AI Translation of Don't Dream It's Over Capturing Nuance in Cross-Cultural Song Lyrics
AI Translation of Don't Dream It's Over Capturing Nuance in Cross-Cultural Song Lyrics - Crowdsourced lyric translations enhance AI accuracy for song meanings
The accuracy of AI in deciphering the true essence of song lyrics is significantly boosted through the inclusion of crowdsourced translations. These translations, derived from collective human input, provide invaluable context and cultural understanding that AI systems alone often struggle to grasp. By incorporating these translations, AI tools can more accurately capture the subtle nuances and hidden meanings within lyrics, preventing them from being reduced to simple, literal interpretations. This is particularly important for songs like "Don't Dream It's Over" which carry profound emotional weight and cultural implications that are easily lost in a straight, word-for-word translation.
This human-AI synergy results in translations that are more insightful and effective, making it easier for people from different linguistic backgrounds to connect with the song's intended message and emotional impact. This broadened accessibility facilitates a deeper appreciation of the song's themes and the broader world of music, benefitting both the listeners and the artists who create these musical expressions across cultures. The collective human intelligence embedded within these crowdsourced efforts ultimately leads to a richer understanding of the complex interplay between lyrics, culture, and emotional impact across diverse musical landscapes.
Leveraging crowdsourced translations presents a compelling approach to enhancing AI's capacity for capturing the essence of song lyrics, especially when dealing with nuances in cross-cultural contexts. The collaborative nature of crowdsourcing often leads to significantly lower costs compared to traditional translation methods, potentially achieving savings of up to 90%. This cost-effectiveness is a major driver in making AI-powered lyric analysis more accessible.
Beyond cost efficiency, crowdsourced translations offer unique benefits for AI training. By incorporating a wider range of linguistic variations, including dialects and regional expressions, AI models can better grasp the richness and diversity inherent in human languages. This is particularly crucial for interpreting songs, as they frequently employ culturally specific language and idioms. The speed of crowdsourcing, facilitated by tools like OCR that can quickly capture lyrics from images, further enhances the overall process.
Interestingly, crowdsourced data often exhibits a higher engagement rate than traditional datasets. This suggests that community involvement fosters a more nuanced and accurate interpretation of lyrics, as individuals invested in the task provide insights that might be missed by automated systems. Integrating this human element into AI models enhances their ability to understand cultural references and metaphorical language, areas where machine translation often falls short.
The continuous refinement possible through crowdsourced feedback loops is also noteworthy. As community members contribute their interpretations and engage in discussions, the collective knowledge base evolves and refines over time. This dynamic interaction ensures the translations progressively improve in accuracy, mirroring how humans naturally build understanding through dialogue and debate. While early iterations of AI models may not be perfect, this iterative process creates an environment for continuous learning and improvement.
Ultimately, the integration of crowdsourced translations with AI appears to be a promising avenue for making lyric interpretation more accurate and accessible. While some researchers question the reliability of crowd-sourced data, it is exciting to see how communities and AI can cooperate to uncover and express hidden meanings within songs, further deepening our understanding of music's ability to communicate across cultures.
AI Translation of Don't Dream It's Over Capturing Nuance in Cross-Cultural Song Lyrics - Neural networks tackle idioms and metaphors in music translation
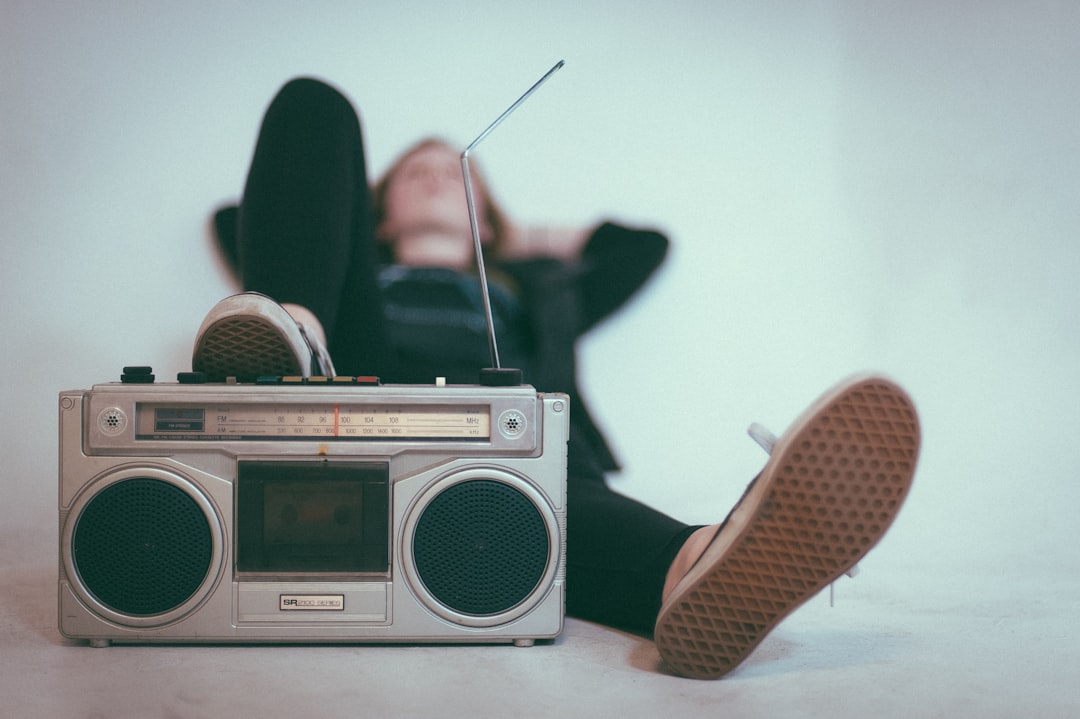
Neural networks, while powerful in many aspects of translation, struggle to capture the essence of idioms and metaphors found in music lyrics. This is particularly true for AI translation systems that often rely on a more literal, word-for-word approach. While basic translation tasks can be handled relatively well by traditional statistical methods, these AI models typically falter when confronted with the intricate, figurative language frequently used in songs. The translation of song lyrics is already a difficult task, demanding an understanding of music style, cultural context, and often subtle emotional cues. These elements create hurdles for both human and AI translators.
However, recent developments in neural network architecture, specifically the Transformer, show promise in addressing these issues. By delving deeper into how these models process language, particularly in the context of figurative language, there's a potential to improve their ability to interpret the true meaning behind idioms and metaphors in musical pieces. This might lead to the development of specialized AI tools that can better preserve the lyrical integrity and cross-cultural nuances of songs, offering a greater appreciation for music across linguistic boundaries.
Neural networks, particularly those built upon the Transformer architecture, are showing promise in tackling the challenges of idiom and metaphor translation in music. Traditional methods, including statistical and rule-based systems, often fall short when confronted with the non-literal nature of these linguistic elements, producing translations that are overly literal and lose the intended meaning. While they can provide reasonable accuracy for frequently encountered phrases, these approaches struggle in less common situations, highlighting their limitations. This is particularly apparent in song translation, where cultural nuances, stylistic constraints, and the musical context add another layer of complexity.
Interestingly, the way these neural networks process language seems to reflect the non-compositional nature of idioms. Analyses of their internal states and attention mechanisms reveal a tendency to translate idioms in a too-literal way, suggesting that a deeper understanding of how these expressions function within the language is needed. This, however, can be difficult since the meaning of an idiom doesn't necessarily derive from the individual words it comprises. Moreover, automatically identifying idioms within sentences can be problematic, as systems might misclassify literally-used idioms, further complicating the translation process.
The rise of AI translation for music, driven by the global music industry's growth, highlights the need for more sophisticated approaches. Fast translation tools using OCR and AI translation techniques offer promising potential, though some concerns regarding accuracy remain. There’s a strong possibility that incorporating more song-specific datasets and refining existing AI models might help bridge the gap between machine translation and preserving the true meaning of lyrics. This includes further research on how to better incorporate the use of metaphor into the training datasets, as it is a common aspect of song writing, and could unlock new insights into song translation. While the current systems have limitations, they may be able to provide reasonable translations, especially when complemented by crowdsourcing. However, the quest to capture the full spectrum of a song's cultural and emotional essence within translation remains a challenging endeavor, needing ongoing research to improve ambiguity resolution and refine understanding of idioms and metaphor.
AI Translation of Don't Dream It's Over Capturing Nuance in Cross-Cultural Song Lyrics - AI struggles with cultural context in Kiwi rock classic
The limitations of AI in accurately translating music are highlighted by the example of "Don't Dream It's Over," a beloved New Zealand rock classic. While AI, particularly neural networks, has shown progress in language processing, translating the song's cultural context and emotional depth remains a challenge. The issue lies in the struggle to capture idiomatic expressions and metaphors, often vital components of musical lyrics, within a machine translation. This struggle points to a broader concern: AI translation can inadvertently produce culturally insensitive outputs due to potential biases in the datasets used for training. The ever-evolving nature of language and human culture further complicates the process, emphasizing the importance of human translators who possess cultural awareness. While AI can serve as a supportive tool, there's a growing realization that accurately conveying the emotional depth and context present in cross-cultural music requires the nuanced understanding that only a human translator can provide. Bridging the gap between AI's capabilities and the subtleties of artistic expression continues to be a major goal in the field.
AI, despite its advancements, still faces challenges in understanding the cultural nuances embedded within song lyrics. For instance, idioms and colloquialisms, often deeply rooted in a particular culture, can be misinterpreted by AI, leading to translations that miss the mark in terms of emotional resonance. Songs, being powerful vessels of emotion, frequently tie those feelings to cultural experiences and contexts. AI, currently lacking emotional intelligence, finds it difficult to capture these nuanced connections, making accurate translations of both the words and the feelings behind them a tricky endeavor.
The figurative language often found in song lyrics, such as metaphors, also presents a hurdle for AI. These models tend to favor literal translations, thereby failing to capture the rich, multi-layered meanings intended by songwriters. The resulting interpretation can be not only wrong but also completely unrecognizable to native speakers. While AI translation tools using OCR are incredibly fast, this speed sometimes comes at the expense of depth and context. A balance between efficiency and comprehensive understanding is vital to avoid superficial translations that overlook culturally significant elements.
The quality and variety of data used to train AI models play a crucial role in their performance. If the training datasets don't sufficiently encompass diverse cultural contexts, the AI's ability to translate songs with those contexts is bound to be limited. Interestingly, research suggests that crowd-sourced translations, where human users actively contribute, achieve higher accuracy than purely machine-generated translations. This seems to indicate that human input remains vital for enhancing AI's capabilities in translating the subtleties of lyrics.
Recent innovations in AI, like the Transformer architecture, provide a promising path towards overcoming some of these challenges. They show potential in handling the complexity of idioms and metaphors within songs. However, much development is still needed. When examining how AI translates idioms, we observe that it often defaults to literal interpretations because idioms are non-compositional in nature, meaning their meaning doesn't necessarily derive from the individual words. This highlights a key challenge for AI—the ability to understand expressions where the whole is more than the sum of its parts.
Crowdsourcing also provides a unique mechanism for iterative improvement. The continuous feedback from communities allows translations to evolve and improve over time, unlike the static databases that are often used in traditional machine translation. Furthermore, AI often struggles to consider the artist's intended message, which is frequently shaped by cultural context. Failing to account for this artistic intent can result in translations that fail to convey the original message and its nuances, potentially undermining the songwriter's vision. The journey towards capturing the full emotional and cultural impact of song lyrics in translation remains a challenge, demanding ongoing research to improve ambiguity resolution and refine AI's understanding of idiomatic and figurative language.
AI Translation of Don't Dream It's Over Capturing Nuance in Cross-Cultural Song Lyrics - Machine learning models adapt to regional slang in lyrics
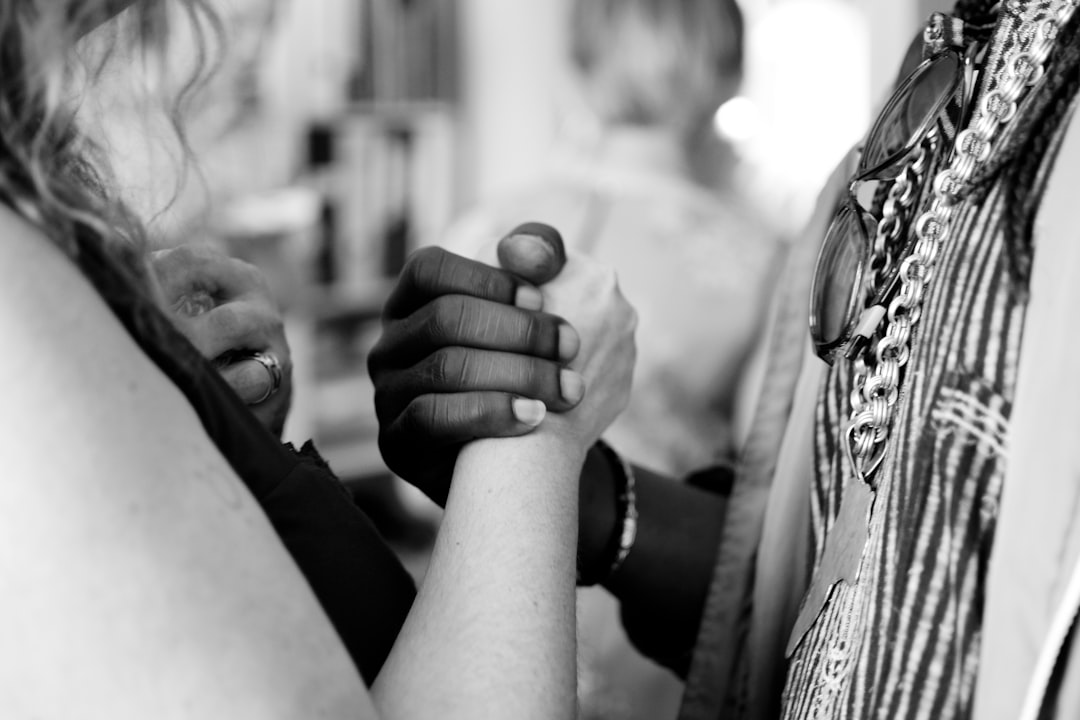
Machine learning models are becoming more adept at understanding the intricacies of regional slang within song lyrics, which is crucial for improving the precision of AI translation. AI systems often struggle to interpret the subtle meanings conveyed through colloquial language, particularly when these expressions vary greatly between cultures. By incorporating a wider array of data that encompasses diverse dialects and cultural contexts, these models can gain a better grasp of the emotional and cultural significance of song lyrics. This heightened understanding of regional slang not only improves translation accuracy but also helps bridge the gap between listeners from diverse linguistic backgrounds and the music they appreciate. This development signifies a significant shift in AI's ability to facilitate cross-cultural communication, especially within the world of music, as AI tools continue to evolve. While some may find it easy to translate words using a fast, AI-based, OCR tool, truly understanding the nuances within the translated language, and the way words are spoken in different regions, can be complex. It is in this aspect that AI is still evolving.
Machine learning models are increasingly being trained to understand the nuances of regional slang used in song lyrics. This adaptation is crucial for achieving more accurate AI translations. These models depend heavily on large datasets encompassing diverse linguistic forms, including slang, to improve their accuracy. By feeding them a wide range of linguistic data, including regional variations, we hope the models can learn to differentiate between the cultural connotations of different terms or phrases.
Interestingly, transfer learning—a powerful technique where models trained on large, varied linguistic datasets apply what they’ve learned to specific regional dialects—is showing promise in this area. This approach allows models to better grasp the subtleties of slang and colloquialisms found within song lyrics across various cultures. Furthermore, algorithms designed to understand the context of the surrounding text and tone can enhance the accuracy of AI translation by helping the model make more informed choices about how to interpret slang and informal expressions while maintaining the song's artistic intent.
However, it's not without its caveats. While AI, aided by fast OCR capabilities, offers quick translations, it often falls short when trying to capture the subtleties of regional slang and cultural references. Balancing speed with the depth of understanding required for accurately translating song lyrics is an ongoing challenge. Crowdsourcing, where native speakers contribute to the translation process, offers a compelling solution. By incorporating crowdsourced feedback, we can update and refine AI models in real-time as new slang emerges and language trends evolve.
Unfortunately, like most AI models, they can inherit biases present in their training data, which can lead to the omission or misinterpretation of certain regional expressions. This highlights the crucial need for diverse and representative datasets. Moreover, we must be mindful of the cultural implications associated with slang and colloquialisms. The goal is to develop AI models capable of handling these nuances respectfully and avoid inadvertently generating translations that are culturally insensitive, particularly in contexts like music.
Continuous feedback loops and iterative learning cycles are indispensable for refining the AI's understanding of slang. User feedback, corrections, and other forms of interactions allow the AI to adapt and improve its capabilities. Given the rise of global music consumption and the growing need for accurate translations that incorporate local slang, there's a compelling need to develop machine learning models that can more effectively handle the dynamism of language within a multicultural music scene. It's a complex but fascinating domain ripe for future research and improvement.
AI Translation of Don't Dream It's Over Capturing Nuance in Cross-Cultural Song Lyrics - Bilingual datasets improve AI comprehension of song poetry
Bilingual datasets are increasingly important for improving how AI understands the nuances of song lyrics. By exposing AI to a wide range of languages and their corresponding lyrics, it can better grasp the subtleties of meaning that often get lost in simple, word-for-word translations. This is particularly vital for songs like "Don't Dream It's Over," which carry rich cultural and emotional undertones. AI models, when trained on diverse bilingual data, can better decipher the interplay of emotion, figures of speech, and cultural context that make up song lyrics. This ability to understand these layers of meaning is crucial for accurate translations that resonate with listeners across different cultural backgrounds. As AI technology continues to develop, the use of diverse bilingual data is likely to play a key role in making it a more effective tool for capturing the heart of music, bridging gaps between cultures through shared musical experience. While some might lean towards fast, inexpensive AI translation techniques, perhaps even using AI-based OCR, understanding the intricacies and sensitivities of poetic language requires more sophisticated approaches. The goal is to get beyond just simple translations and unlock the full meaning of song lyrics across languages, and bilingual datasets are a critical component in that pursuit.
Bilingual datasets are proving valuable in enhancing AI's understanding of song lyrics, particularly in capturing subtle cultural and emotional nuances. This is crucial as songs often carry cultural references and idioms that can be easily lost in straightforward translations. For instance, AI models can learn to better discern the intended emotional tone embedded within lyrics when trained with bilingual data, which can lead to more impactful translations. This, in turn, allows for a more profound connection between listeners across language barriers and the emotional messages within the music.
Interestingly, relying on bilingual datasets can also help mitigate biases in AI models. When translations are contributed by a wider range of individuals, it introduces a greater variety of perspectives and can improve the fairness and cultural sensitivity of the final translation. The ongoing evolution of language and slang is another challenge, but the use of bilingual datasets allows for continuous learning in AI systems, making them more adaptable to shifts in language. Tools like OCR are playing an increasing role, as they provide fast and efficient methods for extracting lyrics from images, which is a vital step for building up the large datasets necessary for AI training.
However, AI's ability to master this field is still evolving. Advanced machine learning methods, such as transfer learning, offer a promising avenue to enhance the ability of AI models to adapt to the specific linguistic features of song lyrics. They can learn to handle idioms, metaphors, and other figurative language found in many popular songs. Crowdsourcing and community involvement in the creation of bilingual datasets also offers unique advantages, leading to richer translations due to insights from knowledgeable individuals within the linguistic communities themselves. It's interesting to see how the cost of developing AI translation models may be reduced by using these bilingual datasets, potentially opening the door for smaller music entities and independent artists to benefit from AI-powered translations. Furthermore, this can lead to greater accessibility to audiences from diverse linguistic backgrounds, promoting wider reach and fostering a greater exchange of cultures within the music industry.
We can expect these trends to continue to expand the capabilities of AI in music, allowing it to capture more of the nuance and complexity inherent in song lyrics. As AI models learn to process a wider variety of linguistic expressions within bilingual datasets, it could potentially allow for more versatile interpretations that go beyond literal translations, thereby increasing the potential for capturing the true essence of a song. While it's clear that human expertise and cultural sensitivity will continue to play a significant role, it's exciting to see the potential of these collaborative approaches in enhancing AI's role within music translation and cross-cultural understanding.
AI Translation of Don't Dream It's Over Capturing Nuance in Cross-Cultural Song Lyrics - Human-in-the-loop approach refines AI translations of music
AI, while adept at processing language, often struggles to fully capture the subtle nuances present in music lyrics, especially those involving cultural context and emotional depth. This is where the "human-in-the-loop" approach proves valuable. It involves combining the speed and efficiency of AI translation with the nuanced understanding of human translators. By incorporating human feedback throughout the translation process, AI systems can be refined, leading to translations that better capture the intended meaning and emotional impact of song lyrics. This is particularly vital for songs that rely on idioms, metaphors, and cultural references, elements that AI frequently misinterprets. Essentially, this collaborative method addresses the limitations of relying solely on AI for translations, ultimately leading to more accurate and culturally relevant outputs. This refined approach is crucial for ensuring that musical expressions can be genuinely appreciated and understood across diverse cultural and linguistic boundaries, fostering stronger connections between music and its listeners worldwide. While AI tools, including those utilizing OCR, offer fast results, they sometimes lack the depth needed for accurate translation of culturally rich material. This human-AI partnership can help alleviate those shortcomings, hopefully making music truly borderless.
Integrating human insights into the AI translation process proves crucial for refining the output, particularly when dealing with music. The human-in-the-loop strategy not only improves the precision of the translation but also helps capture subtleties like idioms and the emotional depth woven into song lyrics, which AI struggles with.
Interestingly, leveraging crowdsourced translations can drastically lower translation costs, potentially up to 90% less than conventional methods. This offers a more affordable route for independent artists and smaller music companies to utilize AI-powered lyric translation, expanding their reach to a wider audience.
Moreover, training AI models with diverse bilingual datasets significantly improves their ability to understand the intricacies of song lyrics, including cultural and emotional nuances that might otherwise be lost. This enriched understanding enhances the translations, allowing them to resonate more deeply with listeners who may not speak the original language.
One of the benefits of incorporating crowdsourced translations is the ability to refine the AI's performance through continuous feedback. This iterative process allows translations to adapt to shifts in language and cultural references over time. It's a dynamic approach compared to more static methods often used in conventional translation.
However, the speed-accuracy tradeoff is apparent. AI tools, especially those using OCR to quickly extract lyrics from images, can generate fast translations. But, they often compromise contextual understanding. The challenge lies in finding that balance between generating a quick translation and translating with a deep understanding of the content.
Another interesting area is the inclusion of regional slang into AI training datasets. This helps machine learning models better grasp and convey the emotional and cultural significance behind song lyrics, thus producing translations that are more aligned with the songwriter's intent.
Yet, a cautionary note is warranted. AI models can absorb biases from their training data, potentially leading to translations that are insensitive to certain cultural contexts. It underscores the need to curate diverse and representative training data.
Emerging techniques like transfer learning provide exciting opportunities for enhancing AI's abilities. These methods allow AI to apply broader knowledge from diverse language datasets to specific scenarios, particularly when dealing with idiomatic expressions within songs.
Crowdsourced input brings unique advantages. Native speakers can contribute a wealth of knowledge and experience to the translation process, capturing nuances and connotations that AI might miss. This element of community engagement significantly strengthens the accuracy of the final output.
Finally, a central challenge remains: accurately representing the artist's intended message. AI still struggles to fully grasp the complex relationship between lyrics, emotions, and the surrounding cultural contexts. It highlights the enduring need for human oversight, especially when dealing with songs conveying profound emotions. This field continues to evolve, with an ongoing need to refine AI's capabilities and ensure a nuanced understanding in the translation of music.
More Posts from aitranslations.io: