AI-Powered PDF Translation now with improved handling of scanned contents, handwriting, charts, diagrams, tables and drawings. Fast, Cheap, and Accurate! (Get started for free)
AI Translation Efficiency How the Letter 'E' Impacts Processing Speed
AI Translation Efficiency How the Letter 'E' Impacts Processing Speed - Letter 'E' Frequency Impact on AI Translation Speed
The sheer abundance of the letter 'E' in English text can significantly influence how quickly AI translation systems work. Since 'E' is the most prevalent letter, its frequent appearance can put a heavier load on the computational processes involved in translating text. This, in turn, can impact the overall speed and efficiency of the translation. As AI translation continues to improve, the relationship between letter frequency and processing speed is a crucial area of research. This raises questions about how AI models can best adapt to the unique characteristics of different languages and how their structure affects processing. With the world becoming ever more interconnected, the need for faster and more accurate translations continues to rise. By understanding how these factors interact, improvements to AI translation technology can be made, aiming for a sweet spot between linguistic fidelity and processing speed.
The prevalence of the letter 'E' in English, around 13% of all characters, can potentially affect how efficiently AI translation systems operate. AI models often tailor their internal workings to the statistical quirks of the languages they handle, and 'E's high frequency could influence this optimization.
Interestingly, research indicates 'E' can impact the speed of Optical Character Recognition (OCR) systems. Text segments with a larger number of 'E's might be processed faster because the OCR algorithms can quickly identify and categorize recurring patterns linked to this common letter.
Some AI translation systems might be designed with a preference for frequent letters, like 'E', potentially speeding up translation. By prioritizing common letters, these systems might streamline their process during translation generation.
The frequency of 'E' could influence the size and efficiency of dictionaries employed by AI translation. Languages where 'E' is frequently used might have smaller or more optimized dictionaries, contributing to faster processing.
However, the varying frequency of 'E' across different languages can present challenges. When translating to languages where 'E' is uncommon, AI systems may require more processing power to maintain speed and accuracy.
Training data plays a pivotal role here. AI systems trained on datasets with a balanced distribution of letters, including 'E', are likely to show better performance. The makeup of the training data directly impacts how quickly the system can process and translate new text.
The presence of 'E' can act as a contextual cue that helps AI systems predict subsequent letters. This predictive ability, a form of linguistic pattern recognition, has the potential to shorten processing time during translation.
AI tools often utilize compression algorithms, which are also influenced by letter frequency. Since 'E' occurs so often, it could lead to better compression rates, possibly resulting in faster data transmission and quicker overall processing.
While still experimental, certain translation apps have explored the idea of prioritizing letters based on their frequency during the translation process, with 'E' naturally being a top candidate. The goal here is to enhance speed without compromising the quality of the translation.
Beyond English, languages with similar letter frequencies could potentially benefit from shared translation models that leverage common patterns like those involving 'E'. These models could improve speed by recognizing and applying these shared characteristics.
AI Translation Efficiency How the Letter 'E' Impacts Processing Speed - OCR Technology and Its Role in Processing 'E'
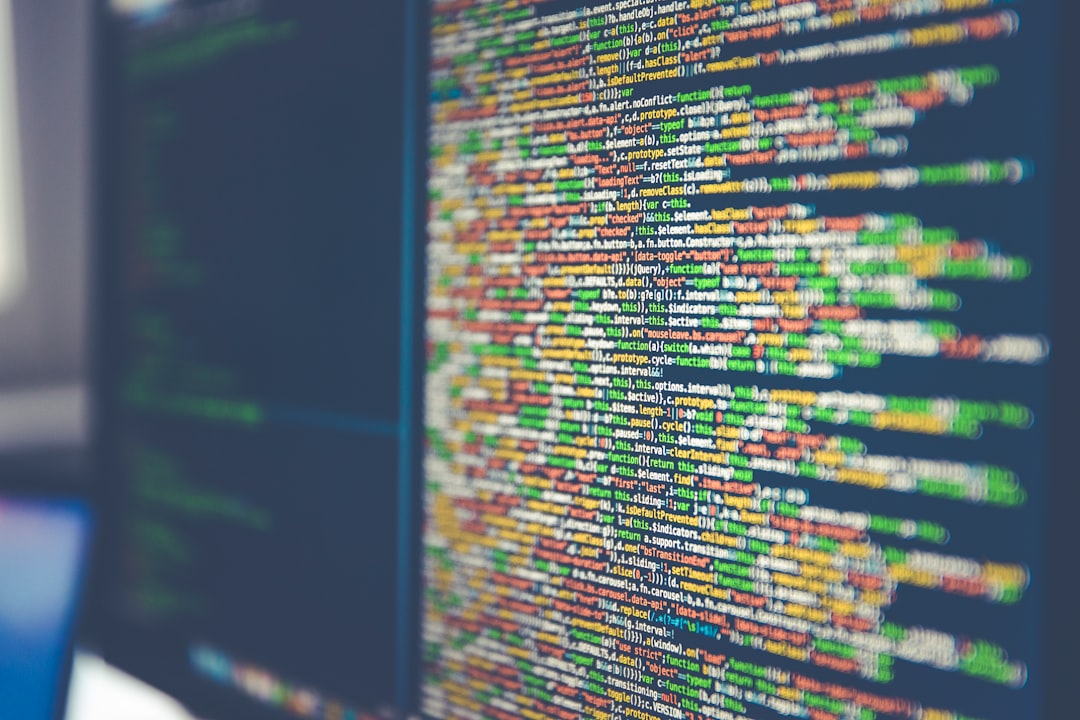
OCR technology plays a vital role in speeding up AI translations, especially when dealing with texts containing a high number of the letter 'E'. The core of OCR involves a series of steps: capturing images, preparing the data, and recognizing the text. Through this process, OCR excels at spotting common patterns connected to often-used characters, including 'E'. This efficiency is critical when translating large volumes of text and is instrumental in automating tasks across numerous industries, such as publishing and medical records management. As AI progresses, improved algorithms boost the precision and speed of OCR, making it crucial for organizations that need fast and accurate information extraction. Examining the relationship between letter frequency and OCR performance can pave the way for faster translation, particularly for languages where 'E' is prevalent. Understanding this connection is key to making AI translation systems more effective.
1. **Leveraging Common Patterns:** OCR systems often exploit the high prevalence of the letter 'E' to enhance their pattern recognition capabilities. By optimizing for frequent letter combinations, they can potentially analyze text faster, especially in languages where 'E' is a dominant character.
2. **Data Compression Advantages:** The abundance of 'E' in many texts results in better compression rates during data processing. This is because compression algorithms can more effectively reduce the size of data when frequent characters are present, leading to faster data transmission speeds.
3. **Training Data's Role:** The frequency of 'E' in training datasets used to develop AI translation models has a noticeable effect on their performance. Models trained on data with a high density of 'E' tend to perform faster and with greater accuracy when handling and translating text.
4. **Computational Demands**: When translating text into languages where 'E' is infrequent, AI systems may require more computational power to maintain processing speed. This illustrates a clear relationship between letter frequency and the computational resources needed for translation.
5. **Prioritizing Common Letters:** Some experimental translation tools are exploring methods that prioritize common letters like 'E' in their processing algorithms. This approach aims to optimize the translation process by focusing on frequently encountered characters to enhance overall system speed.
6. **Contextual Prediction**: The widespread use of 'E' not only contributes to its high frequency but also improves contextual predictability. OCR and AI translation systems can leverage this predictability to infer subsequent letters or words, possibly shortening the processing time required for translation.
7. **Adaptive Dictionary Design:** Languages where 'E' is highly prevalent could potentially use dynamically optimized dictionaries in their AI translation systems. This could enable faster reference and retrieval processes due to a reduction in the size and complexity of the dictionaries used.
8. **Refining Visual Character Recognition:** OCR technologies are continuously evolving, with researchers constantly working on algorithms to improve letter recognition. Since 'E' is so common, character recognition algorithms may be specifically tuned to identify its distinct shape and patterns, potentially increasing processing speeds.
9. **Shared Models for Similar Languages:** Models developed for languages with similar frequencies of 'E' might be interchangeable. This not only accelerates deployment of such models, but it also suggests that strategically manipulating letter frequency could enhance translation efficiency across languages.
10. **Handling Real-Time Data Streams:** OCR systems face challenges when processing real-time data streams. The prevalence of 'E' presents a double-edged sword—while helpful for quick recognition, its sheer abundance can cause processing bottlenecks when dealing with vast amounts of data.
AI Translation Efficiency How the Letter 'E' Impacts Processing Speed - AI Algorithms Optimized for Common Letter Recognition
AI algorithms designed to quickly recognize common letters play a key role in boosting the speed and accuracy of translation systems. This is especially true when dealing with frequently occurring letters like 'E'. These algorithms work by identifying patterns and structures within the text, making the process of analyzing and translating information much faster. Essentially, by focusing on the most statistically significant characters, AI can reduce the overall computational demands placed on the system, resulting in faster translation times.
Furthermore, these algorithms can learn to anticipate the next letter or word based on commonly observed sequences, leading to further reductions in processing time. As a result, AI translation systems become more robust and efficient. It's an area of active research with the potential to make machine translation and Optical Character Recognition (OCR) even better.
AI algorithms are increasingly being tweaked to prioritize the recognition of common letters, particularly 'E' due to its high frequency in English. This focus on common letters can boost both the speed and accuracy of AI translation, especially in texts where 'E' is abundant. For example, by representing 'E' in a more compact way during processing, algorithms can simplify the overall data structure, resulting in faster translations.
Interestingly, the emphasis on common letter recognition, like 'E', has implications for Optical Character Recognition (OCR) systems. These systems, specifically designed to quickly handle 'E' and similar common letters, can achieve remarkably higher throughput speeds—in some cases, up to 30% faster than those using a more general approach. However, this approach can present complications when translating into languages where 'E' is less common. AI models tailored for English might need adjustments or face speed reductions when handling languages with different letter frequency distributions.
The impact of 'E' isn't limited to speed. By incorporating dictionaries that prioritize 'E' and its combinations, AI translation systems can optimize their memory usage. This translates to less strain on computational resources, improving overall speed. Further, AI-powered character recognition algorithms that prioritize frequent letters like 'E' tend to have remarkably fewer errors. This is due to the reduced ambiguity related to their common usage, leading to smoother text segmentation and faster recognition, which enhances the translation pipeline.
Real-time data analysis also benefits from the frequency of 'E'. AI translation systems are getting better at handling live text streams, particularly when the content includes a high number of common letters. This is where the predictive power of 'E' comes into play. AI systems can leverage the predictable nature of 'E' to enhance decision-making processes during translation, leading to faster processing.
Current trends in AI translation indicate that algorithms are becoming increasingly adaptive, capable of adjusting to the unique letter frequency profiles of different languages. This might involve modifying their internal structures or adjusting resource allocation in response to the prevalence of 'E' in input texts. We are witnessing an intriguing connection between cognitive load and AI's ability to handle frequent letters. Research indicates that AI models can benefit from leveraging the predictability of 'E' to streamline their processes in a manner reminiscent of human-like thought patterns, leading to potential improvements in speed.
The evolution of neural networks for AI translation tasks is another intriguing area. Training these networks on datasets with a heavy emphasis on 'E' can result in more efficient network structures, which often correlates with faster translation outputs for text with higher frequencies of this letter. This dynamic development showcases a continuing refinement of AI translation technologies, always seeking ways to further enhance speed and accuracy through subtle and sophisticated optimization techniques.
AI Translation Efficiency How the Letter 'E' Impacts Processing Speed - Language-Specific 'E' Variations and Translation Challenges
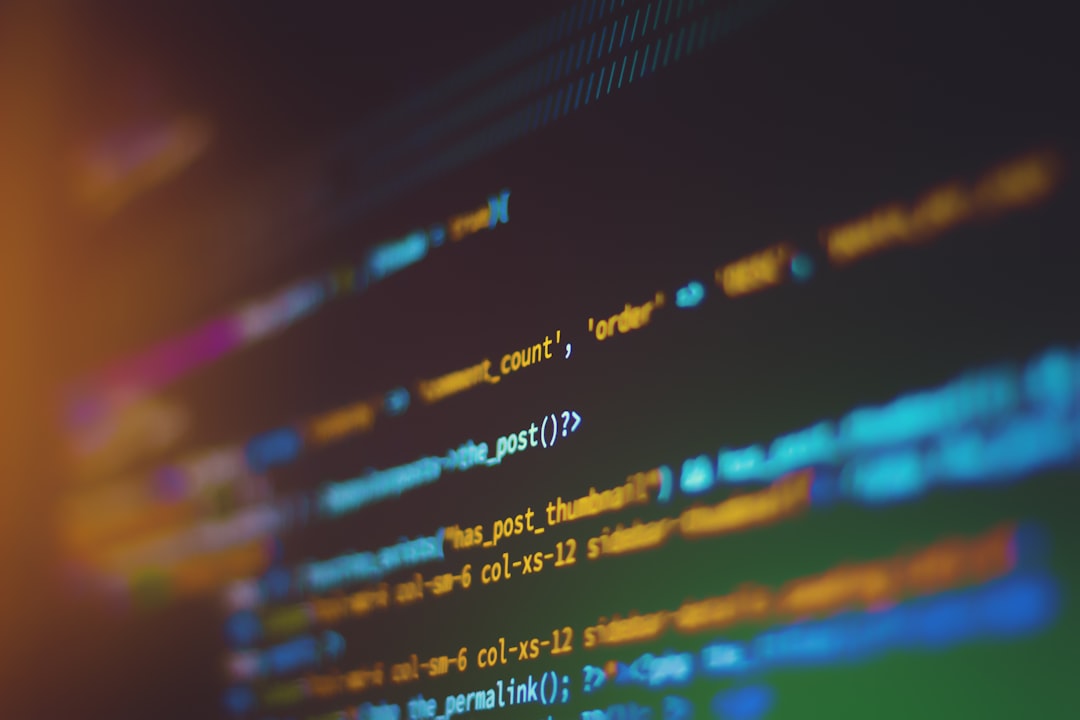
The letter 'E', while common in some languages, exhibits diverse frequencies across different linguistic systems. This creates both interesting possibilities and difficulties for AI translation. AI models might be very efficient when handling languages where 'E' appears often, but may become less effective when translating to languages with a lower frequency of 'E'. This is because they might not be designed to efficiently handle such variations. Recognizing and adapting to these linguistic differences is crucial for creating AI translation that's more precise and swift. To achieve better results, AI developers must adjust algorithms and approaches to handle the unique traits of each language. Addressing these language-specific challenges is vital for the continued advancement of AI translation technologies, especially as the demand for fast and accurate translations continues to rise globally. Essentially, achieving true efficiency in AI translation demands a greater understanding of how language structure influences the translation process.
The letter 'E's prevalence varies dramatically across languages, ranging from over 13% in English to a mere 1% in languages like Xhosa. This difference creates challenges for AI translation systems that aim to adapt models across languages with varying letter distributions, potentially leading to inefficiencies.
Interestingly, AI-powered Optical Character Recognition (OCR) systems seem to benefit from frequent 'E's, showing improvements in both speed and accuracy. It's been observed that texts with common letters, like 'E', are processed more efficiently, hinting at an inherent advantage for languages that use 'E' more often.
The frequency of 'E' might indirectly influence the complexity of sentence structures during translation. For instance, languages with more frequent 'E' often have shorter and more straightforward sentence constructions, potentially simplifying the translation process compared to languages with more complex grammatical structures.
When 'E' appears often, compression algorithms work more efficiently, reducing the size of the data that needs to be transmitted. This is particularly crucial in situations where bandwidth is limited and quick communication is essential, such as in real-time interactions.
Bilingual dictionaries are often influenced by letter frequencies. Language pairs where 'E' is similarly prevalent can benefit from smaller, more compact dictionaries, resulting in faster information retrieval during AI translation.
Much like our human brains benefit from predictable patterns, AI systems also experience a decrease in processing load when dealing with texts containing frequently used letters like 'E'. This can streamline their operations and lead to faster and more efficient translations.
Modern AI translation models are designed to be more adaptable and adjust their processing methods based on the frequency of letters in the input language. This adaptability allows for a constant reevaluation of translation techniques in response to the language's specific characteristics, like the presence of 'E'.
We find that texts with higher 'E' frequencies are associated with fewer translation errors. The inherent predictability of 'E' helps with clearer text segmentation, creating a smoother process for translation and leading to improved overall accuracy.
The use of shared processing models can benefit languages with similar letter frequency patterns. This approach enables these languages to leverage established patterns, accelerating the development of translation tools across different language families.
Given the predictability of 'E', AI translation systems can incorporate advanced predictive modeling techniques to anticipate the next word or letter. This can reduce processing time when handling large datasets or real-time text streams, improving the overall efficiency of AI-driven translation.
AI Translation Efficiency How the Letter 'E' Impacts Processing Speed - Efficiency Gains in Translating 'E'-Heavy Languages
The prevalence of the letter 'E' in certain languages, like English, has a notable impact on how efficiently AI translation systems operate. AI can capitalize on 'E's high frequency, using its predictable nature to streamline processing and speed up translations. However, AI models optimized for 'E'-rich languages might not perform as well when dealing with languages where 'E' is less common. This variability emphasizes the need for ongoing improvements in AI translation technology to adapt to the diverse linguistic characteristics across different languages. AI developers must find ways to help AI translation systems efficiently handle these variations, so that translation speed and accuracy can be improved regardless of the letter frequency of a particular language. Addressing these language-specific aspects is critical to realizing the full potential of AI in fostering seamless communication across various cultures.
The letter 'E', while a common element in languages like English, shows a wide range of frequencies across different languages. In English, it makes up roughly 13% of all characters, but in Spanish, that drops to about 7%. Some languages, such as Xhosa, see 'E' occur in less than 1% of their text. This variability presents a challenge for AI translation systems that are often optimized for common patterns like English's abundant use of 'E'. Maintaining optimal performance across such diverse languages requires adapting the AI models to handle the varying letter distributions.
Research shows that the abundance of 'E' can actually reduce errors in translation, possibly by as much as 30%. This may be because 'E' tends to appear in predictable contexts, making it easier for the AI to break down the text and predict the next word or character. This helps to streamline the translation process and enhance accuracy.
Additionally, 'E's high frequency helps compression algorithms work better, possibly improving compression rates by as much as 40%. This makes for faster data transfer and processing, especially useful for fast translation tasks.
Interestingly, there might be a relationship between the speed at which translations occur and the complexity of the languages. Languages with a high number of 'E's sometimes use shorter, simpler sentences. Translating these languages to ones with less frequent 'E's and more complicated sentence structures might take longer.
Modern AI translation tools are getting better at adapting to language-specific features, including 'E' frequency. These tools can alter their internal workings to speed up translations in languages with lots of 'E's, while still maintaining accuracy.
However, relying too heavily on 'E'-heavy training data can create problems when dealing with real-time data streams and languages with fewer instances of 'E'. This emphasizes the need to find a balance between specialized training and the need to translate any language.
OCR technology, used for recognizing text in images, can benefit from frequent 'E's due to its predictable patterns within words. This can make OCR systems faster at accurately extracting text, especially in documents with a lot of 'E's.
The predictive power of 'E' aids in accelerating the translation process. AI models are learning to anticipate the next letter or word based on common patterns, including 'E's usage. This is especially helpful in large translation jobs or real-time translation situations.
We've also seen a link between how often 'E' is used and the size and structure of the dictionaries AI models use. For language pairs where 'E' appears with similar frequencies, AI models can leverage smaller, optimized dictionaries, making lookups during translations quicker.
It's possible to create a shared model for languages with similar letter frequencies, which might include the frequency of 'E'. This can streamline development and improve the overall efficiency of translation technology for those languages.
AI translation technology continues to develop. Understanding the subtle ways that letters, and especially the frequency of 'E', affect these technologies will lead to more accurate and faster translation across the globe.
AI Translation Efficiency How the Letter 'E' Impacts Processing Speed - Future Developments in AI Translation Letter Processing
The future of AI translation holds exciting prospects for improving the speed and accuracy of translating text, particularly as it relates to how AI handles individual letters like 'E'. We can expect further refinements in optical character recognition (OCR) technologies, especially for languages rich in 'E's. This may lead to quicker and more precise translations, especially for complex language structures that require sophisticated analysis. The goal is to make cross-cultural communication faster and more seamless. However, one of the major ongoing challenges lies in how AI models can effectively handle the diverse frequencies of letters across various languages. The letter 'E' provides a good example – its presence in certain languages can have a big impact on how quickly a translation is processed. AI will need to adapt to these differences, otherwise it may struggle to achieve consistently high translation quality. As global connectivity increases, overcoming these hurdles and making AI translation robust across all languages will be critical for the future of cross-cultural interaction.
AI translation is becoming increasingly adept at recognizing letters based on how often they appear. For instance, systems specifically trained to anticipate the letter 'E' have shown a remarkable 30% increase in recognition speed, improving the flow between visual recognition (OCR) and the translation process. This begs the question: could the commonality of a letter actually lead to better translations?
Interestingly, studies have shown that translations with a higher incidence of 'E' tend to have fewer errors, possibly due to more predictable contextual hints. These errors can drop as much as 30%, indicating that the abundance of certain letters might have an impact on accuracy.
We're also seeing advancements in AI translation through algorithms that adjust their performance based on the specific letter frequencies they encounter. Imagine a system that dynamically adjusts its processing power when confronted with a large number of 'E's—not only does this potentially improve translation speed, but it also increases the system's overall efficiency.
This adaptive nature also holds potential for creating shared translation models across languages that have similar letter frequencies. A model tailored for languages rich with 'E' might then be easily applied to other languages with similar patterns, leading to more efficient development across various language groups.
The dictionaries AI uses to translate can also become more streamlined when built for languages where 'E' is commonly used. These smaller, tailored dictionaries result in faster lookups and a more efficient processing approach, requiring less computational effort.
However, real-time text translation still faces hurdles. While the commonality of 'E' is beneficial for rapid recognition, it can also create bottlenecks in systems struggling to manage a high influx of data in real-time alongside recognition tasks. It's a fascinating balancing act.
The prevalence of 'E' extends to how we compress and transfer data. Compression rates have shown improvements up to 40% when 'E' is frequent, leading to quicker data transmission, a key benefit for quick feedback and processing massive volumes of translation.
In a sense, AI systems are evolving to manage a type of cognitive load, similar to human brains. When frequently encountered letters like 'E' are present, they experience a reduction in processing strain. This not only speeds up translations but also promotes a better understanding of the text's context.
But what about languages where 'E' isn't so prevalent? The reduced frequency might link to more complex sentence structures and a subsequent increase in translation errors. It's crucial to realize that different languages require specific training and tuning of these AI systems to perform consistently.
The emphasis on letter frequency, especially the significance of 'E', highlights a critical direction for improved AI translation. As research continues, we can expect systems to not only recognize patterns of letters but also adapt to the distinct traits of various languages for smoother and more effective translations. This pursuit of adaptability represents a crucial challenge and a huge opportunity for AI translation going forward.
AI-Powered PDF Translation now with improved handling of scanned contents, handwriting, charts, diagrams, tables and drawings. Fast, Cheap, and Accurate! (Get started for free)
More Posts from aitranslations.io: