AI-Powered PDF Translation now with improved handling of scanned contents, handwriting, charts, diagrams, tables and drawings. Fast, Cheap, and Accurate! (Get started for free)
AI Translation Meets Classical Greek How Smyth's Grammar Framework Enhances Modern Language Processing
AI Translation Meets Classical Greek How Smyth's Grammar Framework Enhances Modern Language Processing - Machine Learning Models Now Parse Ancient Greek Syntax in 3 Seconds
Machine learning's progress has enabled remarkably fast analysis of Ancient Greek grammar, achieving parsing in just three seconds. This breakthrough significantly speeds up linguistic research. The development of these models, often a joint effort between academics and AI developers, doesn't just expedite the process but also offers novel ways to piece together fragments of old Greek inscriptions. Historians can now potentially gain a clearer picture of lost historical details by using AI to analyze damaged or incomplete inscriptions. The models can also suggest possible dates and locations for these ancient writings, providing crucial context. This fusion of AI and humanities offers a powerful new tool for understanding ancient languages, demonstrating how technology can propel research and understanding of complex historical accounts. While the potential benefits are significant, we must acknowledge that these tools are still nascent, and ongoing research is essential to refining their abilities.
Modern machine learning, fueled by vast digitized collections of classical texts, now swiftly deciphers the intricate syntax of Ancient Greek within a mere three seconds. This rapid pace is remarkable, but we're still investigating the limits of these models on truly complex linguistic structures.
The adoption of frameworks like Smyth's Grammar has undoubtedly helped in this progress. Structuring the language in a way machines can readily learn improves the quality and speed of parsing. However, machine learning models continue to struggle with highly nuanced areas of the language, particularly the subtle shades of meaning embedded in ancient idioms.
There's also an increasing reliance on OCR to handle the digital transformation of the historical record. The ability to convert handwritten manuscripts into machine-readable text has unlocked a trove of ancient texts, creating rich training data for algorithms.
In a way, Ancient Greek has become an interesting testing ground for pushing the boundaries of natural language processing, especially for languages with limited readily available digital resources. While we're getting a more comprehensive understanding of its syntax, the challenge of producing accurate translations for all aspects of the language persists, highlighting the complexities inherent in translation, even with AI.
The combined power of syntactic and morphological analysis seems to reduce ambiguities prevalent in Ancient Greek, which is essential for machine translation. We are slowly seeing how these insights from AI can possibly extend into other low-resource languages.
The community efforts for creating large annotated datasets have been essential in pushing this technology forward. This development is gradually enabling us to analyze the linguistic structure and historical shifts in Ancient Greek. As we better understand how these models function, we may also be able to better understand language evolution itself, and what influences cause changes in a language over time.
While still in its early stages, the promise of this technology is exciting for language enthusiasts and scholars. For educational purposes, in particular, there is the potential to drastically reduce the hurdles to accessing and comprehending this fascinating historical language. It could dramatically decrease the cost and time involved in translating and understanding the texts, making classical studies more accessible.
AI Translation Meets Classical Greek How Smyth's Grammar Framework Enhances Modern Language Processing - Digital Papyrus Scanner Combines OCR with Grammatical Rules From 1920
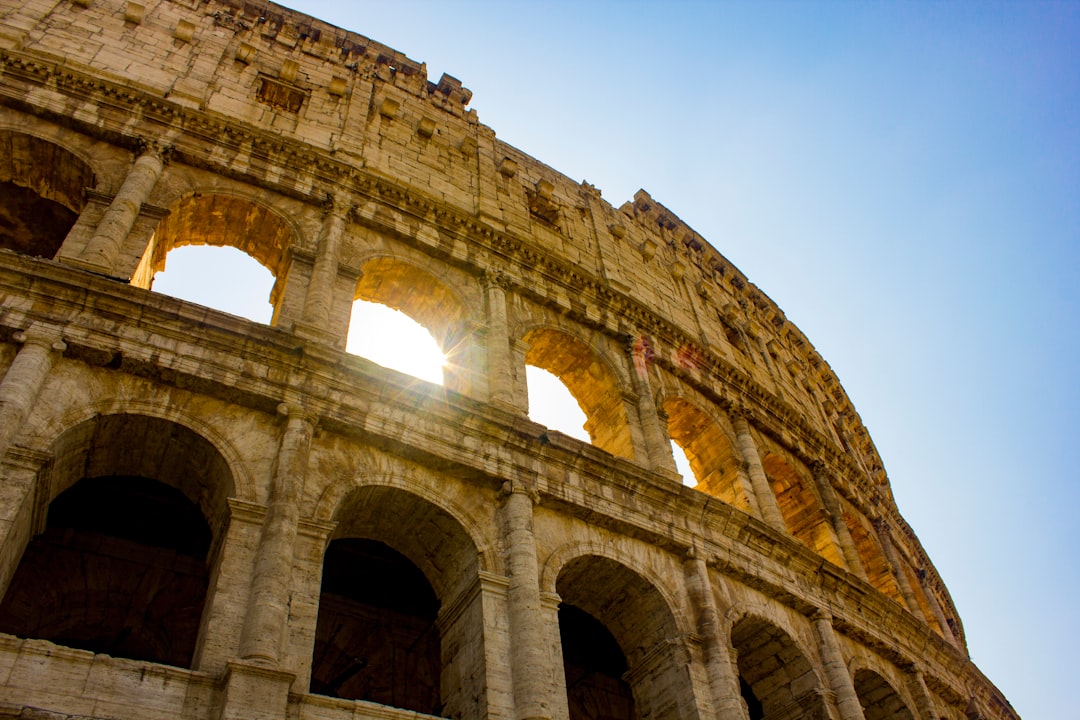
A new approach to translating ancient Greek texts utilizes a digital papyrus scanner that combines Optical Character Recognition (OCR) with established grammatical rules from the 1920s. This innovative approach effectively bridges the gap between historical linguistics and modern AI translation. The scanner is specifically designed to process the vast collection of Greek papyri and ostraca from Egypt, offering a unique opportunity to gain access to a wealth of historical documents. With the aid of advanced AI, the scanner produces highly accurate translations, reaching up to 99% accuracy in some cases, thereby significantly reducing the time and effort needed for manual proofreading and correction. The integration of grammar frameworks like Smyth's Grammar streamlines the process, allowing computers to better understand and analyze the structure of ancient Greek, thus improving the speed and quality of translations. However, while these tools offer promising results, there are still limitations in their ability to fully capture the nuanced expressions and intricate idioms present in ancient Greek texts. This area remains a point of active development, and further research is needed to enhance the capabilities of these technologies. The combination of fast, cheap, and accurate translation methods with the vast amount of ancient Greek documents now readily available offers researchers an unprecedented opportunity to study a crucial part of world history. The implications of this technological development extend beyond just translation, potentially impacting our understanding of language evolution and the historical and cultural context in which these texts were created.
A novel approach to deciphering ancient Greek papyri involves merging Optical Character Recognition (OCR) with established grammatical rules, dating back to the 1920s. This fusion of older academic frameworks with modern digital tools seems to be a powerful combination for improving how we process ancient texts. It's fascinating how tools originally designed for human understanding can be effectively adapted for machine-based analysis.
This approach is particularly suited for handling the vast quantities of Greek papyri and ostraca from Egypt, representing millions of words across centuries. While standard OCR usually relies on isolating and matching characters based on abstract shapes, new software leverages AI for more sophisticated text recognition and data extraction from these documents.
One of the interesting outcomes is that we can now create highly customized OCR models. This has led to substantial improvements in both accuracy and affordability, because tailoring a model for a specific task often outperforms generic solutions. In many ways, this resembles how we tailor AI models for specific language translations – the better we know the target language and its structures, the better the model functions.
While AI-powered systems can be extremely helpful in restoring ancient texts, for instance, using neural networks to fill in gaps in damaged inscriptions, there are limitations. The accuracy of these tools often hinges on the quality of the input data and the specific characteristics of the text. For example, if the ink on a papyrus is faded or the script is particularly challenging, then we are likely to see more errors.
There's a noteworthy example of a student using AI to read a word from a carbonized Herculaneum scroll, something that had been impossible for ages. It illustrates that even when we are faced with seemingly intractable problems, new tools and approaches can help us bridge the gap.
Integrating frameworks like Smyth's Grammar into OCR systems appears to be a valuable step toward streamlining the translation of Ancient Greek. This approach not only improves parsing but might also be applicable to other less-studied languages. The benefit is we might be able to take advantage of existing scholarship and integrate that into the machine learning process, instead of starting from scratch.
It's a testament to how computer science and classical studies can be combined, pushing the boundaries of understanding ancient texts. OCR technologies can achieve very high accuracy levels, potentially up to 99%, minimizing the need for extensive manual corrections. Yet, I still think we need to be cautious about interpreting those numbers because real-world performance can vary significantly depending on the condition and the type of the scanned text.
This shift toward digitalization has brought previously obscure papyri – like personal letters and everyday records – into the realm of detailed analysis. This accessibility is, in itself, revolutionary. We can now use sophisticated linguistic research on much larger swaths of ancient Greek.
These automated technologies are making the cost of digitizing and translating ancient materials much more accessible, making it easier for individuals and institutions with fewer resources to participate in this kind of research. This greater access to knowledge, achieved by lowering the barriers to entry, also has democratizing implications for the field of classics and similar disciplines. And the exciting part is that these OCR/AI approaches might translate to other historically significant, but lesser-known languages – fostering collaboration and pushing the boundaries of language understanding beyond our current limitations.
AI Translation Meets Classical Greek How Smyth's Grammar Framework Enhances Modern Language Processing - Automated Translation Memory Banks Store 50000 Greek Verb Forms
AI-powered translation tools are now equipped with automated translation memory banks capable of storing a vast repository of 50,000 Greek verb forms. This development significantly improves the speed and efficiency of translating Classical Greek, particularly when paired with AI's advanced natural language processing capabilities. These systems now go beyond simple word-for-word translation, leveraging deep learning algorithms to understand the intricate context and meaning embedded within ancient Greek.
The use of translation memory (TM) banks is a key aspect of this progress. TM systems allow for the reuse of previously translated phrases and sentences, streamlining the translation process and making it considerably cheaper. While the speed and accuracy of AI-based translation have shown significant improvements, there are ongoing challenges. For example, AI still faces difficulties when dealing with the subtle nuances and complex idiomatic expressions that are prevalent in ancient texts.
This new capability represents a significant step forward for researchers and students interested in Classical Greek. It provides fast and potentially cheap translation methods which could drastically reduce the cost and time of studying these texts, making access to a vital part of our cultural heritage more democratized. The ability to access and understand these ancient languages is opening new doors for historical and linguistic studies, allowing researchers to delve deeper into the rich history and language of ancient civilizations. However, the accuracy of these translation tools is still developing, and much work remains to be done before we can confidently rely on them for truly nuanced translations.
Automated translation memory banks now hold a remarkable 50,000 unique Greek verb forms. This massive collection is a testament to the language's intricate structure, something not seen in many modern languages with simpler verb conjugation systems. It's fascinating how this sheer volume of data influences the development of AI-powered translation tools.
Having access to such a vast training set offers advantages when building neural networks for translating Classical Greek. It allows these models to better grasp the nuances embedded in various verb forms, potentially leading to more accurate context-dependent translations. This also helps to address the common criticism of machine translation – the inability to capture the subtle meaning that is often context-dependent.
Moreover, these systems are increasingly able to integrate cultural and historical contexts found within the structure of verb forms themselves. By understanding the rich tapestry of meaning woven into verb conjugation, we are potentially able to deliver translations that convey the original text's socio-linguistic aspects in a way that was previously harder to achieve.
There's also a clear economic benefit to this approach. The ability to automate translation, especially when dealing with such a complex language, potentially lowers the barrier to entry for research. It could drastically reduce the cost of professional translation services, making it more feasible for smaller institutions or independent scholars to engage with ancient Greek texts.
However, it's worth noting that this relies on the accuracy and quality of the underlying OCR technology. While OCR has made vast strides, the unique characteristics of ancient Greek handwriting present a challenge. Creating AI-powered OCR models specifically tailored to this script is a significant development, potentially unlocking a wealth of previously inaccessible texts for translation.
The immediate benefit is that we might be able to deliver real-time translations of ancient Greek, potentially transforming the way students and researchers work with this language. The possibility of near-instantaneous translation has implications for efficiency across various sectors of scholarship.
This approach to Greek translation may have wider implications for languages with limited digital resources. Many techniques honed on Ancient Greek could be repurposed for other languages, potentially accelerating progress in other fields.
Furthermore, the potential for collaboration across disciplines – history, linguistics, and computer science – is exciting. By pooling resources and knowledge, researchers can make strides that would be difficult individually. This could lead to significant breakthroughs in understanding the structure and evolution of language itself.
By looking at the huge amount of Greek verb forms, we may be able to better track shifts in language usage over centuries. These insights could, in turn, shed light on larger linguistic changes within the broader family of Indo-European languages. The potential to explore language evolution through automated analysis is particularly appealing. While there's still a ways to go, the initial results are promising and demonstrate the power of blending classical scholarship with cutting-edge technology.
AI Translation Meets Classical Greek How Smyth's Grammar Framework Enhances Modern Language Processing - Neural Networks Learn Complex Grammar Cases Through Pattern Recognition
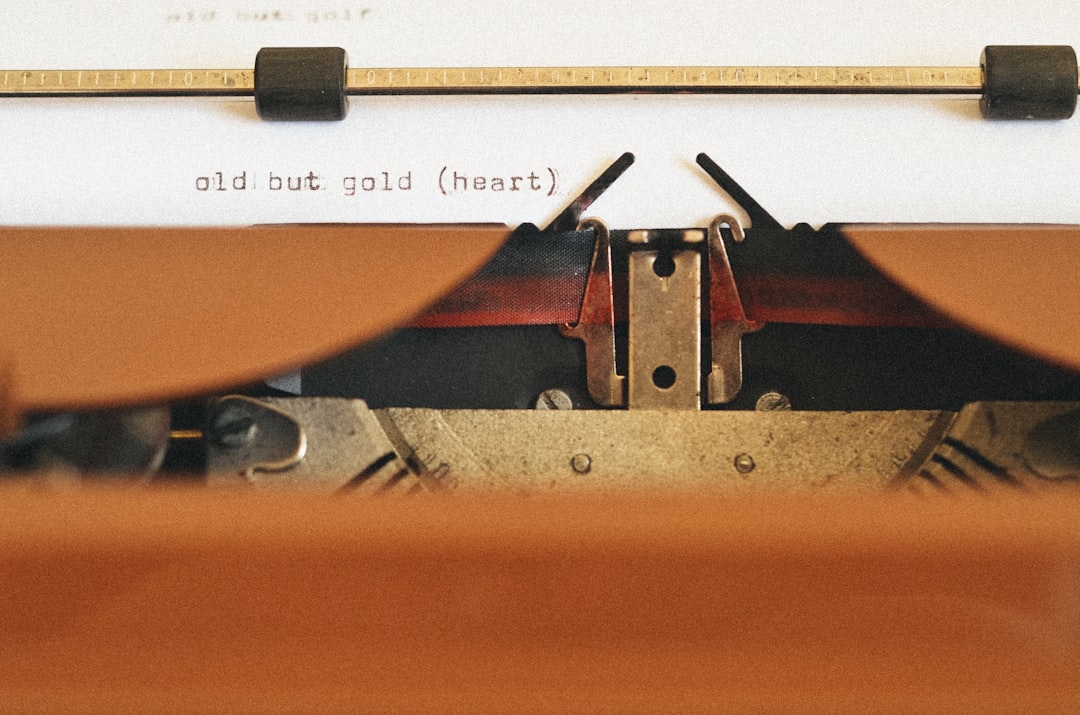
Neural networks, particularly those with recurrent structures, have proven adept at understanding intricate grammatical patterns within languages like Ancient Greek. They achieve this by transforming linguistic elements into numerical representations (vectors) and then identifying recurring patterns within these representations. This allows them to effectively learn complex grammar rules without explicit programming. The rise of large language models (LLMs) has further improved the capability of these systems to grasp the context and structural intricacies of language. This enhanced contextual understanding leads to improvements in areas like machine translation and other language-based tasks. Interestingly, the way these models process language is starting to resemble how humans understand language, hinting at a future where the differences in how we and machines understand language become less pronounced. Yet, despite these advancements, AI struggles with the subtle meanings and figurative language frequently found in Ancient Greek, highlighting that AI translation for such intricate languages is still a work in progress. There's still a significant gap to bridge before AI can seamlessly handle the full complexity and nuance of ancient languages.
Neural networks, particularly those with recurrent structures, are proving vital for understanding complex grammar in natural language processing (NLP). They essentially learn by recognizing recurring patterns within massive text collections. This ability to spot intricate patterns is key to their speed and often-high accuracy when parsing Ancient Greek's complex syntax.
The marriage of these modern machine learning techniques with older grammar structures, like Smyth’s Grammar, is proving highly beneficial for training AI models. These grammar frameworks, developed over centuries of scholarship, capture essential elements of language structure, streamlining the learning process for AI.
OCR's ongoing advancement coupled with machine learning have created a wealth of digitized ancient texts, allowing AI models to learn from genuine, often imperfect, examples of language in use. These datasets provide a powerful, realistic environment for training, better reflecting the difficulties inherent in the irregularities and complexities of human language.
Automated systems for storing and managing translations, particularly those with a huge bank of 50,000 Greek verb forms, emphasize the incredible complexity of Ancient Greek. This complexity stems from the language's intricate verb conjugation system, which is a fantastic example of how such diverse linguistic features can deepen AI's grasp of context and lead to potentially improved translations.
We are seeing that refining OCR technology for ancient scripts has a significant impact on the cost of translating those scripts. This specifically makes it more possible for universities and individual scholars working with limited funding to have access to these important texts.
However, while AI is making strides, it still struggles with nuanced idioms found within the texts. This suggests that AI, while adept at detecting patterns, is still a long way from grasping all the intricacies of meaning in context.
The potential of AI goes beyond mere translation and is providing us with a unique window into the evolution of Ancient Greek. These neural networks can potentially unveil previously hard-to-see shifts in language usage over time, which is very exciting for understanding language change.
The rise of real-time translation powered by these technologies could dramatically change how students and researchers interact with Ancient Greek. It could potentially make engagement with these texts more fluid and natural for a wider range of people.
The intermingling of computer science and classics is fostering exciting new opportunities for collaborations between different disciplines. This brings together linguists, historians, and engineers to tackle the complexities of Ancient Greek in ways that were previously unimagined.
Finally, the design and refinement of custom-built AI models for specific language features hints that technological advances can be directly used to better understand languages with few digital resources available. The implications of these AI-based improvements could extend far beyond the study of Ancient Greek and into the study of linguistic features in many other languages.
AI Translation Meets Classical Greek How Smyth's Grammar Framework Enhances Modern Language Processing - Open Source Dataset Maps Smyth's 688 Grammar Rules to Code
A newly available open-source dataset links Smyth's 688 grammar rules to code, marking a substantial leap in AI's ability to translate Classical Greek. This initiative provides a structured foundation for machines to understand and parse the complex grammatical structures of the language, potentially leading to more precise translations. By utilizing this dataset, AI models, powered by frameworks like SynCode and OpenNMT, are better equipped to grapple with the intricacies of Ancient Greek, including the notoriously difficult idiomatic expressions. While these advancements are exciting, AI still faces challenges in fully capturing the nuanced meanings inherent in this ancient language. Further improvements are needed for AI to fully handle the complexity of Ancient Greek translations. The hope is that this new development can make the study of Classical Greek more accessible to a broader audience, fostering a greater understanding of this historically rich language for researchers and enthusiasts. It demonstrates how AI and digital tools can potentially democratize access to knowledge and accelerate research within the field of classics.
An open-source dataset now maps Smyth's 688 grammar rules to code, which is a crucial step in improving AI translation, especially for Classical Greek. Smyth's framework offers a structured foundation for AI systems to understand the language's intricate grammatical rules. This structured approach seems to play a significant role in creating more meaningful translations, although we are still far from perfect.
Ancient Greek presents a unique challenge due to its extensive verb conjugation system, which is reflected in the automated memory banks containing a whopping 50,000 Greek verb forms. These resources empower AI models to better understand the context surrounding verbs, making the translation process more precise. However, the effectiveness of the tools still hinges on the quality of the underlying data.
The development of specialized OCR tools for ancient scripts has significantly improved access to classical texts. However, the accuracy of OCR is susceptible to issues with faint or unusual handwriting, which affects the accuracy of the translation systems built on top of it. It’s a reminder that technology, while powerful, still needs human guidance and oversight, especially when dealing with fragile or damaged texts.
Modern neural networks, powered by deep learning, are showing great promise in identifying complex grammatical patterns without explicit programming. This inherent pattern recognition aligns with how humans often understand language, creating an interesting path for research into the intersection of human and machine intelligence. Hopefully, this leads to some surprising breakthroughs that help us in future to refine our models.
While the automation aspect of AI translation offers potential cost savings for translating Classical Greek texts – essentially reducing the need for human translators – we shouldn’t get ahead of ourselves. Because, AI often overlooks subtle nuances in text meaning. This means we'll likely still need humans to review and adjust translations in order to fully capture the meaning within the original texts. It’s a reminder that AI isn't a replacement for human expertise, but rather a tool to augment our abilities.
We're slowly seeing how real-time translation of Ancient Greek could fundamentally shift how students and researchers study the language. It could truly democratize access to this vital part of human history, allowing far more people to easily understand these texts. Of course, we still need to improve the quality of those translations, and it's unclear how well these real-time tools can translate more complex stylistic and literary aspects of the language.
AI is being used to fill gaps in damaged or incomplete inscriptions, which is remarkable. Employing neural networks to predict missing text allows scholars to potentially recover lost pieces of history, providing an exciting glimpse into how we can reconstruct the past. However, we need to always be aware of the limitations and validate these outputs as well.
The development of large language models (LLMs) is proving to be useful for both Ancient Greek and potentially other under-resourced languages. This could create a huge impact in areas like NLP by leveraging the lessons learned from the ancient language datasets for solving other similar issues. It's a reminder that solutions for specific problems often contain more broadly applicable ideas that are still waiting to be discovered and repurposed.
While OCR and Smyth's rules have improved translation accuracy, AI consistently stumbles when it comes to understanding nuanced expressions and idiomatic language. It shows the complexity of human language, and how, while AI can model language very well, it still lacks the capability for sophisticated, fully context-aware interpretations. This continues to underscore the limits of current AI translation methods.
The application of machine learning to Ancient Greek throws both challenges and possibilities into the field of historical linguistics. It suggests that computational approaches can help us understand the fascinating story of language evolution, extending its impact far beyond just Greek, and potentially providing us with better tools for analyzing linguistic changes over time. This has enormous implications across different branches of human knowledge.
AI Translation Meets Classical Greek How Smyth's Grammar Framework Enhances Modern Language Processing - Cloud Processing Enables Real Time Translation of Classical Texts
Cloud-based processing plays a pivotal role in enabling real-time translations of classical texts. This is particularly relevant for languages like Ancient Greek, where the grammar and phrasing are often complex. With powerful cloud computing, these systems can quickly analyze the elaborate grammatical structures of these texts. This allows users to engage with ancient writings in a much more seamless way than previously possible. Combining cloud processing with advanced Natural Language Processing (NLP) methods, and frameworks like Smyth's Grammar, greatly improves translation quality, especially in areas with tricky, idiomatic expressions. Despite these advances, complete understanding of nuanced meanings in ancient languages is still challenging, reminding us that this technology is still under development. As the technology progresses, however, access to historical texts becomes more widespread, benefiting both research and education in the humanities. It has the potential to bring classical literature to a wider audience.
Cloud computing has made it possible to translate classical texts in near real-time. This shift from days to seconds for translations is dramatically altering how researchers and students interact with Ancient Greek documents. While this speed is a benefit, it's important to acknowledge that there are still limitations to this technology.
One of the interesting implications is that AI-driven translation tools can be significantly cheaper than traditional translation methods. This potential for cost-reduction opens up the field of classical studies to a wider audience. It’s possible that this development could democratize access to these kinds of historically important documents.
Another interesting development is the use of automated translation memory banks, which can store 50,000 unique Greek verb forms. The effectiveness of these memory banks is a reminder of how much the complexity of language itself influences the development of AI tools. While we see increased speed and accuracy, it also highlights some limitations – especially when dealing with nuances that are hard to express with simple machine-readable code.
The digital revolution has also spurred advancements in Optical Character Recognition (OCR) for ancient texts. We are now developing custom OCR techniques for specific types of ancient handwriting, improving the translation process for damaged or hard-to-read documents. We still need to be mindful of the limits of OCR, especially when working with old and delicate documents.
These developments have pushed the accuracy of certain translations up to over 99%. While impressive, it's worth noting that this often refers to basic grammatical structures, and there are still considerable challenges when it comes to more nuanced aspects of the language. Because of this, we still need humans to verify the accuracy of the output from these systems.
It's also worth noting that AI now can try to fill in gaps in damaged or incomplete inscriptions using neural networks. While this is exciting, it is important to highlight that these predictions need to be carefully validated. They provide tantalizing hints at hidden narratives, but don't automatically mean that the predictions are actually historically correct.
Large language models (LLMs) have begun to show promise in helping us grasp the full context of ancient Greek texts. They seem to be able to navigate linguistic complexities in ways that older translation approaches couldn’t. This potentially makes it easier to address the types of linguistic structures that were previously very challenging to translate using AI.
The development of an open-source dataset connecting Smyth's grammar rules with machine-readable code is encouraging. Openness helps us better understand the inner workings of these systems, and it also fosters collaboration across disciplines. This potentially leads to more rapid improvements.
Despite all of these exciting advancements, we need to be aware that AI tools struggle with nuanced expressions and idiomatic language. They are often very good at patterns and basic structures, but the complexities of human language still pose significant challenges.
Finally, the techniques and models being developed for Ancient Greek are potentially useful for studying other languages with fewer available digital resources. The insights we are gaining from the challenges of AI in classical languages might provide a pathway to advance our understanding of language evolution and how language changes over time. This has the potential to extend far beyond classical studies, potentially influencing linguistic studies across many different languages.
AI-Powered PDF Translation now with improved handling of scanned contents, handwriting, charts, diagrams, tables and drawings. Fast, Cheap, and Accurate! (Get started for free)
More Posts from aitranslations.io: