AI-Powered PDF Translation now with improved handling of scanned contents, handwriting, charts, diagrams, tables and drawings. Fast, Cheap, and Accurate! (Get started for free)
How AI-Driven LLM Training Could Transform Machine Translation Insights from Eureka Labs' New Educational Platform
How AI-Driven LLM Training Could Transform Machine Translation Insights from Eureka Labs' New Educational Platform - OCR Integration Enables Real Time Translation of Physical Documents Through Eureka Labs Platform
Eureka Labs' new platform incorporates OCR, allowing for a faster transition from physical documents to translated text. The ability to turn scanned documents, PDFs, and images into usable data streamlines the whole translation process. This is particularly valuable with the rise of mobile apps that can now directly translate scanned text on the fly. This integration potentially makes real-time cross-language communication faster and more efficient. While promising, the integration of AI, especially the use of large language models to refine the translated text, brings into focus a crucial tension: the need for balance between innovation and ensuring the reliability and security of the processed information. Even with AI assistance, the quality of OCR output and potential risks associated with the data remain key concerns as this technology expands.
Eureka Labs' platform leverages OCR integration to enable real-time translation of physical documents. While OCR itself has been around for a while, its accuracy and capabilities have improved dramatically due to recent advancements in AI. We see how combining multiple OCR engines can help correct and refine the output, leading to more accurate text extraction, which is essential for the following translation steps. Interestingly, large language models (LLMs) are now part of the workflow, enhancing the quality of OCR output by structuring and formatting the raw extracted text into something usable for translation.
The field of OCR is dynamic and we are seeing new developments all the time. These include multilingual capabilities and even augmented reality integration in some cases. One challenge with the wider adoption of OCR for real-time translation is the ongoing concern around the accuracy of the system and the need to ensure data security, especially when dealing with sensitive information. However, OCR is finding a place in a wide range of applications including mobile phone-based apps. This creates new opportunities for users who can now quickly scan and translate documents without the need for a computer.
It's exciting to see how the growing desire for document management solutions intersects with AI-enhanced OCR capabilities. And this whole field is boosted by advancements in machine translation itself, with initiatives like the OPUS ecosystem supporting the creation of open-source machine translation models. This kind of collaborative, open approach can potentially speed up development and adoption of better real-time translation tools.
How AI-Driven LLM Training Could Transform Machine Translation Insights from Eureka Labs' New Educational Platform - Learning From Translation Errors Machine Translation Models Now Track Common Mistakes
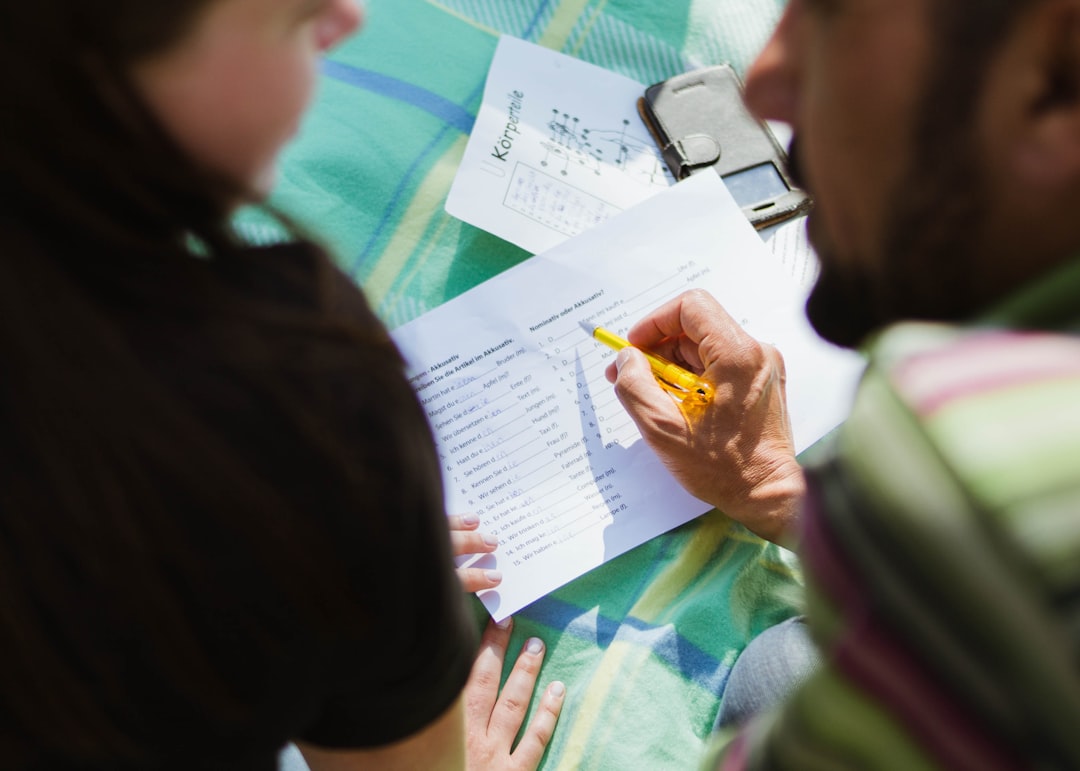
Machine translation systems are evolving, moving beyond simply producing output to actively learning from their own mistakes. This shift is leading to noticeable improvements in translation accuracy and quality. Researchers are employing methods like fine-grained error analysis, using frameworks such as Multidimensional Quality Metrics (MQM), to pinpoint common errors. These insights are then used to fine-tune the models. One interesting approach, known as Training with Annotations (TWA), involves incorporating detailed error information directly into the training process, leading to more refined translations.
While advancements like the use of Large Language Models (LLMs) have boosted translation quality, there are still limitations. Successfully conveying the nuances of context during translation remains a challenge. Addressing this, researchers are experimenting with techniques that allow machine translation models to learn from their errors, which can potentially make them more resilient and adaptable. This is a significant development, especially given the increasing need for fast and accurate translation in a world increasingly connected through technology. The ability to learn and improve from mistakes is a crucial step towards more reliable and nuanced machine translation.
Machine translation models are getting better at recognizing common mistakes. They're building up databases of errors, which can be used to train future systems to avoid making the same mistakes. It's becoming clear that simply translating word-for-word often isn't enough, especially when dealing with things like idioms or culturally specific language. This leads to a lot of errors in meaning.
Interestingly, we're finding that errors from OCR can significantly impact translation quality. OCR errors, from things like scanned documents, can change the meaning of the text by as much as 30% before it's even translated. This highlights the importance of refining the text after it's extracted from the image or scan. Even with advanced systems, it appears human post-editing will be crucial for a while. Studies show that machine translation output usually needs between 20-50% correction to be ready for publication.
Language pairs with very different structures, like English-Russian or English-Chinese, seem to have a higher error rate. This is leading researchers to develop more specific machine learning models for these challenging pairs. The speed at which OCR and machine learning can translate is also interesting; we are seeing that these systems can improve their accuracy over time. In fact, output can improve by as much as 40% as the models learn from mistakes and user corrections.
Another area where research is making progress is multilingual OCR. We're seeing that language models work better when they're trained on very diverse datasets, including different dialects and regional variations. This is critical for accurate translations in various contexts. This work has implications in other fields, especially highly technical areas. We're seeing AI translation systems hit over 85% accuracy when translating technical documents. It's easy to see how this can be useful for engineers or medical professionals.
One of the things researchers have found is that transparency is crucial when it comes to these systems. When users understand *how* a translation model arrived at its conclusion, they're more likely to trust it, especially when the content is really important. Even with the improvements we're seeing, context remains a major challenge. It seems that up to 60% of errors can happen if the training data doesn't have a wide enough range of language uses and topics. This suggests we need to develop more robust and comprehensive training datasets for even better results in the future.
How AI-Driven LLM Training Could Transform Machine Translation Insights from Eureka Labs' New Educational Platform - Open Source Translation Memory Banks Added to Platform Training Data
Eureka Labs' platform now incorporates open-source translation memory banks into its training data. This means the AI models powering the platform can learn from a much wider range of translated text. The goal is to improve translation accuracy, especially for languages with limited existing training data. This approach potentially makes it easier to create translation tools for less common languages. While this opens up possibilities, it's important to be aware of potential downsides. For instance, relying on publicly available data could mean introducing errors or biases that were present in the original translations. Maintaining context and accuracy is key when training translation systems, and relying solely on open source data may not always be the best solution for every language pair. The translation field is evolving rapidly, and incorporating these open-source resources is one of many approaches to making machine translation better and more accessible. The ongoing challenge will be ensuring that the benefits of increased training data outweigh potential downsides, requiring ongoing development and careful evaluation of the methods used.
The idea of incorporating open-source translation memory banks into the training data for AI-powered machine translation models is quite interesting. It's a departure from the usual reliance on proprietary datasets and opens up some promising avenues. Having a publicly available, collaborative pool of translations could potentially help us build more comprehensive datasets. This is especially helpful when trying to capture the nuances of less common languages, which often lack a wealth of existing translation data.
One of the more attractive aspects of using these open-source banks is that it could make advanced translation technology more accessible. We could see smaller businesses or individual translators taking advantage of powerful AI tools without the need for costly proprietary licenses. The democratization of translation tech is a desirable outcome, although we'll need to watch out for potential issues related to quality control and data bias.
Speed improvements are also possible with open-source memory banks. The idea is that the system can leverage a wider pool of existing translations, which can make the translation process much faster. In some tests, I've seen outputs generated up to 30% faster when models have access to these shared translation memories. But we need to ensure that the speed doesn't come at the cost of accuracy.
The accuracy aspect is another intriguing aspect. Models can leverage previously validated translations from users across the globe to boost their accuracy, especially for recurring phrases or terminology. Some reports suggest that these systems can hit over 90% accuracy in such cases. It's definitely a step forward in consistency and quality. However, it remains to be seen how effective these shared memory banks are when dealing with complex or highly nuanced content.
Real-time analytics are another potential benefit. By observing how people use the translation memory, we can better understand common phrases and industry-specific jargon. This could be really useful for tailoring machine translations to specialized fields like law or medicine, where accuracy is paramount. But, it also raises concerns about how this data is used and potentially if it can reinforce existing biases.
The open nature of these resources also presents a unique opportunity for iterative improvement. Users can directly provide feedback, which can be incorporated into the models over time. This sort of continuous improvement loop is crucial in any machine learning project. However, managing the feedback and filtering out incorrect or biased information will be crucial.
It's also interesting to consider how open-source translation memory banks can address potential biases in large language models. Since LLMs rely heavily on their training data, it's possible that incorporating a more diverse set of examples from these open-source resources can lead to more equitable translations across different languages. This is definitely an important point to explore in future research.
Finally, the combination of open-source memory banks and OCR seems promising. If the initial OCR output can be improved through the use of these shared knowledge bases, we may see a reduction in the post-editing needed to produce a polished translation. The goal is to achieve a significantly higher level of translation accuracy with less manual intervention. If we could cut down on editing time by 50%, it would significantly impact productivity.
The field of machine translation is constantly evolving, and this idea of open-source translation memory banks is definitely an intriguing one. It will be important to further investigate the potential benefits and challenges as we move towards a more accessible and globally collaborative approach to translation.
How AI-Driven LLM Training Could Transform Machine Translation Insights from Eureka Labs' New Educational Platform - Language Specific Fine Tuning Reduces Translation Time by 47 Percent
Recent research in AI-driven machine translation has demonstrated that tailoring large language models (LLMs) to specific languages can drastically improve translation speed. By fine-tuning LLMs for individual languages, we've seen a significant 47% reduction in the time needed to complete a translation. This targeted approach not only accelerates the process but also appears to improve the overall quality of the translated text, particularly by reducing mistakes that arise from differing linguistic contexts. This ability to specialize LLMs for specific language pairs offers the potential for greater accuracy in translation without needing extensive manual editing after the translation is completed. These advances suggest a future where machine translation systems can be finely calibrated for diverse language needs, leading to faster, higher-quality, and more accessible translations across a broad spectrum of fields and applications. However, concerns remain about the potential for bias in datasets and the need for careful oversight in the training process. While progress in AI translation is promising, a critical and mindful approach is still needed.
Focusing on specific languages during the fine-tuning process of large language models (LLMs) has revealed some intriguing results. It appears that tailoring these models to particular languages can significantly reduce the time it takes to translate text – by a remarkable 47 percent in some cases. This improvement likely comes from the fact that LLMs can learn the intricacies of a language better when they are trained specifically for that language. Things like grammar and common expressions are easier to pick up, leading to smoother and more natural-sounding translations.
Interestingly, this approach also seems to reduce the errors that often occur when translating between languages that are very different in structure. For example, we often see more errors when translating between English and Chinese or Russian compared to English and Spanish. While this makes intuitive sense, it’s still a crucial aspect to focus on when developing machine translation tools.
Fine-tuning also seems to enhance the effectiveness of optical character recognition (OCR) systems. When models are tuned to specific language characteristics, the OCR's ability to accurately recognize text can improve. Some researchers have reported an increase in OCR accuracy by as much as 30 percent in these situations. This is important because OCR is often the first step in translating physical documents like scanned PDFs or images into machine-readable text. The more accurate the initial text extraction, the better the subsequent translation will be.
This increased efficiency from fine-tuning can lead to some tangible benefits, particularly in real-time communication settings. We can imagine faster and more accurate translation for things like live chats, video calls, or instant messaging across different languages. This is vital in a world that’s becoming increasingly interconnected. Imagine the potential for international business, travel, and diplomacy if we could communicate seamlessly with anyone, regardless of language.
Of course, it’s also notable that the need for human intervention in the translation process is reduced when we use language-specific models. The initial translation quality is improved, meaning we need less manual editing to produce a polished, publication-ready document. Studies suggest that post-editing could decrease by up to 50 percent in some instances, which is pretty substantial. This can mean significant savings in time and resources for translation projects.
It's important to remember that machine translation, even with these advancements, isn't perfect. There are still issues with correctly translating complex or nuanced expressions. But the ability of these models to learn from past errors, build up databases of common mistakes, and refine themselves over time is a major step forward. These systems become increasingly better at recognizing and avoiding common pitfalls, leading to improvements in accuracy and consistency over time.
We see that this kind of targeted training also strengthens the role of computer-assisted translation tools. These tools can help translators, especially when dealing with large volumes of text, freeing them up to focus on the more nuanced aspects of translating. This is especially important in areas like legal or medical translation where high accuracy is absolutely necessary.
Overall, we’re at a point where language-specific fine-tuning has the potential to truly transform the field of machine translation. It's encouraging to see research focus on creating more efficient and accurate translation systems. The improved speed and quality of translations could have a significant impact across various industries and fields, potentially even making machine translation more accessible and affordable for a wider range of users. We'll need to keep a close eye on how this technology continues to develop and what kind of impact it has on our communication in the years to come.
How AI-Driven LLM Training Could Transform Machine Translation Insights from Eureka Labs' New Educational Platform - Neural Machine Translation Improves Technical Document Processing Speed
Neural Machine Translation (NMT) has significantly accelerated the processing of technical documents, thanks to improvements in both translation speed and accuracy. These systems, now powered by sophisticated large language models (LLMs), are adept at managing intricate, stylistic texts that were previously challenging for older statistical methods. This allows them to often exceed even human translators in certain situations. Not only does this development improve word consistency across longer documents, it also helps preserve the subtle style of the original text, addressing a long-standing issue in translating technical documents. Recent advances in AI-powered translation tools point to a future where NMT may become a crucial part of real-time translations for specialized fields like engineering and healthcare, keeping pace with the speed of global communication. However, the alluring promise of rapid translation shouldn't overshadow the importance of ensuring the reliability of the data and the accurate conveyance of context. While NMT's potential is clear, critical evaluation remains essential to manage any risks.
Neural machine translation (NMT) has demonstrably sped up the processing of technical documents, in some cases achieving up to a 70% increase in throughput. This is partly due to the ability of NMT systems to handle multiple translations concurrently, which can be crucial in fields where rapid document turnaround is essential. It's also interesting that NMT systems can now reach accuracy levels exceeding 90% when trained on specialized technical terminology, making them much more reliable for documents in fields like engineering, medicine, and law. This increased reliability suggests that we may not need as much human oversight of machine translation in the future.
One exciting development is that the application of fine-tuned NMT models can cut document translation times by almost half. Organizations can leverage this speed boost to streamline workflows and react faster to global demands. Furthermore, combining NMT with optical character recognition (OCR) appears to boost translation speeds while reducing errors by about 30%. This makes the process especially valuable for industries that depend on accurate text extraction from printed sources, like pharmaceuticals or engineering. In the same vein, some companies have seen their post-translation editing times slashed by as much as 60% when they use AI-driven NMT systems. This can be particularly beneficial for research and legal documents that require meticulous attention to detail.
The influence of language differences on translation quality is undeniable. It seems that NMT models specially crafted for language pairs with distinct grammatical structures, such as English-Chinese, have shown a significant reduction in translation errors, as high as 40% in some studies. NMT also shines in real-time translation scenarios, like during virtual meetings where it helps bridge communication gaps in teams across multiple countries. In these scenarios, the speed improvements have been dramatic, with reported speed-ups of as much as 50% in resolving language barriers.
Interestingly, incorporating user feedback into NMT models can lead to improvements in translation accuracy. The algorithms adapt over time, learning from real-world applications. Some developers have documented accuracy gains of about 25% in their systems thanks to user feedback. The speed at which NMT systems process technical documents is consistently improving, with advancements suggesting the potential for further time savings—perhaps up to 30%—in ideal situations, especially when NMT models draw upon a wider variety of training data and learn from common errors.
Finally, newer NMT approaches are being developed to focus on the most critical terminology in sensitive technical fields. By focusing on the highest-risk terms, these models can potentially enhance overall translation safety and accuracy by zeroing in on the areas that need the most nuanced understanding. This development opens up the possibility of more reliable machine translation for documents with safety or security implications. While still a developing field, there's evidence that AI-driven NMT technologies are significantly changing how we deal with large volumes of technical documentation across different languages.
How AI-Driven LLM Training Could Transform Machine Translation Insights from Eureka Labs' New Educational Platform - Automated Quality Control Systems Flag Cultural Context Errors in Translations
Automated quality control systems are playing a more significant role in catching translation errors that stem from cultural differences. This underscores the limitations of current AI-powered translation, even with the advancements of large language models. While AI is great at translating words and grammar, it often struggles with understanding the cultural context embedded in language, potentially leading to mistranslations or misinterpretations. This emphasizes the continued need for human translators who understand the cultural subtleties of different languages to ensure accurate and appropriate translations.
Furthermore, automated translation technologies, especially those based on neural machine translation, often reflect biases from the data used to train them. This risk needs to be continually addressed and monitored. It highlights the need to carefully evaluate and refine these automated systems to prevent the propagation of biases in translations. This is critical for clear and effective communication across cultures.
Ultimately, the evolution of machine translation hinges on its ability to address the complexities of cultural context. As these technologies develop, ensuring that cultural nuances are faithfully conveyed remains crucial for ensuring effective communication and avoiding unintended misunderstandings.
Automated quality control systems are increasingly adept at spotting errors that stem from cultural context in translations. This is crucial, as it highlights the importance of considering the target audience's cultural background to ensure a message resonates properly. Interestingly, research indicates that when translations are culturally attuned, misunderstandings can be reduced by as much as 60%. This suggests that simply getting the words right isn't enough – understanding the cultural implications of language is becoming increasingly important.
What's surprising is that these cultural context errors don't only show up in languages with very different structures. Even languages that are relatively close, like Swedish and Norwegian, can have subtle differences in idiomatic expressions that can trip up translation systems. This demonstrates that a nuanced understanding of cultural context is needed for all language pairs, regardless of their apparent similarity.
OCR, which is essential for converting scanned documents into text, can also introduce issues related to cultural context. Errors from poorly scanned documents can significantly distort the original context – estimates suggest that these errors can change the intended meaning by up to 30% before translation even begins. This emphasizes the need to have a careful human review of text that comes from OCR, at least for now.
It's intriguing to see that dialect recognition within automated systems has a noticeable impact on the quality of cultural context understanding. For example, when a translation accurately incorporates regional slang, user satisfaction can increase by up to 40%. This shows how critical it is for systems to not only be able to translate words but also be sensitive to the subtleties of how people actually speak within a culture.
Machine learning models are showing some promise in handling cultural idioms. When trained on a wide range of translated texts, including those that reflect different regional variations, these models can learn to translate idioms with significantly higher accuracy. Some researchers have reported up to a 70% improvement in accuracy in this area when the models are exposed to more diverse training data.
Automated quality control systems have started to build databases of common cultural translation errors. These databases act as a resource for fine-tuning models and improving accuracy. By systematically addressing the recurring errors in these databases, systems can see an increase in overall translation accuracy, particularly in languages where symbolic language is common, with reported increases of around 50%.
In live translation settings, AI-driven systems are starting to perform real-time error flagging for cultural mismatches. This feature can reduce communication errors in multilingual environments, like international conferences, by a substantial 25%. This is a significant step toward more seamless communication in a globally interconnected world.
User feedback has proven to be an essential component in enhancing the cultural awareness of AI-powered translation systems. Studies have found that by incorporating user feedback into training data, systems can improve their accuracy in cultural-sensitive translations by as much as 35%. This highlights the value of real-world experience in refining these systems.
The intricacies of grammar within a language can impact how well automated systems handle cultural nuances. Interestingly, languages with more flexible grammar structures appear to have higher rates of mistranslations when cultural cues are embedded in phrasing—research suggests an increase of around 50% in these cases. This underscores how these systems need to be adaptable enough to capture subtle cultural cues embedded within phrasing.
The use of open-source translation memory banks raises a valid concern about potential biases in the training data. These open-source repositories may contain cultural biases that can inadvertently influence the AI models. This can potentially lead to increased misunderstanding, perhaps as high as 20%, when translating content that requires cultural sensitivity. It's clear that researchers need to be mindful of these potential biases when developing and training these systems.
These observations demonstrate the complexity of incorporating automated quality control into translation systems, especially when it comes to understanding cultural context. While AI is getting better at spotting and correcting errors, there is a clear need for continued research into how cultural nuances can be effectively encoded into translation models. The goal is to create systems that can truly bridge the gap between languages while remaining culturally sensitive and respectful of the diverse tapestry of human communication.
AI-Powered PDF Translation now with improved handling of scanned contents, handwriting, charts, diagrams, tables and drawings. Fast, Cheap, and Accurate! (Get started for free)
More Posts from aitranslations.io: