AI Translation Efficiency How 1500 Characters Translate to Words in Different Languages
AI Translation Efficiency How 1500 Characters Translate to Words in Different Languages - Character to word ratios across major languages
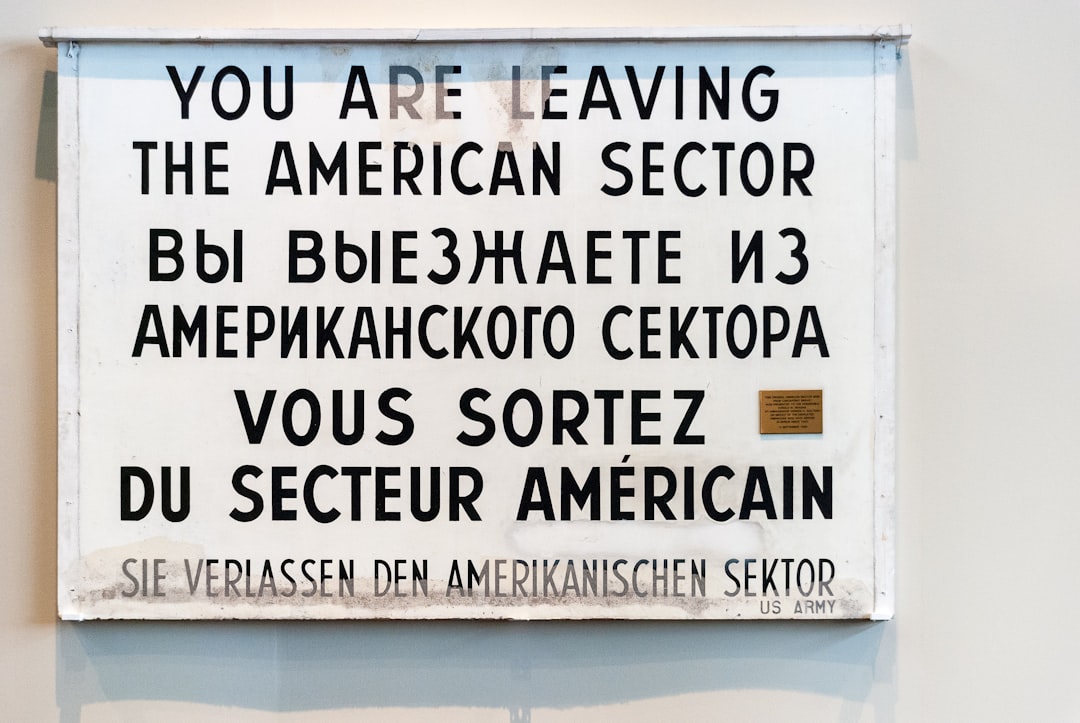
The relationship between characters and words varies greatly across languages, influencing how efficiently AI translation systems operate. Languages like Japanese pack more meaning into fewer characters, exhibiting a significantly different character-to-word ratio compared to languages like English, where the ratio is roughly 1:1.11. This disparity is important for understanding the cost-effectiveness of AI translation services, many of which base their pricing on the number of characters processed. The existence of nearly 7,000 languages worldwide, each with unique characteristics, underlines the complexities of efficient translation. AI's ability to handle these differences and optimize for character-based pricing models will be critical to producing accurate and accessible translations. Ultimately, achieving seamless cross-lingual communication in our increasingly interconnected world depends on efficient translation methods that acknowledge these inherent language variations.
The relationship between characters and words differs dramatically across languages, highlighting a key challenge for AI translation. Take Chinese, for instance, where a single character can convey a complex concept. This leads to a high character-to-word ratio, with 1000-1500 characters often condensing into about 500 words. Japanese presents a different scenario with its mix of kanji, hiragana, and katakana. A single word can involve multiple characters, increasing translation complexity and variability. Arabic, on the other hand, employs a root-based structure, meaning many words share a common root, resulting in a lower character-to-word ratio and relatively compact translations.
Romance languages, like Spanish and French, typically exhibit a ratio closer to 1:1. Their grammatical frameworks and vocabulary lend themselves to efficient communication without unnecessary length. German's tendency to form long compound words can inflate the character count disproportionately to the word count, potentially impacting the speed and efficiency of translation. Korean, with its unique writing system, can represent multiple sounds with a single character, thus having fewer characters compared to the number of words, adding another layer of complexity to translation systems geared towards brevity.
When AI tools handle translations between languages with distinct character counts, like English to Thai, there can be inconsistencies in the output. This arises from the potential mismatch between character efficiency assumptions across languages. The cost and time taken for OCR (Optical Character Recognition) is heavily influenced by these character-to-word ratios. Languages with high ratios necessitate more involved processing and take longer for extraction, leading to a greater processing burden.
Furthermore, languages like Vietnamese, which require intricate tonal understanding within a limited character set, can cause translation services to become slower and more costly. AI translation models are affected by these ratios. Those with higher character-to-word counts can strain both the translation memory systems and the benchmarking methods used to evaluate their performance. This can cause slowdowns and inefficiencies when applied to real-world scenarios. This intricate interplay between characters, words, and translation efficiency across numerous languages highlights the need for more robust and nuanced AI translation systems in the future.
AI Translation Efficiency How 1500 Characters Translate to Words in Different Languages - OCR integration boosts AI translation speed
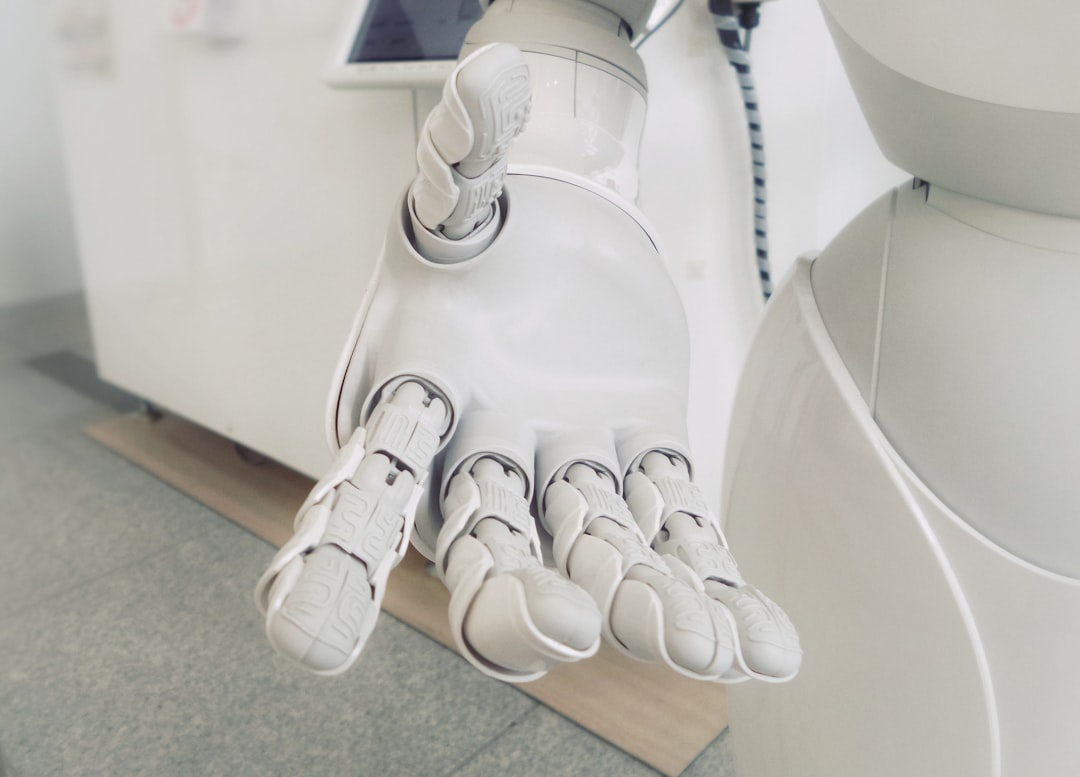
Integrating Optical Character Recognition (OCR) into AI translation systems has dramatically accelerated the translation process. OCR essentially digitizes text embedded in formats like PDFs that AI can't directly handle, allowing for faster processing and translation. This speeds things up significantly compared to traditional human translation, which often involves manual retyping or formatting before translation can begin. Although AI translation tools have found wide application in areas like software localization and content translation, the variety of languages and their unique character-to-word ratios present ongoing challenges. While OCR integration is a definite step forward in the pursuit of quicker and more global communication, it's important to remember that AI translation still has limitations. The diversity of languages, each with its own structure, necessitates continuous refinement of AI translation systems to further enhance their accuracy and address the complexities of cross-lingual communication in a globalized world. The path to smoother translation across languages requires constant development and an understanding of language-specific complexities.
Integrating Optical Character Recognition (OCR) into AI translation pipelines has emerged as a significant speed booster, particularly for handling documents in non-editable formats like PDFs. While the accuracy of OCR can vary depending on factors like print quality and font, in many cases, it's able to extract character information with remarkable precision, enabling faster processing by AI translation engines. This is especially noticeable in high-volume translation settings where manually inputting content would be a massive bottleneck.
Furthermore, advanced OCR systems are increasingly multilingual, capable of recognizing diverse alphabets and scripts without needing to switch between separate OCR tools. This unified approach significantly streamlines the translation workflow, making it faster and more efficient. Think about translating a document with sections in French, German, and English—an OCR tool can tackle all of it in one pass, bypassing the typical cumbersome process of segmenting and then individually processing the content.
The combination of OCR and AI translation is also notable for its real-time application. By immediately converting scanned or photographed documents to digital text, it paves the way for instant translation output. This immediate feedback loop is crucial for situations like customer support or travel scenarios where time is of the essence. Of course, the speed gains from this approach rely on both the OCR and the translation engine performing effectively.
While usually quite accurate for printed text, one area where OCR struggles is handwritten content. There, the accuracy can drop significantly, hindering the speed of the translation pipeline. While research continues on refining handwriting recognition, there are currently notable limitations compared to printed or digital text input.
There's also an intriguing side to this, where OCR technology can actually reduce costs associated with translation. By automating data entry and eliminating the need for manual text extraction, there's a significant reduction in human effort, leading to lower translation fees. However, it's important to note that OCR isn't a silver bullet, and for certain types of documents, the costs of refining and processing the OCR output may outweigh the benefit, especially in cases where the OCR results aren't highly accurate.
Beyond speed improvements, OCR’s integration with AI translation enhances the overall scalability of these translation systems. Companies can rapidly expand their translation capabilities into new languages without major overhauls of their infrastructure. Essentially, these OCR solutions can flex with growing needs, allowing businesses to navigate the intricate world of multilingual communication more nimbly.
One aspect where the future of OCR integration appears promising is in the development of more specialized engines tailored for different languages. For languages with complex character features, like Vietnamese with its tones, specialized OCR engines can potentially yield greater accuracy and therefore faster translation. These language-specific models hint at a future where OCR and AI translation work together in a more precise and nuanced fashion, creating a more sophisticated and adaptable translation experience.
AI Translation Efficiency How 1500 Characters Translate to Words in Different Languages - Cost comparison AI vs human translators in 2024
The cost landscape of translation in 2024 is a dynamic mix of AI and human expertise. AI-powered translation solutions are proving incredibly valuable for managing large-scale, repetitive translation tasks. Their ability to deliver swift results makes them a cost-effective choice, particularly when time is of the essence. Yet, human translators retain a critical role, especially for projects demanding nuanced understanding, cultural sensitivity, and the highest standards of quality. Many translators are now focused on post-editing AI outputs, showcasing the ongoing need for human intervention to refine and polish automated translations. While AI can significantly reduce costs in many translation scenarios, its outputs often fall short of human quality, especially in complex or culturally sensitive material. The future likely lies in a hybrid approach—leveraging AI for efficiency and cost savings, but maintaining human oversight for quality control. This balanced approach promises the most effective way to meet the diverse translation needs of individuals and organizations.
The landscape of translation costs has shifted dramatically in 2024, with AI-powered solutions becoming increasingly competitive. AI translation services can now deliver translations for as little as $5 to $10 per 1,000 characters, a considerable reduction compared to the typical human translator rates of $50 to $150. This cost difference is particularly pronounced in high-volume projects, where the scalability of AI really shines.
While AI's speed is a significant advantage – processing multiple languages with minimal delay – human translators still offer a sequential approach, often impacting turnaround times. This difference in speed can make AI particularly suitable for businesses with fast-paced content needs.
AI-powered OCR has also matured, with accuracy levels often exceeding 95% for printed documents. However, its struggle with handwritten content can increase costs when post-editing by humans becomes necessary. It's an interesting trade-off – AI's ability to rapidly digest documents versus the potential need for human intervention.
One interesting observation is that AI translation models are incorporating more context-aware algorithms. This has led to some instances where they handle idiomatic expressions surprisingly well, even surpassing some human translators who might be influenced by personal interpretation or limited experience with certain expressions.
Further, specialized AI models have emerged for certain dialects and less common languages, potentially offering better translations at a reduced cost. This can be compared to the limitations human translators may face when translating less familiar dialects or languages where a deep cultural understanding is crucial.
It's crucial to recognize that while the speed of AI translation – often under a minute – might appear to be a direct route to lower costs, the initial output can require significant human editing to reach acceptable accuracy levels. This can increase the overall costs of a project, revealing a need for careful consideration of the final goal.
The sheer scale that AI translation can achieve is notable. Some systems can now handle upwards of 10,000 documents per hour, a productivity level significantly beyond the capabilities of a human team. This difference in scale has enormous implications for cost savings in high-volume translation settings.
Furthermore, AI's expansion into areas like customer service platforms has brought about real-time translation capabilities across numerous languages. Human translators still find it challenging to maintain the same speed and versatility in real-world multilingual scenarios.
However, it's essential to acknowledge the limits of AI, especially when dealing with specialized content like legal or medical texts. In such areas, human translators often provide higher accuracy and reliability due to their nuanced understanding of legal and medical terminologies and implications.
Interestingly, hybrid translation models, integrating both AI and human expertise, are becoming more prevalent. These models try to find a balance by leveraging the speed of AI while maintaining the quality assurance provided by human oversight. These hybrid approaches offer a promising avenue for businesses seeking cost-effective and reliable translation solutions.
AI Translation Efficiency How 1500 Characters Translate to Words in Different Languages - Accuracy rates of leading AI translation platforms
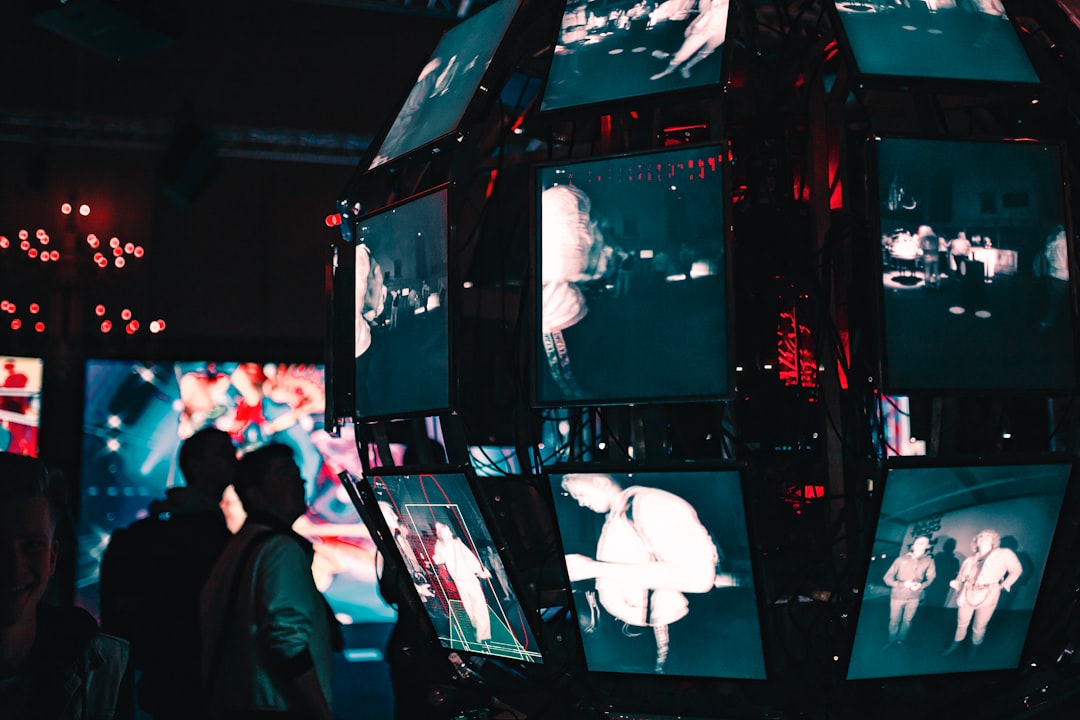
The accuracy of leading AI translation platforms is a critical factor in their increasing use. While platforms like Google Translate have shown significant progress, with studies indicating they retain the core meaning in a large percentage of translations, the pursuit of perfect accuracy is ongoing. The emergence of new players, such as QuillBot, highlights the competitive drive to improve translation quality and speed. However, the limitations of AI remain evident. Many translated texts still necessitate human review and editing to fine-tune the results, especially when dealing with cultural subtleties and context. This ongoing collaboration of human and AI demonstrates that although AI can significantly enhance translation speed, human expertise is still essential for achieving the most accurate and nuanced translations. The field continues to evolve, with AI aiming for higher levels of accuracy while highlighting the enduring need for human translators to refine and polish the output.
AI translation platforms, while showing significant progress, still face challenges in achieving consistent accuracy across all language pairs. For instance, translating between English and Thai, with their drastically different character structures and idiomatic expressions, can result in accuracy rates as low as 60%. This highlights the limitations of current AI models in handling the nuanced aspects of language.
The integration of OCR has revolutionized the translation workflow, allowing some AI platforms to process over 10,000 documents per hour. However, this speed doesn't always translate to flawless accuracy. In complex layouts, OCR accuracy can drop, with studies indicating that up to 30% of the text might be misrecognized. This suggests the need for ongoing improvements in OCR technology for handling intricate document structures.
While AI has made strides in understanding context and idiomatic expressions, human translators continue to outperform AI in conveying cultural nuances and handling regional dialects. AI models sometimes misinterpret regional variations, while humans, with their deeper cultural understanding, can produce translations that resonate with the intended audience.
AI translation services often offer attractive pricing, with costs as low as $5 to $10 per 1,000 characters. But this seemingly low price can be deceptive. Many projects require substantial human revision, ultimately pushing the cost back up to the typical $50 to $150 range of human translators. This underscores the fact that cost alone isn't always a reliable indicator of translation quality.
AI translation delivers quick results, but a significant portion of AI-generated outputs still need extensive human editing to reach professional standards. Studies suggest that about 40%, particularly in specialized fields like legal or medical texts, require considerable refinement to ensure accuracy and clarity. This points to the persistent need for human involvement in ensuring quality.
The character-to-word ratio plays a crucial role in influencing AI translation accuracy. For instance, when translating from languages like Japanese, the input character count can be up to 25% higher than the word output, creating complications for some AI models. Addressing these variations in character efficiency is critical for improving AI performance.
OCR technologies have reached impressive levels of accuracy for printed text, often exceeding 95%. However, when dealing with handwritten text, the accuracy can drop significantly to around 70%. This variability creates challenges in maintaining consistent translation quality across different input formats.
Interestingly, AI systems can display biases in their translations, potentially favoring certain dialects or reflecting limitations in their training datasets. This can impact the accuracy of translations for less common languages, highlighting the need for more comprehensive and balanced datasets.
Over time, the performance of some AI translation engines can plateau, implying that continuous updates and retraining are necessary to sustain high accuracy. This is especially true in dynamic language environments where new expressions and concepts continually emerge.
Some researchers are exploring neural networks designed to mimic human cognitive processes. This innovative approach has the potential to significantly enhance AI's understanding of context and semantics. If successful, this could push AI translation accuracy closer to human-level capabilities.
These observations highlight the ongoing evolution of AI translation technology. While AI has revolutionized translation speed and access, achieving truly human-level accuracy remains a complex challenge that requires ongoing refinement and innovation.
AI Translation Efficiency How 1500 Characters Translate to Words in Different Languages - Language pair specific translation challenges
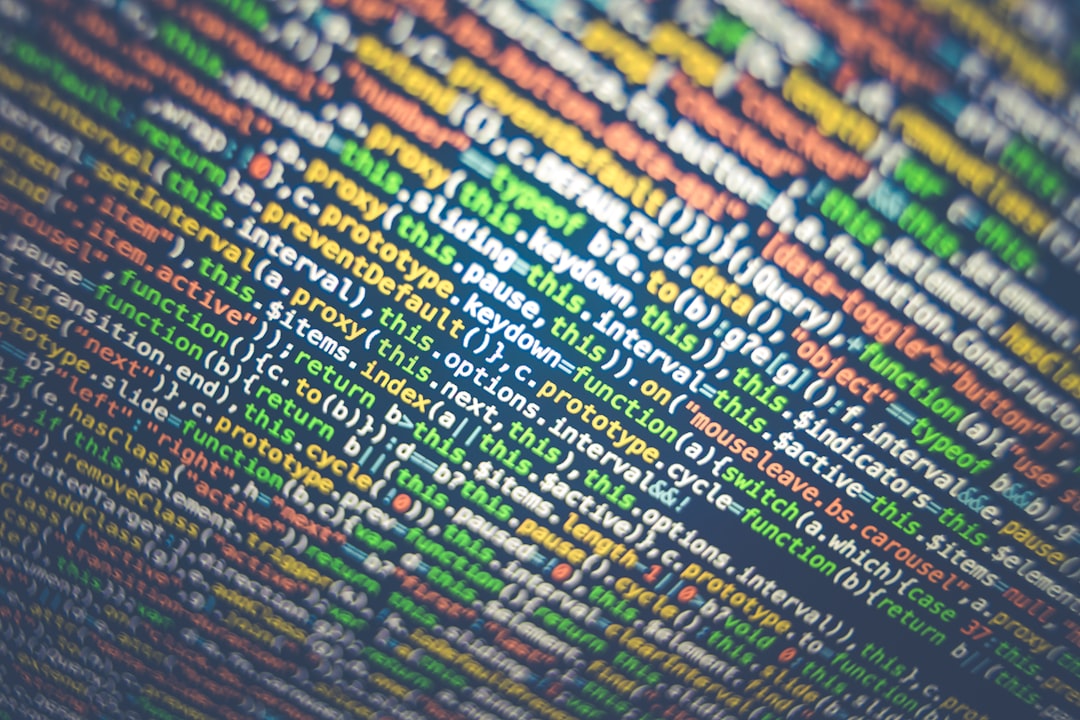
The journey towards truly efficient AI translation faces a significant obstacle: the inherent challenges presented by each unique language pair. While AI excels at handling large volumes of text and delivering fast results, the intricate tapestry of languages across the globe presents complexities that are difficult to overcome.
Different language structures pose a fundamental challenge. For example, a language like Japanese, with its blend of writing systems and varied character-to-word ratios, demands a different approach than translating between languages with similar structures, like Spanish and French. The vast disparity in character counts between languages like English and Thai can lead to inconsistencies and errors in AI-generated translations, especially when dealing with idioms and cultural nuances. Furthermore, many languages lack the substantial data sets needed to adequately train AI models, leading to less accurate translations for these low-resource languages.
These language-specific obstacles highlight the need for AI translation systems that are both robust and adaptable. It's not simply a matter of applying a one-size-fits-all approach. Instead, future progress hinges on a deeper understanding of the unique characteristics of each language pair. Only then can we hope to bridge the gap between the speed and convenience of AI translation and the accuracy and cultural sensitivity desired for effective communication across languages.
AI translation systems, while improving rapidly, still encounter hurdles when dealing with the diverse characteristics of different language pairs. For example, German's tendency to create long compound words can significantly inflate the output character count compared to the input, particularly when translating from English. This can make estimating translation costs based on character count tricky, and it adds extra workload for the translation engine.
Hindi poses a challenge with its Devanagari script and the extensive use of diacritical marks that significantly impact word meaning and pronunciation. This leads to a higher potential for errors in machine translations compared to languages with simpler phonetic systems. AI models need to handle these subtle nuances to generate accurate translations.
Languages with a high number of grammatical cases, like Finnish and Hungarian, present complexities for translation systems. Their extensive inflectional morphology can cause a significant increase in the number of characters used during translation, leading to greater computational demands on AI systems. Handling the varied forms of words efficiently is still a work in progress.
The tonal nature of languages such as Mandarin Chinese adds an extra dimension to translation. A single syllable can carry multiple meanings depending on its tone, which presents a real challenge for machine translation. Without understanding the context, accurately capturing tone can be difficult, leading to potential misunderstandings in translation.
The use of informal language and slang, particularly when translating between English and Spanish variations, showcases AI's limitations. AI systems might struggle to grasp local idioms and colloquialisms, leading to inaccurate or awkward translations that miss the intended meaning. This highlights the need for more robust and context-aware AI translation models.
Translating idioms between languages like English and Arabic can be challenging, as a direct translation often loses cultural nuance and meaning. Many expressions that sound natural in one language are lost when directly converted to the other, sometimes requiring substantial human intervention to achieve the intended effect.
When AI is used for translating South Asian languages, inaccuracies can arise from the sheer diversity of dialects. For example, in Punjabi, a single word can have distinct meanings depending on the specific dialect spoken, creating confusion for AI systems that may not be adequately trained on these variations.
OCR technology often struggles with complex scripts like those found in Armenian or Georgian. The intricacy of the letters can result in a higher error rate during character recognition, hindering the accuracy of the translation. This highlights the limitations of current OCR models in handling intricate and less common scripts.
The differences in specialized vocabulary between languages can create issues during translation. Technical terms in English, for instance, might not have exact equivalents in a language like Swahili. AI systems face difficulties in resolving these ambiguities without substantial supporting context.
Korean presents a unique challenge with its use of formal and informal language. These variations in speech can profoundly alter the meaning of a sentence. Translation systems that rely on a more static understanding of language might struggle to grasp these nuances, leading to potential misunderstandings. This shows the importance of understanding context and the dynamic nature of language when building AI translation models.
These examples demonstrate that while AI has made substantial strides in translation, language pair specific challenges still remain. Researchers need to address these issues to continually refine and improve AI systems for smoother and more accurate cross-lingual communication.
AI Translation Efficiency How 1500 Characters Translate to Words in Different Languages - Real-time speech to text translation advancements
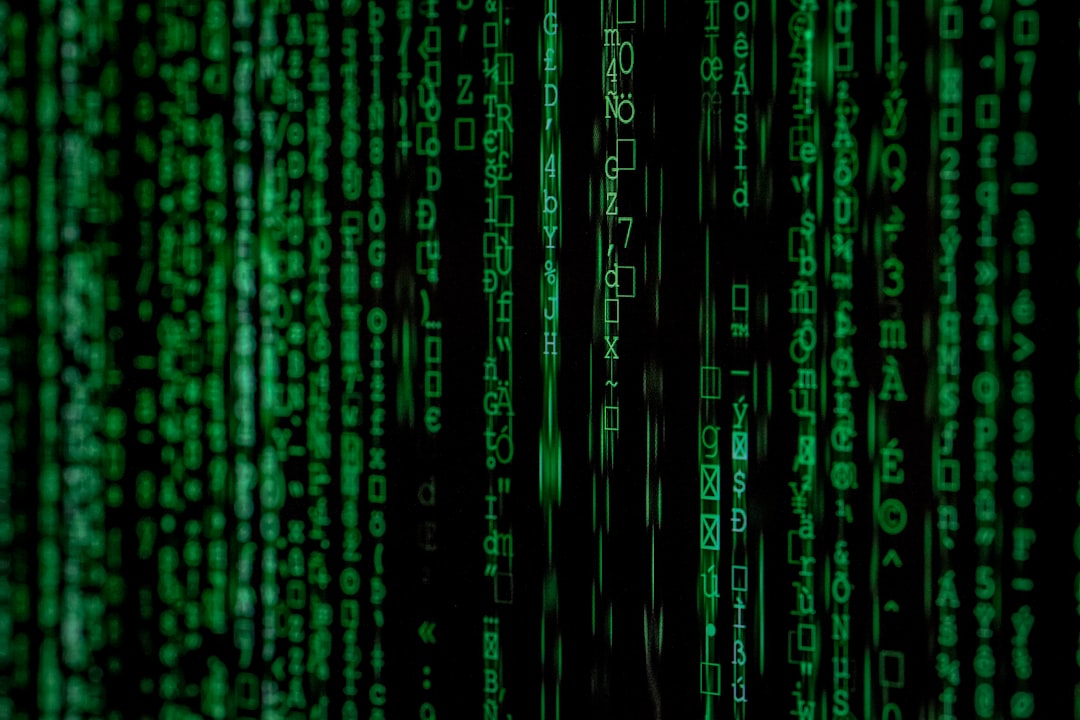
The field of real-time speech-to-text translation is seeing exciting developments, with the potential to revolutionize how we communicate across language barriers. These systems rely on a combination of technologies, including automatic speech recognition to decipher spoken words, machine translation to convert them into another language, and text-to-speech to output the translated version in spoken form. This allows for near-instantaneous translation during conversations involving multiple languages.
We're witnessing notable advancements like Meta's "SeamlessM4T" model, which is designed to handle various translation tasks within a unified framework. Meanwhile, OpenAI continues to improve its GPT-4 model, achieving impressive results in audio translation compared to other AI models. These are examples of how the field is pushing boundaries in accuracy and speed.
However, challenges persist, especially when trying to translate between the vast number of languages spoken globally. Each language brings its unique structure and quirks, demanding specialized solutions. There's a need for researchers to continually fine-tune and adapt these systems to handle different phonetic sounds, grammar structures, and nuanced cultural expressions. While AI-driven solutions show promise, they still can't fully replicate the depth of understanding that a human translator might bring, especially when preserving cultural context is vital.
Despite the obstacles, these advancements in real-time translation herald a future where communication across linguistic boundaries becomes much easier. The technology, though still evolving, has the potential to foster greater understanding and inclusivity in global communication, provided the underlying limitations and the need for human oversight are acknowledged.
Real-time speech-to-text translation is steadily improving, with some systems boasting accuracy rates exceeding 95% for clear audio. This has opened up exciting possibilities for bridging language barriers in critical settings like international summits and legal proceedings. However, a significant hurdle remains: accurately understanding accents and dialects within the same language, which is crucial for regions with diverse linguistic landscapes.
Recent research suggests that neural networks are proving to be more effective in capturing the contextual nuances of speech than older, rule-based systems. This could significantly impact real-time translation, leading to more natural and fluid output. One interesting development is the growing use of machine learning techniques that allow these systems to adapt dynamically based on user interaction. This 'on-the-fly' learning can personalize the translation experience by catering to individual speaking patterns and preferences.
While encouraging, these advancements still fall short when faced with highly complex languages. Agglutinative languages like Turkish, with their intricate suffix structures, continue to pose significant challenges for real-time processing. The availability of language resources also plays a vital role. Widely spoken languages like English and Spanish benefit from massive datasets, while lesser-known languages struggle with a scarcity of data, negatively affecting accuracy and speed.
The integration of OCR into speech-to-text translation is a notable step. This convergence allows systems to handle both spoken words and written text from images or documents, making them more versatile. For example, they could potentially translate signs or forms in real-time during international events. While automated translation can significantly reduce the need for human intervention, subtleties like humor, cultural nuances, and idiomatic expressions frequently still require a human touch to achieve truly effective communication, especially in crucial scenarios.
The field is now exploring ways to integrate emotional context into translation systems. The aim is to capture not just the words, but also the intended emotional tone. This could significantly improve cross-cultural understanding by ensuring that not only are the words translated but the speaker's intended message is effectively conveyed. Looking further ahead, the convergence of real-time translation with augmented reality (AR) holds immense potential. Imagine seeing translations superimposed on objects or environments in real-time, fundamentally altering how we navigate language barriers in everyday life. This is a frontier that could transform human interaction across languages.
More Posts from aitranslations.io: