AI-Powered PDF Translation now with improved handling of scanned contents, handwriting, charts, diagrams, tables and drawings. Fast, Cheap, and Accurate! (Get started for free)
AI Translation Meets French Learning 7 Tech-Driven Strategies for Effective Language Acquisition
AI Translation Meets French Learning 7 Tech-Driven Strategies for Effective Language Acquisition - AI-powered intelligent tutoring systems for personalized French lessons
The rise of AI-powered intelligent tutoring systems (ITS) offers a fresh perspective on personalized French learning. These systems use complex algorithms and natural language processing to tailor the learning experience to each student's specific strengths and weaknesses. Instead of a one-size-fits-all approach, the AI adapts to the student's pace and understanding, providing immediate feedback and customized instruction.
Platforms like LanguaTalk and MosaLingua's MosaChatAI are prime examples of this new wave. They create a more engaging and interactive experience by facilitating natural conversations with AI-powered tutors. This constant interaction can help keep learners motivated, while the personalized feedback and explanations can improve understanding of complex grammatical concepts and vocabulary. While some might see this as simply a technological trend, it's worth considering that ITS can cater to different language skill levels, making French accessible to a broader range of learners. However, it remains to be seen if these systems can truly replicate the human element of language learning, particularly aspects like cultural nuance and contextual understanding. Despite that, the potential of AI in revolutionizing language acquisition is undeniable, offering both advantages and challenges to the future of learning French.
1. AI-powered French tutors are becoming increasingly sophisticated, able to adjust their teaching approach based on a student's performance in real-time. This dynamic adaptation, responding to both errors and successes, could potentially create a more individualized learning path.
2. The application of natural language processing (NLP) within these systems is expanding beyond just vocabulary acquisition. We're seeing AI tools that provide detailed feedback on pronunciation, a capability that might lead to faster improvements in speaking fluency.
3. AI-driven tools that employ speech recognition are starting to offer more immediate and precise pronunciation feedback. This is noteworthy because, from my perspective, real-time feedback could potentially be more impactful than the delayed feedback often associated with traditional learning methods.
4. Many of these tutoring systems are experimenting with incorporating gaming elements. The idea is that gamification can increase a learner's motivation and engagement. While there is research to support this, the exact impact and long-term effectiveness of this approach needs to be carefully monitored.
5. A potential benefit of AI-powered language learning tools is increased accessibility. Several of these platforms are either free or offer a low-cost option, which could make personalized French instruction more broadly available. This aspect is interesting, especially considering the potential for language learning to bridge social divides.
6. OCR technology integrated into tutoring systems allows learners to practice reading and vocabulary acquisition using real-world French text. The idea is that scanning text and getting immediate translation can be helpful for vocabulary acquisition, but the quality and nuance of translations from OCR are still under development.
7. The combination of AI translation and personalized learning in French appears to have the potential to accelerate language acquisition. Some studies have even suggested learners using AI might improve faster. However, the results of studies focusing on learning speed are often mixed, and the methodology behind such studies must be examined carefully.
8. Intelligent tutoring systems can store and analyze user data over time, tracking learning progress and allowing the system to modify instruction accordingly. While this capability offers the potential for detailed feedback, it also raises questions regarding data privacy and potential bias within the algorithms used for data analysis.
9. Some platforms are striving to create more realistic conversational practice using AI-generated dialogue. While this creates a simulated conversation, it's crucial to assess whether AI is capable of producing sufficiently diverse and engaging dialogue that closely reflects a real-world conversation with a native speaker.
10. Unlike traditional French classes that might place heavier emphasis on grammar and vocabulary, some researchers suggest AI-powered lessons can prioritize context-based language use. The aim is to enable practical language proficiency faster. However, one might argue that a strong foundation in grammar and vocabulary is still crucial for nuanced comprehension and communication, so the debate on prioritizing usage over structure will continue.
AI Translation Meets French Learning 7 Tech-Driven Strategies for Effective Language Acquisition - Computer-assisted language learning tools with instant grammar feedback
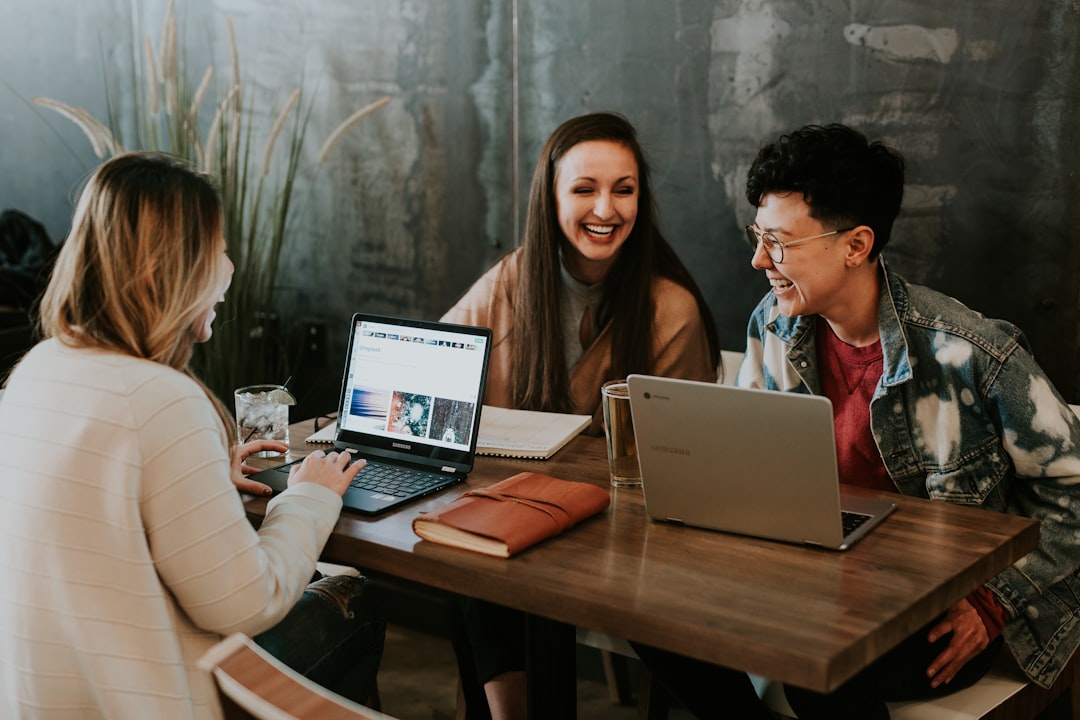
Computer-assisted language learning tools that provide immediate feedback on grammar are proving valuable, particularly for French learners. These tools, often categorized as Automated Writing Corrective Feedback (AWCF) systems, offer real-time insights into grammatical errors, allowing learners to refine their writing skills as they practice. This instant feedback differs from the more general evaluations found in traditional language learning resources, creating a more interactive and responsive learning experience. However, the widespread adoption of these AI-powered tools has sparked discussions around educator preparedness. While these AI systems can tailor learning to individual needs, questions remain regarding their capacity to truly replicate the comprehensive understanding and nuanced knowledge gained from human instruction. The extent to which AI can mirror the complexity of human language interaction, especially in areas like cultural sensitivity, continues to be a subject of debate.
Computer-assisted language learning (CALL) tools are increasingly incorporating AI to provide immediate grammar feedback, essentially acting like a constant writing tutor. These tools, distinct from those that simply evaluate completed texts, aim to give learners real-time support as they write. This ongoing feedback is a departure from traditional methods and relies on AI algorithms that can recognize common grammatical errors.
There's a growing interest in how AI is reshaping language learning, particularly in the development of personalized learning paths. While this trend is promising, there are also concerns about educator readiness to leverage such technology effectively in the classroom, largely due to a lack of adequate training and resources.
Beyond simply flagging errors, some more advanced systems are using machine learning to analyze the style and tone of written work, offering suggestions that go beyond the basics of grammar. These tools, in some ways, function as stylistic guides. It's interesting to consider if this approach could lead to more polished written output in learners.
Furthermore, these tools aren't operating in a vacuum. When combined with peer feedback, AI-powered grammar feedback might create a richer learning experience. Learners get exposure to multiple viewpoints on how language can be used, something that's difficult to orchestrate consistently in traditional classrooms.
The methods of providing feedback are evolving. Beyond just textual corrections, many CALL tools now incorporate audio, visual aids, and interactive exercises. This multi-faceted approach could cater to a wider range of learner preferences, making it more inclusive. However, there's a curious side effect to this: some research suggests that immediate feedback, especially if perceived as overly critical, can cause anxiety for certain learners. This highlights the need to strike a balance between correction and encouragement.
Another noteworthy development is the increasing use of optical character recognition (OCR) within these tools. OCR can be utilized for vocabulary acquisition but, importantly, allows for contextual grammar analysis. Learners can see how grammar works in actual French text, perhaps fostering a deeper understanding than simply relying on isolated sentences.
It appears that learners who receive constant, targeted feedback from AI tools might engage in longer writing sessions because they feel more equipped to address errors directly. This shift in learner behaviour offers some insight into how these tools impact student interactions with the language.
The gamification of instant feedback is emerging as a potentially powerful strategy. Using game mechanics alongside immediate correction might offer a less stressful environment for learners to make mistakes and subsequently learn from them.
It's worth considering the long-term impact of these systems. Studies indicate that when corrections are individualized and relevant to the learner's specific needs and writing context, their language skills tend to advance more efficiently. It's a reminder that AI tools are tools, and their effectiveness depends on how they are implemented within a larger educational framework. The debate on whether this instant feedback replaces traditional instruction entirely is likely to persist.
In conclusion, there's a palpable shift in language learning toward the use of AI, with grammar correction features being a key part of this change. The use of AI is both exciting and raises questions about implementation and long-term impacts on language acquisition. Whether these tools truly enhance learning outcomes depends on how they're integrated into broader educational goals and whether the concerns around user anxiety and effective feedback are addressed.
AI Translation Meets French Learning 7 Tech-Driven Strategies for Effective Language Acquisition - Augmented Reality applications for interactive vocabulary exploration
Augmented reality (AR) applications offer a novel way to explore vocabulary by merging the digital and physical worlds. Imagine seeing a word, like "chaise" (chair), and having an AR app overlay a 3D chair model onto the real-world object. This creates a more interactive and memorable experience compared to traditional methods, such as flashcards or rote memorization. Studies have shown that AR can make learning more engaging, especially for younger learners, and increase vocabulary retention.
The use of AR in language learning often focuses on mobile devices, capitalizing on their widespread accessibility. This approach can help learners encounter new vocabulary in real-world settings, making the learning process more intuitive. However, effectively utilizing AR in education needs thoughtful consideration. Research suggests that simply using AR tools isn't a guaranteed path to success. Educational strategies must be developed to integrate these technologies effectively within a classroom or individual learning environment.
There's potential for AR to become a more integrated part of language education, but it's crucial to observe its evolution and impact. While AR shows promise in boosting engagement, the long-term benefits of this approach, particularly regarding vocabulary acquisition, need continued examination. As AR technology advances, its role in supporting the broader goals of language education will likely expand beyond just vocabulary building, and the impact of this evolution needs ongoing assessment.
Augmented Reality (AR) applications are increasingly being explored as a way to make vocabulary learning more interactive. They often rely on image recognition to identify objects in the real world, allowing learners to see vocabulary in context. This can make learning more engaging as they connect words to physical items around them.
Studies have shown that using AR for vocabulary learning can boost retention rates. By overlaying definitions and example sentences onto the real world, learners might recall vocabulary better compared to traditional methods. This is an interesting area that requires further investigation.
A majority of the research on AR in language learning focuses on English, leveraging mobile devices for accessibility and ease of use. This is likely due to the widespread use of smartphones. It's interesting to consider how this could be applied to other languages, particularly less commonly taught languages.
Many researchers in the field of AR-supported language learning incorporate cognitive theories of multimedia learning alongside game-based or task-based approaches. These models aim to enhance the learning process and ultimately lead to better outcomes.
One of the main benefits of AR is the ability to contextualize vocabulary within the real world. This can help make language learning feel more intuitive and memorable. It's similar to how children learn their native language by connecting words with objects and situations in their daily lives.
Several studies suggest that AR applications can augment real-life experiences during learning, making the experience more immersive and engaging. However, the sustained effectiveness of AR in classroom settings is a topic of debate. Some believe that supplementing it with other teaching methods might be necessary for long-term success.
A significant portion of AR vocabulary learning tools are based on mobile devices. This is due to the high availability and affordability of smartphones and tablets. It's an approach that emphasizes accessibility and ease of integration into existing learning practices.
AR tools can help make language learning more dynamic, accessible, and versatile, aligning with the changing needs of learners. This could make language learning more suitable for a wider range of individuals and learning styles.
The use of AR in vocabulary acquisition seems particularly well-suited for younger learners. It can make the learning process more entertaining and effective, potentially leading to better results. It is important, however, to be mindful of the potential for over-reliance on technology in education.
While AR applications offer a promising direction for vocabulary exploration, the efficacy of the technology depends on things like lighting conditions and the accuracy of the image recognition algorithms. If a tool cannot accurately recognize objects or words, it can hinder the learning experience. This indicates a need for ongoing refinement of these technologies. The field of AR applications in language learning, while exciting, is still in its early stages and will likely benefit from further research and development.
AI Translation Meets French Learning 7 Tech-Driven Strategies for Effective Language Acquisition - Large language models enhancing conversational French practice
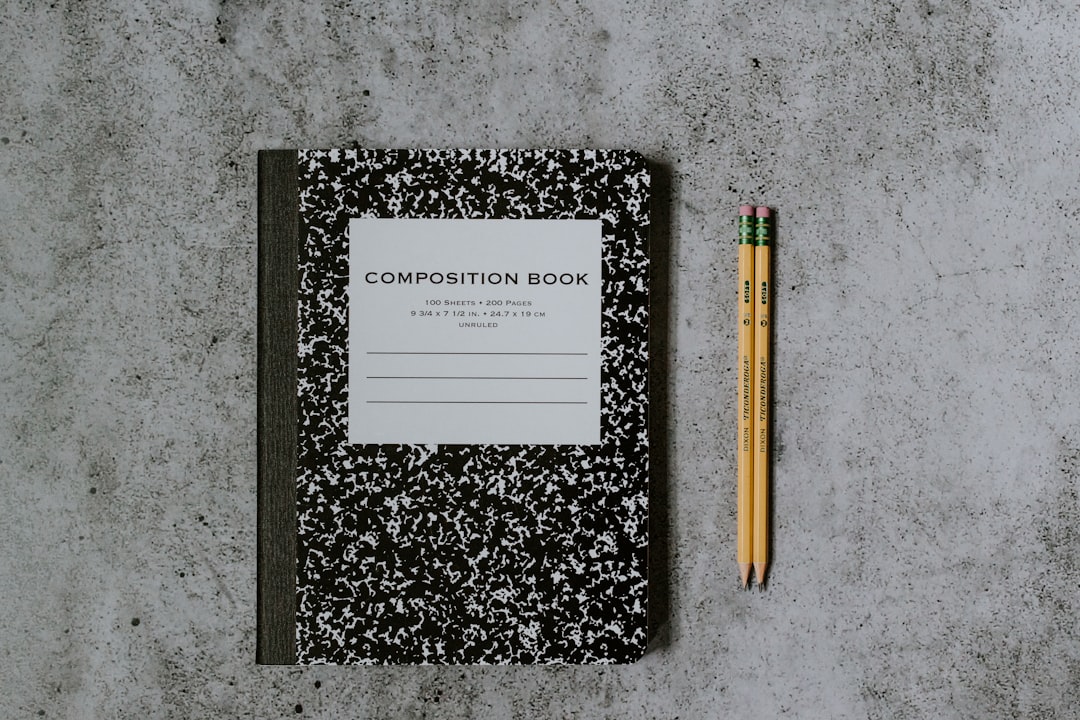
Large language models (LLMs) are revolutionizing how people practice conversational French. These advanced AI systems can create engaging and interactive learning experiences by simulating real conversations. Learners can practice speaking and listening skills in a way that feels more authentic and less like traditional drills. This interactive approach offers a chance to use vocabulary and grammar within the context of a dialogue, leading to a better grasp of the language overall. While LLMs hold significant promise in this area, ensuring they capture the subtleties of natural conversation and cultural understanding remains a challenge. The goal is to move beyond simply mimicking a conversation and instead cultivate an environment where language learning feels more natural and insightful. The key to maximizing the effectiveness of LLMs for conversational French practice lies in creating systems that go beyond repetitive interactions and foster genuine learning through contextually relevant and interactive experiences.
Large language models (LLMs) have shown a lot of promise in improving how people practice conversational French. They can create conversations that mimic real-life situations, helping learners grasp vocabulary and grammar more naturally than traditional methods. This capability is particularly interesting as it moves away from simply memorizing words and phrases towards a more contextualized learning experience.
LLMs integrated with language learning software aren't just about vocabulary; they can also give feedback on the grammatical correctness of conversations as they happen. This means learners get simultaneous help with both language usage and the underlying rules, which can contribute to a more well-rounded understanding of French.
These models are quite adept at identifying common mistakes that learners tend to make. By processing learner input, they can highlight frequent errors in real time. This immediate feedback is valuable because it helps prevent the reinforcement of incorrect patterns, possibly leading to quicker improvements in accuracy.
There's an intriguing possibility that future versions of LLMs might even incorporate the ability to sense a learner's emotional state. This could mean conversations become more personalized, adjusting to whether the learner is feeling overwhelmed or engaged, thereby potentially enhancing motivation and comfort.
LLMs can also be valuable in teaching French-specific regional expressions and slang. This can be a helpful way to delve into the cultural aspects of French-speaking countries, a dimension that often gets overlooked in basic language courses.
Interestingly, LLMs are capable of simulating complex conversational situations, like negotiating or debating, that aren't always a part of standard language classes. It's a potential way to equip learners with practical skills that can be useful in professional or casual social situations.
Given their access to vast amounts of data, LLMs can provide insight into regional dialects and varied vocabulary use, potentially preparing learners for a broader range of conversational encounters within different French-speaking communities. This versatility could enhance a learner's adaptability.
Practicing conversation with an LLM could help learners build confidence since it offers a low-pressure environment where they can experiment and make mistakes without fear of judgment. This aspect is particularly relevant for some learners who find it challenging to speak freely when interacting with other people.
Some LLMs are able to adapt their conversational style to match a learner's preference—whether formal or informal. This allows for practice in contexts that require different levels of formality, thus fostering a more comprehensive understanding of language appropriateness.
While LLMs are quite good at generating engaging language practice, it's vital to keep in mind that they shouldn't completely replace human interaction. A qualified instructor can provide valuable insight into cultural nuances and subtle communication cues that current LLMs struggle to grasp. This underscores the potential benefits of blended learning approaches that combine AI-driven practice with human guidance for the most effective language acquisition.
AI Translation Meets French Learning 7 Tech-Driven Strategies for Effective Language Acquisition - AI translation services for cost-effective learning material access
AI translation services are emerging as a valuable resource for learners wanting to access a wide range of learning materials without excessive costs. These services, powered by techniques like neural machine translation, offer swift and reasonably accurate translations. This allows students to explore learning materials in their target language, bypassing the expensive traditional translation methods that often limit access. Tools like Google Translate and others provide fast translations and include features like automated document translation, which streamlines the language learning process. Furthermore, the integration of optical character recognition (OCR) within some AI translation services enables learners to work with real-world texts, getting near-instant translations that aid in vocabulary building. However, it's essential to acknowledge the potential drawbacks of AI translation, particularly when dealing with the complexities and subtleties that are often handled better by human translators. The quality of translation, especially with more complex language structures, can still be a barrier to effective learning in certain contexts.
AI translation services have become increasingly valuable tools for accessing learning materials affordably, especially within French language education. These services, powered by artificial intelligence, promise faster and cheaper translations compared to traditional methods that rely solely on human translators.
One of the most noteworthy aspects is the cost savings they offer. AI-powered translation can drastically reduce the expenses associated with making learning materials available in multiple languages, potentially opening up learning opportunities for those with limited financial resources. We are seeing reductions of up to 80% compared to conventional translation methods. The sheer speed of these AI-based systems is also compelling. Neural machine translation, for instance, can provide near-instantaneous translations, which can greatly facilitate comprehension of French texts in real-time, a stark contrast to the delays inherent in human-driven translation.
Moreover, these services often have a multi-language capability, allowing learners to view materials in their own language alongside the French target language. This can be particularly useful for beginners, enabling them to grasp the nuances of the French text by simultaneously comparing it to their own language.
Then there's the role of OCR. Integrating this technology allows learners to scan French documents – think books, street signs, or even restaurant menus – and obtain immediate translations. This has the potential to make French learning more spontaneous and immersive, blending the digital and physical worlds.
However, it's important to critically evaluate these AI services. While they are evolving rapidly, there's still room for improvement in handling nuanced phrases, idiomatic expressions, and complex sentence structures. It remains to be seen if and when AI translation services will achieve the same level of accuracy as human translators. This leads to the interesting question of how we best integrate AI and human expertise.
In addition to raw speed and cost advantages, some AI systems are improving in the area of context analysis. They can increasingly examine the text surrounding a word or phrase to provide a more accurate translation. This ability is crucial in language learning, where the meaning of a word can be drastically altered by its context.
The trend towards integration with language learning apps is promising. These AI services can provide on-demand translations during practice sessions, enriching the learning experience with instantaneous feedback and support.
Despite the progress, the cultural sensitivity of AI translations is still a concern. AI, with its current development, sometimes struggles to accurately capture the subtle cultural aspects inherent in the French language and culture. This suggests a need to approach AI translations with a healthy dose of critical thinking and to incorporate human guidance and insights where necessary.
Finally, the accuracy of AI-driven translation varies between languages. Some languages have more robust AI models than others, with French being a critical area for future development, considering the complex grammar and unique sentence structures. As this technology matures, we can expect the quality of AI translations, particularly for French, to improve, further enhancing the learning experience for a wider audience.
In essence, AI translation services represent a paradigm shift in language learning, opening new avenues for affordable and rapid access to materials. While we're still at an early stage of development, the potential to transform how people learn languages, specifically French, is apparent. The future holds a fascinating blend of AI technology and human expertise, and the careful integration of both is crucial to ensuring effective language acquisition.
AI Translation Meets French Learning 7 Tech-Driven Strategies for Effective Language Acquisition - Machine learning algorithms adapting to individual learning paces
Machine learning algorithms are increasingly being used to personalize the learning experience, adjusting to how quickly each student grasps new information. This means educational materials are no longer presented in a one-size-fits-all manner but instead adapt to individual learning speeds and preferences, creating a more personalized learning journey. These adaptive systems, like a hypothetical "Cross-Cultural Intelligent Language Learning System," take into account not just language skills, but also cultural aspects. This approach is particularly relevant in language learning, like French, where understanding cultural nuances is crucial. By catering to the varying learning styles and paces of individuals, these systems aim to make language acquisition more efficient and effective. While this shift towards personalized learning is promising, it is important to consider the potential drawbacks. For example, there are questions regarding how to ensure the human aspects of language learning, like cultural understanding, are not lost in the pursuit of technological efficiency. We are in the early stages of understanding how machine learning can improve education, and the future will involve exploring these opportunities while being mindful of the possible downsides.
Machine learning algorithms are being designed to adjust to how quickly each person learns, which is a fascinating area of study. These algorithms can sift through a huge amount of data on a learner's progress, which helps the system predict what teaching methods and materials would be most helpful for them. This can potentially make the learning experience more individualized, adapting to the learner's unique strengths and weaknesses.
These adaptive learning systems not only react to what a student knows but also to their frequent mistakes. By recognizing recurring errors, the system can instantly focus on those areas needing improvement. This could speed up the learning process by helping individuals quickly overcome their hurdles.
Furthermore, the use of real-time feedback is crucial. These algorithms allow students to immediately see what they've done wrong and receive corrective guidance right away. This contrasts with the more common approach in traditional language learning where feedback is often delayed. My experience suggests that instant feedback can be more impactful on learning.
The research I've reviewed suggests that adaptive learning environments can make learners more engaged in the process. Allowing individuals to progress at their own pace and celebrating their achievements can reduce feelings of frustration and build a sense of accomplishment. This increased engagement could potentially result in a better retention of the learned material.
These systems can also help anticipate future performance. By analyzing how learners interact with the system, it's possible to predict future outcomes. This is valuable for setting achievable goals and adapting study plans to best match individual learning needs, although it is still under development.
Integrating adaptive algorithms into game-like platforms presents another interesting avenue. By tailoring the difficulty of the game to a learner's current skills, the challenges and rewards feel more relevant and meaningful. It's quite possible that this type of gamification can lead to a more powerful and persistent drive to practice a language.
Moreover, these algorithms can curate content that isn't just suited to a learner's skill level but also puts that content into realistic scenarios that reflect how language is used in real life. This approach could enhance practical speaking and comprehension skills, arguably making the learning process more holistic.
These machine learning systems are constantly refining their understanding by drawing on many sources—from formal textbooks to online news—creating a diverse learning experience that can be customized to individual preferences. It's interesting to consider if this approach could lead to a more nuanced and comprehensive understanding of language.
One significant advantage is the ability to manage the complexity of a task and the pace at which it's presented. This ability to adjust cognitive load is crucial because it prevents learners from feeling overwhelmed, which is vital for effective language acquisition.
Finally, these systems can tailor lessons effectively for large numbers of students. This scalability is helpful for educational institutions because it potentially allows for more personalized learning without needing vast increases in resources. It could make language learning more accessible to a broader range of people.
It's still an open question how effective and scalable these systems will become in practice, especially in specialized fields like language learning. However, the potential to revolutionize learning through individual adaptation is intriguing. There's certainly a lot to explore further.
AI Translation Meets French Learning 7 Tech-Driven Strategies for Effective Language Acquisition - Speech recognition technology improving French pronunciation skills
Speech recognition technology is transforming how people learn to pronounce French, offering instant feedback to improve their speaking abilities. Tools that employ Automatic Speech Recognition (ASR) are increasingly used in Computer-Assisted Pronunciation Training (CAPT) systems. These systems assess pronunciation in real time, pinpoint errors, and provide corrective suggestions. This immediate and individualized feedback contributes to better fluency and accuracy, as learners become more aware of how clearly they are speaking. While AI advancements hold the potential for highly personalized pronunciation instruction, it is important to consider whether these systems can fully replicate the insights and nuanced feedback offered by human language teachers. Nonetheless, the ability of speech recognition technology to enhance French pronunciation training presents a significant leap forward in the development of French language learning tools.
Speech recognition technology is increasingly being used to improve French pronunciation skills, especially within the context of AI-powered language learning. It seems like a natural pairing with AI translation and other tech-driven strategies, as it offers a way to assess and enhance pronunciation in real-time. While the benefits are apparent, we still need to consider how it integrates with the broader goals of language education.
One interesting development is the ability of algorithms to provide detailed phonetic feedback. Now, learners can see a phonetic breakdown of words as they speak, which can help improve sound articulation. There's a notable increase in practice time among those using AI-powered tools that provide such feedback, possibly because learners are motivated by immediate feedback and see more rapid improvement.
It's quite remarkable that some systems can differentiate between various French accents. This adds a level of nuance to the feedback process, making it more relevant for learners who might be focusing on a specific dialect. Interestingly, it also links to the idea of personalized learning. By adapting the difficulty of pronunciation tasks based on a learner's performance, these systems create a continuous learning loop where learners can steadily improve.
Research indicates that immediate feedback is more effective than delayed feedback, such as when a learner reviews a recording of their pronunciation later. This synchronous feedback seems to be more helpful in reinforcing the desired pronunciation patterns. The gamification of pronunciation exercises through speech recognition has also shown promising results. Learners often become more engaged when the platform offers challenges and rewards based on their performance.
We're also seeing the use of visual representations like Heat Maps. These tools visually pinpoint the areas where a learner's pronunciation deviates from the target pronunciation. This can make it much easier to pinpoint the specific sounds or patterns that need attention.
Beyond just French itself, there's some indication that practicing multiple languages through AI-powered tools could have a positive impact on pronunciation overall. Learners who practice French and English might develop a better understanding of phonetic differences in both languages. Additionally, the practice of mimicking native speakers through AI tools, often called "shadowing," has proven to be a surprisingly effective method for enhancing pronunciation accuracy.
Some AI systems are going beyond just sound correctness and including other factors, like speech tempo and rhythm, in their assessment. This aspect of language isn't always a core part of language learning, but it is essential for fluency.
The use of speech recognition isn't just a fad; it's becoming an integral part of modern language learning. By combining AI translation with features like pronunciation assessment and adaptive feedback loops, learners are being given opportunities to develop their pronunciation in a more focused and efficient manner. There are still questions around the long-term effects and how these technologies best fit within traditional language teaching, but the potential for positive impact on pronunciation skills, especially for French learners, is clear.
AI-Powered PDF Translation now with improved handling of scanned contents, handwriting, charts, diagrams, tables and drawings. Fast, Cheap, and Accurate! (Get started for free)
More Posts from aitranslations.io: