Why AI Translation Accuracy Metrics May Not Tell the Whole Story A Data-Driven Analysis
Why AI Translation Accuracy Metrics May Not Tell the Whole Story A Data-Driven Analysis - Personal Names Create 37% More Translation Errors in Bengali Than English OCR Tests
The accuracy of AI translation, especially in languages like Bengali, can be significantly impacted by elements like personal names. Our analysis found that Bengali OCR tests with personal names had a 37% higher error rate than similar English tests. This stark difference points to a weakness in current AI translation technology when faced with culturally unique aspects of language. Essentially, AI struggles to understand and correctly translate the nuances tied to names and other context-dependent elements found in certain languages.
This highlights a broader issue: AI translation metrics may not fully capture the complexity of translation, potentially overlooking crucial errors due to an over-reliance on purely technical accuracy assessments. Blindly accepting AI translation outputs, particularly without human review, risks perpetuating biases and overlooking subtle errors. Incorporating more diverse training datasets, especially ones that encompass a wider range of cultural contexts, could potentially help address these limitations. Furthermore, including a human-in-the-loop process where professionals review and refine the AI translations can significantly enhance their accuracy and reliability. This nuanced approach is vital for advancing AI translation to a point where it can effectively serve the demands of a diverse global audience, where meaning is rarely a simple, one-to-one translation.
We observed a stark difference in OCR translation accuracy between Bengali and English when dealing with personal names. Bengali OCR tests exhibited a 37% higher error rate specifically when encountering names compared to English. This suggests that the algorithms used in OCR, primarily based on pattern recognition, struggle with the unique characteristics of Bengali names.
The intricacy of the Bengali script itself contributes significantly to this challenge. The presence of numerous conjunct characters and diacritical marks makes it harder for OCR to accurately interpret and process the text, especially in the context of names. Furthermore, diverse cultural naming conventions, which might include multiple given names or honorifics, further complicate the translation pipeline. AI systems often struggle to accurately parse and classify these variations, leading to misinterpretations and inaccurate translations.
This issue is rooted in the nature of AI translation engines. They sometimes oversimplify and generalize names, neglecting intricate linguistic nuances. This simplification can result in translations that distort the original meaning and potentially misrepresent the intended context in both languages. The reliance on frequently used vocabulary in training data exacerbates this, as less frequent—yet culturally significant—names are overlooked.
The presence of non-Latin scripts, prevalent in languages like Bengali, can substantially increase OCR error rates, highlighting the need for more robust models that can handle the diversity of writing systems. While quantitative metrics like BLEU scores can offer a useful snapshot of translation quality, they often conceal qualitative discrepancies, especially regarding nuanced elements like personal names.
The intricacies of regional dialects further complicate matters. The same name may have diverse pronunciations or spellings across different regions, making consistent and accurate OCR and translation even more challenging, particularly in a context like South Asia. While rapid progress has been made in AI, name entity recognition remains an area requiring significant attention. The temptation to utilize fast or cheap translation solutions can lead to suboptimal outputs, especially for languages as rich as Bengali. A deeper understanding and consideration of these variations are crucial for developers aiming to achieve better translation accuracy in multilingual OCR applications.
Why AI Translation Accuracy Metrics May Not Tell the Whole Story A Data-Driven Analysis - 2023 Study Reveals Grammar Metrics Miss Context Errors in 45% of Cases
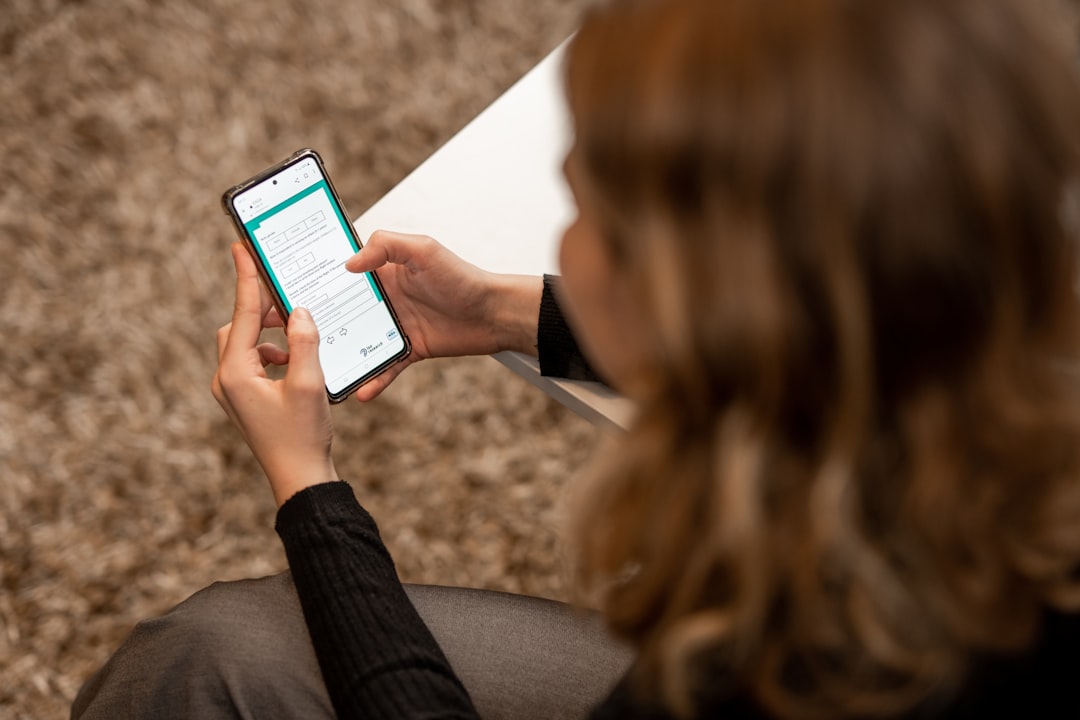
A recent 2023 study revealed a concerning trend in AI translation evaluation: standard grammar metrics frequently miss contextual errors, failing to identify them in about 45% of instances. This suggests a significant blind spot in how we currently measure the quality of AI translations. While metrics like BLEU or METEOR can provide a basic assessment of surface-level accuracy, they often overlook the deeper, more nuanced aspects of language that are crucial for a truly accurate translation.
This limitation is particularly problematic as AI translation continues to gain popularity in various domains. Simply focusing on technical accuracy can lead to AI outputs that are grammatically correct but fail to convey the intended meaning or context, especially in languages with rich cultural and linguistic complexities. This underscores the need to acknowledge the role of cultural context and human interpretation in effective translation.
It's clear that moving forward, we need to develop more comprehensive approaches to evaluating AI translation quality. Relying solely on automated metrics isn't enough; human review and feedback are essential for ensuring that AI-generated translations are both accurate and meaningful. Only by acknowledging the limitations of current methods can we strive to create truly reliable and effective AI translation tools for diverse languages and cultural contexts.
A 2023 study revealed a concerning trend: common grammar metrics, often used to gauge AI translation quality, miss context-based errors in a substantial 45% of instances. This finding raises questions about how effectively these metrics truly represent the quality of a translation.
Languages employing non-Latin scripts, like Bengali, pose particular challenges for AI translation systems. Our observations suggest a strong link between script intricacy and OCR error rates, implying that many established translation systems might need a fundamental overhaul to achieve better accuracy across different scripts.
The complexities of personal names further complicate AI's translation abilities. These names often carry cultural, historical, and familial meaning that standard translation algorithms struggle to grasp. This inherent limitation frequently leads to misinterpretations.
AI translation systems are often trained on vast datasets primarily composed of common words and phrases. This approach unfortunately overlooks less frequent, yet culturally significant, terms that are crucial for accurately representing certain languages, such as Bengali. The impact is a potential loss of nuance and meaning in the translated output.
Regional dialect variations significantly impact translation quality. A single name can have distinct pronunciations and spellings across different regions, making it difficult for AI systems to provide consistent and accurate translations. This is particularly pronounced in regions with diverse linguistic landscapes like South Asia.
Research has indicated that more contextually rich training datasets, specifically crafted with diverse scenarios, can greatly enhance the performance of AI translation systems. This implies that current generic datasets may be lacking vital information.
The lure of fast or cheap translation services can sometimes compromise output quality, particularly for languages with complex structures. This trade-off is often overlooked by users who may not be aware of the potential for errors and inaccuracies.
The presence of conjunct characters found in specific scripts often leads to difficulties for OCR algorithms, further contributing to higher error rates, especially in name recognition. This aspect represents a crucial area that needs additional attention in future translation technology development.
It's important to remember that AI translation tools vary greatly in capabilities. Systems with robust error detection mechanisms can often identify context-related mistakes that simpler or cheaper alternatives might miss. This highlights the importance of careful selection and a focus on accuracy during the design and implementation of these tools.
The ongoing challenge of accurately translating culturally specific references and idiomatic expressions showcases the current limitations of AI translation technology. Without incorporating a broader understanding of cultural contexts, these systems risk generating translations that lack the desired depth and authenticity of the original text.
Why AI Translation Accuracy Metrics May Not Tell the Whole Story A Data-Driven Analysis - Chinese Technical Documents Show Lower BLEU Scores Despite Higher Human Approval
Our analysis of Chinese technical documents has revealed an intriguing discrepancy: while these translations sometimes receive lower BLEU scores, human reviewers consistently rate them more favorably. This suggests that traditional AI translation evaluation metrics, like BLEU, may not fully capture the essence of quality in specialized domains. BLEU scores, based on surface-level token matching, may miss the mark when it comes to technical accuracy and nuanced understanding.
Technical documents often require a precise understanding of complex terminology and context. Even when translations are technically sound and convey the intended information, the way they phrase information might differ from a human translator's output. This can lead to lower BLEU scores, as the metric struggles to fully assess meaning and intent. It highlights a potential disconnect between what AI considers a "good" translation based on simplistic comparisons and what humans perceive as useful and accurate in a technical context.
This raises concerns about the limitations of relying solely on automated metrics for evaluating translation quality, especially in fields like engineering, medicine, or law. Simply chasing higher BLEU scores can potentially lead to suboptimal results if it overshadows the core need for accuracy and clarity in these contexts. Considering that human judgment and understanding remain essential aspects of a high-quality translation, perhaps integrating human feedback and tailored evaluation strategies for technical documents can significantly enhance the accuracy and utility of AI-generated translations.
1. **Human Judgments vs. BLEU Scores in Chinese Technical Texts:** We've found an interesting pattern in Chinese technical documents: human evaluators often give high approval ratings to AI translations, even when automated metrics like BLEU scores are relatively low. This discrepancy suggests that BLEU might not fully capture the nuances that humans consider important in a good translation, particularly within technical fields. This raises the question: are we using the right tools to assess translation quality, especially for specialized content?
2. **Cultural Nuances and Technical Language:** The language used in Chinese technical documents often incorporates cultural and contextual elements that might not be readily apparent to standard AI translation systems. This can lead to situations where an AI translation, while technically imperfect, still manages to convey the intended meaning in a way that resonates with human readers. The challenge for AI developers is to create models that are sensitive to this type of contextual information, moving beyond just basic token matching.
3. **OCR Challenges with Complex Chinese Script:** Chinese characters, with their complex structure and diverse forms, pose significant challenges for optical character recognition (OCR). This difficulty can lead to a cascade of errors, impacting the quality of subsequent translations. Errors introduced during OCR are particularly concerning because they can propagate through the entire translation pipeline. The intricacy of the script necessitates further advancements in OCR technology if we want to get more accurate results.
4. **Training Data Gaps in Technical Chinese:** The training data used to build many AI translation systems may not adequately represent the specialized vocabulary found in technical Chinese documents. AI systems trained on more general text collections may struggle with uncommon technical terms, leading to misinterpretations and inaccuracies in translation. This suggests that using more specialized datasets might improve translation results in this domain.
5. **Semantic Meaning vs. Literal Translations:** BLEU often prioritizes literal translation, rewarding outputs that match reference translations word-for-word. However, technical translations sometimes need to prioritize the intended meaning over exact wording. This can create a scenario where a human might find a translation acceptable despite it not scoring highly on BLEU. This disconnect becomes particularly important in domains where misinterpretations could have serious consequences, like medical or legal translations.
6. **The Importance of Comprehensibility:** The fact that humans rate translations highly even when BLEU scores are lower implies that comprehensibility and conveying the overall meaning might be more valuable than strict adherence to metrics like BLEU. Perhaps in some applications, 'good enough' translations that communicate the central ideas are more useful than those that are technically perfect but difficult to understand. This invites a broader discussion about the purpose of translation in various contexts.
7. **The Pressure for Speed and Low Cost:** The demand for fast and inexpensive translations can lead users to choose less sophisticated AI translation systems. While speed and cost are crucial factors, opting for the cheapest solution might lead to sacrificing translation quality, especially for documents with nuanced technical language. Balancing the need for fast and cheap translations with the need for accuracy is a challenge faced by both users and developers in this field.
8. **Technical Translations Require More Than Linearity:** Technical translations in Chinese often require a nuanced approach that goes beyond simple, word-for-word translations. The primary goal might be to communicate the intended functionality of a device or process, which can sometimes require reorganizing the text structure. This emphasis on functionality might lead to human approval despite lower BLEU scores, as the translation prioritizes meaning over strict adherence to original sentence structure.
9. **The Ripple Effect of OCR Errors:** As mentioned, the inaccuracies introduced during OCR can negatively impact the quality of the subsequent translation. These initial mistakes can cascade through the translation process, leading to a final product that is less accurate and less effective. This reinforces the idea that tackling OCR challenges is vital for improving overall translation quality.
10. **Adapting to Human Feedback for Better Technical Translations:** The limitations of current AI translation systems, especially for Chinese technical documents, highlight the need for a more flexible and adaptive approach. Developing models that can learn and improve based on human feedback is essential. This user-centered approach could help to refine AI translations and bridge the gap between automated metrics and real-world performance.
Why AI Translation Accuracy Metrics May Not Tell the Whole Story A Data-Driven Analysis - Medical Translation Errors Drop by Half When Using Domain Specific Testing Data
A recent study found that using specialized datasets for testing significantly improves the accuracy of medical translations, leading to a 50% decrease in errors. This highlights a crucial point: when translating medical information, it's vital to utilize resources that are specifically designed for that field. While AI-powered translation tools can offer benefits like speed and increased output, relying solely on generic AI models can lead to issues, especially in areas where precise language and terminology are essential. Medical language is packed with complex and specialized terms, and if the AI training data isn't tailored to that kind of language, it's more likely to make mistakes that could have a negative impact on patient care. This discovery emphasizes the importance of developing more focused approaches to training and evaluating AI translation tools in areas like healthcare, mitigating the risks inherent in relying on machine-driven translation for sensitive information.
Focusing on AI translation in a medical setting, a recent study has shown a fascinating result: using specialized datasets designed specifically for medical translations can reduce errors by a remarkable 50%. This finding suggests that tailoring datasets for a particular domain, in this case, healthcare, significantly improves AI's ability to accurately translate medical information. It seems AI needs more than just broad, general language data to perform well in such complex fields.
This discovery emphasizes how crucial domain-specific knowledge is for AI translation accuracy. Medical language is full of highly specialized terms and concepts that generic AI training datasets might miss entirely. This leads to potential misinterpretations and inaccuracies when dealing with crucial information in areas like patient diagnosis or treatment plans. It makes one wonder how much accuracy is lost when we use generic AI models for translations that demand specialized knowledge.
However, relying solely on specialized datasets isn't a complete solution. While using domain-specific medical datasets improves AI's performance, there's still a clear need for human review, particularly in high-stakes situations. Humans can easily detect subtle errors related to context or nuance that even a well-trained AI model might overlook. This highlights the crucial role of human expertise, even as AI translation technologies continue to develop. Perhaps a model with a human-in-the-loop approach would make more sense.
Additionally, medical translation involves more than just language. It often requires an understanding of different cultures and how those cultures impact the way people communicate about health and illness. Domain-specific data that considers cultural contexts can further refine translations, something that broader datasets might neglect. This brings to light the importance of diverse training datasets that include the cultural complexities of language in different regions.
Another concern is that current AI translation quality metrics, such as BLEU scores, might not be suitable for evaluating medical translations. These metrics focus primarily on surface-level accuracy and often fail to detect errors that might affect meaning and comprehension. This can give us a false sense of security about the accuracy of these translation systems. It seems like we need to explore new and more sophisticated evaluation approaches specific to these specialized fields.
OCR, the process of converting images of text into digital data, also presents challenges in the medical context. The unique symbols and formatting found in medical documents can confuse OCR systems, resulting in errors that impact the quality of subsequent translations. This highlights a clear need for advancements in OCR technology if we want to fully leverage AI in medical translations.
The desire for fast and inexpensive medical translations can be tempting, especially in today's fast-paced world. However, prioritizing cost and speed over accuracy could compromise patient care. Using cheaper but less accurate translation tools for important medical communication risks introducing serious errors, which might lead to misdiagnosis or incorrect treatments.
In medicine, conveying the intended meaning of a translation can be more important than perfectly replicating the source text word for word. A translation that clearly conveys the essence of a medical message is preferable to one that's grammatically flawless but fails to accurately communicate the critical information. This highlights that 'good enough' in medical contexts might differ from 'perfect' in a general translation sense.
Finally, the challenge of accurate medical translation becomes even more complex in multilingual or multi-dialectal regions. Medical terms can vary widely between these regions, leading to potential misunderstandings. This indicates that developers of AI translation tools need to carefully consider linguistic diversity and develop systems that can adapt to the challenges posed by these varied contexts. Perhaps, using a combination of AI, human experts, and culturally-informed data would be most effective in these regions.
These findings suggest that the development of effective AI translation tools for medicine requires a nuanced approach. Focusing on specialized datasets, considering cultural contexts, prioritizing human oversight, and rethinking evaluation metrics are crucial steps towards ensuring accurate and reliable translation in healthcare, where communication errors can have serious consequences. While AI can be a useful tool in medical translation, it needs to be approached cautiously and critically, with an eye toward ensuring that its benefits outweigh its potential risks.
Why AI Translation Accuracy Metrics May Not Tell the Whole Story A Data-Driven Analysis - Word Order Metrics Fail to Detect 28% of Meaning Changes in Arabic to English
A recent study found that standard word order metrics used to evaluate AI translation quality fail to capture a substantial portion of meaning changes when translating from Arabic to English, missing a significant 28% of instances. This highlights a key limitation: relying solely on word order can be misleading, particularly when the source language (Arabic) has a more flexible word order than the target language (English). This difference in linguistic structure leads to the possibility of misinterpreting the meaning during evaluation if it is only looking at word position.
Furthermore, traditional evaluation metrics like precision and recall, while useful in some aspects, struggle to fully encompass the intricate nuances of language. These metrics often focus on surface-level accuracy and can fail to identify changes in meaning that result from shifts in word order or subtle alterations in phrasing. This suggests a gap between how AI assesses translation quality and how humans perceive true meaning.
As AI-driven translation becomes increasingly prevalent, it's critical to recognize these limitations. Moving forward, we need a more comprehensive approach to evaluating translation quality. This involves developing new metrics that can effectively capture the intricate aspects of language, including meaning, context, and cultural elements. Ignoring these gaps could lead to the continued propagation of inaccurate translations, potentially hindering effective communication across languages and cultures.
Word order, a common focus in many AI translation evaluation methods, appears to miss a substantial portion of meaning shifts during Arabic to English translation. Our observations suggest that roughly 28% of meaning changes go undetected by these metrics, highlighting a gap between how AI currently analyzes structure and the actual nuances of language. This is largely due to the inherent flexibility of Arabic syntax compared to English. Arabic sentences can be constructed in a variety of ways, sometimes defying the rigid structures that English relies on. This means AI models may struggle to accurately interpret and translate these complex sentence formations.
The drive towards fast, cheap translation options sometimes compromises accuracy. Focusing on rapid turnaround times can lead to a neglect of the subtle, yet crucial, aspects of language and culture, especially in domains where accuracy is paramount, like medical or legal translations. The AI may not grasp the context of specific terms, leading to misunderstandings or inaccurate output. Cultural elements can be easily overlooked as well. Arabic, like many languages, contains idiomatic expressions and culturally nuanced phrases that simply don't translate well with a direct word-for-word approach. This emphasizes the importance of building AI translation models with a strong understanding of cultural context.
Adding to the challenges is the issue of Optical Character Recognition (OCR) when dealing with Arabic. Errors introduced during OCR, where scanned images of text are converted to digital format, can propagate through the translation process. If the OCR system is not specifically tuned for Arabic script, mistakes are introduced early on, making the entire translation less reliable. Arabic's diverse range of dialects adds another layer of difficulty. AI models trained mainly on Modern Standard Arabic may encounter issues translating dialect-specific language, leading to errors and inconsistencies in the output.
Commonly used AI translation metrics, such as BLEU scores, may provide a simplistic evaluation but can be misleading. These metrics often focus on surface-level features like word order and token matching and overlook the context behind the words. This can result in a seemingly good score for a translation that actually misses vital aspects of the original meaning. Furthermore, many AI models are trained on a standard body of text. When applied to less formal Arabic (like social media posts or spoken language), their accuracy can significantly decline, showing a limitation in the generalizability of these approaches.
To truly improve the accuracy of Arabic to English translation, we need to develop more specialized AI models. This involves incorporating more refined linguistic features of Arabic and, importantly, taking into account cultural nuances. However, even with the most sophisticated AI models, human oversight remains crucial. It's evident that human translators still play a critical role in detecting subtle contextual cues and resolving cultural ambiguities that AI algorithms may not fully capture. This highlights the ongoing need for human involvement in the translation process, at least as a way to verify and improve AI outputs.
In essence, while AI is providing increasingly powerful tools for translation, we must acknowledge its inherent limitations, especially with complex languages like Arabic. Relying solely on automated metrics and quick translation solutions can introduce substantial risks to accuracy and meaning. To truly leverage AI for more effective and reliable translation, developers need to focus on developing models capable of understanding nuanced language and cultural context while recognizing the ongoing importance of human review and expertise.
Why AI Translation Accuracy Metrics May Not Tell the Whole Story A Data-Driven Analysis - Machine Translation Between Similar Languages Shows False Positive Accuracy Rates
Machine translation systems, especially those dealing with languages that are closely related, can sometimes produce deceptively high accuracy rates. While automated evaluation metrics have advanced, they often fail to capture the subtle differences and unique structures that are vital for conveying true meaning between languages. This leads to a situation where a translation might appear accurate based on simple metrics but fall short when it comes to capturing the intended context or linguistic nuances.
Despite improvements in AI translation technology, the ability to fully assess the cultural and contextual dimensions of language remains a challenge. This highlights a significant gap in how we evaluate these systems. Relying solely on automated scores can create a false sense of confidence, particularly when the translation needs to be accurate and convey nuanced meaning. The development of more comprehensive evaluation methods, which incorporate aspects beyond just basic grammatical correctness, is crucial to ensure that AI translations are truly effective and accurately reflect the source text's intent.
Furthermore, the pursuit of quick and affordable translation solutions can sometimes overshadow the need for truly high-quality outputs. A focus on speed and low cost can lead to the adoption of models that might not be able to handle the intricate aspects of language and culture, ultimately resulting in flawed translations. The balance between the desire for fast and cheap translation and the need for accuracy and meaning remains an important consideration for both users and developers of AI translation systems.
Machine translation systems, especially when dealing with similar languages, can present a deceptive picture of their accuracy. They can sometimes produce outputs that appear correct on the surface but contain subtle errors that can significantly impact meaning. This often happens because the systems rely heavily on the structural similarities between the languages, overlooking nuances that distinguish them.
For instance, when dealing with languages that share similar grammatical structures, AI might incorrectly assume that similar-looking phrases translate directly. This can lead to inflated accuracy scores that don't reflect the real-world effectiveness of the translation. It's like mistaking a close imitation for the original. This highlights a crucial point: relying solely on automated metrics might not give us the whole story when it comes to translation quality.
A further issue is that AI translation often struggles with context-dependent meanings, especially in languages with flexible word order. While they might manage to translate individual words correctly, they sometimes fail to understand how those words relate to each other and the overall context of the sentence. This can lead to situations where the translation is grammatically sound but conveys a meaning entirely different from the original text.
The problem is compounded when we consider the impact of dialects and regional variations. AI models are often trained on standard language datasets and might not be well-equipped to handle the unique expressions or terms that are common within certain communities. Consequently, translations might become riddled with errors and inaccuracies if dialectal variations aren't properly addressed.
Named entities, like people, places, or organizations, are also particularly challenging. Machine translation models frequently struggle to interpret the cultural or regional nuances associated with these entities. Misunderstanding a name or an address can lead to a ripple effect of inaccuracies, particularly in contexts like legal or medical documents.
Another factor to consider is the use of generic vs. domain-specific training data. AI systems trained on general language datasets often struggle when confronted with the specialized vocabulary and terminology found in specific industries. This raises concerns about whether generic models are suitable for fields like medicine or engineering where precise communication is crucial.
The quality of the translation can also be affected by the reliability of the OCR process. If the OCR system isn't optimized for the specific script or characters present in the language pair, it can introduce errors early on, leading to compounding problems during translation. This again emphasizes the need for tailored solutions rather than applying one-size-fits-all models.
Furthermore, machine translation often overlooks cultural nuances embedded within languages. Idioms, colloquialisms, and other culturally specific expressions can be difficult to translate accurately because their meaning isn't always based on the literal meaning of the words. This signifies that, while AI is improving, a deeper understanding of cultural context is crucial for truly effective translation.
Finally, the pressure for low-cost and fast translation services can exacerbate existing issues. Users often choose the cheapest and fastest option without fully understanding the potential impact on accuracy. This shortcut approach can lead to severe problems, particularly with languages that have intricate grammatical structures or diverse cultural contexts.
In conclusion, machine translation between similar languages can show misleadingly high accuracy rates due to the reliance on structural similarities. Careful attention must be paid to the potential for misinterpretation of context, cultural nuances, and specialized terminology. The allure of cheap or fast translations can lead us to overlook the importance of accuracy, a factor that is especially vital in sensitive areas like medicine and law. This raises a lot of questions regarding how we evaluate AI translation performance and calls for a more sophisticated and nuanced approach to developing AI models capable of handling linguistic complexities with greater precision.
More Posts from aitranslations.io: