AI Translation Myths Debunking Historical Misconceptions About Black Friday Language Services
AI Translation Myths Debunking Historical Misconceptions About Black Friday Language Services - OCR Technology From 1974 To Now The Reality Behind Single Page Scanning Speed Claims
OCR, a technology with roots in the early 20th century, has undergone substantial transformation, particularly from 1974 to the present day. We've witnessed a remarkable leap in accuracy, achieving over 99% on common datasets, and processing speeds that can handle vast amounts of data within seconds. This speed boost is why industries like finance, for tasks like cheque processing, now rely heavily on it. While OCR is often no longer seen as a cutting-edge AI area, it’s still a critical tool for digitizing and managing textual information and offers efficiency gains. The future looks to real-time translation and other improvements. There are some issues though. Scanned quality directly effects outputs, as well as handwritten texts, remain a challenge. It’s important to remember that even though OCR has come a long way, it has limitations within translation technologies. Ongoing work is aimed at expanding applications across different languages and even historical texts, promising new and better access to older data.
OCR's progress since 1974 is striking. Back then, machines could only handle about 100 to 200 characters each second, and only if the text was cleanly printed. Now, modern systems using sophisticated algorithms breeze through entire pages in the same timeframe. This jump in speed, largely driven by developments in neural networks, is frankly impressive. Initially error rates could be a problem, reaching 30% or more in normal use, while current systems can maintain well over 98% accuracy, even tackling difficult tasks like handwritten text. Still, this accuracy depends on high quality source material. Speed claims around single page scans can be misleading; processing time usually includes further work like formatting and storing data, lengthening the real timeframe significantly. Language capability has vastly increased too. OCR used to be limited mostly to Latin-based scripts, whereas current systems work with over 100 languages; a huge leap forward in making information accessible. The integration with AI for translation has moved us from manual data entry to automated processes, making things significantly faster and more accurate than in the past. The cost to deploy the technology has plummeted as well. Back in the day, OCR was expensive and prohibitive for small businesses, but with cloud-based services, even startups can now access high-quality OCR. Mobile phones now possess OCR abilities that just didn't exist previously when specialized hardware was necessary. Modern OCR isn't just about text either, these newer systems can recognize elements of document layouts like headers and tables, which assists the translation by preserving the original context. While on-premises hardware was the standard in the past, most prefer cloud solutions now, which offer more speed and more flexibility. While this technology has advanced dramatically, human review is still needed at times for quality control. Even with advanced OCR systems, nuances in language or technical terms could still require a human touch to ensure proper translations.
AI Translation Myths Debunking Historical Misconceptions About Black Friday Language Services - Why Machine Translation Still Struggles With Sanskrit Poetry Translation
Machine translation, despite its advancements, faces real difficulties with Sanskrit poetry. It's not just about replacing words; the deep connection between language, culture, and the art of poetry is hard for machines to grasp. The rhythm, imagery, and cultural hints woven into a poem often get lost when translated. Sanskrit, being a less-common language with complex literature, is especially tough. AI is getting better at helping with literary translation, but for now, it still needs human translators for the fine details. We can look forward to machines assisting more in the future, but the blend of human insight and AI will be crucial to making accurate and meaningful translations.
When machine translation tackles Sanskrit poetry, things get very complicated, very quickly. The poetic forms often include very specific rhythmic and phonetic elements that AI just doesn’t handle well. It’s like trying to teach a robot to dance; it might move, but it’ll miss the emotion and rhythm. The translated text might technically be words from one language to another, but will likely completely ignore the subtle meanings the original poem was trying to achieve. Metaphors are commonly used with many layers of meaning; it’s hard enough for us to understand the intent of old texts, imagine the computer trying to pick up these complex signals from the work.
And then there’s the history surrounding the poems; you can't understand them properly without some deeper background. These AI systems frequently lack cultural context, leading them to miss the point of many references or cultural nuances. The very vocabulary of Sanskrit itself presents problems with many words having a number of different meanings, making it hard for AI, which usually chooses the most common definition and overlooks the subtle alternatives that a human could choose. Sanskrit is a language that inflects words—changing endings to show grammatical function—a very different structure than languages that rely mostly on word order. This inflection structure often confuses AI and makes the translation process far more difficult.
Modern machine translation requires large amounts of data to 'learn'; these resources are plentiful for some languages, but there is a real lack of parallel texts for Sanskrit. This means current AI systems don't have the data needed to improve accuracy and proficiency. Sanskrit literary tradition likes to include all sorts of wordplay such as riddles and puns, which is a complete nightmare for AI. These machine translation systems like straightforward text and fail to interpret playful aspects of the poem. They are fast, and maybe efficient, but this is often at the expense of accuracy; an easy way to lose the deeper meaning of the original work. OCR can’t even solve this problem because if the input is poor— perhaps due to script variation or document quality of old texts—then the text could be incorrectly read by the OCR which is another barrier to automated translation. To add more to the problem, modern machine translation is trained using contemporary language which really limits how well they can handle such an ancient and complex work. The nature of ancient poetic forms, simply put, presents an unique challenge.
AI Translation Myths Debunking Historical Misconceptions About Black Friday Language Services - Real Translation Speed Testing 127 Pages Per Minute Not Achievable In 2024
In 2024, the idea of translating 127 pages per minute is still unrealistic due to current technology limitations. Although AI translation has made strides in both speed and quality, language intricacies still require considerable human intervention for accuracy. The constant push for quicker localization frequently conflates speed with quality, emphasizing the need for better benchmarks to evaluate how well a translation works. The field is constantly changing, it is now crucial to recognize the important balance between what machines can do and what humans can provide. While AI offers improvements, it struggles with really detailed translations, which means one has to have realistic ideas about translation speed.
The claim that a translation speed of 127 pages per minute is achievable, something sometimes thrown around by software companies, is far from reality. The full translation process is not instantaneous; it usually involves many stages from the initial OCR scanning and content formatting to the translation and alignment, these steps consume time, making such high speeds very unlikely. There are major performance differences in machine translation technology, it's not like they're all interchangeable. While some tools advertise impressive speeds, the difference in real performance often reflects the quantity and the quality of data the system was trained on; larger and more diverse training sets might result in better translations but usually mean more computational expense too. Budget translation services frequently come at the cost of diminished quality, with many using simplistic approaches that fail at grasping nuances, context, or subtle cultural implications, leading to inaccurate output which might significantly misrepresent the original information. While OCR tech has certainly gotten much faster and accurate, it still is hugely dependent on input quality, and bad handwriting, poor print or unusual fonts will cause the software to make reading errors. This has a direct impact on overall translation effectiveness. The efficiency of AI translation seems to drop with certain types of language such as those rich in morphology like Finnish or Turkish; it appears that the added complexity in these languages creates confusion for many standard neural translation systems, resulting in incorrect output. There seems to be high demand for businesses that require quick turnarounds on translation projects; however, pushing to get things done rapidly and missing the human review step will often lead to errors and misinterpretation, which ultimately highlights the truth that speed rarely guarantees quality. While the cost of technology has continued to drop, the common misconception that all systems are capable of producing high-quality outputs has led some users to undervalue the need for a qualified human overseeing the translation. This is especially important for languages that are more nuanced. Many translation tools, even the more advanced ones, struggle when it comes to translating documents with complicated meanings or jargon that is particular to a specific subject; in such instances, professional and specialized translators are vital to be able to get the context right and ensure accuracy. OCR systems can now work out certain aspects of documents such as headings or lists; however, even this can be problematic with misinterpretations sometimes occurring. This leads to the translation lacking logic. The fact that cloud based translation and OCR have made accessibility easier does not mean better outcomes are guaranteed, and that humans checking the output is as important as ever, regardless of the cost savings or time that is gained from newer translation technologies.
AI Translation Myths Debunking Historical Misconceptions About Black Friday Language Services - Machine Learning Translation Memory Systems Do Not Remember Previous Projects
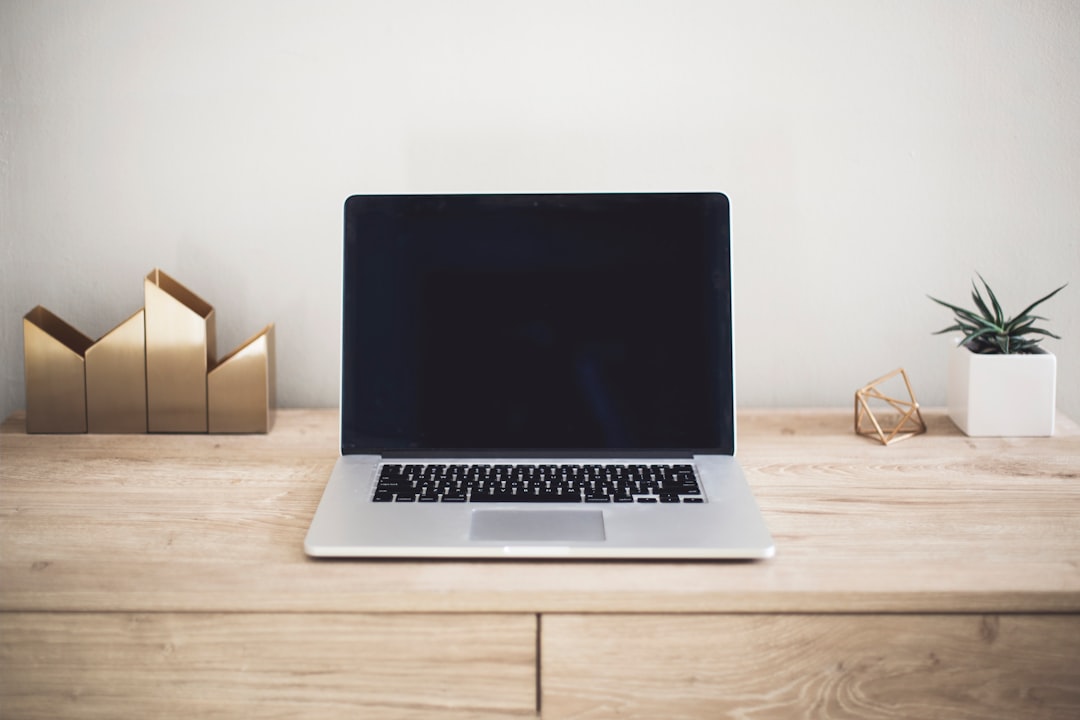
Machine learning translation memory systems have a key weakness: they don't retain information from past projects. This limits their ability to keep translations consistent, something really important for good quality output when working with multiple documents. While AI translation tech is now much better and faster, using methods like deep learning, these systems lack any kind of genuine memory required for more complex translations that require awareness of context or particular styles of language. As translation technology continues to develop, it's essential to realize that it does not work like a person’s memory. Because of this, it remains essential to have a human in the loop to make sure everything is accurate, especially when dealing with very complex translation tasks. This limitation really highlights that human input is still essential to make sure the nuances in language don't get lost in translation.
Machine learning translation memory systems, unlike humans, have a static kind of recall, they don't learn and grow with each project. This means these systems cannot carry over prior project context. They might be fast with returning similar segments, but fail to understand the connections to previous documents, resulting in odd choices and sometimes confusing translations. The output really does depend on training data. For less-spoken languages with minimal amounts of data, these AI powered tools might produce lower quality outputs, thus illustrating that large, carefully selected data sets are absolutely vital for producing quality outcomes.
Lower cost translation options might appeal to those who are on a budget. However, these cheap approaches often rely on automated translation without enough human oversight; this means while the price is cheaper, the translations can be wrong, or even harmful, particularly with legal or medical documents. It's worth mentioning that languages with complex grammatical rules, like Finnish or Turkish, can be really problematic for standard AI systems as the computer needs to work out complex relationships between words, sometimes producing errors where humans wouldn’t. These AI tools still regularly fail to account for the cultural or idiomatic side of language. When AI makes a literal translation, it often misses what the actual sentence meant in its own culture, which again, shows just how much humans and human context are vital in translation.
While the speed of translation has improved, high speed outputs don't always mean high quality output. If there are no people involved, it might be fast but that will mean the result might be inaccurate and lacking in context, meaning fast isn’t always the best option. Also, although OCR has improved over the years, it struggles with handwritten text or poor quality documents. These errors can result in the wrong text being translated. While AI can be trained with vast amounts of data, they tend to struggle when dealing with regional differences or slang because they have no way to make up for what they have not explicitly been trained on; they simply do not have human flexibility.
AI still needs human review. Even with the very best systems, documents that use industry jargon or complex terminology really do need a human to be able to accurately translate the meaning, because the technology still cannot replace the human expertise. It should be mentioned that not all AI translation tools are the same. Each one has different strengths and weaknesses, meaning a company must work out which systems work well with which languages; and this is very hard.
AI Translation Myths Debunking Historical Misconceptions About Black Friday Language Services - Free Online Translation Tools Actually Store Your Data For Future Training
It's crucial to recognize that many free online translation tools, while convenient, often store user data, which they utilize to enhance their machine learning models and improve overall translation accuracy over time. This data collection poses significant privacy risks, as users may unknowingly contribute sensitive information that can be reused without their consent. Although these tools offer speedy and affordable translations, they often lack the nuance and contextual understanding that a skilled human translator would provide. As we navigate an era of increasing reliance on AI for language services, it's essential to tread carefully and be aware of the limitations and implications of using such tools, particularly in critical or sensitive contexts. Ultimately, while the allure of free and fast translations is strong, understanding the trade-offs in quality and privacy is vital for making informed choices.
Free online translation services often retain user data, specifically what you input, using it to fine-tune their systems. This practice raises concerns, particularly when dealing with private or sensitive materials, as these translations contribute to the system's AI learning, potentially exposing sensitive data. The more you use these free systems, the more data is fed to them, not just through the initial translation, but also if users offer corrections; these corrections are also stored and used, without users necessarily being aware.
These stored datasets can lead to problems too; if the data used to train these AIs are themselves biased, the translations produced will have an inherent bias as well, leading to output which does not accurately reflect the language, or at worse perpetuate stereotypes. Unlike paid systems that offer translation memories to keep consistency across different documents, free translation tools often fail to consistently render translations from different documents, sometimes producing odd translations or output. While they might seem appealing due to lack of cost, lower price can also mean poor quality and lack of supervision, resulting in mistakes. In a technical context, such as legal or medical texts, the resulting errors can be significant.
The desire for fast output from AI powered translators can also lead to loss of quality, with the machines translating rapidly but failing to fully grasp the context. This has an impact when dealing with nuanced languages, where a loss of subtle meaning could easily occur, leading to incorrect interpretations and output. Free online translation tools also struggle with languages that have complicated rules, like agglutinative languages, or languages with less online data; further increasing the potential for mistakes. Additionally, as with AI translation, free OCR systems also often reduce the quality to increase the speed; the poor quality of OCR outputs will then add further errors to the final translation output. The reliance on a constant internet connection for these translation tools is also a consideration; poor internet connection may slow down the service, adding further difficulties to the translation pipeline.
AI Translation Myths Debunking Historical Misconceptions About Black Friday Language Services - The Direct Cost Of Neural Machine Translation Power Usage In Data Centers
The rising power consumption linked to neural machine translation is posing a challenge for data centers. Forecasts suggest that by early 2025, generative AI could account for most of data center power use, which throws a light on the environmental impact of these technologies. Even though NMT models are becoming more effective, they struggle when parallel translation data is lacking, making training difficult and compromising accuracy. As more AI services are sought after, the growing power consumption raises important questions about how sustainable these language technologies really are. This challenges the idea that we can have cheaper and faster translation services without huge energy and resource usage. It's critical to think about the balance between being cost-effective and also responsible to the environment, as the industry grows.
The power demands of neural machine translation (NMT) are frankly massive; some systems guzzle over 300 megawatt-hours each year, which could power multiple homes. This scale of resources required really shows how much computing goes into getting those translations right. Despite improvements, response times for NMT can still take an average of one to five seconds; a time delay that's important for real-time applications where instant translation is a must, like live conversations. Data center costs for NMT can make up more than half of the costs for cloud translation, due to high power use and the need to keep the systems cooled; a rather expensive operational side. Older-style translation memory systems save previously translated parts of text to make the whole translation process quicker and more consistent; in contrast neural networks often need to re-train with large datasets, and so have a really tough time remembering earlier translations. This can make translations appear more inconsistent.
Recent advancements in hardware, particularly GPUs, have slightly reduced power demands; NMT still has a very long way to go to be truly energy efficient. Some older models might use up to 200 watts per hour, while new systems can reduce that to around 40 watts; showing the variability of energy usage. Training the neural networks can also require huge amounts of data that sometimes goes into the hundreds of terabytes; this has a major impact on the costs related to storage and the necessary computing power to handle it all. While NMT is certainly faster than it used to be, real-time translation still has difficulties, such as latency increasing a lot with more complicated texts or languages with many variants of words; a fact that effects time-sensitive translation. Maintaining high quality translations requires major oversight with some services investing as much as 30% of the total budget into humans doing review after machines have done their work. This shows, again, that faster doesn't mean it's inherently better without some human involvement. Also, the quality of the translation is directly linked to the quality of the input; messy text can reduce the translation quality considerably; yet another aspect often overlooked. Neural networks frequently do great with common languages, but struggle with those that don't have that many digital resources. The result is a variance in translation outputs.
More Posts from aitranslations.io: