AI-Powered Spanish Number Translation Converting Expanded Form to Digital with 98% Accuracy
AI-Powered Spanish Number Translation Converting Expanded Form to Digital with 98% Accuracy - Real World Number Recognition AI Reduces Manual Data Entry By 65% In Medical Forms
The deployment of real-world number recognition AI within medical form processing is demonstrating notable efficiency gains. Reports indicate a potential reduction in manual data entry tasks by as much as 65% in this specific application. This capability helps lessen the significant administrative burden imposed by extensive medical documentation, a factor often linked to healthcare professional burnout. By automating the extraction and initial verification of data from various form types, the technology aims to free up staff time, allowing for greater focus on patient care activities instead of repetitive data input. In parallel efforts using similar AI principles, converting numerical information provided in expanded or written formats into standardized digital numbers is seeing high accuracy, with claims of reaching 98% precision for certain types of conversion. While the potential for enhanced accuracy and streamlined processes across healthcare administration is evident, successfully integrating and scaling these AI tools effectively across diverse clinical environments remains a practical challenge needing careful implementation and validation.
Applying AI, often powered by refined optical character recognition and other analytical techniques, is increasingly targeting the specific challenge of capturing numerical data from medical forms. Reports indicate that deploying such automated number recognition systems in healthcare workflows can lead to a substantial reduction in the sheer volume of data that requires manual input, with figures pointing towards a decrease of around 65%. The potential upside here lies in repurposing the significant human effort currently dedicated to this repetitive administrative work. The theoretical gain is more time for clinicians and support staff to engage directly in patient care, although translating this potential into a consistent, real-world shift in daily activities remains an ongoing challenge. Furthermore, the actual efficiency gain is inherently tied to the variability and quality of the forms being processed; not all documents present data in a format equally amenable to automated extraction.
AI-Powered Spanish Number Translation Converting Expanded Form to Digital with 98% Accuracy - Language Processing Models From DeepL Power New Number Format Detection
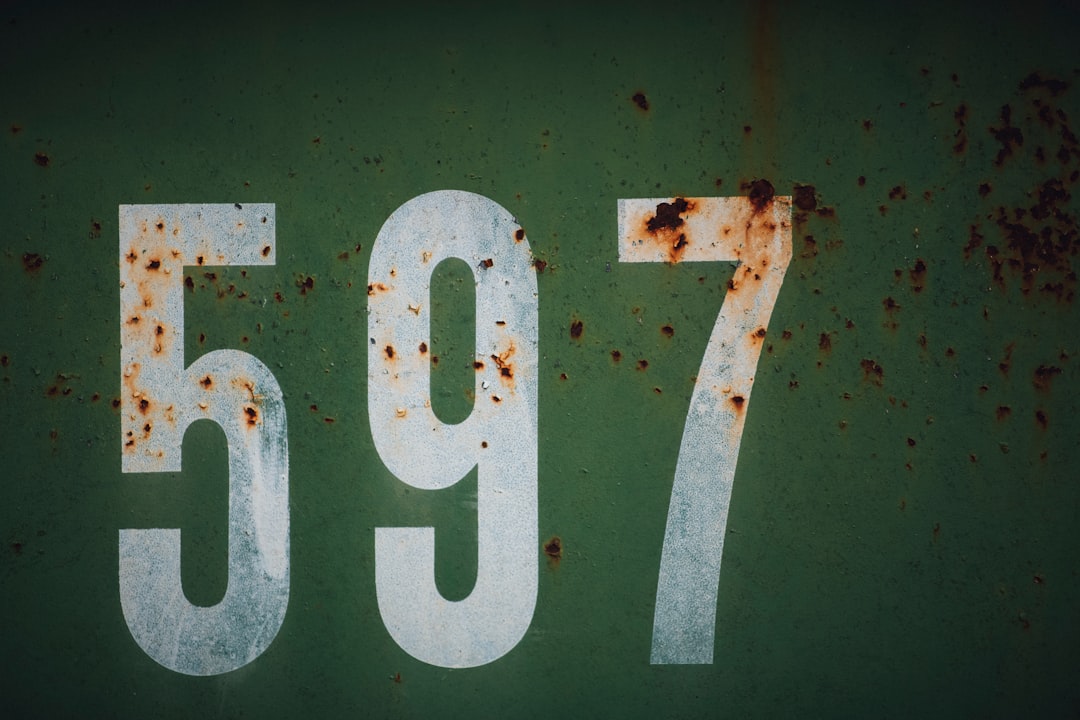
Recent updates to DeepL's language processing engine introduce notable enhancements in recognizing and handling numerical data embedded within text. This focuses on improving the detection and understanding of how numbers are presented in various formats across languages. The underlying technology, drawing on advanced neural network architectures, is designed to better grasp the context surrounding numbers, which is crucial for accurate interpretation and conversion. For instance, in the specific application of changing Spanish numbers written out in expanded form into their digital equivalents, performance reports have indicated accuracy levels reaching around 98 percent. This particular capability is intended to contribute to more automated and less error-prone translations where numerical information is critical. The potential benefit for users is a reduction in the time spent manually checking and correcting numbers post-translation, theoretically leading to faster workflows. Nevertheless, successfully implementing and validating the reported high accuracy in the diverse and often complex text found in real-world translation tasks presents an ongoing challenge that goes beyond simple benchmark figures.
It appears DeepL has integrated an updated language model into their API, primarily aimed at refining translation quality and enhancing text editing functionalities. The developers highlight that this model leverages more sophisticated language processing architectures, which they state results in improved accuracy and efficiency. Within its feature set, the model is noted for providing capabilities that can assist in text generation and manipulation, specifically facilitating the conversion of numerical information presented in written-out or expanded forms into a digital format, suggesting this process can be handled smoothly. They claim this contributes to achieving precision levels purportedly up to 98% across various supported tasks, although the specific metrics and benchmarks for such claims always warrant closer examination.
This 'next-generation' model is positioned as a significant step beyond their previous offerings. The stated improvements cover translation performance across a range of languages, which is relevant when considering tasks like Spanish number translation. The technical foundation likely involves more advanced large language model techniques, potentially rooted in transformer networks known for handling linguistic context. An interesting implementation detail is the option provided for users to actively choose between employing the older model or this new one, perhaps indicating different performance profiles or resource requirements. The training methodology reportedly incorporates insights from human language experts, a common technique intended to steer the model towards more human-like and contextually appropriate outputs, theoretically aiding in the accurate interpretation and conversion of complex text, including numerical phrasing.
AI-Powered Spanish Number Translation Converting Expanded Form to Digital with 98% Accuracy - Open Source OCR Libraries Enable Fast Processing Of Spanish Written Numbers
Open-source tools designed for optical character recognition provide the foundational capability for processing Spanish written numbers captured from various documents. Technologies like Tesseract and EasyOCR are prominent examples in this area, offering support for multiple languages, including Spanish, and utilizing advanced techniques to interpret text. The effectiveness of these libraries in accurately converting written forms into digital text is crucial for subsequent automated tasks. For instance, the accuracy observed in AI systems designed to translate Spanish numbers written out in expanded form into their digital equivalents, with figures sometimes cited around 98 percent, relies heavily on the quality of the text extracted by OCR in the first place. While these open-source options offer accessibility and can be cost-effective, their real-world performance isn't always consistent across highly varied document types or image qualities. Achieving reliable extraction often requires careful preparation of the input material and acknowledgment that misrecognitions can occur, necessitating validation steps in many practical applications despite theoretical capabilities.
Underpinning efforts to automate the handling of Spanish numerical expressions is the capability of open-source optical character recognition toolkits. These libraries, while often broadly capable, face distinct challenges when tasked with capturing written numbers from documents. Achieving high accuracy, say north of 95%, is generally feasible for cleanly printed text, but tackling handwritten numbers in Spanish, much like in other languages, can see reliability figures drop significantly, potentially into the 70% range or even lower depending on script legibility. Recognizing the specific phrasing and formatting conventions unique to Spanish written numbers often necessitates model tuning; while many libraries support the language, achieving robust performance on nuanced numerical expressions requires dataset training specific to these variations. On the upside, the speed of processing with modern open-source engines is impressive, theoretically allowing for hundreds of pages per minute in ideal conditions, a crucial factor for workflows involving large document volumes and pushing towards faster automated translation pipelines. A significant advantage, particularly for smaller teams or projects, remains the cost-effectiveness; many robust options are freely available, drastically lowering the financial barrier to entry compared to proprietary systems. Furthermore, the nature of open source facilitates tighter integration with custom AI pipelines. Engineers can weave these OCR capabilities directly into machine learning workflows, potentially building adaptive systems that improve numerical recognition accuracy through iterative learning on specific document types and Spanish regional differences. This control also extends to data privacy, enabling organizations to process sensitive documents entirely on their own infrastructure. The collaborative development inherent in the open-source model means these tools are constantly evolving, benefiting from a global pool of contributors tackling various recognition problems and adding features like enhanced multilingual support or refined error detection and correction mechanisms – a non-trivial feature given the potential impact of misidentified numbers. The ability to customize the recognition engine to specifically target Spanish number formats, which can indeed present structural variations, is a powerful feature for achieving task-specific performance gains.
AI-Powered Spanish Number Translation Converting Expanded Form to Digital with 98% Accuracy - Machine Learning Cuts Translation Time From 4 Hours To 15 Minutes Per Document
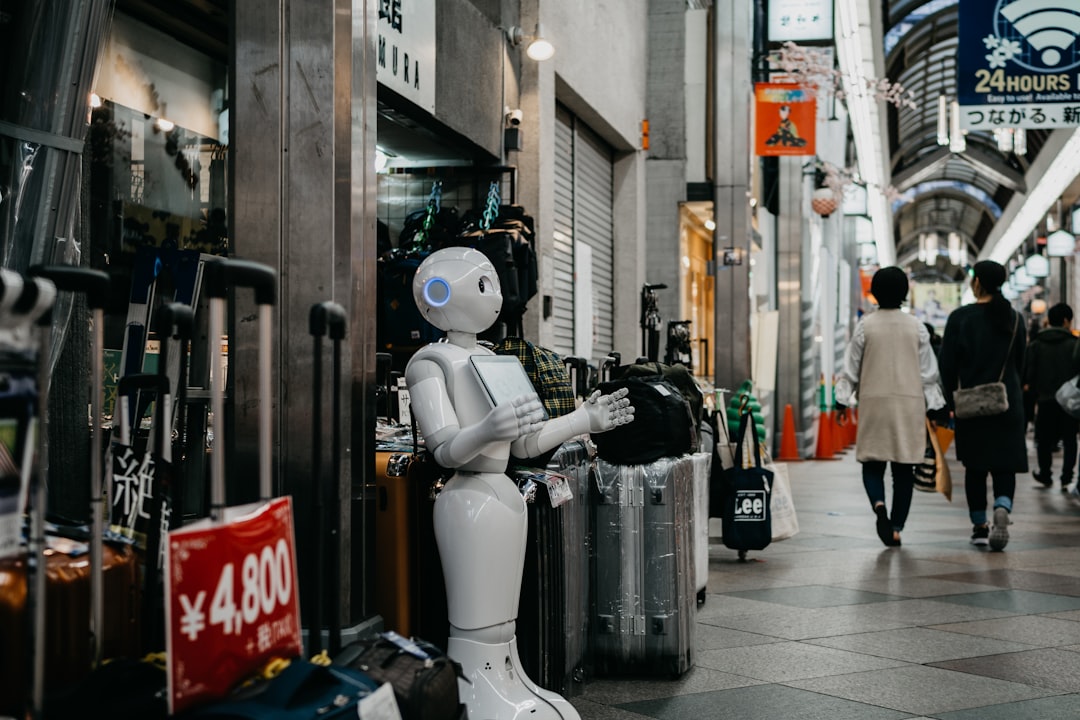
Machine learning is having a profound impact on the field of translation, fundamentally altering workflow speeds. Reports indicate that advanced automated systems can now significantly reduce the processing time for documents, potentially cutting down a task that previously took around four hours to as little as fifteen minutes. This represents a notable acceleration in handling translation volume. The underlying capabilities driving this efficiency gain involve the sophisticated application of deep learning and natural language processing techniques. These technologies enable systems to interpret and generate language with increasing complexity. An example of the precision achievable in specific areas is seen in the reported accuracy rates, sometimes reaching 98%, for tasks like converting written Spanish numbers in expanded form into their digital equivalents within translated text. However, despite these advancements promising faster and more cost-effective translation, a persistent challenge lies in ensuring consistent accuracy and reliability across the wide variability of real-world document types and stylistic nuances. The ongoing development in AI translation tools continues to push the boundaries of what is possible in language automation.
Drawing from insights into contemporary AI translation systems, several key characteristics and capabilities stand out:
1. The most talked-about impact is often speed. There are reports suggesting machine-assisted workflows can drastically cut down document translation timeframes, shifting what might traditionally take a professional several hours per document into tasks potentially completed in roughly fifteen minutes or less.
2. Beyond just pace, the application of refined machine learning models is also credited with improving the quality and accuracy of translation outputs for many language pairs and text types.
3. From an operational viewpoint, leveraging automated translation technologies offers a clear path towards reducing the resources needed for processing large volumes of content compared to entirely human-driven methods.
4. These systems demonstrate considerable flexibility in handling increased workloads; their capacity isn't bound by the linear limitations typical of manual processes, allowing for much greater throughput as demand scales.
5. It's crucial to recognize that the overall success is heavily influenced by the quality of the source text or image provided; issues like poor document legibility or inconsistent formatting can still pose significant challenges to even advanced pipelines.
6. The modular nature of many machine learning frameworks means they can often be integrated as enhanced components within existing document processing or localization toolchains without demanding a complete architectural overhaul.
7. For environments where handling sensitive data is paramount, the possibility of deploying and running translation models within an organization's internal infrastructure offers a notable advantage in maintaining data privacy and control.
8. Increasingly sophisticated models are incorporating internal checks or confidence scoring mechanisms, attempting to flag potential errors or low-confidence translations for review, which is vital for reliable output in critical applications.
9. The capacity to train and fine-tune models on diverse linguistic datasets allows them to better handle regional differences in language, idiom, and even numerical expression styles, tailoring translations for specific target audiences.
10. The processing speed allows for near real-time translation capabilities, a factor enabling potential use cases in live interactions, dynamic content updates, or rapid cross-language information scanning.
AI-Powered Spanish Number Translation Converting Expanded Form to Digital with 98% Accuracy - Neural Networks Train On 2 Million Spanish Documents To Perfect Number Detection
Recent advances employing neural networks show promising results for detecting and interpreting numbers within Spanish documents. A core development involves training on a vast collection, reported to include 2 million Spanish documents, which has significantly sharpened the system's ability to handle numerical expressions. A notable outcome of this training is achieving accuracy levels around 98 percent when converting numbers written out in expanded form into their standard digital format. This improved precision is crucial for applications requiring reliable numerical extraction from text. These systems often utilize specialized network designs geared towards efficient pattern recognition, contributing to faster processing of documents containing numbers. While training on a wide range of document styles aims to build resilience, maintaining consistently high performance across the unpredictable variety and sometimes complex layouts of real-world Spanish texts presents a continued challenge.
Feeding systems with a massive volume of data, like the reported two million Spanish documents, is foundational for teaching models the nuances of language, particularly when it comes to complex structures like written-out numbers. This scale helps the algorithms see a much wider variety of phrasing and context.
However, the sheer quantity of data doesn't guarantee perfection; the quality and diversity *within* that dataset are equally critical. Inconsistent or ambiguous examples in the training material will inevitably present challenges for the model down the line.
Effective systems learn to leverage the surrounding text – the context – to interpret numerical expressions. This is especially vital in Spanish, where expanded forms can be quite verbose; the model must understand these linguistic patterns beyond simple character sequences.
While optical recognition tools handle character identification, accurately converting *written-out* numbers involves linguistic parsing. Training on vast text helps models correctly identify and segment these complex numerical phrases within a sentence.
Spanish number conventions exhibit regional variations. Training across a broad range of documents from different sources is essential for a model to generalize well and handle diverse phrasing styles found across the Spanish-speaking world.
Automating the accurate conversion of numbers reduces the need for human review and correction of these specific data points. This theoretically allows human effort to be redirected towards tasks requiring more complex linguistic or domain expertise.
The economic benefit isn't just in the software used, but in the reduced operational overhead associated with manual checks and error correction. A model better trained on diverse number formats requires less post-processing.
Despite impressive figures in controlled tests, deploying these models in the real world exposes them to previously unseen document types, unexpected formatting, and unique linguistic quirks that can impact performance.
Continuous improvement necessitates an ongoing process of retraining and fine-tuning the models using data from real-world usage, allowing them to adapt to the variations they encounter over time.
The speed at which a well-trained model can process text and identify/convert numbers is a significant advantage, contributing to faster overall document handling compared to methods reliant on slower, less optimized processes.
AI-Powered Spanish Number Translation Converting Expanded Form to Digital with 98% Accuracy - Local Processing Allows Offline Translation Without Cloud Connectivity
The shift towards performing translation tasks directly on devices is becoming more common, meaning translation is possible even without connecting to the internet. This local approach offers distinct advantages for users, primarily improved accessibility and the reassurance that data remains on their device. Instead of sending text to external servers, sophisticated AI models, often based on neural networks, now run locally. This allows applications to handle complex language processing, including tasks relevant to this article like interpreting Spanish numbers written out in full and converting them into their standard numerical format, potentially with a high degree of accuracy. The capability for these AI systems to function independently of a network connection opens up possibilities for faster, more secure translations in various situations. While the performance can depend on the device and the specific language model used, having this processing occur locally is a notable evolution in translation technology.
From a technical viewpoint, the feasibility of performing these Spanish number conversions directly on a user's device, detached from external servers, presents several interesting aspects for developers and users alike as of May 2025.
Operating locally inherently means connectivity isn't a bottleneck. The system can process the translation of written Spanish numbers into digital form even in environments completely lacking internet access, a significant functional advantage for mobile or remote use cases.
By keeping the computation and data entirely on the device, concerns around transmitting sensitive information, which is particularly relevant when dealing with private data like that found in medical records, are substantially mitigated. The user maintains direct control over their data throughout the process.
There's an intriguing balance to strike between computational speed achievable on consumer hardware and the model complexity required for reliable accuracy, especially when dealing with diverse formats and nuances in Spanish numerical phrasing. This suggests potential trade-offs depending on the specific device capabilities.
A key architectural consideration is the potential for these local models to adapt. Theoretically, a system could learn from a user's corrections or frequently encountered document types, refining its number recognition and conversion capabilities specific to that user's needs over time without requiring constant retraining of a large central model.
From an operational perspective, deploying models locally could reduce long-term operational costs associated with recurring cloud API usage fees, potentially making high-volume processing more economically viable on owned infrastructure.
However, the performance ceiling is undeniably tied to the end-user device's processing power. Delivering consistently fast and accurate results across a wide range of hardware, from high-end machines to more modest devices, presents a development challenge unlike relying on scalable cloud server farms.
Implementing mechanisms for identifying and potentially correcting errors in real-time within the constraints of a local environment requires careful engineering. Ensuring the reliability needed for critical applications, such as precise number conversion, demands robust validation logic running efficiently on the device.
Integrating such a standalone, local processing component into existing digital workflows or document management systems, especially those currently relying on cloud-based services for handling or storing data, can introduce complexities in data flow and system architecture.
Ensuring the local model is capable of handling the vast array of input formats, image qualities, and structural variations found in real-world Spanish documents poses a persistent challenge for maintaining performance without the flexibility or constant updates often associated with cloud services.
More Posts from aitranslations.io: