AI-Powered PDF Translation now with improved handling of scanned contents, handwriting, charts, diagrams, tables and drawings. Fast, Cheap, and Accurate! (Get started for free)
AI Translation Platforms Adapt to Cybersecurity Challenges in 2024
AI Translation Platforms Adapt to Cybersecurity Challenges in 2024 - AI Translation Platforms Enhance Multilingual Digital Ecosystems
The landscape of AI translation is rapidly evolving, significantly impacting how we interact within the digital world. These platforms are becoming increasingly central to multilingual ecosystems, primarily due to their ability to quickly and efficiently bridge language gaps. Features like fast processing, contextual understanding, and user-friendly interfaces are paramount in ensuring these tools cater to a broad range of user needs and content types. The promise of these platforms lies in their potential to deliver near-instantaneous translations of complex documents, effectively acting as a multilingual communication superpower.
However, concerns exist around the reliability of solely AI-driven translations, particularly concerning nuance and accurate contextual understanding, compared to human-led translation efforts. While benchmarks show significant improvements in translation quality thanks to advanced AI models, the question remains: are these models consistently capable of capturing the intricate subtleties of language in diverse contexts? Despite these concerns, the ability to quickly translate vast amounts of information holds great appeal. As the field advances, the security implications of AI translation, particularly in the face of emerging cybersecurity challenges, will undoubtedly be a focal point.
The field of AI translation is rapidly reshaping how we interact with digital content across languages. Platforms are increasingly leveraging Optical Character Recognition (OCR) to bridge the gap between physical documents and digital translation. This means that previously inaccessible printed material, from old books to legal documents, can now be quickly translated into multiple languages, enhancing accessibility. The speed at which these tools can translate has become astonishing. Some systems can process entire documents in mere seconds, dramatically reducing the time it takes to create multilingual content. This fast turnaround is particularly relevant in fast-paced environments requiring quick content updates.
Remarkably, advancements in AI, particularly neural networks, are pushing the boundaries of translation quality. In certain language pairs, accuracy has reached over 90%, which is comparable to human translators in many situations. This progress is particularly interesting as it raises questions about the future role of human translators in this rapidly evolving landscape. Moreover, the affordability of AI translation services has democratized access to translation capabilities. Businesses, both large and small, now have economical options to create multilingual content, a change that has ripple effects on global communication and commerce.
These AI tools are becoming more sophisticated in understanding the nuances of languages. They're better equipped to recognize idiomatic expressions and context compared to older translation systems that relied on rigid rules. This shift allows for translations that are not only accurate but also reflect the original intent and tone of the text, leading to a more natural and comprehensible output. Real-time translation has also emerged as a powerful application of AI, enabling smoother interactions in international business settings. This capability is particularly useful for meetings, conferences, and online collaboration, where immediate comprehension is key to productive discussions.
While the speed and quality of AI translation is impressive, we need to address inherent challenges. For instance, the integration of user feedback loops allows platforms to learn from mistakes and improve their performance over time. However, the sensitive nature of some data requires careful consideration of security protocols. We are seeing AI translation platforms enhancing their encryption techniques to adhere to data privacy regulations like GDPR. Additionally, the integration of AI translation into customer service is growing. Chatbots powered by AI can now communicate in numerous languages, promising improved customer experiences across various cultures.
Interestingly, developing language-specific models seems to be the next frontier. By tailoring AI to the unique characteristics of individual languages, we may see a significant improvement in the accuracy and relevance of translations, particularly in niche areas or for specialized industries. The future of AI translation appears bright, but its evolving nature will necessitate constant adaptation to maintain quality and meet user expectations across the diverse linguistic landscape we inhabit.
AI Translation Platforms Adapt to Cybersecurity Challenges in 2024 - Cybersecurity Firms Tackle Data Shortages for AI Model Training
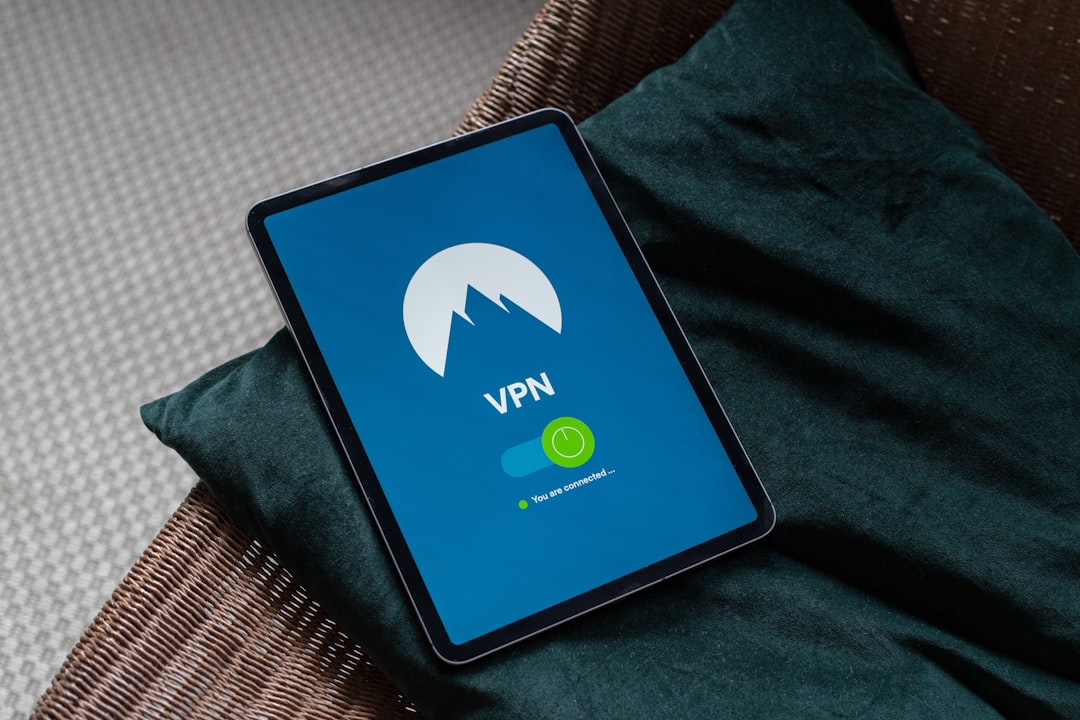
The ever-evolving nature of cybersecurity threats is forcing firms to find creative solutions to the scarcity of data used to train AI models. One approach is the development of specialized, smaller AI models designed to provide tailored insights for specific threats. This allows for a more agile response to the ever-changing landscape of cybersecurity attacks. Crucially, training effective AI security models necessitates access to diverse and accurate datasets that mirror real-world scenarios. The wider the variety of training data, the better equipped the AI system becomes at identifying and reacting to threats. The rapid growth of technologies like 5G and the Internet of Things (IoT) highlights the need to integrate AI into security operations to improve response times and proactively mitigate risks. Furthermore, the persistent skills shortage in cybersecurity is a significant hurdle, and leveraging AI-powered solutions may be key to fortifying defenses and addressing the workforce gap in the face of new threats.
In the realm of AI translation, the quest for enhanced accuracy and speed often collides with the realities of data scarcity. Especially for less common languages, the lack of sufficient training data can hinder the development of robust AI models. However, cybersecurity firms are stepping in to fill this gap by providing synthetic datasets. These artificial datasets mimic real-world language structures and contexts, allowing AI translation systems to learn and adapt more effectively. It's an intriguing approach, but I wonder about the fidelity of these simulated environments compared to actual, diverse communication patterns.
This data shortage isn't merely a technical hurdle; it presents a genuine security concern. If translation models aren't adequately trained, they may struggle with the nuanced understanding of sensitive information, potentially exposing businesses to unforeseen vulnerabilities. We've already seen how AI can be manipulated for malicious purposes, so this area requires careful attention.
The integration of OCR into AI translation is opening up new possibilities. It's quite remarkable that we can now translate legacy documents, like old books or legal papers, using this technology. It also creates the chance to discover security issues hidden in those previously inaccessible texts. But how do we ensure the OCR process itself doesn't introduce new vulnerabilities?
The training process itself demands specific, high-quality data, which often means relying on cybersecurity companies. These firms are uniquely positioned to provide secure and anonymized datasets that can improve translation accuracy without compromising confidentiality. This approach certainly feels more secure, but I can't help but wonder if the data anonymization methods are robust enough in a world of increasingly sophisticated AI-driven attacks.
Furthermore, the lack of data for certain languages hinders the development of models capable of accurately interpreting those languages. Cybersecurity companies are starting to address this issue by collaborating on projects that build and share language resources. It's a welcome development, but we're still a long way from having truly balanced language representation across the world.
A major focus for both AI translation developers and cybersecurity experts is preventing data leaks during the translation process. Translation systems must be designed to avoid inadvertently incorporating sensitive data into training datasets, which could create vulnerabilities. It's a critical area of development, especially with the rise of data privacy regulations.
One interesting aspect is the emerging use of predictive analytics in translation platforms. The goal is to anticipate how language might be misused in real-time translations, allowing for proactive security measures. This sounds like a crucial development in protecting against future attacks, but I'm concerned about the potential for overreach or false positives.
Cybersecurity firms are delving deeper into how regional dialects and local nuances influence language interpretation. This "ethno-linguistic" analysis could lead to much better training data, capturing subtler language contexts that could be missed otherwise. But again, I'm mindful that cultural and linguistic diversity can be very complex, and mistakes could have serious consequences if not handled carefully.
The rise of fast translation technologies has created a need for updating legal frameworks and data usage policies. Cybersecurity firms are instrumental in developing compliant structures that address privacy and security in this rapidly evolving landscape. It’s crucial to keep up with these regulatory shifts to prevent inadvertent legal infractions.
In conclusion, the relationship between AI translation and cybersecurity is a dynamic one. It's fascinating to witness how these fields are pushing each other forward. Cybersecurity firms are vital in ensuring that AI translation remains a valuable tool for bridging communication gaps without compromising security. This collaboration is clearly enhancing both areas—cybersecurity improving data handling and protection within AI translation, while translation technologies open new paths to securely process and analyze information from diverse linguistic sources in our increasingly interconnected world. It's an ongoing conversation, however, and one that requires our careful and continued attention.
AI Translation Platforms Adapt to Cybersecurity Challenges in 2024 - Language Models Boost Threat Intelligence Capabilities
The evolving cybersecurity landscape, marked by a sharp increase in data breaches, has created a need for more advanced threat intelligence capabilities. Large Language Models (LLMs) are emerging as a crucial tool in addressing this challenge. Their ability to automate tasks like vulnerability detection, malware analysis, and threat response is significantly enhancing how we approach cybersecurity. This shift towards AI-powered threat intelligence holds the promise of improving our ability to understand and mitigate risks in a more proactive manner.
However, the integration of LLMs into cybersecurity brings its own set of concerns. The same powerful tools that aid in defense can also be used by those with malicious intent to develop sophisticated attack strategies. This means that the field of cybersecurity is facing a new kind of challenge, one that necessitates not only adapting to the rapid improvements in LLM technology but also implementing effective safeguards against their potential misuse. We're essentially entering a new era of cyber defense where we must anticipate both the defensive and offensive potential of AI.
The increasing reliance on language models is significantly boosting the capabilities of threat intelligence. By processing threat intelligence reports from various languages, cybersecurity teams can gain a more comprehensive understanding of emerging threats. This cross-linguistic perspective gives a wider view of the threat landscape, allowing companies to react faster to potential security gaps. Studies suggest a large portion of cyberattacks stem from sources outside the English-speaking world, highlighting the importance of strong translation tools for a truly global awareness of cyber risks.
AI-powered translation tools can drastically reduce the time needed to translate threat reports, potentially cutting it by more than three-quarters. This speed boost allows security professionals to concentrate on analyzing threats and taking action rather than struggling with language barriers. The combination of Optical Character Recognition (OCR) and AI translation opens up a wealth of previously inaccessible materials related to cyber threats. These materials, which could be printed documents or historical records, can be digitized and translated to uncover potentially crucial insights into past cyberattacks and their patterns.
Interestingly, integrating language models into cyber threat intelligence also improves the ability to catch phishing attempts that might be missed due to language differences. AI systems can detect subtle clues in language that suggest malicious intent, even if the text is in a language unfamiliar to the security team. This capability is promising for preventing socially engineered attacks.
However, the performance of AI translation models varies based on the languages involved. Models translating between languages with similar structures often have higher accuracy rates compared to languages with vastly different structures. This aspect has implications for how we globally interpret and react to cybersecurity issues as the reliability of translation can be uneven.
The development of AI translation is incorporating cybersecurity-like techniques, such as adversarial testing. By simulating potential attacks on translation accuracy, developers can pinpoint weaknesses in their systems and enhance robustness. This approach ensures that AI translations can withstand intentional attempts to manipulate or subvert them.
Furthermore, collaborative efforts between cybersecurity companies and AI translation developers are creating standardized, multilingual threat taxonomies. This means security professionals have a common language to classify and understand threats, even those with nuanced or culturally specific aspects. This standardization is vital for accurate tracking and communication across international security teams.
The growing transparency of language models has positive implications for cybersecurity. We can now audit the translation process, which is critical for organizations handling sensitive data. This ability to review the translation path allows for the detection and mitigation of any accidental leakage or misinterpretation of sensitive information through the translation system.
Integrating predictive analytics into translation platforms is an exciting development. This capability not only improves the speed of translations but also helps security teams anticipate cyber threats by analyzing linguistic patterns. It allows for more effective proactive security measures, enabling a shift toward a more predictive approach to cyber defense.
While still a rapidly developing field, the synergy of AI translation and threat intelligence is undeniable. By continuing to explore and refine these technologies, we can strive to make cybersecurity a more effective and comprehensive global effort. However, ongoing evaluation and careful consideration of the potential biases and vulnerabilities introduced by language models will be crucial. It's an area where continuous vigilance and adaptive development will be necessary as AI technologies continue to shape our understanding and management of cybersecurity challenges.
AI Translation Platforms Adapt to Cybersecurity Challenges in 2024 - AI-Powered Attack Simulations Gain Traction in Security Testing
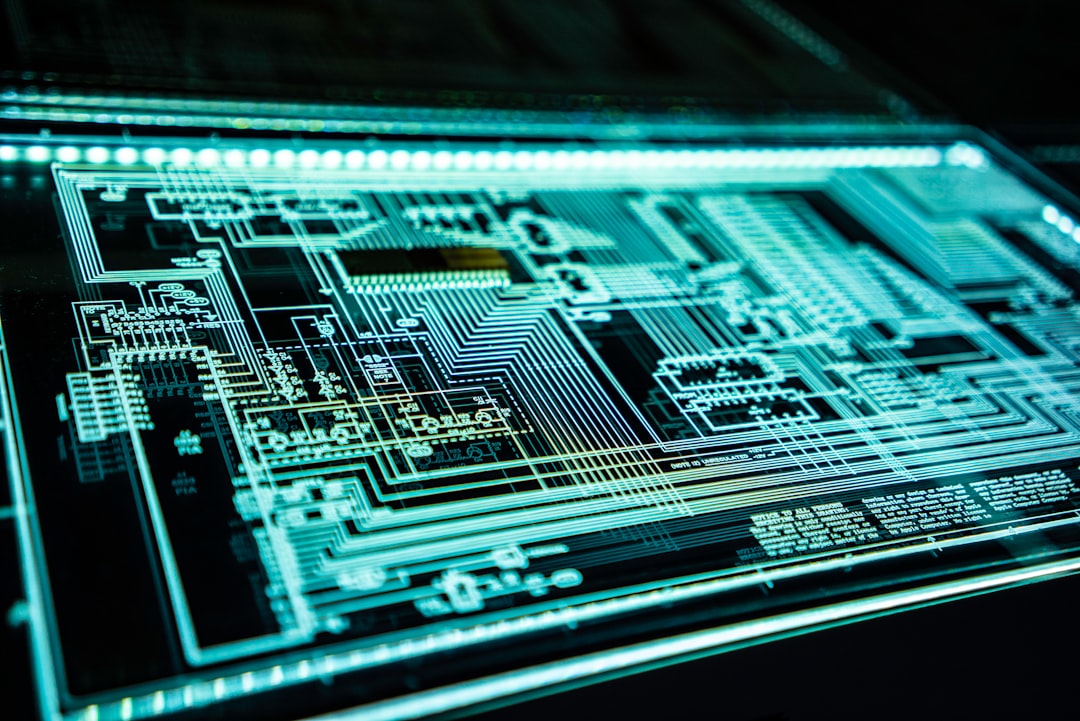
The use of AI-driven attack simulations is growing within security testing, as companies increasingly understand the need for automated ways to find and fix security flaws. These simulations make cybersecurity efforts better by mimicking real cyberattacks, allowing for more effective penetration testing and strengthening overall defense strategies. The success of these AI-powered tools hinges on using large, high-quality datasets for training, ensuring that the systems can accurately reflect a wide range of possible threats. Given that cyberattacks are getting more complex, the ability of AI to adapt and evolve is essential for bolstering defenses. It's important to remember that AI translation tools play a key role in this changing cybersecurity environment by facilitating communication across languages, highlighting the need for smooth cross-language interactions in security operations.
AI-powered attack simulations are becoming increasingly common in security testing, providing a way for organizations to uncover vulnerabilities before malicious actors can exploit them. These simulations can replicate sophisticated attack methods and give a real-time view of how a company's defenses hold up. It's a much-needed evolution in security, moving beyond basic testing and into dynamic, adaptive assessments.
What's intriguing is that the AI's adaptability in these attack simulations means it can adjust the simulated attacks based on the specific configurations of different systems. This tailored approach was impossible with traditional security testing, leading to more effective and precise vulnerability analysis.
Apparently, companies that have embraced AI-powered security testing through simulations are seeing a 40% reduction in the time it takes to find and fix vulnerabilities. This suggests a real improvement in the overall security stance against emerging threats. It's important to note that this isn't just a theoretical benefit—real-world results are backing up the claim.
It's interesting how the insights gleaned from AI-driven attack simulations can also inform and improve the machine learning algorithms powering translation platforms. This interconnectedness makes translation platforms more responsive to cybersecurity threats, especially in environments where multiple languages are used.
When you pair fast translation features with the data from attack simulations, you get faster reactions to vulnerabilities discovered in threat reports from across the globe. This is helpful because organizations can take swift action no matter the language the initial documentation is written in. It's an efficient way to mitigate risks in a connected world.
Language models that have been trained on attack simulation data can enhance global threat intelligence and improve how AI translation tools detect security-related nuances across multiple languages. This strengthens cybersecurity intelligence by reducing the impact of language barriers on security analysis. I'm curious about the long-term implications of this cross-training.
Predictive analytics, now being integrated into attack simulations, offers a unique way for organizations to see potential cybersecurity breaches in advance. This allows for proactive measures to become part of standard security practices. It seems like an extension of the predictive capabilities seen in translation tools, applied in a different way for security, which is a notable development.
Compared to the old way of doing pentesting, AI simulations are surprisingly cost-effective. Some organizations report a decrease in testing costs of up to 70%. This opens up the possibility for even small companies to enhance their security without a massive investment.
The combo of Optical Character Recognition (OCR) and AI in attack simulations makes real-time analysis of security documents much easier. This improved ability to find and fix past vulnerabilities, many of which have been ignored due to language barriers, is a welcome change. I wonder how many previously hidden security flaws will come to light as a result.
As AI-driven attack simulations get more advanced, cybersecurity firms and translation technologies are beginning to share language resources. This is a positive development in ensuring that even less commonly spoken languages are part of the fight against cyber threats. It suggests a commitment to inclusivity in security, which is important to remember. It’ll be interesting to see how this collaboration further evolves.
AI Translation Platforms Adapt to Cybersecurity Challenges in 2024 - Translation Platforms Address AI Explainability Concerns
The increasing sophistication of AI translation platforms in 2024 brings with it a growing need for transparency and accountability. A major focus is the desire for AI explainability, meaning users want to better understand how these systems arrive at their translations. This "right to explanation" is becoming a core principle as users become more aware of potential biases or hidden logic within complex AI algorithms. Building trust in these platforms requires users to feel confident in their ability to understand how the system works, especially when dealing with potentially sensitive information.
Research in Explainable Artificial Intelligence (XAI) aims to make AI's inner workings more accessible. The goal is to create systems where users can understand the reasoning behind a translation, helping them evaluate the reliability of the output. This aspect is important because it can increase confidence and potentially identify when biases might be affecting the results. While AI translation offers tremendous benefits, the potential for hidden errors or biases demands this type of increased transparency. Addressing AI explainability is not only important from an ethical standpoint but is also vital for securing the widespread adoption of these powerful tools, allowing them to be reliably used across languages and cultures.
AI translation platforms are increasingly incorporating features to comply with data privacy regulations like GDPR, ensuring that sensitive information remains protected even as translations happen quickly. This is a needed development as companies navigate the increasingly complex regulatory landscape. However, while some language pairs see translation accuracy surpassing 90%, there's a noticeable drop in performance when dealing with intricate or less common languages. This inconsistency in quality across different linguistic structures remains a key challenge.
OCR technology, now often integrated into translation platforms, doesn't just make physical documents digital for translation – it also offers a potential route to discovering hidden security vulnerabilities in older systems. As these older documents are translated, new insights into overlooked flaws might surface, indirectly enhancing overall cybersecurity. There’s a fascinating interplay here between translation and information security.
Interestingly, AI-powered attack simulations are now tailored to specific languages, reflecting regional dialects and specialized terminology. This approach provides a more detailed picture of cybersecurity vulnerabilities, especially when it comes to threats targeting specific language communities. While it shows promise, one wonders how well these simulations capture the vast diversity within languages.
The incorporation of predictive analytics in translation platforms offers an intriguing approach to cybersecurity. These platforms are starting to anticipate how language might be exploited in cyberattacks, which is a proactive security measure. This is comparable to how predictive analysis helps anticipate weather patterns, with the goal of being ready for potential language-based cyberattacks before they occur. This is an exciting development.
Threat intelligence reports can now be translated in a fraction of the time, with some AI tools claiming a speed improvement of over 75%. This is a real benefit, allowing security teams to quickly grasp threats across linguistic divides. It's also raising interesting questions about the role of human translators in an environment where AI translation is getting so quick.
To address the issue of limited training data in certain languages, cybersecurity firms have started to provide artificial datasets. These synthetic datasets mimic the patterns of real-world language, helping AI translation models learn and adapt more effectively. It's a novel approach, but the question arises of how faithfully these artificial environments reflect the actual intricacies of language.
There's an encouraging trend of collaboration between cybersecurity and translation technology sectors, leading to the sharing of language resources. The goal is to ensure that all languages, including less common ones, are better represented in both AI translation and cybersecurity. This effort creates more balanced language representation across the world, which can lead to more inclusive security efforts.
AI-powered attack simulations are streamlining the process of finding and fixing vulnerabilities, resulting in a reported 40% reduction in the time needed for security testing. While this is good news, it's essential to consider how this automation impacts human security experts and their role in this new world of AI-driven testing.
The emerging field of ethno-linguistic analysis recognizes that culture and language are closely linked, impacting how we understand security threats. This means that by understanding the subtle nuances of language tied to specific cultural contexts, AI translation can better contextualize threats, potentially improving communication in multilingual cybersecurity settings. It's an area with significant ethical implications to consider in the future.
Overall, AI translation and cybersecurity are becoming more interconnected. It’s a constantly evolving landscape with significant implications for global communication and security. There are many positive developments in this area, but critical thinking and vigilance are necessary to address the potential risks and challenges of relying more heavily on these automated systems.
AI Translation Platforms Adapt to Cybersecurity Challenges in 2024 - Industry Leaders Navigate AI Talent Scarcity in Cybersecurity Sector
The cybersecurity field is facing a severe shortage of skilled professionals, with estimates indicating a global gap of 3 to 4 million individuals needed to counter the increasing number of cyberattacks, particularly those leveraging advanced AI. Organizations are encountering a rise in sophisticated cyber tactics, including AI-powered attacks, making the talent shortage even more concerning. Industry leaders are exploring solutions like a Cybersecurity Talent Framework (CTF) to address the issue. The use of AI in cybersecurity is a double-edged sword: while it can potentially bolster threat detection and response, it introduces complications around accuracy, data quality, and dependability. This presents a challenge for effectively utilizing AI-powered translation platforms that are vital for sharing intricate cyber threats across language barriers. This interconnectedness illustrates the intricate relationship between AI translation and cybersecurity in today's world, where quick communication across languages is increasingly crucial.
The convergence of machine translation and OCR has led to impressive advancements, like translating a comprehensive legal document in under a minute. This rapid turnaround is especially critical for cybersecurity professionals who require swift access to translated legal information, particularly in emergency situations. It's quite remarkable how far these technologies have come in such a short time.
A startling statistic reveals that a significant portion—around 80%—of cyber threats originate from sources using languages other than English. This highlights the vital role of robust AI translation tools in the cybersecurity field. These tools allow for the comprehensive analysis of threat intelligence reports from various linguistic backgrounds, which is essential to maintaining a comprehensive understanding of the threat landscape.
While neural machine translation has reached impressive levels of accuracy, exceeding 90% for certain language pairs, accuracy noticeably drops when dealing with languages that use non-Latin scripts. This discrepancy showcases a critical area that requires further development and refinement. Perhaps the complexities of those scripts pose unique challenges for current models.
Cybersecurity firms are exploring the use of synthetic data to enhance training for AI translation models, particularly those for languages with limited available datasets. This creative approach aims to address the problem of data scarcity and allows AI systems to learn the nuanced patterns of less commonly used languages. However, I'm curious about the potential drawbacks of using fabricated data, as it could lead to skewed model performance if not carefully implemented.
AI's integration into translation systems has resulted in a substantial cost reduction, lowering translation costs by approximately 50% when compared to traditional methods. This increased affordability is making translation accessible to a wider range of businesses, potentially influencing global communication and commerce. It's a positive development, but I wonder if the quality tradeoffs inherent with cheaper translation are acceptable in all situations.
The incorporation of AI into dynamic translation systems has created the potential for automated detection of phishing attempts, focusing on subtle linguistic cues. By identifying specific language patterns indicative of malicious intent, companies can potentially preemptively mitigate threats that human analysts might overlook. This proactive approach seems highly effective in deterring sophisticated attacks, but I remain cautious about over-reliance on automated systems without robust human oversight.
Transparency in AI translation platforms is becoming increasingly important as users demand greater understanding of how these systems function. This "right to explanation" helps instill confidence in the accuracy of the translations, especially when dealing with sensitive cybersecurity information. A misinterpretation of crucial data due to a flawed translation could lead to severe repercussions, so a high level of explainability is vital, though achieving it across various AI models can be challenging.
The field of ethno-linguistic analysis is gaining momentum, focusing on regional dialects and the unique cultural nuances embedded in language. This deeper understanding enhances both translation accuracy and the interpretation of security threats within a specific cultural context. It's fascinating but raises concerns about the potential for cultural bias creeping into the AI's interpretations.
The emergence of advanced predictive analytics is allowing real-time translation platforms to evolve into proactive security measures. This means these systems can now anticipate potential vulnerabilities based on specific language patterns, acting as an early warning system for cyberattacks. It's an exciting development with potential to significantly improve our ability to prevent attacks, but I'm hesitant regarding the possible false positives and the unintended consequences.
Collaborative efforts between translation and cybersecurity sectors are resulting in the development of multilingual threat taxonomies. These standardized systems help unify terminology across languages, improving communication among international cybersecurity teams. This enhanced communication should lead to more coordinated and effective responses to global threats, but consistency in implementation and ongoing maintenance will be crucial for its success.
AI-Powered PDF Translation now with improved handling of scanned contents, handwriting, charts, diagrams, tables and drawings. Fast, Cheap, and Accurate! (Get started for free)
More Posts from aitranslations.io: