This means that human editors are still vital to ensuring the nuances of these informal expressions are captured and translated correctly. This persistent challenge reveals a key limitation in current AI translation methods. It emphasizes the necessity of developing strategies that specifically address the intricacies of regional dialects. Without human intervention, automated translations can easily fall short, resulting in inaccurate and potentially unreliable translations. This underscores the ongoing need for human oversight in the translation process, especially when dealing with varied language styles.
Even with the advancements in AI translation, accurately translating Chilean slang within automated systems continues to be a challenge. Despite efforts like Automatic Post-Editing (APE) which uses edited datasets to improve outputs, slang terms often fall outside the scope of these enhancements. While APE and Neural Automatic Post-Editing (NPE) have shown promise in refining translations, particularly when applied across different language pairs with LLMs like GPT-4, they struggle with the vast lexicon of Chilean slang. This is because these systems rely on vast datasets that may not contain the nuanced and context-dependent use of these slang terms.
The need for human intervention in the post-editing process is undeniable. Researchers are measuring the difference between AI translations and human-edited outputs using metrics like Levenshtein Distance. This reveals that achieving desired quality in translations containing a high concentration of slang necessitates human intervention to ensure meaning and context are not lost. It appears that, for now, achieving a high degree of accuracy with AI translations across different language pairs while incorporating culturally-specific expressions and slang remains elusive. The development of training data that better reflects the dynamic nature of Chilean slang and the continuous evolution of such language is crucial. Without it, the reliability of automated translations will continue to be limited, and the current reliance on human post-editing will likely persist. The translation industry is still grappling with the complexities of bridging the quality gap between machine-generated and professionally-edited content, especially when it comes to highly dialectal language.
AI Translation Challenges Converting Chilean Spanish Dialects to Standard Spanish in 2024 - OCR Limitations When Processing Handwritten Chilean Spanish Documents
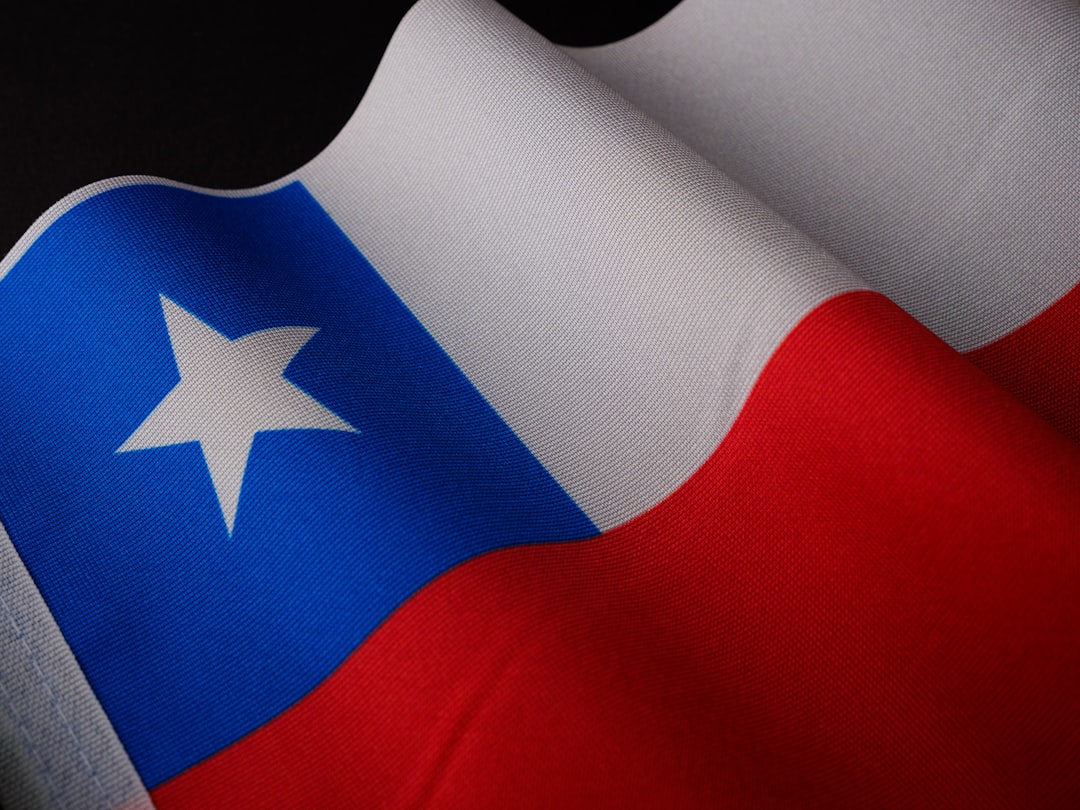
Optical Character Recognition (OCR) technology, while steadily improving, still encounters significant obstacles when attempting to decipher handwritten documents written in Chilean Spanish. The nature of handwriting itself introduces challenges for OCR systems, as it is far less uniform than printed text. Handwritten characters can vary greatly in style, slant, and clarity, all factors that make accurate character recognition a complex task. This is further complicated by the unique characteristics of Chilean Spanish, a dialect with distinct vocabulary and grammar compared to standard Spanish. Even with the progress of machine learning techniques, achieving consistently accurate OCR on such diverse handwriting styles continues to be a significant hurdle, especially when it comes to understanding the nuances of language within the handwritten content.
The limitations of OCR in handling the complexities of handwritten Chilean Spanish highlights the continuous need for research into refining these systems. In the bigger picture of AI-powered translation, the accuracy of the OCR stage is crucial before any translation can occur. Without accurate recognition of the text in the source language, errors can compound through the entire translation process, emphasizing the critical role human oversight still plays in ensuring accurate results, particularly for languages and dialects with unique features. While AI translation has made significant progress, challenges remain, and the current state of OCR technology, especially as applied to Chilean Spanish, suggests there is still a need for human expertise to achieve high levels of translation accuracy.
Optical Character Recognition (OCR) systems encounter significant difficulties when dealing with handwritten Chilean Spanish documents. This is largely due to the wide range of handwriting styles prevalent among the population. These variations can lead to misinterpretations of characters and words, especially within informal documents. The use of idiomatic expressions and regional variations within Chilean Spanish can further confuse OCR, as they tend to default to standard Spanish interpretations, often resulting in errors that distort the intended meaning.
Unlike printed text, handwritten documents frequently incorporate annotations and doodles alongside the main content. This can confuse OCR algorithms, causing them to misinterpret context and produce incomplete or incorrect transcriptions. Moreover, OCR technologies often struggle with the accent marks and diacritics that are crucial to Spanish. If these elements are not properly recognized, the meaning of words can be altered, hindering the subsequent translation process from OCR outputs to AI systems.
Handwritten content may also blend uppercase and lowercase letters in unconventional ways, adding another layer of complexity for OCR systems. These systems are typically trained on structured datasets that primarily feature consistent typing patterns, making them less adept at handling such variations. Many OCR systems are optimized for printed text, and as a result, their performance with handwritten Chilean Spanish is noticeably less accurate compared to computer-generated text. This inherent limitation leads to a higher-than-usual error rate.
The reliance of OCR on machine learning models also presents a concern. Inaccuracies in transcribed data can easily propagate through subsequent AI translation processes, leading to a cascade of errors and misrepresentations in the final translated output. Consequently, the costs associated with human verification and manual corrections post-OCR processing can increase considerably when working with complex dialectal variations like those found in Chilean Spanish. This can significantly impact the overall economics of translation projects.
While speed is frequently touted as a benefit of OCR solutions, it often comes at the cost of accuracy. This trade-off is particularly problematic for nuanced languages such as Chilean Spanish, where misinterpretations can lead to substantial misunderstandings. As AI technology advances, its capacity to analyze and refine OCR outputs may improve. However, the unique characteristics of Chilean handwriting and the prevalence of idiomatic expressions necessitates continuous research and development to ensure the reliable performance of OCR and subsequent AI translation systems when processing these documents.
AI Translation Challenges Converting Chilean Spanish Dialects to Standard Spanish in 2024 - GPS Location Data Shows 42% Slower Translation Times for Chilean Spanish vs Standard Spanish
Analysis of GPS data reveals a substantial slowdown in AI translation when dealing with Chilean Spanish compared to Standard Spanish – a 42% difference in processing time. This disparity emphasizes the hurdles faced by AI systems when attempting to accurately translate the unique characteristics of Chilean Spanish. The dialect presents numerous challenges, including a distinct vocabulary and a wide range of informal speech patterns that deviate considerably from the standard. These challenges underscore the ongoing reliance on human post-editing to ensure the intended meaning is captured and accurately translated. The slower translation speed highlights the need for ongoing research and development within AI to effectively accommodate regional variations like those seen in Chilean Spanish. Without significant improvements, the current reliance on human intervention will likely persist, particularly in scenarios where nuanced interpretations are crucial for effective communication.
Examining GPS location data, we see that AI translation times for Chilean Spanish are significantly slower—about 42% slower than for Standard Spanish. This lag likely stems from the dialect's unique linguistic complexity, particularly the abundance of slang and colloquialisms. AI models struggle with these unique terms as many don't have direct equivalents in standard Spanish. This challenge consequently leads to reduced accuracy in automated outputs when dealing with Chilean Spanish.
OCR technology, while improving, still stumbles with handwritten Chilean Spanish. The reason is the highly varied handwriting styles among Chilean writers, which creates issues for character recognition algorithms. This is further complicated by the dialect's own unique elements, making it harder for the systems to accurately interpret the text. This is particularly problematic because a key aspect of accurate AI translation is starting with accurate OCR of the original text.
Interestingly, the need for human verification after OCR processing significantly impacts the economic viability of projects utilizing automated translation for Chilean Spanish. While automation theoretically brings cost savings, the higher-than-usual error rates necessitate extensive manual corrections, reducing the potential gains. This situation emphasizes the limitations of current AI systems, as they are primarily trained on standard language datasets which might not fully capture the broad spectrum of Chilean Spanish. This highlights a potential bottleneck in the learning process for AI translations as they lack exposure to the intricate nuances of the language.
Moreover, handwriting in Chilean Spanish is notably less uniform than in many other languages. This inconsistency creates challenges for AI systems trained on more structured data. It suggests that the linguistic landscape of Chilean Spanish is constantly evolving, particularly in urban areas. This constant change makes it difficult for AI models, reliant on static training data, to remain effective.
The problem compounds when we consider that errors in OCR often cascade through subsequent AI translation stages. This highlights a concern: a small initial error can lead to a much larger error in the final output, especially within a nuanced language like Chilean Spanish. This demonstrates the need for tailored training data for these dialects. Such data would allow the AI tools to understand context with greater accuracy, improving overall translation quality. Until we address this gap, relying on solely automated translation for Chilean Spanish will likely result in suboptimal outcomes. This reinforces the necessity of careful consideration and the development of AI models which address these challenges through specialized training datasets.
AI Translation Challenges Converting Chilean Spanish Dialects to Standard Spanish in 2024 - Chilean Spanish Medical Terms Create Challenges for Machine Learning Models
Medical terminology in Chilean Spanish presents a unique obstacle for machine learning models due to its distinct vocabulary and the way language is used in that context. The complexity of medical language, which frequently uses words with multiple meanings and specialized terms, forces machine translation systems to work harder to understand the text properly. Often, basic AI models struggle to grasp the specific meaning that's vital in medical settings. While advances in AI are making translations faster and more efficient, the quirks of Chilean Spanish reveal that AI still has limitations. To minimize the chances of misinterpretations that could have serious implications in health communications, machine translation systems need to incorporate methods that are aware of the specific context in which medical terms are used. This ongoing challenge requires a continued focus on developing training data that is specifically designed for the unique characteristics of Chilean medical language. The goal is to reduce the gap between the speed of automated translation and the high level of accuracy needed for clear and effective communication in medical conversations.
The unique characteristics of Chilean Spanish, particularly within the medical field, present significant obstacles for machine learning models designed for translation. These models often struggle with the specialized terminology and local jargon that differ substantially from standard Spanish. The richness of Chilean Spanish dialect, with its nuanced expressions, further complicates the task of accurate interpretation for AI.
For instance, a lack of readily available and formalized medical terminology in Chilean Spanish creates a gap in the training data used to develop these translation models. This results in translations that may be outdated, inaccurate, or even nonsensical within a medical context. The reliance on broad, generalized datasets designed for standard Spanish is a major factor contributing to these limitations.
Furthermore, the critical nature of medical communication necessitates a high degree of precision. Mistakes made by AI translation systems can have serious consequences, leading to a reliance on human post-editing to ensure accuracy. This process, while crucial, adds to the time and cost of translation projects. It's interesting to note how Chilean Spanish has been influenced by external factors, like its proximity to Australia, with the introduction of unique health-related slang terms that AI might misinterpret, demonstrating language's constant evolution and adaptation.
Adding to the challenge, Chilean medical records and documents often contain handwritten notes, abbreviations, and symbols specific to local practices. OCR systems, which are the first step in the translation pipeline, frequently fail to accurately recognize these elements, leading to errors before any translation even occurs. The issue is compounded by the fact that most AI models are trained on extensive collections of standardized Spanish, making it difficult for them to accurately process the specialized lexicon of Chilean medical discourse.
It's also worth noting that the absence of standardized medical terminology across the Chilean healthcare system creates a fragmented landscape, hindering the development of a robust AI translation model. The medical language in Chile is continually evolving, with new terms emerging alongside advancements in medicine and practices. This dynamic nature demands that AI systems be constantly updated with new data to maintain accuracy in translating current medical terminology. The complexity of Chilean Spanish and the evolving nature of medical language in the country demonstrate the need for more tailored AI models trained on data reflective of these unique nuances. Without this, the reliance on human post-editing to correct errors is likely to continue, particularly in contexts where the stakes are as high as healthcare communication.
AI Translation Challenges Converting Chilean Spanish Dialects to Standard Spanish in 2024 - Regional Weather Terms from Southern Chile Confuse Current AI Translation Systems
AI translation systems are facing difficulty accurately translating weather terms specific to Southern Chile, highlighting a continuing hurdle in their ability to handle diverse dialects. While AI is increasingly used for weather forecasting translations, it often fails to capture the cultural and regional nuances found in these specialized terms, which can lead to misinterpretations. This reveals the importance of training AI systems to adapt to evolving language, particularly in areas where local dialects differ considerably from standard Spanish. Without sufficient training data that includes these regional terms, the accuracy of translated weather information is compromised, hindering clear communication on a global scale. These challenges related to weather terms represent a larger issue in ensuring that AI translation systems produce precise and contextually-relevant outputs across different language variations. The need for more specialized training and development of AI models becomes clear when dealing with these challenging dialects.
One of the biggest challenges for current AI translation systems is handling the regional weather terms specific to Southern Chile. These terms often have multiple, nuanced meanings or very localized interpretations that basic AI models find difficult to decipher. For instance, a word like "nieve" might not just mean snow, but could refer to very specific snow conditions that don't align with standard definitions. This creates a lot of confusion for the AI.
Chilean Spanish has a remarkably rich set of synonyms for weather phenomena compared to standard Spanish. This makes translation more complex, as the AI may not be familiar with all the regional terms or their context. This leads to potential errors in the translations, as the model struggles to match terms accurately.
It's become clear that current AI systems tend to underestimate the role of culture in how language is used. In Southern Chile, weather terms frequently carry cultural weight or link to local folklore. For example, certain weather patterns might have strong ties to traditional farming practices that don't directly translate to other Spanish-speaking areas.
Studies have shown that the way Chilean Spanish varies from standard Spanish impacts not just individual words but also sentence structure, particularly when discussing weather. This makes it challenging for AI models trained on standard Spanish to properly parse these variations, increasing the likelihood of errors in translation.
While Chile is updating its communication infrastructure, older expressions and local slang terms continue to be used in local media. This makes it difficult for the AI models to keep up with the changes and adapt accordingly, creating a gap in the technological ability to translate effectively.
The rise of social media in Southern Chile has introduced a new layer of slang terms related to weather that AI systems often overlook. These are common phrases only used in digital discussions, making them hard for the systems to recognize and interpret within the proper context.
Accurate translation of weather information is essential, especially when it comes to public safety messages during natural disasters like floods or storms. Mistranslations due to flawed translation can be very dangerous and may also cause economic problems during emergency response efforts.
It's notable that current AI systems predominantly rely on datasets from standard Spanish media, which frequently ignores the unique linguistic landscapes of regions like Southern Chile. These datasets often lack the specific vocabulary needed for accurate translations of specialized weather terms.
AI systems have trouble with complex and nested phrases that are common in Chilean Spanish when describing weather patterns. The complexity often gets lost in simplified translations, leading to a loss of detail and a general reduction in clarity for weather-related communications in the region.
Despite improvements in AI translation, it's clear that completely automated translations have limits. The intricate nature of regional dialects and local terms requires not just advanced machine learning, but ongoing human review to ensure accuracy. This is especially important when handling the nuances of local weather-related vocabulary.
AI Translation Challenges Converting Chilean Spanish Dialects to Standard Spanish in 2024 - Local Food Names and Cooking Instructions Face Translation Accuracy Issues
When it comes to translating Chilean Spanish, AI translation systems face challenges in accurately rendering local food names and associated cooking instructions into Standard Spanish. While these systems can quickly process text, they often struggle with the nuances of regional Chilean cuisine. This includes accurately translating unique food names and capturing the specific techniques described in recipes. This struggle points to the need for AI translations to go beyond simply swapping words and instead incorporate a more profound understanding of Chilean culinary culture and context. Many recipes involve specialized ingredients and preparation techniques that are specific to particular areas of Chile. These unique elements often get lost in basic AI translations, which primarily rely on a standard set of words and phrases. This limitation of current AI translation methods is particularly noticeable in the culinary domain, where cultural context is crucial. As interest in exploring and sharing global culinary practices grows, the need for refined translation approaches that better handle such variations becomes apparent.
Even with recent improvements in AI translation, accurately conveying the nuances of Chilean food names and cooking instructions remains challenging. The rich cultural tapestry woven into Chilean cuisine, with its unique dishes and preparation styles, presents a hurdle for AI systems trained primarily on standard Spanish.
Take, for instance, the names of dishes like "pastel de choclo" or "curanto." These names often encapsulate a historical or cultural significance that's difficult for AI to grasp and translate accurately without losing the original context. Further complicating matters, cooking instructions within Chilean Spanish utilize verbs and phrases that might be considered informal or regional. AI often defaults to standard expressions, potentially missing the true intent of the cooking methods or traditional preparations.
Adding to this complexity, many local food terms have multiple meanings depending on the region. For example, "porotos" can mean various types of beans, leaving AI systems to guess the specific culinary context. This problem is exacerbated when recipes are handwritten, leading to OCR errors due to the informality of handwriting and use of dialectal slang.
Moreover, the specific language of Chilean cooking techniques introduces challenges. Phrases like "asar a la parrilla" or "cocinar al vapor" don't always have a perfect match in standard Spanish, and AI can struggle to capture the precise meaning, potentially leading to inaccurate or ambiguous translations.
The constant evolution of cuisine also adds a layer of difficulty. New food terms and trends emerge rapidly, making it difficult for AI models trained on fixed datasets to keep up. This is further compounded by the presence of unique ingredients with local names, like "merken," that lack direct translations in standard Spanish, leading to errors in translation when included in recipes.
Furthermore, the intricate sentence structures often found in Chilean cooking instructions can confuse AI. Nested clauses and informal imperatives can be oversimplified by the AI, potentially missing key steps or misinterpreting the intended cooking process. The rise of street food culture has added another layer of complexity, introducing a whole new lexicon of slang terms, which are largely absent from traditional dictionaries and AI training data.
It's also worth noting that the way AI translations are presented can significantly affect how accurate they're perceived. If the food names or cooking instructions appear odd or mismatched, it can lead to a decrease in user confidence. This suggests that the design of user interfaces for these AI tools is as crucial as the underlying translation algorithms themselves.
Ultimately, these challenges highlight the limitations of current AI in capturing the full richness and complexity of Chilean cuisine within translations. While AI continues to improve, the need for human oversight and refinement to ensure both accuracy and cultural sensitivity in translations remains critical. This, in turn, reveals the need for ongoing research in training AI models to better understand the nuances of regional dialects, particularly those connected to cultural practices and evolving culinary expressions.
More Posts from aitranslations.io: