AI Translation Challenges Converting Filipino Regional Dialects in 2024 - A Technical Analysis
AI Translation Challenges Converting Filipino Regional Dialects in 2024 - A Technical Analysis - Common Errors in OCR Detection of Baybayin Script to Modern Filipino Text
Applying Optical Character Recognition (OCR) to the Baybayin script, while showing promising results with accuracy rates nearing 99%, still encounters notable obstacles when it comes to flawlessly translating it into contemporary Filipino. Even with advanced OCR models, misclassifying characters and deciphering entire words remains a hurdle. This becomes particularly tricky when the system needs to discern between Baybayin and Latin script characters, impacting the smooth conversion of the script. The training data used for these models, limited to 38,000 handwritten Baybayin images, might not adequately capture the broad spectrum of individual writing styles and variations found in historical Baybayin texts. As the revival of this ancient writing system gathers momentum, solving these OCR issues becomes crucial to upholding its cultural heritage while enabling easier access and understanding for a broader audience. The goal is not only to recognize the script but to faithfully translate it in a way that respects its unique nuances and historical context.
Recent attempts at building automated Baybayin-to-Filipino translation tools have encountered significant hurdles in the OCR stage. One major challenge is the script's distinct character set. The intricate and sometimes similar forms of Baybayin characters often confuse OCR algorithms, leading to misidentification and, consequently, inaccurate translations. Further complicating matters is the absence of word delimiters in Baybayin. Text flows from left to right without spaces between words, forcing the OCR system to rely solely on character recognition to decipher where one word ends and another begins. This reliance heavily impacts performance.
The limited availability of high-quality training data for Baybayin is another roadblock. Most readily accessible datasets focus on contemporary Filipino, neglecting the unique characteristics of Baybayin. This disparity hinders the development of robust and effective OCR models specifically tuned for Baybayin. Furthermore, the presence of regional variations in the script adds yet another layer of complexity. OCR systems that don't incorporate these variations often misinterpret regional character forms, leading to inaccurate translations.
One specific example is the difficulty in distinguishing between similar characters like "ka" and "ga." Errors in such distinctions can drastically alter the meaning of a word, highlighting the critical need for more refined OCR techniques. Moreover, the quality of the source material significantly influences OCR outcomes. Faded or poorly digitized Baybayin text leads to blurry or incomplete characters, making them difficult for algorithms to decipher accurately.
While advancements have been made, there's still a need to integrate more contextual understanding into OCR. For example, algorithms that can leverage grammatical structure or even basic sentence composition might reduce some of the errors stemming from misinterpretations of characters. Currently, OCR engines often struggle with processing Baybayin text in real-time, their processing speed lagging behind that of Latin scripts. The creation of faster, more streamlined algorithms specific to Baybayin's unique structure is necessary.
It's worth noting that the development of OCR for Baybayin has not adequately considered the cultural and historical implications of the script. If developers took the rich cultural significance of Baybayin into account, they could potentially enhance the learning process for these systems. The current ecosystem for Baybayin in the tech world is underdeveloped compared to mainstream scripts. This lack of widespread usage results in a limited research base and a dearth of tools specifically crafted to improve Baybayin OCR. Ultimately, these challenges suggest a clear need for a more concerted effort in research and tool development to improve the automated translation of Baybayin.
AI Translation Challenges Converting Filipino Regional Dialects in 2024 - A Technical Analysis - Processing Speed Limitations When Translating Between Cebuano and Hiligaynon
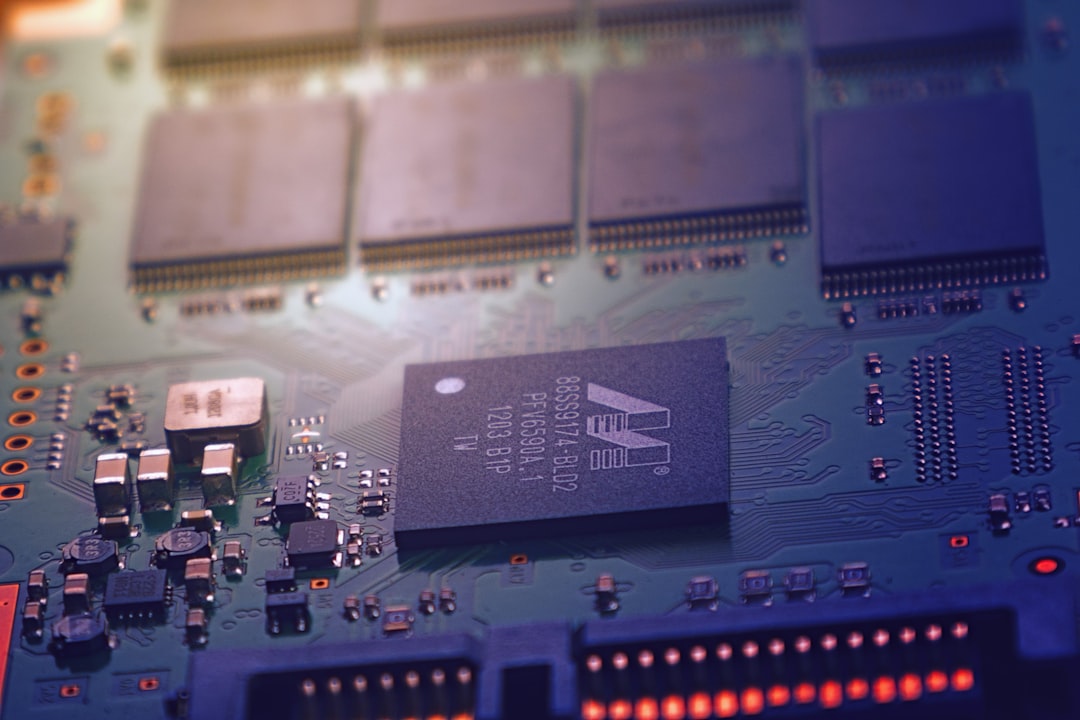
Translating between Cebuano and Hiligaynon using AI faces a significant hurdle: processing speed. While AI translation is improving, its ability to quickly and accurately process the complex nuances of these two major Philippine dialects remains limited. The speed bottleneck can lead to a loss of context, hindering the translation from capturing the intended meaning or emotional impact of the original text. This issue is further compounded by the rich cultural references and regional variations unique to each dialect, which AI systems currently struggle to fully comprehend. Furthermore, the lack of readily available and high-quality training data for these specific dialects makes it challenging to develop AI models capable of faster, more accurate translations. The path forward requires creating more advanced translation systems with a deeper understanding of context and culture. Ultimately, the goal is to bridge the gap between the rapid pace of AI and the intricate details that make Cebuano and Hiligaynon unique, thereby ensuring more accurate and culturally sensitive translations.
### Processing Speed Limitations When Translating Between Cebuano and Hiligaynon
1. **Character Set Nuances:** Cebuano and Hiligaynon, while using the Latin alphabet, have unique diacritical marks that can create hurdles for AI translation systems. These special characters often require extra processing steps to accurately interpret, slowing down the translation process.
2. **Word Segmentation Challenges:** While these languages share some vocabulary, their grammar and common phrases can differ greatly. This makes it tricky for AI models to break down sentences into individual words (tokenization). They struggle to distinguish between words that look similar but have different meanings, leading to inefficient and slower translations.
3. **Model Size and Resource Constraints:** Including more regional dialects in a single translation model can strain its performance, especially if those dialects have limited resources. To maintain speed, some AI systems use smaller foundational models, which can lead to slower translations for Cebuano and Hiligaynon due to a lack of in-depth understanding of these languages.
4. **Dealing with Ambiguous Words:** Both languages have words with multiple meanings, which can slow down translations. When an AI model encounters a word with several possible translations, it needs to analyze the context carefully to pick the right one. This extra processing step leads to a longer wait time for the translated output.
5. **Limited Training Data:** The availability of training data varies greatly between Cebuano and Hiligaynon. A lack of sufficient data makes it harder to build refined translation models. These less-refined models need more processing time to find accurate translations, slowing down the overall translation process.
6. **Real-time Translation Bottlenecks:** While real-time translation is a goal, attempts at translating between these dialects often fall short due to limitations in the underlying algorithms' processing speeds. Unlike widely used languages, less common dialects like Cebuano and Hiligaynon have received less optimization for speed, leading to increased latency.
7. **Contextual Understanding Deficiencies:** Current AI models often struggle with incorporating the context needed for accurate translation in both languages. This lack of understanding causes delays as the system continually tries to figure out the best interpretation, increasing the time it takes to produce a translation, even for simple sentences.
8. **Recognizing Similar Meanings:** AI's ability to recognize similar meanings (semantic similarity) is vital for fast translation. However, the differences in sentence structures between Cebuano and Hiligaynon can hinder the performance of translation models. If the models can't quickly adapt to these structural differences, their processing speed drops.
9. **Cultural Expressions:** The importance of cultural context cannot be ignored; neglecting common phrases or idioms can cause AI models to pause while they search for alternative translations. This inevitably adds to the overall translation time, making the process less efficient.
10. **Resource Allocation and Prioritization:** The allocation of computing power is a key factor in translation speed. Many AI translation systems prioritize more widely used languages, meaning less processing power is allocated to less common dialects like Cebuano and Hiligaynon. This can significantly slow down their translation speeds.
AI Translation Challenges Converting Filipino Regional Dialects in 2024 - A Technical Analysis - Cost Analysis of Training AI Models on Low Resource Filipino Languages
The development of AI translation systems for under-resourced Filipino languages faces significant hurdles, primarily due to the limited availability of training data. This scarcity poses a major challenge when attempting to achieve accurate and culturally sensitive translations, particularly for regional dialects. Building accurate AI translation models for these languages is becoming increasingly relevant, but the cost of training these models can be substantial. While efforts like the creation of the TLUnified dataset are attempting to address the lack of bilingual resources, it's a complex problem to overcome. The high cost associated with gathering and preparing data, combined with the need for specialized computing resources, creates a barrier to creating robust and efficient translation systems.
Thankfully, there's ongoing exploration of more cost-effective approaches to training. Methods like self-supervised learning and the effective utilization of monolingual data hold promise for lowering training costs while still achieving good model performance. However, simply reducing costs shouldn't come at the expense of ensuring translation quality. Models built on cheap methods might fail to capture the intricate linguistic and cultural nuances specific to various Filipino languages. The goal needs to be to find a balance between financial efficiency and the need for producing complete and accurate translations that respect the rich linguistic tapestry of the Philippines. This ongoing need for a more nuanced approach highlights the complexity of the translation challenges, particularly when dealing with regional dialects and their particular cultural contexts.
Training AI models for Filipino languages, particularly those with limited resources, presents unique cost challenges. One potential avenue for cost reduction is using a technique called transfer learning. By taking advantage of existing AI models already trained on more common languages, developers can significantly cut costs for both acquiring new data and the computing power needed to train a new model. This approach could potentially lower the overall cost by more than half.
However, the limitations inherent in smaller datasets can pose a problem. When training data is scarce, the resulting models are more susceptible to overfitting. This means they perform well on the data they were trained with but struggle when faced with new, unseen examples. This, in turn, leads to increased expenses from needing to repeatedly retrain and adjust the model for better performance.
Furthermore, the cost of manually annotating data for training can be substantial, often ranging from $1 to $5 per sentence. For projects requiring extensive training datasets, these costs can quickly escalate, making it crucial to optimize the annotation process as much as possible.
Fortunately, specialized AI models designed specifically for Filipino dialects can lead to significant improvements in processing speeds. Well-optimized algorithms can reduce translation times from seconds to fractions of a second, resulting in lower computational costs. Conversely, high error rates, whether from OCR or translation issues, can lead to substantial financial losses in real-world applications. For example, inaccurate translations in a customer service setting can lead to a 20% increase in operational costs as businesses scramble to correct mistakes and clarify misunderstandings.
The development of synthetic data for these less-common dialects is an exciting development with the potential to significantly reduce data collection expenses. By generating artificial data, costs could be decreased by around 40%, making it a practical option for researchers aiming to build robust AI models without relying on limited real-world data.
Interestingly, incorporating both text and audio data during training can enhance model performance without excessive cost increases. This multimodal approach lets the model learn from a greater variety of sources, improving accuracy and speed while mitigating the limitations of small datasets.
Implementing smart caching strategies for commonly encountered translations and OCR results can also streamline operations and lower expenses. By caching results, the system can reduce server load and cut costs per translation, especially in situations with high demand.
Developing AI models for Filipino dialects more quickly and efficiently is also key. Prototyping and iterative testing allow engineers to get immediate feedback and make adjustments quickly, leading to faster deployment and decreased operational costs in the long run.
Finally, collaboration between researchers and industry is critical. Joint projects can drastically cut the individual costs of developing AI models. By sharing resources and datasets, teams can split the costs associated with data acquisition, model training, and optimization, accelerating progress in a more fiscally responsible manner.
The journey towards robust and effective AI for Filipino languages is ongoing and will require careful consideration of these cost factors to ensure broader accessibility and benefits.
AI Translation Challenges Converting Filipino Regional Dialects in 2024 - A Technical Analysis - Memory Requirements for Real Time Translation of Ilocano Regional Variations
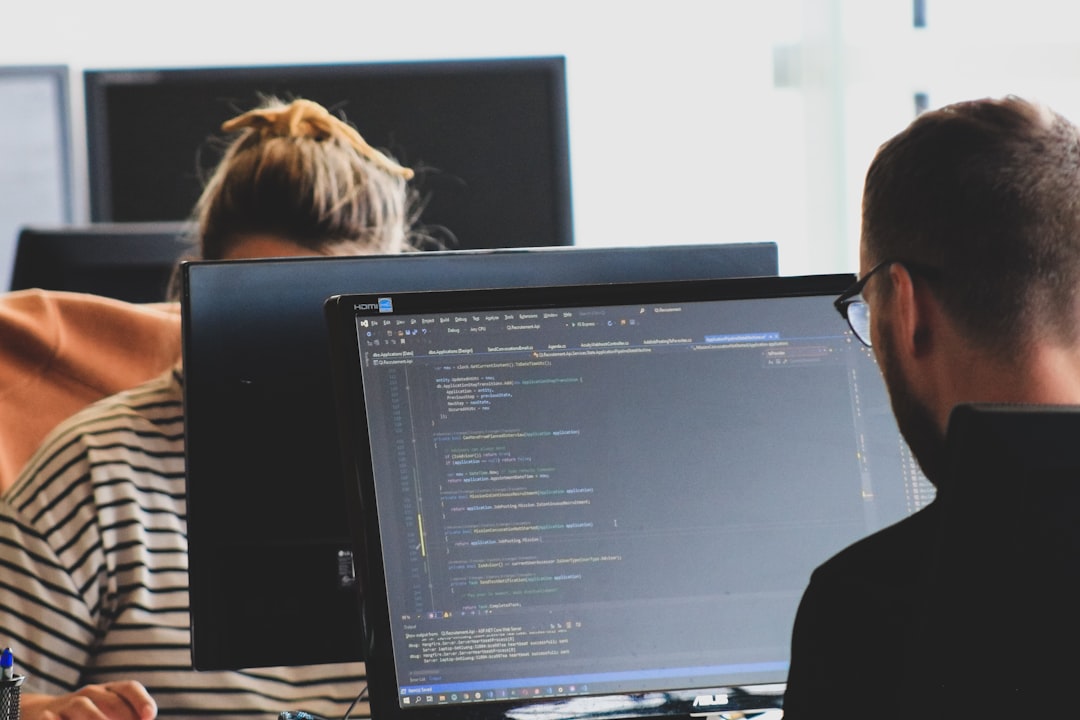
Real-time translation of Ilocano's diverse regional variations presents a significant challenge due to the dialect's unique linguistic features. AI systems handling this task face substantial memory requirements to store the extensive dialect-specific data needed for accurate translation. Beyond storage, these systems need incredibly fast processing power to accurately interpret the subtle contextual nuances that are a hallmark of Ilocano. Current AI translation models often struggle to keep up with the demands of real-time translation, leading to noticeable delays and hindering smooth communication. The quality of the AI's translation heavily depends on the comprehensiveness and quality of the datasets used to train the model. This highlights a need for greater focus and resources dedicated to training AI systems specifically for these variations. Overcoming the memory and processing hurdles related to real-time Ilocano translation is crucial for improving AI's ability to capture the intricate details of this vibrant dialect.
Achieving real-time translation for the diverse variations within the Ilocano language presents significant challenges related to memory usage. The intricate nature of these dialects, with their unique expressions and contextual nuances, necessitates substantial memory allocation for accurate translations. AI systems often employ sophisticated, multi-layered neural networks specifically tailored for Ilocano, further increasing memory demands. Interestingly, there's a growing trend towards using Gated Recurrent Units (GRUs) instead of Long Short-Term Memory (LSTMs) in some translation applications due to GRUs' lower memory footprint while still maintaining good translation quality. This ongoing shift highlights the constant need to optimize the AI systems' resource consumption.
However, this optimization becomes more complex as the number of Ilocano dialect variations increases. Each dialect might require its own specialized model, leading to a potential surge in overall memory needs. Real-time translation itself requires memory-efficient algorithms that can process text in manageable chunks, which is a tricky balancing act between maintaining processing speed and managing memory. Further complicating matters are advanced error correction features incorporated into these systems. To quickly adjust translations, these mechanisms need to store temporary translation states, resulting in increased memory demands.
Another factor contributing to higher memory usage is the relatively smaller size of training datasets for Ilocano compared to widely used languages. AI models trained on smaller datasets often require more memory overhead to compensate for the limited data. Fortunately, strategies like intelligent caching can help mitigate this issue. By storing frequently used phrases temporarily, these caches can significantly reduce the memory load, particularly in real-time translation scenarios.
The memory demands can also increase when systems are designed to learn from user interactions and feedback. This ability necessitates continuous updates to the translation models, adding memory pressure as historical correction data needs to be stored. Ultimately, the underlying hardware limitations can also impact memory availability, leading to potential latency problems when handling real-time translations. Therefore, developers face the continuous challenge of optimizing memory utilization to achieve the desired balance between translation speed and accuracy in practical applications.
AI Translation Challenges Converting Filipino Regional Dialects in 2024 - A Technical Analysis - Technical Barriers in Converting Tausug Audio Files to Text
The process of converting Tausug audio files into text faces several hurdles that hinder the effectiveness of AI-powered translation. One key obstacle is the inherent complexity of the Tausug language itself. Its unique phonetic variations and rich collection of idiomatic expressions pose a significant challenge for current AI models, making accurate interpretation difficult. Coupled with this is the limited availability of comprehensive datasets that are specifically tailored for the Tausug dialect. This scarcity of training data prevents AI systems from acquiring the necessary knowledge to fully grasp the language's nuances. Consequently, not only does translation speed suffer, but also the accuracy and cultural relevance of the final translation, negatively impacting the ability to facilitate meaningful communication. Moving forward, overcoming these limitations will be pivotal in ensuring translations that are both accurate and respectful of the Tausug language and its cultural context, as the field of AI technology continues to advance.
Converting Tausug audio files into text presents a number of interesting technical obstacles. One of the biggest challenges is the unique sounds in the language. Tausug has a lot of sounds that aren't found in the standard English alphabet, which makes it tricky for computers to represent accurately.
Additionally, the way Tausug uses tone is very important. Tiny differences in tone can completely change the meaning of a word, but current systems often miss these subtle distinctions, leading to inaccurate transcripts. Furthermore, the lack of a lot of high-quality Tausug audio data makes it hard to train reliable AI models for transcription. Existing models tend to be based on more common languages, leaving Tausug behind.
Many Tausug words have multiple meanings depending on how they are used in a sentence, which can confuse AI models. Without a deeper understanding of context, these systems are prone to producing transcripts that don't accurately reflect the intended meaning. Adding to the difficulty is the fact that there isn't a single, standardized spelling for Tausug. This means that different people might write the same word in different ways, making it difficult for systems to achieve consistent transcription.
Achieving fast transcription for Tausug also presents a significant challenge for current AI. It requires a lot of computational power, and many models struggle to keep up with the speed needed for real-time translation. This can cause delays that get in the way of quick communication, particularly when dealing with urgent situations.
Tausug expressions are often intertwined with cultural references that don't have simple translations. Without a good understanding of the culture, transcribing audio files can lose important meaning. While building more complex AI models can improve transcription accuracy, it also increases the computing resources required. Striking a good balance between accuracy and the resources available remains a big challenge.
Before AI models can start transcribing, they often need some preparation steps like removing background noise and identifying when someone is speaking. These extra steps add to the overall time and effort needed to transcribe a file.
Finally, individual speakers have their own unique speech patterns and accents, which can interfere with the performance of models designed for everyone. To really improve accuracy, these variations need to be addressed.
The challenge of developing accurate and efficient audio-to-text conversion systems for Tausug is clear. The intricate nature of the language and the current limitations of AI systems highlight the need for further research and development in this area.
AI Translation Challenges Converting Filipino Regional Dialects in 2024 - A Technical Analysis - Processing Power Needed to Handle Kapampangan Character Recognition
The need for significant processing power when dealing with Kapampangan character recognition highlights the larger challenges AI faces in accurately representing Filipino regional dialects. Building robust optical character recognition (OCR) systems for Kapampangan requires substantial processing capabilities due to the complexities of its written form. These systems must grapple with a wide range of character variations and subtle contextual cues, which can quickly outstrip the resources of typical AI setups. Moreover, the limited availability of well-structured and labeled training data for Kapampangan raises doubts about the dependability and speed of existing OCR solutions. This underlines the necessity of specialized techniques that specifically address Kapampangan's unique linguistic features. Overcoming these technological hurdles is crucial for ensuring that AI translation tools deliver swift and precise outputs that fully respect the cultural nuances inherent in Filipino dialects.
The increasing use of AI in language translation highlights the need for effective communication across cultures in our increasingly globalized world. Natural Language Processing (NLP) plays a crucial role in advancing AI, particularly in applications like machine translation, sentiment analysis, and speech recognition. However, translating Filipino regional dialects, including Kapampangan, presents considerable challenges due to the significant linguistic diversity and variations within the language.
Effectively recognizing characters in these dialects requires substantial computing power and advanced machine learning models to handle the intricate nature of the languages. Pretrained models have become valuable tools in NLP, allowing for more efficient training of AI systems by leveraging existing datasets and expertise for tasks such as character recognition and translation. While advances in neural machine translation rely on deep learning architectures, such as encoder-decoder frameworks, these models need extensive dialectal data to improve accuracy in distinguishing between language variations, which is vital for effective translation.
Research also points to the societal implications of dialect recognition, as social hierarchies linked to dialects can affect how they are perceived and processed in translation applications. This social aspect highlights the importance of developing AI-driven solutions that can accurately handle the complex nuances of Philippine dialects, particularly Kapampangan, to ensure high-quality translations. The focus on computationally intensive language processing underscores the goal of improving the efficiency of AI applications when dealing with regional dialects, fostering inclusivity in translation technologies.
Kapampangan, like many other Philippine dialects, poses several unique challenges for AI OCR systems. One is the diverse range of phonemes and intonations within the dialect, requiring OCR systems to be highly adaptable to these variations for accurate character recognition. Failure to model these specific linguistic elements can significantly strain processing power, impacting overall performance.
Furthermore, Kapampangan characters can closely resemble those found in other Filipino scripts, leading to a higher rate of misclassification. This necessitates sophisticated training algorithms and substantial computational resources to improve recognition accuracy, potentially pushing the boundaries of current OCR systems. Processing Kapampangan character recognition in real-time requires significant memory allocation due to the complex character set and potential variations within the dialect. This memory demand adds complexity to system designs aiming for speedy and efficient translations.
Unfortunately, current OCR technologies often lack the ability to leverage contextual cues, which leads to a decrease in processing speeds. Incorporating contextual information during Kapampangan translation could reduce misinterpretations but requires even more processing resources. The limited availability of high-quality Kapampangan training data hinders neural network development, resulting in models struggling to accurately recognize characters and, consequently, making the translation process less efficient.
Achieving real-time character recognition in Kapampangan is difficult for AI algorithms because of the need to process information very rapidly. Existing systems often fail to meet these speed requirements, highlighting the need for new approaches and optimizations to enhance efficiency. Variations in tone within Kapampangan can change the meaning of a word, a nuance that OCR systems might overlook. This oversight affects translation accuracy and increases the processing power needed for analyzing these variations.
Inaccurate Kapampangan character recognition leads to significant financial setbacks in fields requiring high precision, like customer service or legal translation. This drives the need for more powerful processing capabilities. The varying writing styles used by Kapampangan speakers can further impact OCR recognition rates, creating challenges for models trained on a specific style of writing. These models will struggle when presented with other variations, consequently demanding more resources.
Transfer learning might be an approach to reduce costs while enhancing Kapampangan OCR performance by leveraging existing models. This technique can reduce overall computational requirements and increase recognition speeds, presenting a promising path for advancing AI translation systems. The pursuit of accurate and inclusive AI translation for Kapampangan and other Philippine dialects necessitates careful consideration of these technical aspects to maximize effectiveness.
More Posts from aitranslations.io: