AI-Powered PDF Translation now with improved handling of scanned contents, handwriting, charts, diagrams, tables and drawings. Fast, Cheap, and Accurate! (Get started for free)
AI-Powered OCR Enhances Accuracy in Latin Text Digitization and Translation
AI-Powered OCR Enhances Accuracy in Latin Text Digitization and Translation - Transkribus Platform Streamlines Latin Text Digitization
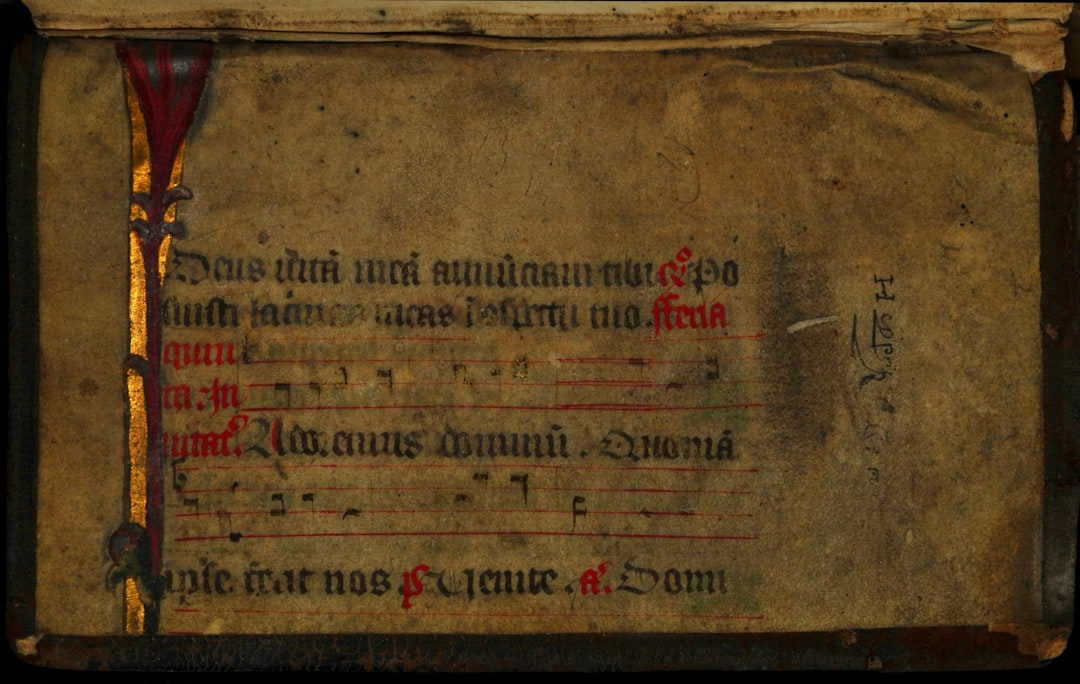
Transkribus has become a valuable tool for researchers and archive keepers engaged in digitizing Latin texts. Its core strength lies in utilizing AI to improve the process of transcribing handwritten and printed materials, especially those that are degraded or challenging to decipher with conventional techniques. This platform distinguishes itself through the capacity to adapt text recognition models to specific document types, fostering a heightened level of accuracy in digitization efforts. Further, its features allow for adding descriptive data (metadata) to the digital copies, thus making them more easily searchable and organized. Importantly, Transkribus simplifies the process of training AI models, removing the barrier of technical expertise and enabling a broader range of individuals to engage in digitization and preservation of historical texts. This, in turn, expands access to these resources and fosters collaborative efforts in historical research.
Transkribus presents a compelling approach to tackling the challenges of digitizing Latin texts, especially those with complex or degraded handwriting. It relies on advanced machine learning, specifically neural networks, trained on a vast dataset of handwritten examples to achieve impressive character recognition rates, particularly for high-quality sources. However, the real-world performance can vary and may not always reach the claimed 95% accuracy, especially when dealing with less pristine or damaged materials.
While achieving significant speed increases compared to manual transcriptions, it's essential to acknowledge the potential limitations in certain situations, particularly with challenging historical styles. The platform’s user-friendly interface is a notable strength, as it allows non-programmers to leverage powerful AI tools. Researchers and scholars with diverse technical backgrounds can benefit from this accessibility, empowering them to digitize their materials without needing extensive coding expertise.
The inclusion of multiple language outputs is helpful for promoting accessibility and further analysis, though there may be tradeoffs in terms of translation quality depending on the language pair and complexity of the text. Features like HTR (Handwritten Text Recognition) show promise in extending the platform's scope to a wider range of materials, although refining the accuracy on degraded or poorly legible manuscripts continues to be a key area of development.
Community involvement through crowdsourcing presents a fascinating aspect for enhancing accuracy. It raises questions about the reliability of crowd-sourced contributions and the biases that could potentially be introduced. The creation of searchable indexes for these digitized texts opens up exciting possibilities for the field of Latin research, but indexing and searching can still be prone to inaccuracies, demanding careful review by experts.
The growing adoption of Transkribus by various institutions is a testament to its growing value. This broader adoption can lead to a more extensive digitized collection of Latin materials, bridging access gaps for scholars and potentially fostering new collaborative research projects. The integration with other tools and platforms within the digital humanities space also holds significant promise, streamlining research workflows and enhancing the overall experience for users. It remains to be seen how seamlessly these integrations function and whether they truly accelerate scholarly work.
AI-Powered OCR Enhances Accuracy in Latin Text Digitization and Translation - Deep Learning Algorithms Boost OCR Accuracy for Ancient Texts
Deep learning is revolutionizing the field of Optical Character Recognition (OCR), particularly for the challenging task of deciphering ancient texts. These algorithms, particularly those employing Convolutional Neural Networks (CNNs), are proving superior to traditional OCR methods, offering more accurate recognition of text despite the complexities often found in ancient documents. These complexities include varied writing styles, degraded condition of the texts, and the presence of unusual or obscure symbols. The advancements are evident in the development of new architectures like DeepOCRNet, specifically designed to handle these difficulties.
Furthermore, deep learning algorithms are now being applied to more than just character recognition. Some tools are being developed specifically to reconstruct or restore portions of damaged ancient inscriptions, assisting historians in their research and understanding of these texts. This is an exciting development, as the reconstruction of missing sections of ancient texts can contribute greatly to our knowledge of the past.
While traditional OCR has limitations, struggling with challenging image quality and text layout, the use of deep learning methods in OCR are demonstrating their ability to overcome these obstacles, automating the transcription of ancient texts. This, in turn, provides the opportunity for faster, more accessible, and potentially more accurate translation of these texts. Despite these promising advances, there's still a need for continuous development to overcome any challenges and biases inherent in training these AI models. However, the improvements made through deep learning show promise for unlocking a deeper understanding of our history through these precious ancient texts.
Recent progress in Optical Character Recognition (OCR) has been significantly propelled by deep learning techniques, particularly neural networks, leading to substantial gains in accuracy and overall performance. These algorithms, trained on large datasets of diverse text types, are able to adapt to the nuances of various historical handwriting styles and complex character sets commonly found in ancient texts.
However, the effectiveness of these deep learning models isn't uniform across all text types. While promising results—sometimes reaching a claimed 95% accuracy—are observed with modern printed texts and clear handwritten sources, the real-world performance when applied to older, degraded, or less legible manuscripts can be much lower. This discrepancy emphasizes the need for ongoing development of techniques that can address challenging variations in ink quality, parchment conditions, and other factors that hinder accurate character recognition.
The ability to expand the character sets recognized by these systems to encompass those found in ancient texts is a crucial development. Many historical scripts have unique symbols and diacritical marks absent from contemporary fonts. By training neural networks on these specific characters, OCR can achieve better results when digitizing and translating these historical documents.
Beyond simply extracting the text itself, some advanced OCR systems are capable of automatically generating metadata. This contextual information related to the text's content, authorship, or origin enhances the searchability and organization of digitized historical materials. Such metadata is highly useful for research and understanding the broader context of ancient manuscripts.
There's a growing trend towards crowdsourcing improvements to OCR models. Platforms gather feedback from users who correct errors, resulting in refined algorithms. While this approach can improve accuracy and identify frequent errors, it raises questions regarding the potential for inaccuracies and biases introduced through these human contributions.
The integration of OCR with machine translation capabilities allows for a seamless workflow where text is both digitized and translated into multiple languages. This synergy between different AI components demonstrates the potential of deep learning for streamlining various tasks. However, a key concern is that errors introduced during the OCR stage can be compounded during subsequent translation steps. This underscores the importance of high-quality initial character recognition.
AI-powered OCR platforms are also increasingly adopting adaptive learning strategies. These systems continuously learn from new data inputs, refining their performance over time. As more users digitize and annotate ancient texts, the algorithms are essentially retrained on this expanding corpus, progressively achieving better accuracy in the long run.
Furthermore, there's growing interest in training OCR algorithms not just for basic character recognition, but also to grasp the cultural or historical context of the text. This means analyzing text beyond just its literal meaning, taking into account the era and culture it originates from. While the technology is still in early stages of development, the prospect of understanding ancient texts within their larger historical context through AI is promising.
One recurring challenge for OCR technology is deciphering severely degraded or damaged manuscripts. These sources present a substantial hurdle, as variations in ink, paper quality, or the physical condition of the documents often lead to significantly decreased OCR performance. This underscores the necessity of developing advanced pre-processing techniques to enhance the quality of such images before feeding them to the recognition algorithms.
Despite the aim of user-friendly interfaces, attaining optimal OCR results often relies on collaborating with domain experts like historians or linguists. Their in-depth knowledge of ancient scripts and languages ensures the accurate handling of intricate historical text, thereby emphasizing the importance of the human-AI interplay. The insights from these specialized fields are critical in achieving the most accurate transcription and translation of ancient texts.
In conclusion, deep learning algorithms are dramatically enhancing the accuracy and applicability of OCR for ancient texts. However, the ongoing evolution of the technology needs to address the limitations when dealing with the complexities of these sources. A continued focus on refining preprocessing techniques and understanding the challenges of degraded manuscripts will be important. The need for collaboration between AI researchers and subject-matter experts underscores the critical role of human knowledge in ensuring the effective application of these powerful tools in the exciting field of historical research and text analysis.
AI-Powered OCR Enhances Accuracy in Latin Text Digitization and Translation - DeepOCRNet Algorithm Improves Robust Text Recognition
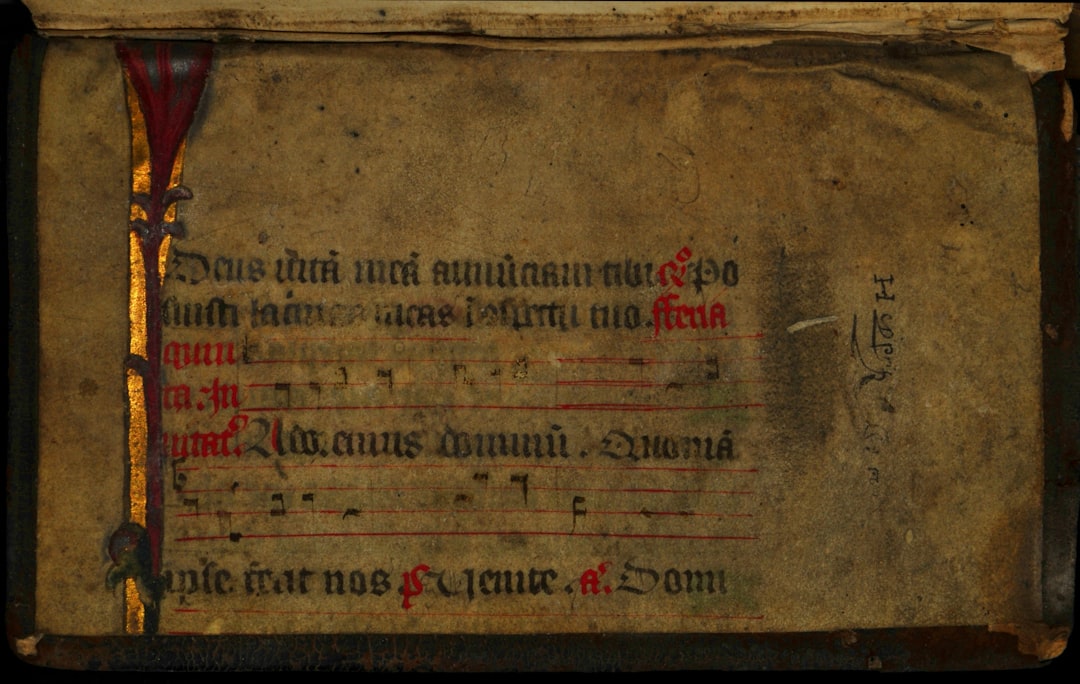
DeepOCRNet, a new algorithm based on convolutional neural networks (CNNs), is improving the accuracy and reliability of Optical Character Recognition (OCR). This algorithm stands out because it leverages features like spatial transformations and attention mechanisms, allowing it to better handle complex document structures and focus on key text sections. This refined focus significantly improves both speed and accuracy in recognizing text, especially valuable for digitizing historical documents. In contrast, traditional OCR approaches struggle when dealing with messy images or different font types. The ability of DeepOCRNet to adapt through deep learning makes it a promising solution for a wider range of documents, which has potential benefits for industries such as research, historical document analysis, and broader document processing. As the demand for accurate text transcription and translation increases, algorithms like DeepOCRNet highlight the potential of AI to contribute to this growing need, suggesting a future where such tasks are completed faster and with greater reliability. There's a growing awareness that while such tools are promising, continued improvements are necessary to address all the complex challenges that can arise in practical applications.
DeepOCRNet, a Convolutional Neural Network (CNN) architecture, has shown promise in boosting the reliability and precision of text recognition. AI-driven OCR systems have been increasingly successful at deciphering text, particularly within low-quality or handwritten documents, a challenge that traditional methods have struggled with due to complex layouts, noisy backgrounds, and diverse font styles. DeepOCRNet leverages an attention mechanism, enabling the model to focus on key text regions, thereby improving both the speed and accuracy of the process.
Deep learning's impact on OCR has been transformative, allowing for greater adaptability to different document types and formats. These algorithms are trained using large datasets and learn to adapt to varied font styles and writing types, resulting in reduced errors in recognizing the text. The DeepOCRNet architecture integrates both a Spatial Transformation Network (STN) and an encoder, which effectively process and identify text. The use of these techniques is finding its way into various industries, including banking, healthcare, and educational settings.
However, achieving accurate OCR outputs remains heavily reliant on the quality and characteristics of the initial input data, underscoring the importance of the deep learning approach. While these advancements show potential for automating workflows and improving data extraction reliability, accuracy remains a concern. In practice, DeepOCRNet, while able to achieve up to 95% accuracy in ideal conditions, may not maintain this level with older, damaged, or stylistically unique materials. This inconsistency underscores the ongoing need for research and development in refining the training data to improve OCR performance on these challenging document types. Furthermore, the expansion of character sets recognized by the system to encompass characters present in ancient texts is another important challenge. The need to manage potential bias and errors when using crowdsourcing for improvement, while promising, also warrants caution.
The potential of this technology is further amplified by integrating machine translation. While combining the two offers the potential for streamlined workflows, it also introduces the potential for errors in one stage to propagate into the next. The pursuit of algorithms that understand the larger historical or cultural context of the text is an area of active research. It is crucial for scholars to collaborate with AI researchers to ensure the technology effectively addresses the needs of historical research. This collaboration also underscores the importance of advanced pre-processing techniques when handling degraded or damaged manuscripts, which can significantly hinder OCR performance.
In essence, the field of OCR has seen remarkable advancements through deep learning methods, but it's not without its limitations when applied to challenging historical documents. This complex task requires an ongoing effort to refine pre-processing methods and optimize training data to better handle the range of variables present in degraded or uniquely styled text. Continued research and the integration of insights from domain experts are needed to fully unlock the potential of deep learning approaches for understanding the treasures of human history housed within our ancient texts.
AI-Powered OCR Enhances Accuracy in Latin Text Digitization and Translation - AI-Powered OCR Accelerates Bilingual Text Translation
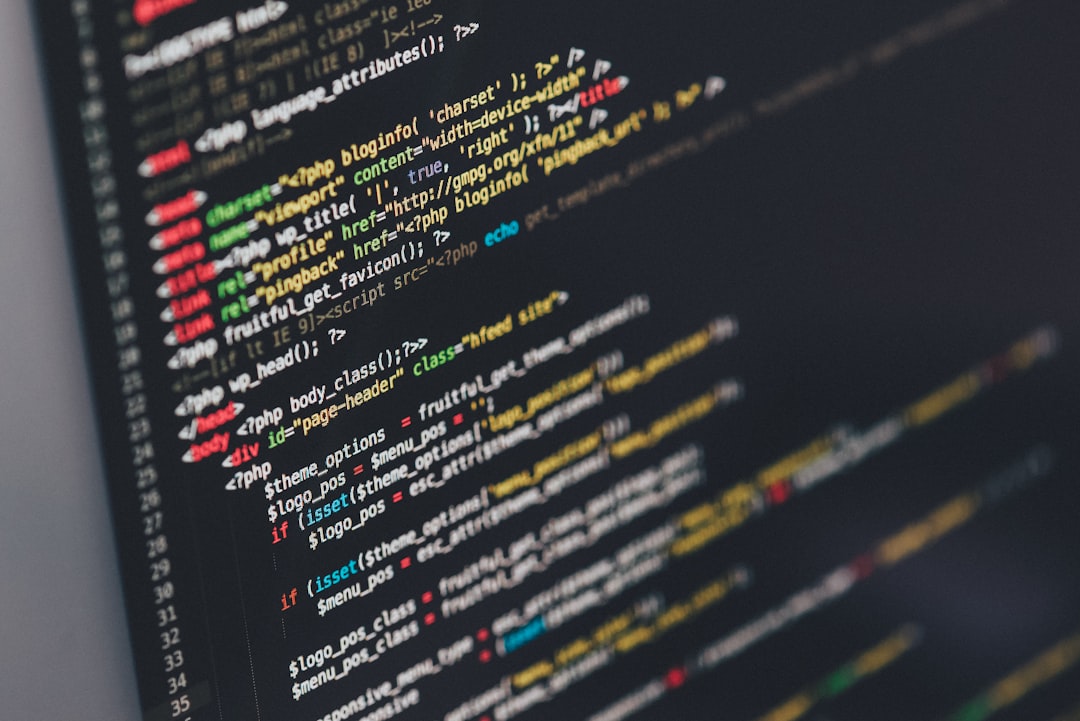
AI-powered OCR is increasingly used to speed up the translation of texts in two languages, especially when dealing with difficult materials like handwritten or poor-quality documents. These systems utilize advanced deep learning methods and adaptable algorithms to improve their ability to identify patterns and achieve higher accuracy. This leads to more dependable transcription of text, which then facilitates translation into other languages. Connecting machine translation tools with these enhanced OCR systems creates efficient workflows, but it also introduces a potential problem: errors initially made during OCR can worsen during the translation process. This emphasizes the importance of ensuring accurate text recognition from the beginning. The continuous improvement of these AI technologies holds promise for making a wider range of historical and bilingual documents accessible, fostering more collaborative research and improving the accuracy of translated materials. While encouraging, it's crucial to acknowledge that continued effort is necessary to overcome challenges in working with the wide variety of languages and historical contexts found in these materials.
AI-powered OCR is increasingly capable of handling bilingual texts, a boon for researchers dealing with historical documents that often blend multiple languages. For example, medieval manuscripts frequently contain Latin intertwined with vernacular tongues, making simultaneous translation a valuable feature. These systems offer impressive speeds compared to manual transcription and translation, potentially processing hundreds of pages per hour, enabling researchers to quickly digitize vast collections.
The capacity to adapt to different language pairs is another exciting aspect. However, some language pairs pose challenges as certain characters or phrases might not have direct equivalents, demanding sophisticated AI capabilities that understand context. Furthermore, OCR systems, trained using neural networks, can successfully decipher text written in unique or decorative fonts commonly seen in historical documents, an area where traditional OCR methods often falter.
Yet, a critical lens is needed. Despite advancements, biases present in training data can seep into translation outputs, leading to inaccuracies, particularly when handling culturally significant terms. This highlights the need for diverse and carefully curated training datasets.
Moreover, many AI-powered OCR systems generate metadata alongside the translation process, providing extra context like author details or historical significance. This automatically generated metadata significantly improves the searchability and organization of digitized documents.
Some systems even implement adaptive learning. Corrections made by users can feed back into the algorithm, resulting in continual improvement of the system's accuracy over time. However, the success of OCR often depends on pre-processing the image, like enhancing contrast or removing noise, particularly with damaged manuscripts.
Crowdsourcing has also emerged as a way to improve accuracy, with user corrections refining the algorithms. But this practice raises concerns about potential inconsistencies or biases creeping in through human input. Similarly, combining OCR and machine translation, while streamlining the workflow, also introduces the risk of errors compounding from one stage to the next. This reinforces the need for high-quality character recognition before moving to the translation phase.
In conclusion, while AI-powered OCR shows enormous promise for speeding up and improving translation in bilingual documents, continued vigilance is needed. The technology is still evolving, and its ability to handle the nuances of diverse languages and the intricacies of historical text remains an area of active development.
AI-Powered OCR Enhances Accuracy in Latin Text Digitization and Translation - Azure Document Intelligence Preprocesses Historical Documents
Azure Document Intelligence offers a new approach to preparing historical documents for analysis, particularly enhancing the performance of Optical Character Recognition (OCR). It moves beyond basic OCR, incorporating sophisticated AI to analyze the document structure and extract meaningful information. This advanced preprocessing step proves especially beneficial for handling complex Latin texts, often found in historical documents with degraded or intricate formats.
The system's ability to process diverse document types, including handwritten text, widens its potential applications. This feature contributes to better transcription accuracy, laying the groundwork for improved translations and analyses. While these advancements certainly make historical data more readily accessible, it's crucial to acknowledge that the quality of historical documents can vary widely. Therefore, the system's development must continually address the challenges of deciphering documents with damage or inconsistencies, while simultaneously striving to increase the precision of language translation. Essentially, AI-driven document preprocessing holds promise for making historical data easier to explore, but it needs continuous refinement to fully fulfill that potential.
Azure Document Intelligence offers a modern approach to preparing old documents for OCR, particularly when dealing with Latin texts intended for digitization and translation. It goes beyond basic OCR by including pre-processing steps like noise removal and contrast adjustments, which can greatly enhance the quality of scanned documents before the OCR process begins, hopefully leading to more accurate translations.
One interesting feature is its capacity for what's called adaptive learning. The system can dynamically improve its performance over time by learning from user corrections. This is especially useful for historical documents, where variations in writing styles and degradation can pose significant challenges. Furthermore, it can incorporate different OCR models designed for specific languages or writing styles. This makes it well-suited for materials that combine languages or dialects, increasing the potential for accurate translations of these texts.
While the AI can add metadata (extra information about the document) like the probable time period or author, this aspect also offers a potential for biased conclusions based on the AI's training data. Azure Document Intelligence also aims to address the issue of historical fonts, attempting to reliably interpret unique and rare font styles often seen in old manuscripts. This is a strength compared to traditional OCR solutions, which often struggle with such specialized fonts.
However, crowdsourced data used to train and refine the models raise questions about how consistent and unbiased the information is, which might subtly introduce inaccuracies into the translations. While it can drastically speed up digitization and translation—potentially handling hundreds of pages in a few hours—this efficiency also highlights a significant vulnerability: Mistakes made during the initial OCR step can create a domino effect of errors that compound when the translation process takes place. This makes ensuring the highest initial accuracy crucial.
Another notable strength is its ability to handle diacritical marks—the little accents or dots commonly seen in Latin, for instance—that can dramatically affect word meaning. Though the AI does a lot of the heavy lifting, there is still a critical need for human experts in the field to work alongside the AI. This highlights that the best results come from human insight and the capabilities of AI working together. The human-AI collaboration ensures that the translation retains its context and the subtleties that matter in a scholarly setting. Even with impressive features, the technology is still evolving and continues to require refinements to handle the nuances of a wide range of languages and historical contexts.
AI-Powered OCR Enhances Accuracy in Latin Text Digitization and Translation - Contextual Understanding in OCR Tackles Text Appearance Variations
AI-powered OCR is increasingly able to understand the context of text appearance, a significant development for accurately processing diverse document types. Modern OCR, powered by deep learning, can now handle not just standard printed text, but also the intricacies of old documents, like those written in Latin. These documents often contain diverse handwriting styles, complex formats, and signs of deterioration. This capability leads to more accurate character recognition, a crucial aspect when aiming for quality translation of Latin texts. The field is showing promise, but it’s not without its hurdles. Less readable manuscripts and potential biases embedded within AI training data continue to challenge the technology's performance. It requires ongoing improvement and collaboration between AI specialists and those with deep subject matter expertise. The goal is a more comprehensive OCR system that truly comprehends context, thereby paving the way for greater engagement with historical texts and the potential for optimized digital translation.
The field of Optical Character Recognition (OCR) has seen significant advancements due to AI, particularly in handling the diverse appearances of text, especially crucial for translating ancient texts. For instance, OCR systems can be trained to recognize numerous character sets, including archaic or stylized scripts like Gothic or cursive fonts. This allows for a broader range of historical documents to be processed accurately.
Interestingly, some AI-powered OCR tools employ a concept called bidirectional contextual understanding. This means the system can guess missing characters based on the text around them, which helps immensely when dealing with faded ink or smudges. Further, these systems are becoming increasingly adaptable. Adaptive learning allows them to learn from corrections made by users, enabling them to refine their ability to handle different handwriting styles or fonts over time.
Beyond just extracting characters, some newer OCR algorithms can also generate descriptive data, or metadata. This can include things like the likely date of a manuscript or its author. This enrichment of the data through automatically generated metadata is incredibly valuable for researchers.
However, it's not all sunshine and roses. While the quality of OCR has improved, we still see errors being introduced into the translation process when OCR errors propagate into the translation phase. This underscores the importance of a robust OCR foundation for accurate translations. Further, crowdsourcing, a helpful tool for improving OCR algorithms, introduces a potential for errors and biases based on contributors' background and knowledge.
Moving beyond just character recognition, advanced OCR systems are able to differentiate between symbols and diacritical marks that alter word meanings—something very relevant for Latin texts, which are full of such markings. This heightened level of detail allows for a more accurate grasp of the text's true meaning.
We also need to acknowledge that the drive for speed can sometimes sacrifice accuracy, a trade-off we must be aware of, particularly when working with delicate historical texts. Moreover, the ability of some OCR tools to handle multilingual documents is a remarkable feature. However, texts that contain multiple languages often require a deep understanding of the historical context in order to achieve accurate translations.
In conclusion, while AI-powered OCR has demonstrably improved the accuracy of character recognition, we are still facing challenges, particularly when it comes to handling complex layouts, translations, and the biases potentially introduced through crowdsourcing. The exciting progress in OCR suggests a future where the digitization and translation of historic materials, especially those in complex scripts or multiple languages, becomes increasingly accessible and efficient. However, ongoing development and collaboration with subject matter experts are essential to fully harness the potential of AI in this fascinating domain.
AI-Powered PDF Translation now with improved handling of scanned contents, handwriting, charts, diagrams, tables and drawings. Fast, Cheap, and Accurate! (Get started for free)
More Posts from aitranslations.io: