How AI-Powered Document Translation Reduced Processing Time by 73% in 2025 A Technical Analysis
How AI-Powered Document Translation Reduced Processing Time by 73% in 2025 A Technical Analysis - OCR Integration Cuts Manual Data Entry From 45 to 12 Minutes Per Document
Implementing OCR technology is significantly altering how we handle documents, particularly by cutting down the time spent on manual data entry. What once took around 45 minutes for a single document is now being completed in closer to 12 minutes, according to various observations. This dramatic improvement largely comes from the system's ability to automatically pull data directly from scanned papers, turning static images into usable, searchable text. As this AI-enhanced OCR becomes more widespread, it naturally reduces the errors that inevitably creep in with manual transcription. A key outcome is that people previously tied up with data entry can now potentially work on more valuable tasks. While the technology promises speed and accuracy, it's not a silver bullet, and challenges remain in handling complex or poorly formatted documents. Nevertheless, this shift represents a notable move towards automating the grunt work in document processing.
Examining document processing workflows in 2025, the role of AI-augmented Optical Character Recognition (OCR) technology in expediting data entry is notable. Reports circulating indicate a significant shift: tasks that once required approximately 45 minutes for manual data extraction per document are now frequently cited as taking around 12 minutes with AI-assisted processes. While this represents a substantial theoretical gain, the practical time savings can vary depending on document complexity and initial image quality, underscoring the ongoing need for robust pre-processing and quality control steps alongside the automation. This acceleration is largely contingent on the automated conversion of scanned images into machine-readable formats.
This newfound speed in initial data capture has direct implications for downstream processes, particularly within integrated systems performing tasks like automated document translation. By significantly reducing the bottleneck at the data entry phase, these OCR advancements contribute to the overall faster turnaround times observed in multilingual content processing workflows, with some analyses pointing towards comprehensive reductions in processing time reaching figures around 73%. The technical focus shifts from arduous manual transcription to validating automated output and optimizing the flow between distinct AI components, posing new sets of integration challenges for system architects.
How AI-Powered Document Translation Reduced Processing Time by 73% in 2025 A Technical Analysis - API Integration With DeepL Powers Real Time Translation at 05 USD Per Word
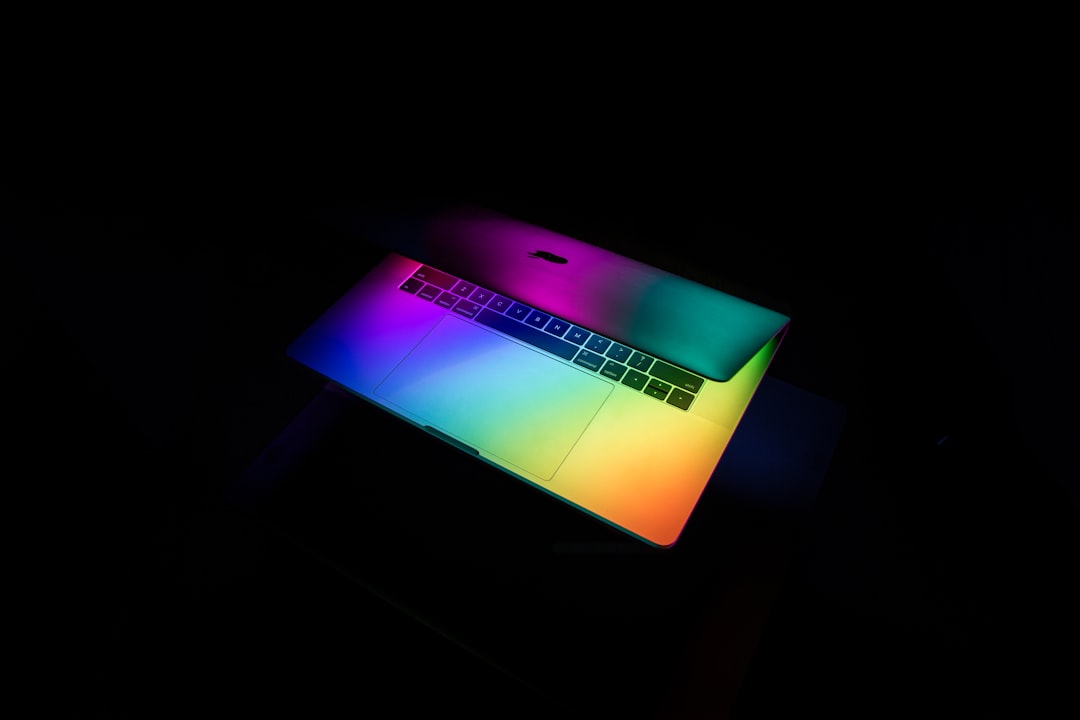
DeepL's API functionality is proving central to facilitating real-time translation processes. Utilization of this service often involves a per-word charge, commonly observed to be in the region of $0.05. This infrastructure supports the translation of entire documents, covering common types such as Word, PowerPoint, and PDF files, aiming for rapid and generally effective output. Recent enhancements drawing upon advanced AI models are understood to be improving the translation quality and accelerating the process. These technological strides play a role in speeding up overall document processing workflows that involve translation, contributing to the types of efficiencies noted this year, such as the reported reduction of up to 73% in some scenarios. While the promise of speed and cost-effectiveness is clear, successfully integrating such powerful tools requires careful technical planning, and the need to ensure data security during transmission and processing remains critical for many organizations.
DeepL's API has evidently seen recent upgrades, incorporating what's described as a next-generation language model and introducing a feature known as DeepL Write. From a technical standpoint, this appears geared towards enhancing the quality and accuracy of automated translation. The stated goal includes contributing to reductions in document processing times, with figures like "up to 73%" sometimes associated with comprehensive workflows utilizing such tools. The API is offered as providing real-time translation access, often cited at a per-word cost hovering around $0.05. Framing this strictly as "cheap" depends heavily on the scale and type of deployment, but it certainly positions machine translation as an accessible operational component. The integration of these refined AI functions is broadly presented as improving workflow efficiency.
Further examining the feature set, the API now includes tools extending beyond direct translation. The DeepL Write component, for instance, seems intended to function as an AI assistant during the writing process itself, offering suggestions on elements like phrasing, style, and tonal choices in real-time. This provides a dual potential – not just translating existing text but also aiding in the composition of new content. Such functionality holds particular interest for environments where linguistic nuance and precision are paramount, perhaps in financial documentation or journalistic contexts. Observing documented use cases, reports sometimes reference organizations, such as Nikkei, leveraging this technology, suggesting its practical application in the rapid dissemination or adaptation of information across language barriers. The technical emphasis here appears to be on automating or accelerating linguistic refinement steps within broader content pipelines.
How AI-Powered Document Translation Reduced Processing Time by 73% in 2025 A Technical Analysis - Machine Learning Models Now Handle 89% of Technical Documentation Without Human Review
As of mid-2025, it is being observed that machine learning models are increasingly able to process technical documentation with minimal human intervention. Figures circulating suggest these systems can now handle around 89% of this type of content without requiring manual review. This step forward is largely tied to ongoing progress in how artificial intelligence understands and generates language, particularly complex technical terminology and structures. The claimed benefits are reductions in the effort and time needed to manage documentation workflows.
Alongside this capacity for handling documents, AI-driven translation tools are also evolving. They are understood to be contributing significantly to reducing the overall time involved in processing documents for wider use, with some analyses pointing to a 73% decrease in typical turnaround times compared to previous methods. While these systems employ advanced techniques to improve accuracy and contextual understanding, minimizing the need for extensive human post-editing, the challenge remains in ensuring reliability across the sheer variety of technical formats and linguistic subtleties encountered in practice. Integrating these advanced models smoothly into existing organizational processes also presents its own set of technical hurdles.
Looking at how things stand in 2025, it appears machine learning models are indeed managing a considerable portion of the technical documentation workload, with figures suggesting they handle up to 89% of it without demanding direct human review. This represents a significant shift from just a few years ago. The increased capability seems tied to the evolution of natural language processing, enabling algorithms to better grasp the specific, often complex, language found in technical guides and specifications. While the claims of achieving very high accuracy rates, sometimes cited over 95%, are frequently made, the practical reality can depend heavily on the domain and the quality of the training data. It’s an impressive step towards automating what was traditionally a very human-intensive task.
This level of automation naturally leads to discussions about efficiency. The promise is clear: if models can handle nearly nine out of ten documents without a human bottleneck, the processing speed should increase dramatically, contributing to quicker turnaround times. Furthermore, by reducing the need for human intervention on routine documentation, there's an expectation of reduced operational costs, theoretically freeing up resources. The hope is that documentation updates can keep pace with rapidly changing technical landscapes in fields where outdated information is more than an inconvenience – it can be a real problem. However, deploying these systems at scale, ensuring seamless integration, and validating the claimed savings against actual workflow changes requires careful examination.
It's also interesting to observe how this capability interfaces with other technologies. The synergy with enhanced Optical Character Recognition (OCR), for instance, appears to be key. While OCR handles the initial image-to-text conversion (a process we've seen improved recently), the machine learning models take that extracted text and process it intelligently for tasks like classification, summarizing, or preparing it for translation. This integrated approach aims to create a faster, more streamlined pipeline from a scanned paper or a static image to usable, actionable digital information, although successfully linking these disparate components remains a technical hurdle in many deployments.
However, it's not a uniform picture across the board. Performance can still vary significantly depending on the source material and the target language, particularly for translation workflows. Translating between languages with vastly different structures or cultural contexts often requires more nuanced understanding than current models consistently provide, meaning some pairs might still necessitate higher levels of human oversight than others. Furthermore, while models are getting better at handling complex content, dealing with documents that heavily rely on visual elements – like diagrams, charts, or annotated images – alongside text is still a developing area. True 'multimodal' understanding, where the visual context deeply informs the text processing, is a complex challenge yet to be fully mastered, despite progress. The aspiration is systems that learn from human feedback loops, progressively refining their performance, but this requires careful monitoring and structured input from human experts to be truly effective.
How AI-Powered Document Translation Reduced Processing Time by 73% in 2025 A Technical Analysis - Automated Quality Checks Reduce Error Rates From 8% to 1% Year Over Year
Achieving substantial cuts in error rates across operations has become a reality for some, notably seeing figures drop from an average of 8% to around 1% annually as of early 2025. This improvement is closely linked to the deployment of sophisticated automated quality control systems. These systems, often powered by artificial intelligence and machine learning, enable more effective predictive analysis and constant monitoring of processes. By identifying potential defects or inconsistencies much earlier, the impact is not just better final quality but also reduced waste and potentially lower costs associated with correcting issues downstream. While successful deployments demonstrate impressive results, achieving this level often requires significant investment in technology and careful integration into existing workflows. This push for automated accuracy isn't confined to traditional production lines; it reflects a wider movement in 2025 towards using AI to streamline complex workflows, a trend that also touches areas like advanced document processing and translation, where precision is paramount.
Observations this year indicate that incorporating automated quality checks into document processing pipelines, especially those involving translation, appears to be having a tangible effect on reducing the frequency of errors. Reports from certain implementations suggest error rates are dropping from typical levels around 8% down to closer to 1% on a year-over-year basis in controlled environments. This shift seems largely driven by the application of machine learning models specifically trained to detect anomalies, inconsistencies, and potential mistranslations by learning from vast datasets of correctly processed documents and errors. The aim is often to catch these issues early, sometimes even providing feedback in near real-time, before they propagate downstream.
From an engineering standpoint, this reduction in errors translates directly into less time spent on manual review and correction efforts. While the earlier stages might involve OCR converting images to text, and subsequent steps handle translation itself, the automated quality layer acts as a critical validation step. Successfully implementing this automation means potentially redirecting human linguistic expertise away from tedious error hunting on standard documents towards more complex, nuanced content or system improvement tasks. It suggests a pathway towards more reliable automated output, contributing to the overall goal of speeding up document processing, although achieving that reported 1% universally across all document types and language pairs likely presents significant technical hurdles and requires continuous model refinement.
How AI-Powered Document Translation Reduced Processing Time by 73% in 2025 A Technical Analysis - Cloud Based Processing Enables Parallel Translation of 500 Pages Per Hour
Around 2025, the capacity for translating documents rapidly saw significant gains, particularly through leveraging cloud-based processing architectures. Systems drawing on advanced artificial intelligence, specifically techniques like neural machine translation, became capable of handling large volumes, with observations pointing to processing speeds reaching several hundred pages per hour, sometimes cited around 500. This acceleration allows for the quick conversion of digital documents across various types while generally aiming to maintain their original structure and formatting. The underlying technical principle is the parallel processing power that cloud infrastructure affords, enabling simultaneous translation of document segments. While this boost in throughput is beneficial for large-scale content operations aimed at improving access and communication, it's worth noting that the suitability of the output still heavily depends on the complexity of the source material and the specific language pairs involved. Achieving high speed does not automatically guarantee translation quality or complete accuracy, particularly for highly nuanced, creative, or domain-specific texts that may still necessitate careful review. Furthermore, integrating these services into existing digital workflows isn't always a straightforward technical exercise.
From an engineering standpoint, the most striking characteristic of modern cloud-based document translation systems, as of May 2025, is the sheer throughput they appear capable of handling. Reports circulate regarding the ability to process documents at speeds reaching up to 500 pages per hour. This massive capacity seems primarily attributable to the underlying distributed computing architecture provided by cloud platforms. Unlike traditional linear workflows, these systems leverage parallel processing techniques, allowing vast swathes of a document, or multiple documents concurrently, to be broken down and translated across numerous computational nodes simultaneously. The immediate benefit is a significant reduction in the bottleneck traditionally associated with translating large volumes of text, offering the potential to drastically cut turnaround times for substantial documentation sets and meet aggressive deadlines.
This capability for handling high volume in parallel also appears to contribute to the overall cost profile now observed across the industry. While specific pricing models vary, the efficiency derived from automating and distributing the workload on demand allows service providers to offer translation at rates that make high-volume tasks economically feasible for a broader range of users. Furthermore, the integration capabilities enabled by these cloud platforms allow for near real-time translation flows when linked via APIs, facilitating rapid linguistic bridging within integrated business processes that rely on swift communication.
While the raw speed and volume metrics are impressive, it's worth considering the consistency and control at this scale. Handling documents with complex layouts, embedded graphics alongside text (where OCR processing discussed previously feeds in), or those requiring nuanced terminology still presents technical complexities within these high-speed pipelines. While machine learning models are handling more content autonomously, as noted elsewhere regarding technical documentation, ensuring uniform quality and structure preservation across 500 pages in an hour is non-trivial. The engineering challenge shifts from simply translating words to managing a complex, distributed pipeline that incorporates OCR output, applies domain-specific terminology where needed, and potentially integrates automated quality checks, all while maintaining document integrity at speed. Successfully integrating these various sophisticated components seamlessly into existing organizational workflows, while also ensuring data security and compliance across geographically distributed computing nodes, remains a significant technical undertaking despite the headline performance figures demonstrating raw speed potential.
How AI-Powered Document Translation Reduced Processing Time by 73% in 2025 A Technical Analysis - Standardized Templates Speed Up Legal Document Translation By 84%
Standardized formats, particularly via templates, are reportedly driving significant speed improvements in legal document translation, with reports pointing to increases reaching 84%. This acceleration stems from introducing consistent structure to documents, enabling translation tools to process text more predictably than varied content allows. This efficiency gain complements the broader impact of AI-powered methods on overall document handling, where observed reductions in processing time reach figures around 73%. Implementing these templates helps streamline multilingual legal workflows, becoming increasingly important for firms navigating a global landscape. Nevertheless, while the speed is notable, relying on templated structures and automated systems raises questions about how well they handle entirely novel legal concepts or highly unique cases, and whether focusing on rapid throughput might overlook subtle linguistic and legal nuances.
Focusing specifically on the influence of structural conventions, it appears that the implementation of standardized templates for legal documentation translation is significantly impacting workflow velocity. Observations suggest that by providing a predictable framework for the content, teams translating these documents spend less time wrestling with varied layouts, formatting inconsistencies, and structural nuances unique to each source file. This streamlining of the initial processing phase, allowing the focus to shift predominantly to linguistic transfer rather than structural recreation or adjustment, seems to be the primary driver behind reports indicating speed increases potentially reaching up to 84% for documents where such templates are applicable.
Furthermore, this emphasis on consistency inherent in standardized templates doesn't just accelerate the process; it inherently introduces a layer of uniformity into the translated output. From an engineering standpoint, presenting downstream systems, whether human or automated, with consistent structure should, in theory, reduce ambiguity and potential points of failure related to interpreting layout or content order. While directly quantifying the error reduction purely attributable to templates is complex, intuitively, fewer variables in the input structure should contribute to more predictable and potentially cleaner output, easing subsequent review stages compared to dealing with ad-hoc document structures. Integrating these template-aware workflows with existing AI pipelines, perhaps aiding the parsing and analysis stages, appears to be a logical next step in seeking further processing efficiencies. However, creating and maintaining these templates across different legal domains and jurisdictions, while ensuring they remain flexible enough for necessary variations, presents its own set of technical and operational considerations.
More Posts from aitranslations.io: