AI-Powered PDF Translation now with improved handling of scanned contents, handwriting, charts, diagrams, tables and drawings. Fast, Cheap, and Accurate! (Get started for free)
How AI Translation Tools Handle Figurative Language 7 Key Challenges in 2024
How AI Translation Tools Handle Figurative Language 7 Key Challenges in 2024 - Contextual Mismatches Between English and Mandarin Idioms in AI Translation Tools
AI translation tools face a significant hurdle when it comes to translating idioms between English and Mandarin. The problem stems from the inherent difference in how these languages express figurative meaning. English and Mandarin idioms often don't map neatly onto each other, resulting in a disconnect. Many translation tools still fall back on literal translations, leading to awkward and inaccurate outputs that fail to capture the intended meaning.
One of the core challenges is the scarcity of idiomatic expressions within the datasets used to train these AI models. Without sufficient exposure to a diverse range of idioms in both languages, the AI struggles to learn the subtle nuances and cultural context associated with them. While advances in machine learning and natural language processing are being used to boost translation speed and precision, these tools are still far from perfect when handling figurative language.
Moving forward, a key priority is to develop more specific training datasets that focus on idiomatic expressions. Equally important is the need for AI models to incorporate a deeper understanding of the cultural underpinnings of both English and Mandarin idioms. Addressing these limitations is crucial for achieving truly effective AI-powered translation in the future, especially when dealing with the complexities of figurative language.
AI translation tools face a significant hurdle when dealing with idioms bridging English and Mandarin, particularly due to the unique ways these languages express figurative language. English idioms, steeped in their own cultural context, often lack direct equivalents in Mandarin, leading to potential misinterpretations.
These tools frequently resort to literal translations, missing the intended figurative meaning and resulting in awkward or nonsensical output for Mandarin speakers. Furthermore, the training data used for these systems often emphasizes basic vocabulary and grammar, neglecting the nuances of idioms. This imbalance leads to a proficiency gap in handling such expressions.
Context plays a vital role in idiom interpretation, but AI systems often struggle to grasp these subtleties, leading to translations that might not fit the intended situation. The cultural baggage associated with some English idioms can be entirely alien to Mandarin speakers, further complicating the translation process.
Moreover, the way words are combined within a phrase (collocations) is crucial for natural-sounding language. AI tools can struggle with this, impacting the fluency of translated idioms. Idioms themselves evolve, yet AI tools might lag in updating their understanding of new variations, hampering their ability to deliver up-to-date translations.
Challenges also extend to the realm of printed text. OCR systems often struggle to accurately interpret stylized or graphically embedded idioms, potentially leading to incorrect translations. The multiple meanings (polysemy) that many words possess can trip up AI systems, as they may rely on the most common interpretation rather than the contextually relevant one.
Adding another layer of complexity is user-generated content on translation platforms. The introduction of slang and unconventional idiomatic expressions further complicates the AI's ability to consistently deliver accurate translations across diverse contexts. In the pursuit of cheap or fast translations, we risk inaccuracies in figurative language. The AI translation landscape thus remains in need of continued development to effectively bridge this language gap, particularly in nuanced areas such as figurative language. The tools available for fast or cheap translation are, as yet, often unsatisfactory with regard to idiom translation.
How AI Translation Tools Handle Figurative Language 7 Key Challenges in 2024 - AI Translation Speed vs Accuracy in Poetry Translation
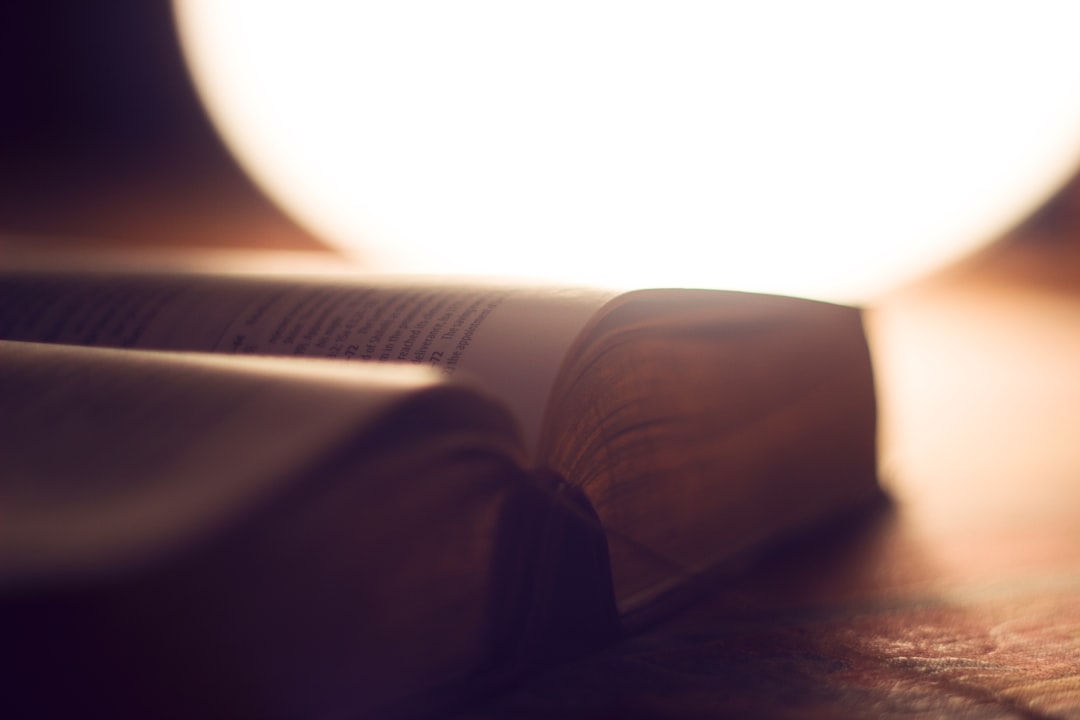
AI translation tools are becoming increasingly fast and readily available, but their ability to translate poetry accurately remains a challenge. While these tools can speed up the process of translation, they often struggle to capture the intricacies of poetic language, especially figurative language and cultural nuances. Research comparing AI-generated translations of poetry with those done by humans suggests that AI translations often fall short in conveying the intended meaning and emotional impact. The subtle layers of meaning and specialized poetic styles are difficult for AI to decipher, leading to translations that may lack the original work's depth. As AI translation continues to develop and be used for fast translations, the question remains whether these tools can ever truly grasp the nuances that define the art of poetry translation. The speed of AI translation may be a boon for some users, but this needs to be balanced against the loss of accuracy, especially when translating a genre as sensitive to meaning and cultural subtleties as poetry.
The speed of AI translation tools is undeniably impressive, with some systems capable of churning out over 1,000 words per minute. However, this rapid pace can come at the expense of accuracy, especially when dealing with the intricate nuances found within poetic works. While recent AI developments have pushed translation accuracy – particularly with transformer models achieving decent scores on literary texts – poetry remains a formidable challenge. This is due to the reliance on elements like rhythm, meter, and culturally specific references that often get lost in the translation process.
OCR technology within AI translation can handle printed poetry, but it often struggles with the creative font choices and layouts common in poetry. This can lead to errors that significantly alter the intended impact of the work. A common problem is that AI translation methods often lean on literal translations, which frequently fail to capture the figurative language vital to poetry. Metaphors and other poetic devices can lose their depth and impact, leading to translations that might misrepresent the poet's intent and emotional impact.
Even with the advancements, AI tools depend heavily on the datasets they are trained on. If these datasets lack a diverse representation of poetic language, translations can end up technically correct but emotionally bland. This can rob the poem of its artistic expression, rendering a rather sterile version. The speed of AI translation can be deceptive. Users can be drawn to fast outputs, but often this translates to sacrificing nuance and depth. This focus on speed can lead to inaccurate translations that completely miss the layers of meaning essential for understanding poetry, effectively undermining the artistry of the original work.
AI algorithms often prioritize word-for-word translations, particularly when under time pressure. While this may appear efficient, it can strip away the stylistic elements crucial to poetry. This approach can often fail to capture the subtleties of wordplay and humor, hindering the effectiveness of the AI system for poetic works.
Recent improvements in context-aware translation techniques are beginning to tackle the accuracy issues that have plagued poetry translation. However, the quality of these translations can vary significantly, especially when encountering culturally specific references. Improving AI translation for poetry requires incorporating a broader understanding of cultural symbolism and literary devices. Without this deeper comprehension, even the fastest and cheapest translations can fail to connect with the reader, leaving them with a hollow rendition of the original poem.
How AI Translation Tools Handle Figurative Language 7 Key Challenges in 2024 - Cultural References Lost in Machine Translation of Movie Subtitles
Movie subtitles often include cultural references that pose a challenge for machine translation tools. These references, sometimes called "cultural bumps," go beyond simple language and rely on a viewer's familiarity with the source culture. Machine translation often fails to capture the intended meaning and nuances embedded in these references because it lacks the necessary cultural understanding. The ability to translate these references accurately is becoming more important as AI translation develops. However, if AI tools don't incorporate a deeper understanding of cultural context, translations can become flat and lose the richness of the original meaning, potentially diminishing the viewer's enjoyment. In 2024, improving the ability of AI translation tools to handle these cultural elements is a key step towards creating more effective and engaging experiences for viewers of translated films.
One recurring issue in AI translation, especially when it comes to movie subtitles, is the loss of subtle meanings related to cultural references. A joke relying on a specific local event, for example, can become completely incomprehensible when translated literally, robbing the scene of its intended humor and connection with the audience. This highlights the challenge of conveying subtext, something AI systems still struggle with.
Another challenge arises with regional variations in language. A phrase common in one English-speaking region might have no equivalent in another, leading to translations that are confusing or culturally jarring for viewers. This issue demonstrates the limitations of AI in capturing regional dialects and colloquialisms.
Furthermore, humor—with its intricate interplay of cultural knowledge and wordplay—is a particularly tough nut for AI translation to crack. A pun based on a specific cultural reference, for instance, can be completely lost in translation, causing audiences to miss out on essential comedic moments. This loss of comedic nuance can impact character development and audience engagement.
OCR technology, while helpful for processing subtitles from video, also poses problems for AI translation when it encounters stylized or colorful subtitles. The distortions introduced by the visual variations can lead to errors, potentially misinterpreting or dropping crucial lines of dialogue, ultimately impacting the viewer’s experience.
The rapid pace of language evolution, particularly with online slang and youth culture, adds another layer of difficulty. AI tools often struggle to keep up with these changes, making translations of current idioms quickly sound dated or even nonsensical.
The contextual nature of idioms is another key area where AI stumbles. An idiom can carry different meanings in various contexts, conveying a negative sentiment in one setting and a positive one in another. AI tools, without the ability to properly assess context, can misinterpret the intended meaning, resulting in translations that significantly skew the sentiment of the dialogue.
AI systems frequently overlook how words naturally combine to form common phrases (collocations). This leads to translations with awkward and unnatural phrasing, disrupting the flow of the dialogue and hindering viewer immersion in the story.
Cultural allusions, such as references to historical figures or local folklore, often don't translate well using current AI methods. These tools frequently fail to capture the depth of such references, resulting in subtitles that fail to resonate with audiences who understand the original cultural context.
The quest for cheap or quick translation sometimes prioritizes speed over a nuanced understanding of the language. While this might provide rapid translations, it often compromises the quality, particularly when handling culturally rich material that requires careful interpretation.
Lastly, the issue of polysemy—words with multiple meanings—presents a further obstacle for AI translation. AI tools often favor the most common meaning, which might not fit the specific context of figurative language, potentially leading to inaccurate or misleading translations that can disrupt the narrative flow of the film.
These limitations point to the ongoing need for refinement and improvement in AI translation, particularly for complex content like movie subtitles, where cultural understanding and context are essential for achieving a faithful and engaging translation.
How AI Translation Tools Handle Figurative Language 7 Key Challenges in 2024 - Puns and Wordplay Recognition Limitations in Current AI Systems
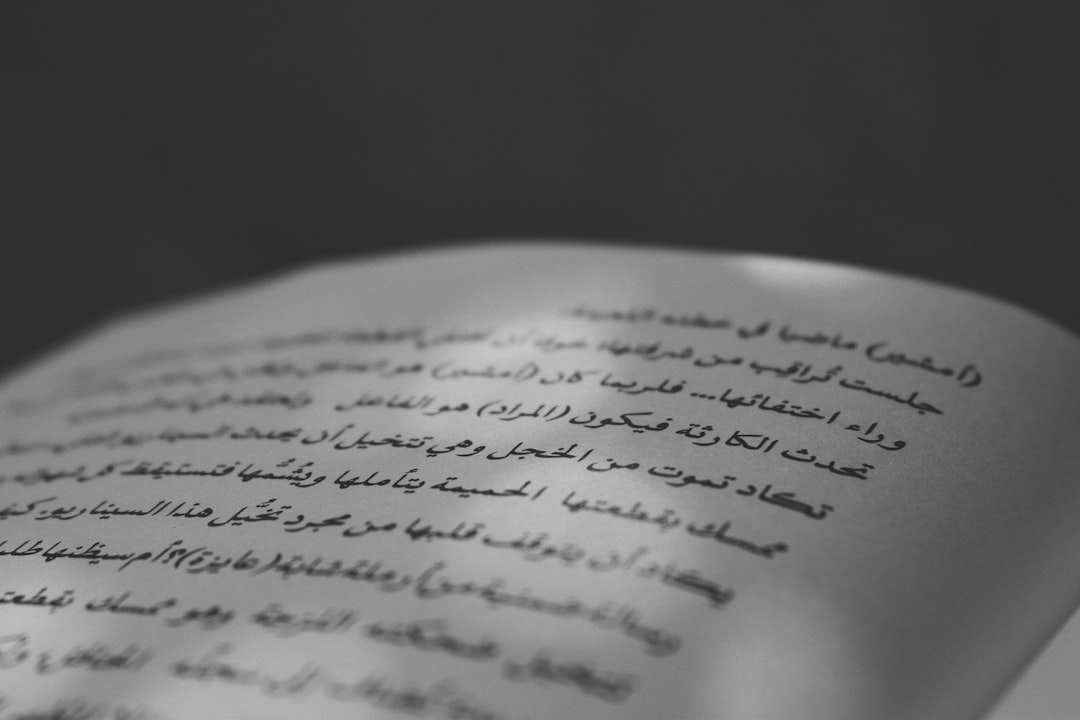
Current AI systems face a significant hurdle in understanding puns and other types of wordplay, which are crucial elements of humor in language. These systems often rely on breaking down text into individual tokens, which can hinder their ability to grasp the interconnectedness of words within a joke. This makes it difficult for AI to generate truly insightful and funny puns or translations that accurately capture the humor of the original text. Despite advances in machine learning, AI models still struggle to decipher the subtle nuances and cultural contexts embedded within wordplay. They frequently fail to grasp the intended meaning, especially when humor relies on culturally specific references. This limitation is a key obstacle preventing AI from being effectively used for creative writing or tasks requiring a genuine understanding of humor. To enhance the overall capability of AI in translation and communication, it's critical to improve their understanding of language beyond a basic level. AI models need to develop a richer grasp of context and cultural elements to accurately process and generate text filled with playful language and subtle humor.
1. **Humor's Elusive Nature for AI**: Existing AI systems face a significant hurdle when it comes to grasping and generating humor, especially puns. Puns hinge on wordplay and cultural context, two aspects that AI often struggles to fully grasp. Consequently, translations can lack the intended wit or humor, leading to a rather flat and uninspired rendition of the original.
2. **The Perils of Polysemy**: The existence of words with multiple meanings (polysemy) creates a real challenge for AI systems. They often default to the most common definition, which can result in translations that misinterpret the intended meaning, especially in scenarios involving figurative language.
3. **Cultural Blind Spots**: Many AI translation models are trained on datasets that lack a diverse representation of cultural contexts. This results in misinterpretations of idioms and culturally specific expressions, neglecting the nuances that give them meaning.
4. **Training Data Limitations**: AI translation tools often stumble when dealing with figurative language due to the lack of diverse idiomatic expressions in their training data. This limited exposure hinders their ability to develop a nuanced understanding of language and to adapt to the ever-changing landscape of linguistic innovation.
5. **OCR's Imperfect Vision**: Optical Character Recognition (OCR) technologies, while useful in processing texts, sometimes fall short when deciphering unconventional fonts or graphical elements that frequently accompany idiomatic expressions. This can lead to translation errors that omit or misinterpret essential portions of the original.
6. **Dynamic Language, Static AI**: AI tends to lean towards fixed patterns and expressions, but language is dynamic and continuously evolving. This means that as new idiomatic expressions and slang emerge, AI models might lag, hindering their ability to recognize and accurately translate contemporary language.
7. **A Lack of Literary Finesse**: Translating figurative language in literature, be it metaphors or similes, requires an appreciation of the writer's intent. This type of literary understanding is still lacking in AI systems. As a result, translated texts, while technically accurate, may miss the emotional depth or nuances of the original work.
8. **The Challenge of Word Combinations**: AI systems often struggle with understanding how words naturally combine (collocations), resulting in awkward and unnatural sentence structures in translations. This can break the flow of dialogue or narration, potentially diminishing the clarity of the translated text.
9. **Prioritizing Speed Over Meaning**: The pursuit of fast translations can lead to shortcuts that sacrifice accuracy, particularly when handling figurative language. The need for speed can result in outputs that fail to capture the layers of meaning inherent in the original text, undermining the core message.
10. **The Regional Divide**: AI systems are proficient in translating basic phrases, but they often struggle with regional variations and dialects that color idiomatic expressions. This results in translations that might confuse or even alienate audiences from different linguistic backgrounds.
These observations highlight the ongoing need for improvement in AI translation tools, especially when it comes to navigating the complexities of figurative language and cross-cultural communication. While AI translation offers impressive speed and accessibility, its limitations when handling subtle nuances in language and culture highlight the ongoing need for development and refinement in this field.
How AI Translation Tools Handle Figurative Language 7 Key Challenges in 2024 - Regional Dialect Processing Gaps in Neural Translation Networks
AI translation tools are increasingly tasked with handling diverse languages, but they often stumble when confronted with regional dialects. This is particularly relevant in areas with a high density of languages and dialects, like India, where over 1,500 dialects present a formidable challenge. While neural machine translation (NMT) offers sophisticated methods for understanding entire phrases and sentences, improving accuracy when translating regional variations remains a challenge. One core issue is the lack of extensive, parallel datasets that showcase how these dialects are translated. Without adequate training data, neural networks struggle to learn the unique grammatical structures and vocabulary differences present in regional speech. This can lead to translations that are inaccurate and fail to capture the intended meaning or cultural nuances. It highlights a pressing need to expand the range of dialects covered in training data. The goal of fostering improved cross-cultural understanding through AI relies on addressing this issue of dialect-specific translation, thereby enhancing the overall usability and precision of these tools. Moving forward, overcoming these gaps in dialect processing is essential to ensure AI translation technology truly achieves its promise of breaking down language barriers, especially when users speak in their local dialects.
Neural translation networks, despite their advancements, still face challenges when it comes to accurately handling regional dialects. This issue stems from their heavy reliance on training data primarily based on standard language forms. As a result, the nuances and unique characteristics of dialects often get lost in translation. The quality of the training data is a major factor; if it predominantly includes formal or standardized language, the AI might struggle to accurately capture the quirks and unique expressions that define a specific region's dialect.
Furthermore, AI systems aren't always adept at understanding the context in which a dialectal expression is used, which can lead to translations that seem out of place or nonsensical. For example, a common idiom in one region might have no direct equivalent in another, and the AI might simply resort to a literal translation that loses the intended meaning. The desire for speed in translation, while a desirable feature, can worsen this problem. AI models optimized for speed might favor literal translations over the more nuanced aspects of dialectal expressions, leading to a loss of depth and humor.
Another hurdle comes from the fact that many words can have multiple meanings (polysemy), and these meanings can differ across dialects. AI systems might default to the most common interpretation, potentially misrepresenting dialect-specific expressions that carry special significance within a particular region. The constantly evolving nature of language, especially slang and regional vernacular, also creates difficulties. AI systems can find it hard to keep up with these changes, and translations can quickly become dated and fail to capture contemporary expressions favored by younger generations.
OCR technology, while helpful in reading text, can also introduce issues when dealing with dialects. This is especially true for handwritten materials or texts using non-standard fonts common in certain regions. These limitations can result in inaccurate or incomplete translations due to errors in the initial recognition of the dialectal text. Beyond just language, the cultural context that shapes a dialect also presents a challenge. AI systems often lack the cultural sensitivity needed to understand the subtle humor, sarcasm, or emotional weight conveyed within a dialect.
Additionally, AI tools can misinterpret how words typically combine within dialects, leading to awkwardly phrased or unnatural translations. This can significantly alter the intended message and undermine the impact of the original expression. The rise of user-generated content adds another layer of complexity. AI systems encounter unconventional regional dialects and slang, and they might struggle to adapt, resulting in inconsistent translation quality, particularly in the realm of fast or budget translations where speed is prioritized.
These limitations suggest that the field of AI translation still requires significant development to adequately address the specific challenges posed by regional dialects. While speed and accessibility are important aspects of AI translation, it's crucial to remember that its ability to handle the nuanced and diverse world of regional dialects is far from perfect. Continued research and improvement are necessary for AI translation to become a truly inclusive tool for communication across various language communities.
How AI Translation Tools Handle Figurative Language 7 Key Challenges in 2024 - AI Translation Struggles with Ancient Text Metaphors
AI translation tools face difficulties when dealing with the metaphorical and figurative language common in ancient texts. The unique and often subtle nature of ancient metaphors doesn't easily translate into modern languages, leading to results that may not fully capture the original meaning or cultural context. Additionally, AI models often struggle to capture the stylistic elements crucial to conveying emotion or authorial intent. This can lead to translations that sound bland and miss the mark in representing the nuanced tone of the original. Researchers are continuously working to improve AI translation, but a deeper comprehension of historical languages and their cultural context is crucial to producing effective translations of texts filled with figurative language. The ongoing improvements in these tools bring into focus the balance between speed and the more nuanced understanding required for accurately handling the complex nature of ancient texts.
AI translation tools are facing a tough time when it comes to accurately handling the metaphorical language prevalent in ancient texts. These texts are often packed with metaphors, each capable of carrying multiple layers of meaning, which is a challenge for AI systems that typically break down language in a more straightforward way. The tools often end up with simplified translations that miss the mark.
A major issue is that many metaphors in ancient texts heavily depend on the situation they're found in. AI tools haven't quite mastered understanding these subtleties and frequently misread figurative language because they don't have the same kind of contextual awareness that human language learners develop.
Another factor contributing to the issue is the way these AI models are trained. Most of them are built using modern language datasets, which doesn't give them enough exposure to the unique structure and expressions found in ancient languages. Consequently, they don't handle metaphorical language very well if it's not something they've seen a lot of in their training data.
Further, the metaphors in ancient texts frequently contain specific cultural references that are unfamiliar to us today. Because AI tools don't have the same deep understanding of cultural context, they might translate these references literally, resulting in translations that miss or distort their true significance.
Furthermore, ancient texts often use symbols, which are common within their cultural context. However, AI systems mainly focus on the meanings of words and struggle with these symbols, often producing translations that fail to accurately capture the intended metaphorical meaning.
Adding to the challenge is the complex sentence structure found in many ancient languages, which makes it tricky to understand the link between words and their metaphorical meaning. This can lead to AI systems making errors in interpretation, resulting in translations that are technically correct but miss the actual intended message.
Even when dealing with digitized versions of these texts using OCR, we run into problems. The technology can make mistakes, particularly with stylized or less common scripts. These errors can then lead to further inaccuracies in how AI interprets the metaphorical content.
The continuous evolution of language also complicates things. Metaphors from ancient languages often don't have direct equivalents in our modern language. AI, which often relies on existing dictionaries and vocabularies, may not be ready for these unique expressions and can end up with inaccurate or awkward wording.
It also becomes evident that many ancient metaphors embody complex philosophical concepts that are essential for understanding the text. AI translations, unfortunately, can miss these deeper layers of meaning and just offer a superficial interpretation.
Finally, because of all these complexities when translating ancient metaphorical language, it's obvious that human expertise is still crucial. AI tools frequently lack the intuitive understanding and interpretive abilities necessary to truly capture the richness of these ancient metaphors. The need for human input to achieve an accurate understanding of the text, in this context, becomes clear. It seems that for now, AI tools, despite being fast and readily accessible, may not be the perfect solution for handling the nuances of ancient metaphors without human intervention.
How AI Translation Tools Handle Figurative Language 7 Key Challenges in 2024 - Slang Expression Errors in Social Media Translation Tools
AI translation tools, while increasingly adept at handling various language tasks, still face hurdles when it comes to accurately translating slang used on social media. Slang is a constantly changing aspect of language, differing greatly across cultures and age groups, making it difficult for AI to keep up. This dynamic nature often leads to translations that either miss the intended meaning or simply produce bland, inaccurate results that lack the vibrancy of the original slang.
While AI translation offers a clear benefit in speed and accessibility, the drive for fast or cheap translations can lead to overlooking the subtle, playful aspects of slang. As a result, translated slang can sound outdated, stiff, or simply fail to connect with the audience. This highlights an ongoing need for AI translation technology to improve its understanding of informal language, especially as online communication continues to evolve and rely on slang expressions. The focus on speed in AI translation sometimes detracts from the effort needed to really understand and capture the evolving nuances of everyday speech.
AI translation tools, while improving, still face a significant challenge when it comes to accurately translating slang expressions found in social media. The issue lies in the inherent nature of slang, which is constantly evolving and often relies on specific cultural contexts. For example, a slang term that's widely used today might be obsolete in a few months, leading to AI translations that sound outdated or completely miss the mark.
Furthermore, slang frequently has multiple meanings depending on the situation. Without a strong grasp of the surrounding conversation or cultural nuances, AI systems often misinterpret these expressions, sometimes creating awkward or even confusing translations. A large part of this issue stems from the limitations of the training data used to build these AI models. Often, the datasets primarily consist of formal language, neglecting the more informal aspects of language, like slang. This bias can severely limit the AI's ability to accurately translate slang and adapt to its ever-changing nature.
The explosion of user-generated content on social media has exacerbated this problem. Social media platforms like Twitter and TikTok create fertile ground for unique slang and unconventional expressions to emerge. AI tools are often caught off guard by this dynamic, struggling to accurately interpret these rapidly changing forms of language and often failing to grasp the subtle emotional or cultural context embedded within them.
Adding to the challenge is the polysemous nature of slang words – the ability for one word to have many meanings. AI models may automatically choose the most common meaning, potentially misinterpreting the slang and producing translations that deviate from the original intent. This issue becomes even more pronounced when considering the strong cultural ties often associated with slang. Without a sufficient understanding of the target culture, AI models can produce translations that seem nonsensical or lifeless, lacking the vibrancy and emotional depth of the original.
The way slang terms interact with each other also poses a challenge for AI. These patterns (collocations) are often different from how standard language is used. As a result, AI translations can sound stiff or unnatural, disrupting the conversational flow. Similarly, OCR systems can struggle with stylized or visually creative forms of slang, especially in the context of images and memes, hindering the translation process.
The fast-paced nature of language evolution, especially in social media, constantly outpaces the development of AI tools. New slang is born every day, and AI models lack the ability to adapt in real-time, frequently making their translations sound out of date. When speed and low cost are prioritized in translation, accuracy in handling slang is often compromised. This emphasis on fast and cheap translation can create a scenario where translations lose much of the original sentiment and nuance, ultimately defeating the purpose of communication in a dynamic social environment.
In conclusion, while AI translation tools are becoming increasingly sophisticated, their ability to handle the complexities of slang still needs improvement. The fast-paced evolution of slang and its reliance on nuanced cultural understanding highlight the ongoing need for development in this area. It suggests that, while AI can provide quick and accessible translations, they might not always be the most suitable choice when dealing with informal language, particularly in the realm of social media, where authentic and effective communication hinges on understanding cultural context and evolving slang.
AI-Powered PDF Translation now with improved handling of scanned contents, handwriting, charts, diagrams, tables and drawings. Fast, Cheap, and Accurate! (Get started for free)
More Posts from aitranslations.io: