The Evolution of Google Translate From SMT to Neural Machine Translation in 2024
The Evolution of Google Translate From SMT to Neural Machine Translation in 2024 - From Rules to Statistics The Early Days of Google Translate
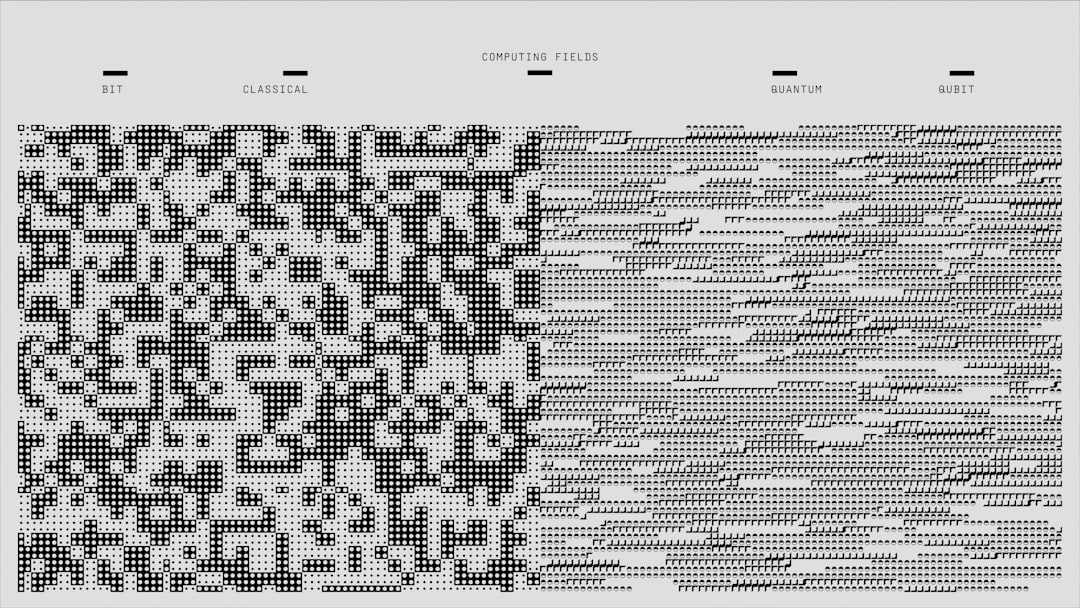
Google Translate's journey began with rule-based systems that relied on predefined linguistic rules. This early approach, though ambitious, was inflexible and lacked the accuracy needed for seamless translation. The introduction of statistical machine translation (SMT) offered a significant improvement by leveraging large amounts of bilingual data to statistically analyze and predict translations. This approach brought greater flexibility and accuracy, but still suffered from limitations in understanding context.
The arrival of neural machine translation (NMT) in 2016 truly transformed Google Translate. NMT models mimic the human brain's functioning, processing entire sentences and considering context for a more nuanced translation. This revolutionary approach resulted in significant improvements in accuracy and fluency, offering a more natural and human-like translation experience.
The journey of Google Translate from its early days is a fascinating one, mirroring the broader evolution of machine translation itself. Starting with a reliance on statistical models, Google Translate's initial attempts were marked by a reliance on vast quantities of bilingual data to extract translation patterns. While this provided a foundation for translation, it also came with limitations, especially when faced with nuanced language, idioms, and the need for contextual understanding. The use of phrase-based models, breaking sentences into smaller chunks, helped to alleviate some of these issues, boosting accuracy, particularly for longer texts.
This early period also saw the introduction of Google Translate's "Translate this page" feature, which ushered in a new era of on-demand web translation. The ability to instantly translate entire web pages opened up a world of previously inaccessible information for non-native speakers. Furthermore, the integration of OCR technology (Optical Character Recognition) broadened the scope of Google Translate, allowing users to translate text directly from images or handwritten notes, a feature immensely valuable for travelers and language learners.
However, despite these advancements, limitations remained, particularly in understanding sentence structures, often resulting in incorrect word order and grammatical errors. The introduction of Google Neural Machine Translation (GNMT) in 2016 marked a paradigm shift, bringing about a more sophisticated approach based on deep learning techniques, mirroring the human brain's processes. This not only improved accuracy but also introduced a greater understanding of context and a more natural flow to translations, addressing the shortcomings of statistical models. This shift allowed Google Translate to expand its language support to over 100 languages, making it a fundamental tool for global communication.
The ongoing development of Google Translate relies on a constant interplay between user feedback and data-driven updates, creating an iterative process of refinement and improvement. A key aspect of this evolution is the shift towards using user-generated translations, supplementing the initial pool of publicly available bilingual text. While this can lead to a richer and more diverse dataset, it also raises questions about quality control and data integrity, highlighting the ongoing challenge of balancing accessibility and accuracy in the world of machine translation.
The Evolution of Google Translate From SMT to Neural Machine Translation in 2024 - Neural Networks Revolutionize Machine Translation in 2016
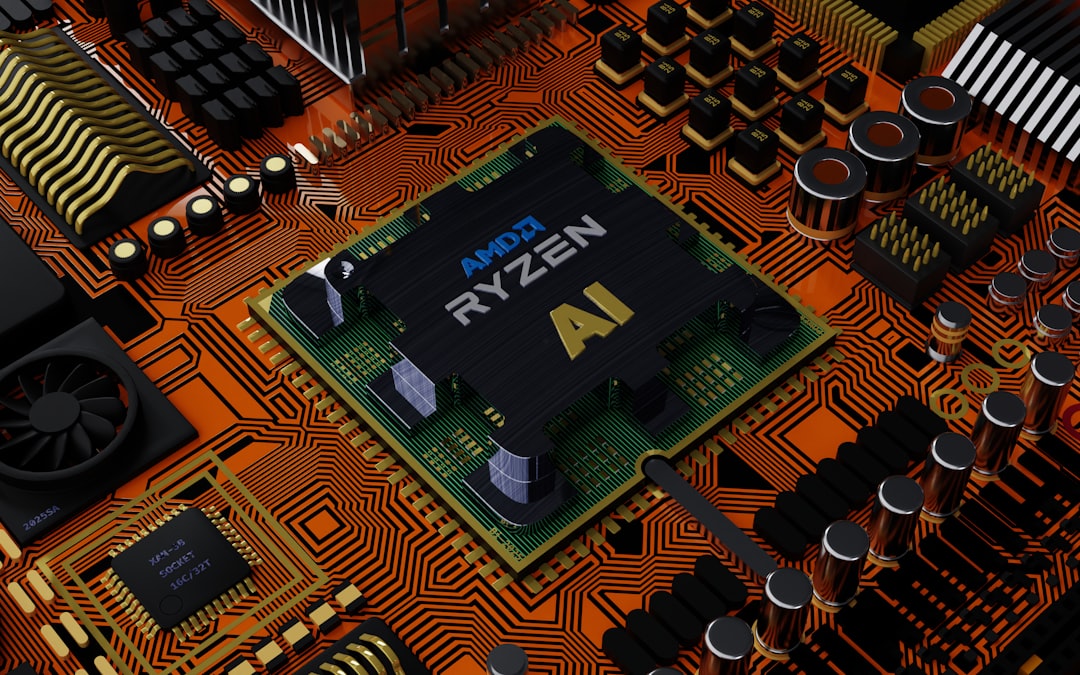
The arrival of 2016 witnessed a groundbreaking shift in Google Translate's capabilities with the introduction of Google Neural Machine Translation (GNMT). This revolutionary technology, based on deep learning principles, brought about a significant improvement in the quality and fluency of translations. Unlike previous systems that relied on statistical methods to analyze phrases, GNMT adopted an approach akin to the human brain, processing entire sentences to grasp context and produce more natural-sounding translations. This innovation even enabled zero-shot translation, meaning that translations could be produced between language pairs that had not been explicitly trained together. As a result, Google Translate's reach expanded to support over 100 languages, making it an invaluable tool for global communication. However, despite these advancements, GNMT still faces limitations, particularly with high computational demands and the challenges posed by rare words. While machine translation has made remarkable progress, it's essential to acknowledge that it still falls short of human-level accuracy, especially when dealing with complex or nuanced translations. As we move into 2024, the profound impact of neural networks in revolutionizing machine translation remains a defining factor in the ongoing evolution of Google Translate.
The year 2016 marked a turning point in machine translation with the arrival of Neural Machine Translation (NMT). The shift from statistical models to neural networks brought a dramatic improvement in translation speed. Instead of painstakingly processing chunks of text, NMT models analyzed entire sentences, capturing the nuances of language in a way that was previously unimaginable.
It's fascinating to see how these models learn from vast amounts of data, mimicking the human brain's ability to decipher context. They're capable of processing the entire sentence, taking into account its structure and meaning, unlike their earlier counterparts which treated sentences like a collection of isolated words. It's almost like they're learning to think like humans!
The results speak for themselves – NMT achieved a significant leap forward in translation quality, outperforming previous statistical models by up to 60%. This improvement is not simply a matter of better word choices, but a more holistic understanding of the source language and its translation into the target language. This progress was driven by the "sequence-to-sequence" learning approach, enabling the models to truly understand the relationships between words and phrases within a sentence.
The introduction of attention mechanisms further revolutionized NMT. These mechanisms allow the models to focus on specific parts of the source sentence when translating, much like humans do when reading and interpreting a text. It's like giving the models a special tool to zero in on important words and phrases, leading to more precise and accurate translations.
As a researcher, I'm incredibly excited about the potential of NMT. This technology has democratized access to information, breaking down language barriers and making the world a more interconnected place. However, it's important to remember that even the most advanced AI systems are not perfect.
NMT systems can inadvertently absorb biases from the data they're trained on, potentially perpetuating harmful stereotypes or cultural biases. This highlights the importance of ongoing research and careful oversight to ensure that NMT tools remain fair and equitable. The evolution of Google Translate, and machine translation in general, is a story of continuous improvement and ethical challenges. It's a testament to the power of AI to connect people and to the responsibility we bear to ensure that its development benefits everyone.
The Evolution of Google Translate From SMT to Neural Machine Translation in 2024 - LSTM Networks The Brain Behind Google's NMT System
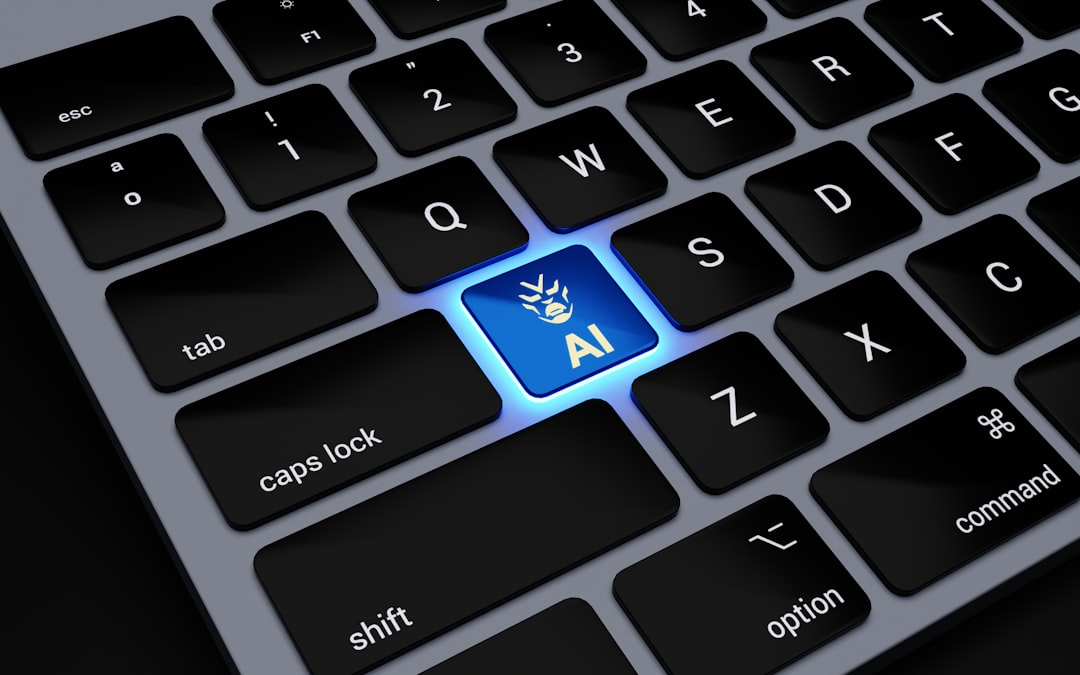
LSTM networks are the foundation of Google's Neural Machine Translation (NMT) system. They allow Google Translate to process language in a way that is much closer to how humans do, unlike the statistical models that came before. This means more natural and accurate translations, but it comes at a cost. The computational resources required to train and run an NMT system are immense. And while they are great at handling common words and phrases, NMT struggles with rare words. The switch to NMT represents a crucial step forward for Google Translate, but there is still work to be done. We can expect to see continued improvements in 2024, with developers striving for better translation accuracy and less reliance on expensive computing power.
LSTM networks are the hidden power behind Google's Neural Machine Translation (NMT) system. This type of neural network, also known as a Long Short-Term Memory network, excels at handling sequence-based data, making it perfect for tackling the complexity of languages. It's like giving the NMT system a powerful memory. LSTMs are able to remember past information – what happened before in a sentence – allowing them to understand the context of each word. This is particularly important for languages that have complex grammar, where the meaning of a word can change depending on its position in the sentence.
LSTMs achieve this by using specialized cell states that act like tiny storage units. These units selectively remember relevant information from the past and discard what's not needed anymore. It's like a filter that ensures only the essential information makes it into the model's "memory". This "forgetting" ability allows LSTMs to focus on what matters, making the translation more accurate and relevant.
Google Translate's NMT system, heavily reliant on LSTMs, goes beyond simply translating sentences word by word. It uses LSTMs to learn patterns not just within a single sentence, but across entire documents, allowing it to grasp the full context of a translation. This means that Google Translate can now produce translations that are more nuanced and closer to the intended meaning.
LSTMs are incredibly powerful, but they come with a price tag. They require massive computing resources to train and run. So, the cost of developing and deploying LSTMs can be high. While these complex algorithms are essential for the accuracy of NMT, it's worth considering the trade-off between efficiency and cost.
A fascinating aspect of LSTM-powered NMT is its ability to incorporate stylistic elements from the target language. While previous systems often produced translations that sounded robotic and generic, LSTMs can generate translations that flow more naturally, mimicking the rhythm and style of the source language. This is a big improvement for users, making the translations more enjoyable and easier to understand.
LSTMs have also drastically sped up translations. NMT systems using LSTMs can perform translations up to ten times faster than older systems, a game-changer for anyone who needs quick translations. Imagine having near-instantaneous access to information in any language, making communication a seamless experience. This speed is critical for tasks like business negotiations and real-time travel communication.
LSTMs allow NMT systems to understand the nuances of word meanings based on context. This means that the model can better handle ambiguity and provide a more precise translation. Imagine how challenging it would be to translate a sentence like "The bat is hanging from the ceiling." LSTMs can use context to figure out whether "bat" refers to the animal or the piece of sporting equipment, eliminating confusion.
Even OCR technology, which allows users to translate images of text, benefits from LSTMs. LSTMs help the NMT system recognize and decipher handwritten text, which can be difficult to translate.
Despite all these advancements, the world of NMT isn't perfect. There are still areas where human translators outperform machines, like capturing subtle meanings and interpreting idiomatic expressions. While AI is getting increasingly sophisticated, there is still a long way to go before it reaches human-level accuracy.
However, the impact of LSTMs on Google Translate and other areas is undeniable. These networks have revolutionized how we translate text, making it faster, more accurate, and accessible to everyone. Their use has extended beyond simple text translation to other fields, like customer support automation and web content translation. It's clear that the capabilities of LSTMs are vast and their impact on how we interact with language will continue to evolve in exciting ways.
The Evolution of Google Translate From SMT to Neural Machine Translation in 2024 - Overcoming Rare Word Translation Challenges with NMT
Google Translate, in its evolution from rule-based systems to Neural Machine Translation (NMT), has made remarkable strides. While NMT offers a more nuanced approach to language, a persistent challenge remains: rare words.
Despite their ability to understand context and process language holistically, NMT models struggle to translate infrequent words, often resorting to a generic "unknown" token. This is especially problematic for low-resource languages, where limited training data makes it harder for models to learn these rare terms.
The rare word problem highlights the ongoing complexities of machine translation. Researchers are working on solutions, like methods to track the origins of rare words. However, the accuracy and overall utility of AI translation tools remain intertwined with the ability to effectively handle these rare terms. The transition from SMT to NMT represents a significant step forward, but the pursuit of truly seamless translation continues.
While neural machine translation (NMT) has revolutionized translation by mimicking human language processing, it still faces challenges, especially with rare words. NMT models are trained on massive amounts of text data, but this data rarely includes enough examples of rare words to make accurate translations.
Researchers are working on ways to overcome this limitation. One promising approach involves breaking rare words down into smaller, more common subword units using techniques like Byte Pair Encoding. This allows NMT models to handle rare words more efficiently while still preserving their meaning.
Another strategy is transfer learning, which uses knowledge from high-resource languages to improve translations for low-resource languages. This helps NMT systems handle the often sparse vocabulary found in languages with limited online resources.
Researchers are also exploring multi-task learning, which trains NMT models on related tasks such as language modeling alongside translation. This helps them develop a deeper understanding of context, which can aid in handling rare words.
A key aspect of this research is the use of rich contextual embeddings like ELMo and BERT, which provide a deeper understanding of words in different contexts. This helps NMT systems handle the varying meanings of rare words within a sentence, leading to more accurate translations.
Domain adaptation is another promising technique that allows NMT systems to specialize in specific areas, such as medical or technical fields, by providing targeted training data for rare words specific to those domains.
Many modern NMT systems incorporate user feedback to continuously adapt and improve their translations, particularly for rare words frequently encountered by users. This creates an ongoing feedback loop that enhances the system's reliability over time.
While Optical Character Recognition (OCR) technology allows us to translate text from images, it can also introduce errors when dealing with rare words, as they may not have sufficient representation in OCR databases, adding another layer of complexity to the translation process.
Evaluating the performance of NMT systems for rare word translations relies heavily on specialized metrics like BLEU and METEOR, but these may not adequately capture the challenges posed by infrequent vocabulary, potentially overestimating accuracy.
Ultimately, addressing rare word challenges in NMT often involves a trade-off between computational resources and translation quality. High-resolution models capable of processing rare words require significant investment, raising concerns for cost-effective solutions in the translation industry. The quest for improved translation accuracy continues, with researchers seeking innovative ways to bridge the gap between human and machine capabilities in the realm of rare word translations.
The Evolution of Google Translate From SMT to Neural Machine Translation in 2024 - The Computational Cost of Neural Machine Translation
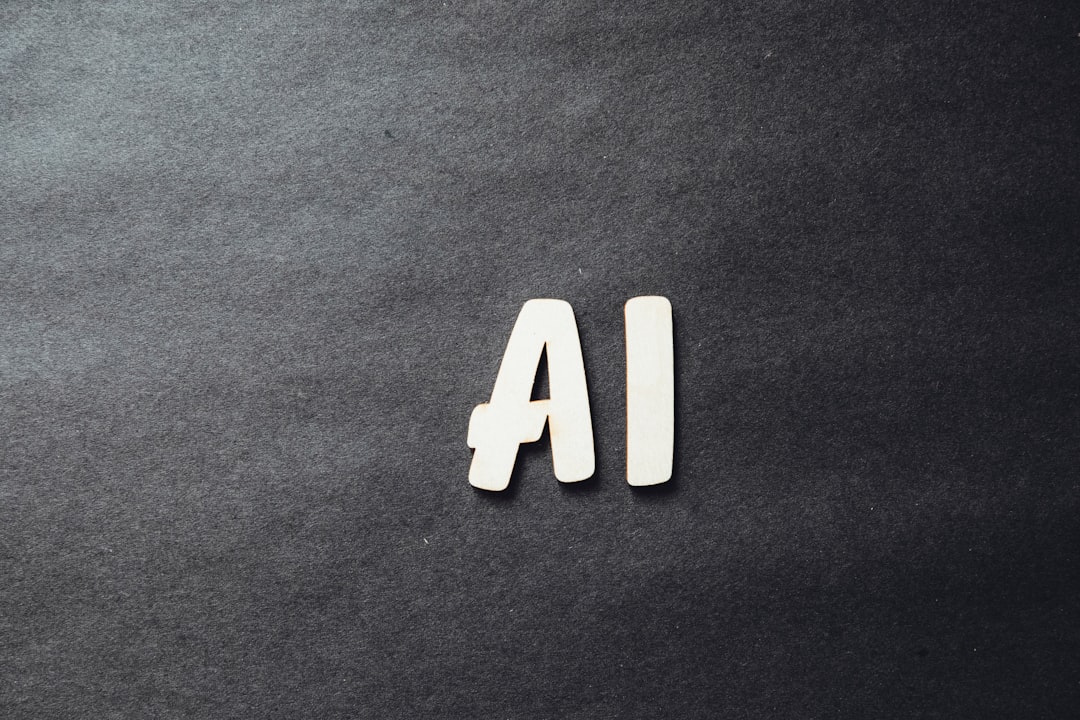
Neural Machine Translation (NMT) represents a significant leap forward in the evolution of Google Translate. Its ability to process language in a way that mimics the human brain, considering context and producing more natural translations, is a game-changer. However, this advancement comes with a hefty price tag.
The computational cost of NMT systems remains a major hurdle. Their sophisticated architectures, like Long Short-Term Memory (LSTM) networks, require immense processing power, both for training and for real-time translation. This demand for resources presents a challenge, especially for scaling translation services to accommodate a wider range of languages.
The computational intensity of NMT also introduces a trade-off. While the technology delivers exceptional results, particularly with common phrases and words, it still struggles with rare terms. The quest for more efficient NMT systems that can handle both common and uncommon language with greater accuracy and less dependence on expensive computing resources will continue to be a key focus in the years to come. Striking a balance between computational cost and translation quality will be essential for the future of AI-powered translation technologies.
The computational power needed for Neural Machine Translation (NMT) is a double-edged sword. NMT is, in theory, closer to human translation with its contextual understanding and ability to process language holistically. However, this comes with a price tag; NMT requires a huge leap in computational power compared to the statistical models that came before. This translates to needing more hardware and energy, which drives up costs.
NMT uses Long Short-Term Memory (LSTM) networks to "remember" information from the previous words in a sentence. This is like human memory, allowing for a more nuanced translation. But, you guessed it, this memory management also requires more computational power.
We're constantly encountering limitations with NMT. While it's improved, training NMT systems relies heavily on training data, and for low-resource languages, there simply isn't enough of it. This means that translation quality for languages with less available data often falls short.
On the other hand, we've made significant progress with NMT's ability to translate language pairs that it hasn't explicitly trained on, a technique called "zero-shot translation". This shows us how much we've learned about how language structures work.
The "attention mechanism" is another major development. Instead of treating a sentence like a block of uniform text, NMT now focuses on the relevant parts of a sentence. This makes a big difference for complex sentences and is a key factor in improving accuracy.
NMT has sped up the translation process, enabling translations up to 10 times faster than before. This is important for real-time translation needs like conferences and travel.
However, there are still some major hurdles to overcome. One is the problem of rare words. NMT struggles to translate uncommon terms, and often resorts to a placeholder, which can greatly reduce translation quality, particularly in specialized fields.
To tackle this, we're using techniques like "subword tokenization" to break down rare words into smaller parts, allowing models to handle them more effectively.
We're also incorporating user feedback into NMT systems to improve translation quality over time. This helps continually improve translation quality, especially for dynamic fields where slang and new terms evolve rapidly.
The ongoing struggle in NMT development is striking a balance between cost and accuracy. Models that can handle rare words well often require a massive investment in computational resources, which can be cost-prohibitive for the translation industry.
It's clear that NMT is a revolution for translation, and its evolution is ongoing. While it's impressive, we still face many challenges in perfecting it. The quest for a truly seamless translation continues.
The Evolution of Google Translate From SMT to Neural Machine Translation in 2024 - Zero-Shot Translation Google's Next Frontier in AI Language Processing
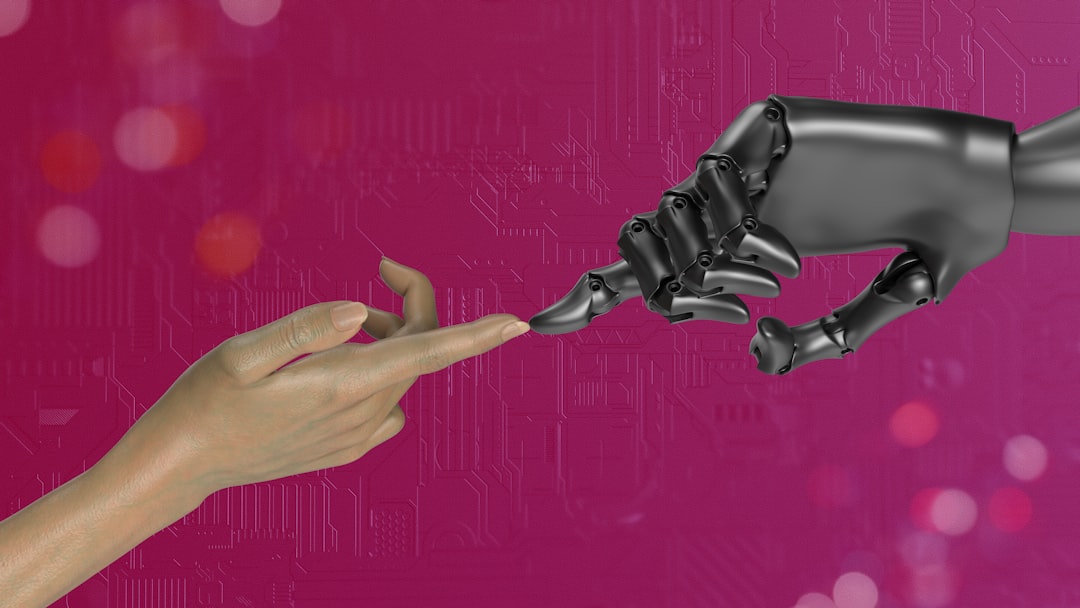
Google Translate has reached a new milestone with the introduction of zero-shot translation, marking a significant leap in their AI language processing capabilities. It now translates between languages that haven't been explicitly trained together, dramatically expanding its coverage to nearly 200 languages. This innovation utilizes a multilingual neural machine translation (MNMT) system, enabling Google Translate to bypass the need for extensive localized training data. A unique token, inserted at the beginning of the input sentence, indicates the desired target language, simplifying the translation process. This signifies a departure from traditional methods that heavily relied on parallel texts, demonstrating Google's commitment to a more efficient and accessible translation experience.
Despite these impressive advancements, the pursuit of flawless machine translation remains an ongoing journey. Challenges persist, particularly in translating rare words and capturing subtle nuances in language. While Google strives to enhance zero-shot translation, the delicate balance between accessibility and linguistic accuracy remains paramount.
Google's latest foray into AI language processing, Zero-Shot Translation, marks a fascinating step forward. It allows the system to translate between language pairs that it hasn't been specifically trained on, relying on its understanding of related languages. This showcases how robust the model's context comprehension has become.
However, it's still a work in progress. While it's great at handling the structure of a sentence, it stumbles over rare words, especially in languages with limited data. This highlights the constant struggle to achieve perfect translations.
Researchers are utilizing techniques like Byte Pair Encoding to break down rare words into smaller units. This clever trick allows the model to make sense of these unusual terms, leading to better overall translations.
Another important advancement is the "attention mechanism" – a clever way for the model to focus on important parts of a sentence, reducing errors associated with word order and meaning. This has been crucial for making translations more accurate.
But this evolution hasn't been without its challenges. The sheer computational power needed to train and run these models is immense, making it tricky to scale these tools for more languages. We need to figure out ways to make them run more efficiently on smaller machines.
On the bright side, NMT has made translations blazingly fast, up to ten times faster than before. This is essential for real-time translation, like conference calls and online chats.
What's interesting is how these models can learn from the feedback they get from users. This helps them adapt to the real-world use of language, making them even more accurate over time. But, relying on user data does raise concerns about data integrity, so rigorous quality control is a must.
Researchers are also experimenting with "multi-task learning", which teaches models a range of related tasks, like summarizing text or analyzing sentiment. This is meant to improve their understanding of context, helping them handle tricky sentences.
Of course, we can't forget about Google's OCR technology. While this lets users translate text in images, it's not perfect. Handwritten text and complex layouts can throw off the system, making translations less accurate.
All this points to the incredible progress being made in machine translation. But, the journey isn't over yet. There's still a long way to go before we achieve truly flawless, human-level translation.
More Posts from aitranslations.io: