AI-Powered PDF Translation now with improved handling of scanned contents, handwriting, charts, diagrams, tables and drawings. Fast, Cheap, and Accurate! (Get started for free)
AI Translation in Chaos Leveraging Unconventional Methods for Rapid Language Processing
AI Translation in Chaos Leveraging Unconventional Methods for Rapid Language Processing - Neural Machine Translation Expands to 200+ Languages
Neural Machine Translation has evolved rapidly, now encompassing over 200 languages. This expansion prioritizes languages that previously lacked readily available translation tools, promoting wider digital access. The approach relies on innovative methods, allowing translation between languages even without prior training specifically for those pairings. This "zero-shot" capability is a significant step forward in flexibility and adaptability. Furthermore, a vast dataset built with contributions from thousands of professional translators has led to marked improvements in translation quality and diversity. This improvement is crucial for preserving linguistic variety, particularly for endangered languages. While impressive, it's important to remember that the increasing scope of machine translation requires ongoing evaluation to ensure its efficacy across such a diverse range of languages and cultures. The future of AI-driven translation remains a dynamic space where both progress and critical scrutiny are essential.
AI translation has taken a massive leap forward with the expansion of neural machine translation (NMT) to over 200 languages. This expansion is not just about adding more languages to a list, it's about fundamentally changing how these systems learn and operate. They now leverage a wider array of linguistic data, including contributions from a vast network of translators, enabling models to translate between language pairs they haven't been specifically trained on. This "zero-shot" translation capability is a testament to the advancements in understanding how languages are structured and related.
One interesting aspect is the focus on low-resource languages, many of which previously had limited or no machine translation tools. This development showcases the power of transfer learning, where models trained on a massive, multilingual dataset can be effectively adapted to handle languages with minimal specific training examples. This approach promises to bridge the digital divide and provide access to translation for communities that were previously left out.
Naturally, expanding to such a vast number of languages comes with new challenges. Ensuring translation quality across this diverse range requires innovative data acquisition methods. While promising, there is always the possibility of the new model inheriting biases present within the training data, an area researchers are actively exploring mitigation strategies for. The democratization of language access, through the free availability of this NMT scaling effort, has tremendous implications, both for preserving endangered languages and fostering further research into the future of universal translation. It seems likely that this approach will continue to refine our understanding of how languages are interconnected, paving the way for more nuanced and accurate AI translation across an ever-growing number of languages.
AI Translation in Chaos Leveraging Unconventional Methods for Rapid Language Processing - Metax AI Focuses on Underrepresented Languages
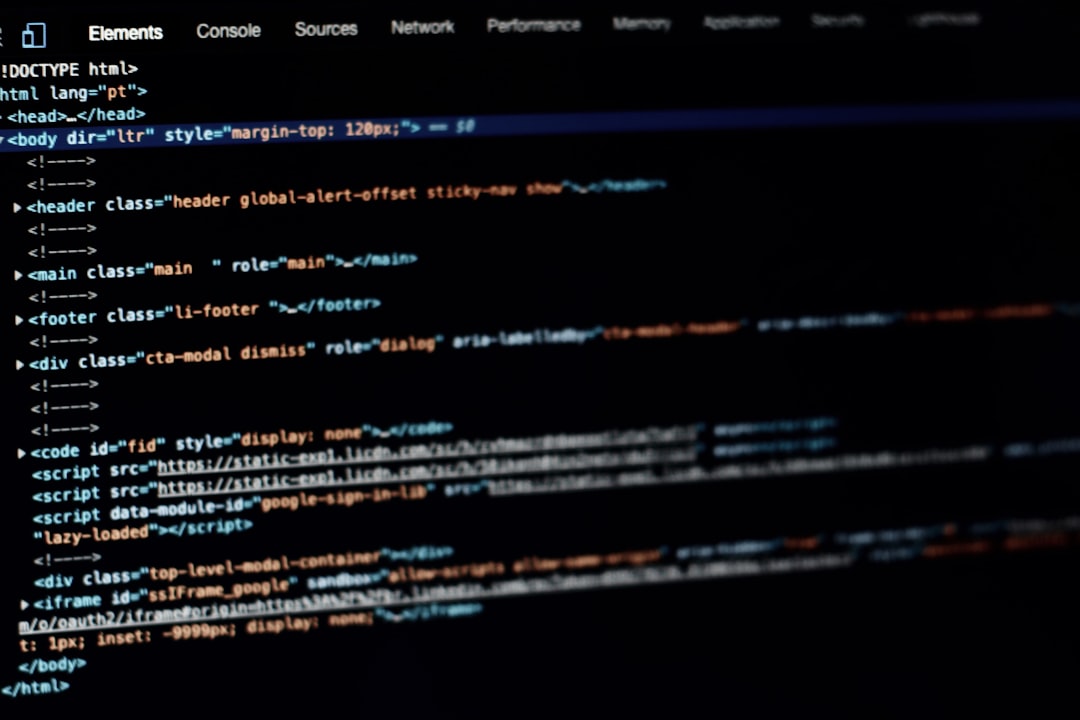
Metax AI's work is focused on a crucial aspect of AI translation: addressing the needs of languages that are often overlooked by mainstream systems. Their efforts, under the "No Language Left Behind" banner, aim to improve translation quality for languages that have historically lacked sufficient translation resources. The core of this effort is the NLLB200 model, a neural machine translation system capable of translating between over 200 languages. This model has been trained on a substantial dataset of professional translations, particularly focusing on improving the quality of translations for the 39 lowest-resource languages in the dataset. While this initiative holds considerable promise for bridging language barriers globally and promoting access to translation for underrepresented communities, critical evaluation of the model's performance remains important. This is particularly crucial to monitor and mitigate any potential biases inherited from the training data. The hope is that by expanding the reach of AI translation in this manner, communication can become easier and more inclusive across diverse cultural and linguistic landscapes. However, ensuring high quality and mitigating bias will require ongoing attention as the technology advances.
One particularly interesting area within AI translation is the growing focus on languages that are less commonly used, often termed "low-resource" languages. A significant portion of the world's languages—a staggering number approaching 7,000—fall into this category. These languages often lack a substantial digital footprint, resulting in a scarcity of readily available translation tools and resources. This disparity creates a significant barrier to information access and communication for the communities that rely on these languages.
Researchers are finding that transfer learning techniques are proving incredibly useful in bridging this gap. By leveraging knowledge gained from more commonly used languages, AI models can be adapted to handle languages with limited training data. This transfer of knowledge, however, isn't always seamless. Low-resource languages can have unique grammatical structures and vocabulary that deviate considerably from higher-resource languages, presenting challenges to standard AI translation models. Collaborating with native speakers and linguists is proving essential to refine models, ensuring they can capture these nuances accurately.
Another related aspect that's growing in importance is OCR, or Optical Character Recognition. OCR tools are vital for extracting text from images, a crucial step when dealing with languages that may not have a strong digital presence. This ability to digitize documents and printed materials makes a wealth of previously inaccessible information available for translation. While there are benefits to expanding translation for these languages, like improving economic opportunities for businesses targeting local communities, there are inherent challenges too. Regional dialects, for example, often possess unique vocabulary and grammar, requiring specific adaptations in the AI models.
The speed of translation is another area of intense focus. Advances in neural network architectures allow parallel processing, enabling faster and more efficient translation. These advancements have significant implications for situations requiring rapid responses, like real-time communication platforms. It's not just about speed, though. AI models are also incorporating context-aware systems that aim to understand the intended meaning of phrases, thereby decreasing the likelihood of awkward or misleading translations, especially when dealing with idioms or culturally specific language.
While the advancements in AI translation for low-resource languages are certainly promising, it's critical to acknowledge persistent concerns about bias. The datasets used to train these models could contain biases that inadvertently get reflected in the translations. Further research is crucial to ensure these models represent a variety of perspectives and do not inadvertently perpetuate stereotypes or misinformation when translating, especially within communities that may be already marginalized. The future of AI translation, in this context, hinges on balancing technological advancements with an awareness of the potential for bias and a commitment to ensuring equitable access to information for all language communities.
AI Translation in Chaos Leveraging Unconventional Methods for Rapid Language Processing - Statistical Machine Translation Evolution Since the 1990s
The field of machine translation underwent a major shift in the late 1980s and 1990s with the emergence of Statistical Machine Translation (SMT). Prior to this, machine translation was largely based on manually crafted rules. SMT, however, brought a data-driven approach, focusing on extracting translation knowledge from vast collections of bilingual text. The core idea, first proposed in the early 1990s, was to treat translation as a machine learning problem, using statistics to analyze patterns and predict translations.
Early SMT systems, developed primarily at IBM, eschewed linguistic rules, instead relying on identifying commonalities between comparable texts across languages. While these systems were innovative, they faced challenges related to complexity and were initially not widely adopted due to the dominance of rule-based methods. However, over two decades, various improvements to SMT paved the way for continuous progress in translation technologies.
The transition from rule-based to SMT was a pivotal moment in the history of machine translation, as it marked a fundamental change in approach. The legacy of SMT remains significant, even as more recent methods, like Neural Machine Translation (NMT), have become increasingly popular. Understanding the progression of SMT is crucial, recognizing both its advancements and limitations, particularly when considering issues like translation quality for less common languages and potential biases in training data. This historical context provides a valuable foundation for navigating the ongoing evolution of AI-powered translation.
Statistical machine translation (SMT), emerging prominently in the 1990s, marked a turning point in the field of translation, shifting the focus from manually crafted rules to data-driven approaches. It was a significant departure from the rule-based methods that had dominated the field since the 1950s. The core idea, first proposed by Brown and colleagues in 1990, centered on extracting translation knowledge from vast amounts of data rather than relying on human-defined linguistic rules. SMT treats translation as a machine learning problem, statistically analyzing large bilingual datasets to identify patterns and predict likely translations.
While several SMT models were formally introduced in 1993, it wasn't immediately widely adopted due to its inherent complexity and the prevailing dominance of rule-based machine translation (RBMT). Early SMT systems, notably those developed at IBM Research, focused on identifying patterns in text across languages, operating without explicit linguistic rules. The growth of readily available internet resources and parallel text datasets played a key role in the increasing viability of SMT, providing the necessary data to fuel these early systems.
Over the next two decades, SMT saw considerable advancements, leading to continuous improvements in machine translation capabilities. However, the mid-2010s witnessed a significant paradigm shift with the rise of neural machine translation (NMT). NMT leverages deep learning techniques that allow it to analyze and understand entire sentences rather than just individual phrases, paving the way for more accurate and nuanced translations. This transition to NMT, which is capable of processing whole sentences rather than isolated words or phrases, represents a huge leap forward in the field.
One interesting facet of NMT is its ability to generate translations for language pairs it hasn't been explicitly trained on. This "zero-shot" translation capacity is a testament to the improvements in how AI systems understand the relationship between different languages. However, this flexibility can lead to challenges in ensuring translation accuracy and quality across such a vast array of languages. It also highlights how NMT's success, and its distribution of improvement across languages, isn't always uniform. Some languages have reaped more benefits than others.
The integration of Optical Character Recognition (OCR) technology further amplified the power of machine translation. OCR’s ability to convert scanned documents and images into machine-readable text makes a wealth of previously inaccessible information readily available for translation, accelerating the process of digitizing historical documents and other resources in diverse languages. This is particularly crucial for preserving languages with limited digital resources.
Yet, NMT isn't without its own set of difficulties. Some NMT models have been observed occasionally generating translations that don't accurately reflect the original source text, often called "hallucinations". These inaccuracies underscore the continued need for vigilance and validation, especially as AI models are increasingly used for applications that demand high accuracy, like critical information dissemination and legal documents.
The evolution of AI translation technologies has been closely intertwined with efforts to address the needs of languages that have historically lacked adequate translation resources, often referred to as "low-resource languages". The ability to translate "low-resource" languages presents tremendous possibilities for global communication and fostering equitable access to information for underrepresented communities. However, challenges remain in ensuring the accuracy and cultural sensitivity of translations, particularly in addressing regional dialects or culturally specific language usages. This work highlights how collaborative efforts and insights from communities are key to developing models that respect and capture the complexities of these languages.
The democratization of translation technologies, driven by SMT and NMT, has reshaped how businesses interact with a globalized marketplace. Cost-effective translation services have made it possible for organizations of all sizes to access AI-powered multilingual support, expanding their reach and responsiveness to a diverse global audience. Despite the positive impact, the need to mitigate bias in translation models remains a critical concern. Ensuring AI translation systems adequately capture cultural nuances, particularly in situations with low-resource languages, is paramount to generating accurate and contextually appropriate translations. It requires close cooperation with native speakers and experts to ensure linguistic and cultural subtleties are preserved.
Looking at the evolution of machine translation, from its early days in the 1990s to today's sophisticated AI-powered systems, illustrates a broader pattern. Machine learning advancements are shifting the emphasis in translation away from rote language memorization and towards a deeper understanding of context and cultural relevance. This evolution underscores the continuous need to balance the potential of technology with a deep understanding of how people communicate across diverse cultures. The future of machine translation likely will continue to improve, yet this improvement must be coupled with ongoing evaluation and attention to promoting accessibility, accuracy, and cultural sensitivity.
AI Translation in Chaos Leveraging Unconventional Methods for Rapid Language Processing - CUBBITT System Challenges Human Translation Quality
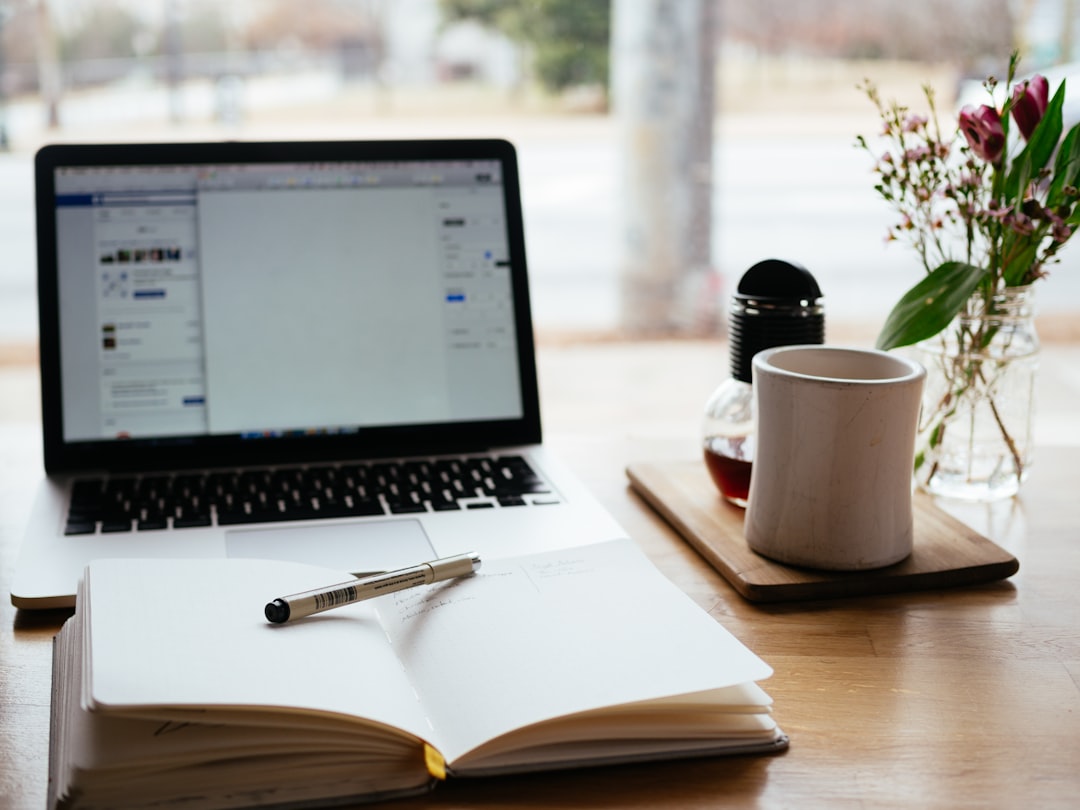
The CUBBITT system represents a significant step forward in AI translation, directly challenging the long-held belief that human translators are irreplaceable in achieving high quality. This system, employing a deep learning approach based on the Transformer architecture, has demonstrated a remarkable ability to match or even surpass human translation quality in specific language pairs, such as English to Czech. Its proficiency in preserving meaning, referred to as "adequacy," particularly stands out. The fact that it has also been proven effective across different languages, including English to French and Polish, suggests versatility.
The CUBBITT system’s emergence is a powerful illustration of how AI is pushing the boundaries of language processing. It raises questions about the very nature of translation and whether humans will remain the sole arbiters of linguistic precision. Furthermore, its open-source nature for non-commercial use shows an ambition to provide wide access to potentially high-quality translation technology.
While CUBBITT’s capabilities are undeniably impressive, the larger landscape of AI translation requires continuous evaluation. There's a need to consider potential issues like biases embedded in training data and the capacity for AI models to accurately capture the nuances of various cultures and languages. The future of AI-driven translation will undoubtedly involve both further technical innovation and a thoughtful exploration of the social implications of increasingly advanced machine translation capabilities.
CUBBITT, a deep learning system, has emerged as a significant challenger to the long-held belief that human translation quality is a benchmark machine translation can't reach. It's particularly notable for achieving superior results in English-to-Czech news translation when compared to professional human translators in a blind evaluation, showcasing its capabilities in maintaining context. The system's success isn't limited to Czech, as it's also been validated for French and Polish, suggesting a broader application across different languages. Where CUBBITT truly excels is in accurately capturing the core meaning of text, exceeding human translators in terms of adequacy. This is achieved through a machine learning program built on the Transformer encoder architecture.
One of CUBBITT's appealing features is its free availability for non-commercial use, making high-quality translation accessible to a wider audience. This research demonstrates that sophisticated machine learning models can achieve, or even surpass, human-level translation quality, forcing us to re-evaluate our assumptions about the limitations of computer translation. CUBBITT's achievements are a major leap forward in machine translation technology, suggesting we are getting closer to a future where human-quality translation is within reach. The development of systems like CUBBITT implies a potential shift in the future of language processing, introducing less conventional approaches to quickly and effectively handle translation tasks.
However, challenges remain. While CUBBITT offers a compelling alternative to traditional translation methods, its training data and the distributed architecture it relies on could introduce unforeseen biases into the translations or create security vulnerabilities. Despite this potential, it's evident that CUBBITT highlights how AI systems, utilizing unorthodox methods, are pushing boundaries and significantly contributing to language processing capabilities.
AI Translation in Chaos Leveraging Unconventional Methods for Rapid Language Processing - AI and NLP Reshape Language Translation Industry
AI and NLP are reshaping the language translation industry, bringing both exciting possibilities and persistent challenges. AI-powered translation systems have significantly improved translation speed and access, particularly for languages previously lacking readily available tools. This push towards "low-resource" languages aims to democratize translation, but it highlights the inherent limitations of AI in fully capturing cultural context and subtle linguistic nuances that human translators naturally grasp. While the advancements in neural machine translation are impressive, achieving consistent accuracy, especially across regional dialects and less common languages, remains a hurdle. Furthermore, the potential for bias embedded within training data necessitates ongoing vigilance to ensure that these powerful new technologies don't perpetuate existing inequalities. The future of AI-driven translation depends on striking a balance between leveraging these innovative technologies and addressing these challenges to create truly inclusive and accurate global communication.
AI's impact on language translation is undeniable, especially with the recent advancements in natural language processing (NLP). We're seeing increasingly accurate real-time translations, particularly useful in applications like video calls and customer service, where swift understanding is key. However, achieving truly fluent and accurate results, especially in casual conversation, remains a challenge.
One of the most noticeable impacts is the cost reduction in translation services. AI-powered translation is significantly cheaper than traditional methods, making it a boon for smaller businesses hoping to connect globally. This shift towards affordability has undeniably democratized access to translation tools.
Preserving endangered languages has also become a focus. The use of OCR in tandem with AI is helping digitize and translate documents written in languages with little digital presence, effectively archiving valuable linguistic resources. It's fascinating to see how a technology developed for entirely different purposes can become integral to preserving a language's existence.
The field of transfer learning has experienced some major improvements recently, enabling AI models to effectively bridge the gap between high-resource languages, like English, and low-resource languages, which might have scant data available for translation. The hope is to enable translation services that serve communities that historically lacked readily available tools.
Modern AI translation models aren't just focused on simple word-for-word replacements. Researchers are working to enhance the understanding of context, idioms, and cultural references. This is a huge step forward towards nuanced and more accurate translations, particularly when accuracy can have significant consequences, such as in sensitive communications.
The sheer volume of public datasets has become a massive driving force in training these AI models. Often originating from government and educational sources, these datasets help refine and broaden a model's abilities, better equipping it to handle the unique nuances of various dialects and language structures.
Despite the progress, AI translation models still struggle with certain language pairs. This is often tied to significant structural or cultural differences. English and Mandarin, for example, have historically been challenging to translate with high accuracy. These linguistic barriers can produce some odd results, requiring human input to ensure the correct message is conveyed.
We're starting to see a new kind of role emerge: AI-driven post-editing. Human translators are now leveraging AI-generated translations as a starting point, refining them and ensuring accurate communication. This collaboration combines speed with accuracy, allowing a translator to focus on the intricate aspects of language and culture that are still difficult for AI to grasp.
The elephant in the room remains the inherent bias in training datasets. Research efforts are ongoing to minimize the impact of these biases. But the potential for these datasets to favor specific dialects, cultures, or perspectives is a serious concern. It's a complex issue, and continuous scrutiny is needed as AI translation models evolve.
The application of AI-powered translation is broadening into specialized areas such as medical and legal contexts. These are environments where accuracy and clarity are vital. This shift highlights the need for specialized vocabulary and the development of models specifically tailored for those particular fields. This illustrates that there's no single "one size fits all" approach to translation.
The trajectory of AI translation is an exciting area of research. There's a clear pattern of increasing capability, but it's also clear that it's a journey that will require ongoing attention to bias, accuracy, and cultural nuance to truly serve its intended purpose.
AI Translation in Chaos Leveraging Unconventional Methods for Rapid Language Processing - Unconventional AI Methods Complement Traditional Techniques
AI translation is increasingly incorporating unconventional methods to supplement traditional techniques, leading to faster and more accessible translations. Deep learning and transfer learning are enabling AI models to better address languages with limited existing translation tools, particularly those considered "low-resource." These languages, often overlooked by traditional methods, are now gaining access to translation services thanks to these newer AI techniques. Furthermore, the integration of OCR technologies empowers translation by expanding the reach of machine translation to previously inaccessible text in images and documents.
Despite the impressive gains, the rapid advancement of AI in translation necessitates a cautious approach. Concerns surrounding potential bias in the training data used by these models need careful consideration. Ensuring culturally appropriate and accurate translations across diverse language groups, particularly those with unique dialects, remains a significant challenge. The future direction of AI in translation hinges on the continuous evaluation and refinement of these methods, balancing innovation with ethical considerations to create more inclusive and effective global communication tools.
The field of AI translation has seen a significant shift towards unconventional methods, complementing traditional techniques. One exciting development is the rapid improvement of OCR. This technology is now quite adept at extracting text from all sorts of complex documents—old books, signs, and even historical manuscripts in multiple languages. This capability opens up a wealth of previously inaccessible information to translation and analysis, which could be extremely valuable for linguistic studies and historical research.
We're also seeing a push towards instant translation. Many AI systems are now capable of real-time translation, effectively making multi-language conversations commonplace. This is a huge advantage for businesses working globally and for diplomacy, where quick comprehension is paramount. However, the need for truly fluent and natural conversation remains an area of ongoing development.
This emphasis on speed and accessibility has also significantly reduced costs. AI-driven translation is considerably cheaper than traditional methods, sometimes by as much as 80 percent. This makes high-quality translation more attainable for smaller businesses and individuals who may not have had the resources previously.
For less commonly spoken languages, a fascinating technique called "transfer learning" is playing an increasingly important role. AI systems trained on more common languages are being adapted to translate these "low-resource" languages, making use of the knowledge gained from the larger datasets to improve accuracy and contextual understanding. It's a clever way to address the disparities in available training data, but the unique grammatical structures and vocabulary of certain languages can still pose challenges.
Models are also becoming more sensitive to cultural context. They are increasingly able to analyze surrounding phrases and idiomatic expressions, providing translations that convey the intended meaning rather than a literal, often awkward interpretation. This is essential for ensuring that translations don't just get the words right, but also the meaning and nuances within the communication.
We are also starting to see AI translations incorporated into more and more digital content, especially video and audio formats. Systems are being developed to create synchronized translations across multiple languages in live broadcasts and pre-recorded videos. This expands content accessibility and could lead to exciting advancements in both entertainment and education.
The CUBBITT system has been a particularly noteworthy development, as it is openly available for non-commercial uses and provides a benchmark for machine translation. Its performance in tests against human translators, especially in languages like Czech, has been impressive in some situations, raising questions about the long-term role of human translators. Of course, further research is needed to explore its broader potential and address any potential biases in its training data.
An increasing emphasis on accurately capturing dialectal variations is also vital. AI models are being specially adapted to account for the intricacies of regional dialects, which can differ considerably from standard forms of a language. This ensures that translations respect local language identities and nuances. This attention to detail is vital for avoiding misunderstandings or inadvertently reinforcing stereotypes.
As we move forward, researchers are developing increasingly sophisticated ways to measure the quality of translations. Metrics like BLEU scores, along with other linguistic assessments, offer more accurate ways to gauge the success of translation systems and identify areas for improvement.
Finally, the ethical implications of using proprietary data for training AI models are becoming a major point of discussion. Balancing innovation and protecting intellectual property or respecting user privacy is critical. This is an area that warrants careful scrutiny as AI translation continues to evolve.
The development of AI-driven translation has been a remarkable journey. We're seeing a blend of established and novel techniques. While the technology is showing great promise, ongoing research, mindful consideration of ethical issues, and open evaluation are all crucial to ensuring that AI translation can fully realize its potential to improve cross-cultural understanding and communication.
AI-Powered PDF Translation now with improved handling of scanned contents, handwriting, charts, diagrams, tables and drawings. Fast, Cheap, and Accurate! (Get started for free)
More Posts from aitranslations.io: