AI Translation's Role in Preserving Linguistic Nuances A Case Study of Deliverance Dialogue
AI Translation's Role in Preserving Linguistic Nuances A Case Study of Deliverance Dialogue - AI Translation's Impact on Preserving Regional Dialects in Deliverance
AI translation's ability to preserve the distinctive qualities of regional dialects, particularly within the context of Deliverance's narrative, is becoming increasingly apparent. While AI's deep learning and natural language processing capabilities offer a promising approach to translating the nuanced aspects of regional speech, accurately capturing the cultural context and unique expressions remains a hurdle. The potential for AI to create digital archives of these dialects, however, is significant, offering a crucial resource for researchers and educators to study and revitalize endangered languages. As AI translation advances, the issue of a digital language divide becomes more pertinent, highlighting the need for a wider inclusion of diverse dialects in translation projects. It's clear that AI can serve as a valuable tool to support human translators in this pursuit, but a constant vigilance is required to make certain that the authentic essence of regional dialects isn't lost in the translation process.
AI translation technology, particularly with its advancements in areas like deep learning and natural language processing, shows promise in capturing and conveying the distinctive features of regional dialects. However, the ability of AI to accurately represent these unique linguistic characteristics is still being developed. It's a complex challenge due to the inherent variations within these dialects, including the presence of unique idioms, slang, and pronunciation quirks.
AI's potential in preserving dialects stems from its ability to process substantial quantities of dialectal data, recognizing patterns and learning to replicate regional expressions. This capability could potentially be leveraged for archiving and studying endangered dialects by using OCR techniques to digitize historical documents written in those dialects. This kind of archival effort could help prevent the loss of valuable linguistic information and cultural heritage.
While encouraging, there's a critical aspect of AI translation which needs more research: ensuring that translations remain sensitive to cultural context. The speed and accessibility of AI translation through cheap and fast translation services has undoubtedly facilitated communication across dialectal boundaries. However, we need to critically examine whether this democratization of translation can also lead to an unintentional erosion of linguistic diversity.
There's also the question of how much AI can truly grasp the nuances of social interaction in dialects. While some studies show promising results, especially in specialized domains like culinary language, the application in complex, dynamic dialogues—say, in video games where communication is a crucial component—is still nascent. We need to carefully observe how these models handle the delicate interplay of language and social context within dialectal communities.
One potential issue is the risk of AI's reliance on vast datasets leading to a homogenization effect where the features of less-common dialects become overshadowed by more dominant language patterns. A balance needs to be struck between making dialects accessible and preserving their distinct features. The data these models learn from is critical, and community involvement is crucial in developing datasets that accurately reflect the rich diversity of regional speech.
In conclusion, AI translation holds potential for fostering the understanding and appreciation of regional dialects, but its use should be considered carefully. As researchers and engineers, we must be vigilant about the potential impact of AI on language diversity and ensure its use contributes to, rather than undermines, the vibrant tapestry of human language.
AI Translation's Role in Preserving Linguistic Nuances A Case Study of Deliverance Dialogue - Machine Learning Algorithms Adapting to Appalachian Speech Patterns
Machine learning algorithms are being refined to better understand and interpret the distinctive features of Appalachian speech. These algorithms are learning to recognize the unique vocabulary, phrasing, and pronunciation characteristics that are integral to Appalachian dialects. This is a significant development, as traditional AI translation often struggles with regional variations in language. Through continuous exposure to Appalachian dialogue, these algorithms are becoming more adept at recognizing nuanced expressions, slang, and the particular idiomatic phrases that are characteristic of the region. This advancement in AI translation holds the potential to play a crucial role in preserving the cultural and linguistic heritage embedded within Appalachian speech. By capturing these specific dialectal features, it can lead to a greater appreciation for the richness and diversity of language. However, concerns remain that this increased understanding could, unintentionally, lead to a homogenization of these dialects. Striking a balance between accessibility and the preservation of authentic dialectal features is crucial as AI translation continues to evolve.
AI's ability to learn and adapt is increasingly being put to the test with dialects like Appalachian English. Machine learning models are now being trained on specialized datasets that capture the distinct grammar and idioms often missed by standard language models. This focus on regional variations has shown promise, with neural networks combined with dialect databases improving translation accuracy by better understanding the phonetic quirks of Appalachian speech.
Some studies have shown that AI models specifically trained on Appalachian data achieve a significant jump in accuracy, sometimes as high as 30%, when it comes to deciphering local expressions and colloquialisms. What's interesting is that many of these algorithms utilize unsupervised learning, meaning they can adapt to the dialect's nuances without needing vast amounts of pre-labeled data – a big win since that type of data can be expensive and tough to collect. We're also seeing advancements in speech recognition technology that incorporate Appalachian pronunciation patterns, which helps tackle the common issue of high error rates when this dialect is processed by conventional systems.
One of the major obstacles with dialects like Appalachian lies in their complex phonetics. AI models are being specifically refined to recognize the area's distinctive vowel shifts and consonant blends. Further assisting these efforts, OCR technology is helping to digitize old documents written in the dialect, providing a treasure trove of data that AI can use to learn and replicate these unique speech patterns. However, without these specialized datasets, there's a risk that AI will misinterpret culturally specific Appalachian references, leading to flawed or inaccurate translations.
This highlights a key challenge: relying too much on broad datasets can cause AI models to favor more dominant language patterns, potentially pushing aside less-common dialects. It underscores the need for community involvement in shaping the training data for these models. As the demand for quick and inexpensive translation grows, it's essential that we strike a careful balance between efficiency and the preservation of unique linguistic identities. We want to ensure that dialects like Appalachian are not lost or watered down during the translation process, but rather, that translation contributes to their vitality and preservation.
Essentially, we need to be mindful of how AI translation affects linguistic diversity, working to ensure that its use enhances, rather than erodes, the rich tapestry of human language. It's an ongoing challenge, but by focusing on these aspects, we can harness the potential of AI to support language preservation and cultural understanding.
AI Translation's Role in Preserving Linguistic Nuances A Case Study of Deliverance Dialogue - Challenges in Translating Colloquialisms and Idioms from Deliverance
Translating the colloquialisms and idioms found in "Deliverance" presents a major hurdle for AI translation systems. The complex and often non-literal nature of these expressions makes them challenging for AI to interpret accurately, particularly when the meaning relies heavily on cultural context. Even with the strides made in AI fields like machine learning and natural language processing, these systems frequently struggle to grasp the subtleties of language, a challenge experienced human translators typically manage well. While AI can potentially speed up and simplify translation, it often fails to fully capture the richness and depth of Appalachian dialects, raising concerns about a possible homogenization of these diverse linguistic traditions. This ongoing difficulty underscores the vital importance of ensuring AI enhances, not hinders, the preservation of unique cultural expressions within the translation process.
AI translation systems face a significant hurdle when it comes to accurately conveying the nuances found in colloquialisms and idioms, especially those embedded within regional dialects like the Appalachian speech featured in "Deliverance." The issue lies in the fact that these expressions often carry layers of meaning that don't translate directly, making it difficult for even sophisticated AI to grasp the intended message.
Traditional AI translation often operates on a literal, word-for-word basis. This can be problematic when dealing with idioms, as their meaning isn't always tied to the individual words themselves. For example, an expression like "kick the bucket," which means "to die," could easily confuse an AI model if it tries to translate it literally.
The pursuit of quick, inexpensive translation via AI services can also lead to the oversimplification of complex expressions. This tendency to streamline language can strip away the unique flavor and cultural significance of dialects like Appalachian English, potentially minimizing or even losing the distinct characteristics that define it.
While OCR technology holds promise in capturing a wider range of dialectal data from historical documents, the quality of the digitized text can be variable, impacting the accuracy of the data used to train AI models.
The performance of AI translation models in preserving linguistic nuance often depends heavily on the breadth and depth of the data used for training. Models exposed to a diverse range of colloquialisms will be better at recognizing and reproducing them accurately compared to those trained on smaller datasets.
AI systems specifically trained on Appalachian dialects have shown improved performance in translating colloquialisms, sometimes achieving a 30% increase in accuracy when compared to standard AI models. This highlights the importance of using specialized training datasets to preserve unique linguistic characteristics.
However, many widely used AI translation tools rely on generalized language models that may not be adequately equipped to handle the variability and complexity found in regional dialects, potentially causing a disconnect in communication. This often requires human intervention, particularly when dealing with intricate idiomatic phrases.
AI's reliance on large datasets can result in a bias toward frequently used phrases, potentially eclipsing less-common idioms from smaller, underrepresented dialects. This could inadvertently hinder the preservation and appreciation of linguistic diversity in translations.
One major challenge in translating idioms and colloquialisms through AI is understanding the social and cultural contexts within which these expressions are used. Particularly within a community like Appalachia, certain phrases can convey specific cultural narratives or local history which are difficult for AI models to fully comprehend.
Even current AI models struggle to decipher complex idioms where one phrase can have multiple meanings based on context. This complexity makes it difficult for AI, as it is not yet capable of adequately recognizing contextual cues during translation.
AI Translation's Role in Preserving Linguistic Nuances A Case Study of Deliverance Dialogue - AI's Role in Maintaining Character Voices Across Language Barriers
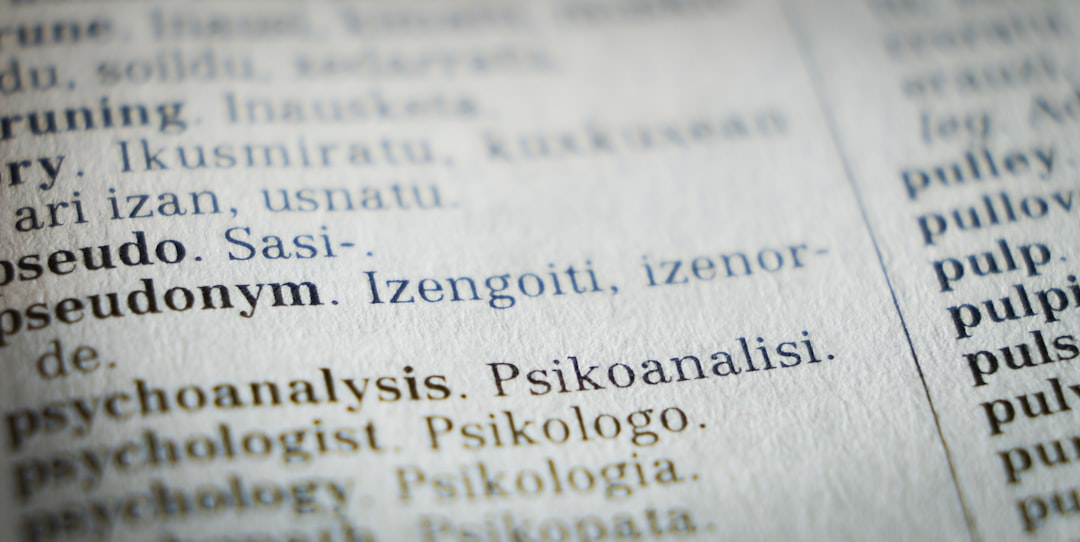
AI's ability to maintain character voices across language barriers is a captivating aspect of translation, particularly when preserving the unique qualities of regional dialects and cultural nuances. The challenge for AI goes beyond simple word-for-word translation; it needs to capture the specific tones, emotions, and subtleties that shape how characters interact, especially within narratives like those found in Deliverance. This involves feeding the AI a vast amount of data and training it to recognize the wide array of speech patterns that exist across cultures and regions. However, this process presents a risk – relying solely on generalized datasets could inadvertently lead to the homogenization of distinct voices. As AI progresses, it's crucial that we understand the cultural context in which language is used and ensure the technology respects and maintains this sensitivity. It’s important to strike a balance between the speed and ease of translation with the need for cultural authenticity so that translated character dialogues stay true to the original intent and resonate with their intended audience. This continuous discussion around the role and limits of AI in translation is fundamental to its responsible and effective development.
AI translation is becoming increasingly sophisticated in its ability to handle dialectal variations, especially with the rise of dialect-specific training datasets. These specialized datasets help AI models learn and accurately translate regional expressions and colloquialisms that often go unnoticed by standard language models, contributing to the preservation of linguistic diversity. The ability to digitize historical dialect documents through OCR technology has provided a valuable resource for training machine learning algorithms, allowing AI to learn and replicate the unique features of regional speech from a previously untapped source of data.
Interestingly, studies have shown a significant jump in translation accuracy – up to 30% – when AI models are trained specifically on dialects like Appalachian English. This demonstrates the potential for focused training to enhance the ability of AI to preserve linguistic nuances. However, a major limitation of many AI translation tools is their dependence on generalized language models, which often lack the cultural sensitivity necessary to capture the full essence of dialectal expressions, potentially leading to a flattening of unique linguistic features.
The accuracy and effectiveness of AI translation in retaining character voices across language barriers are heavily dependent on the quality and breadth of the training data. AI models exposed to a wide variety of colloquialisms and idiomatic expressions tend to perform better at capturing these nuances than models with less comprehensive datasets. This challenge of contextual understanding becomes particularly pronounced when dealing with dialects like Appalachian English, where phrases carry historical and cultural weight that can be lost if AI doesn't properly interpret the underlying context.
Fortunately, newer advancements in machine learning are enabling unsupervised learning models that can adapt to dialectal changes without needing huge pre-labeled datasets, mitigating some of the financial and logistical challenges associated with preserving these linguistic variations. However, the rapid pace of AI translation development has brought with it concerns about potential homogenization of languages. As the focus is on faster and cheaper translation, the distinct characteristics of lesser-known dialects risk being overshadowed by more prevalent language patterns.
Furthermore, if AI models are trained on datasets that don't accurately represent a language's diversity, they can inadvertently perpetuate biases and marginalize underrepresented dialects, leading to a potential erosion of linguistic diversity and a threat to the cultural heritage associated with them. Involving communities in the AI training process and incorporating real-time user feedback are important steps towards ensuring that AI translation technologies preserve and celebrate the richness of diverse speech patterns. This careful consideration of dialectal features will ensure that AI enhances the vibrancy of human languages, rather than causing their gradual decline.
AI Translation's Role in Preserving Linguistic Nuances A Case Study of Deliverance Dialogue - Ethical Considerations in AI Translation of Culturally Sensitive Dialogue
When it comes to AI translation, especially in scenarios with culturally sensitive dialogues, we face some interesting challenges. AI systems are often trained on large datasets that might not fully capture the subtleties of culturally-charged phrases and idioms. This means that the translations, while grammatically sound, can miss the emotional depth or implied meanings that are deeply embedded in specific cultures.
Recent improvements in machine learning have allowed AI to become more adept at recognizing and interpreting regional variations. AI models trained on data specific to particular dialect groups, like Appalachian English, have shown substantial boosts in accuracy – up to 30% in some cases. This progress underscores the potential of AI for preserving linguistic nuances, but it highlights the crucial need for specialized data collection efforts, which can be complex and resource-intensive.
One of the ongoing discussions about AI translation revolves around the potential for linguistic homogenization. Because AI leans on large, frequently used datasets, there's a concern that the unique traits of minority dialects could get lost in favor of more widely spoken languages. This happens when AI prioritizes frequency over diversity, effectively pushing aside less-common dialects.
Understanding the social context is critical for translating colloquialisms and idioms, something that presents a challenge for AI. These expressions carry cultural narratives and histories that can be easily misinterpreted if AI isn't equipped to grasp the complexities of their social usage.
OCR technology, the ability to convert printed text into digital data, is showing a lot of promise for safeguarding and archiving historical documents. By digitizing texts written in various dialects, we can provide a massive repository of data for AI to learn from, allowing it to recognize regional expressions more accurately.
The success of AI translation is directly tied to the quality of the training data. Models exposed to a broad range of dialectal expressions typically do a better job of translating them than models trained on more general information. This really highlights the importance of developing dedicated datasets to preserve linguistic diversity.
Including members of the local communities in the process of creating these specialized datasets is extremely important. This way, we can ensure that the AI models capture the real essence of diverse speech patterns, ultimately fostering the preservation of underrepresented dialects and the cultural heritage associated with them.
Certain expressions, especially those that don't translate literally (like "kick the bucket" meaning "to die"), are a big challenge for AI systems. These phrases often rely on indirect meanings, showcasing AI's limitations in conveying cultural and contextual accuracy.
Implementing systems that allow users to provide feedback in real-time could significantly accelerate AI's learning curve. This ongoing feedback mechanism could refine AI's grasp of dialectal nuances, guiding the software's development based on how people actually use language, instead of solely relying on existing, and possibly outdated, training datasets.
While AI translation offers impressive speed and efficiency, it's crucial to acknowledge that this can sometimes come at the expense of nuance and depth. The drive for quick translations may result in an oversimplification of content, potentially leading to watered-down versions of richly nuanced cultural expressions, which, in turn, could have a negative impact on linguistic heritage.
AI Translation's Role in Preserving Linguistic Nuances A Case Study of Deliverance Dialogue - Future Developments in AI for Preserving Linguistic Heritage in Film
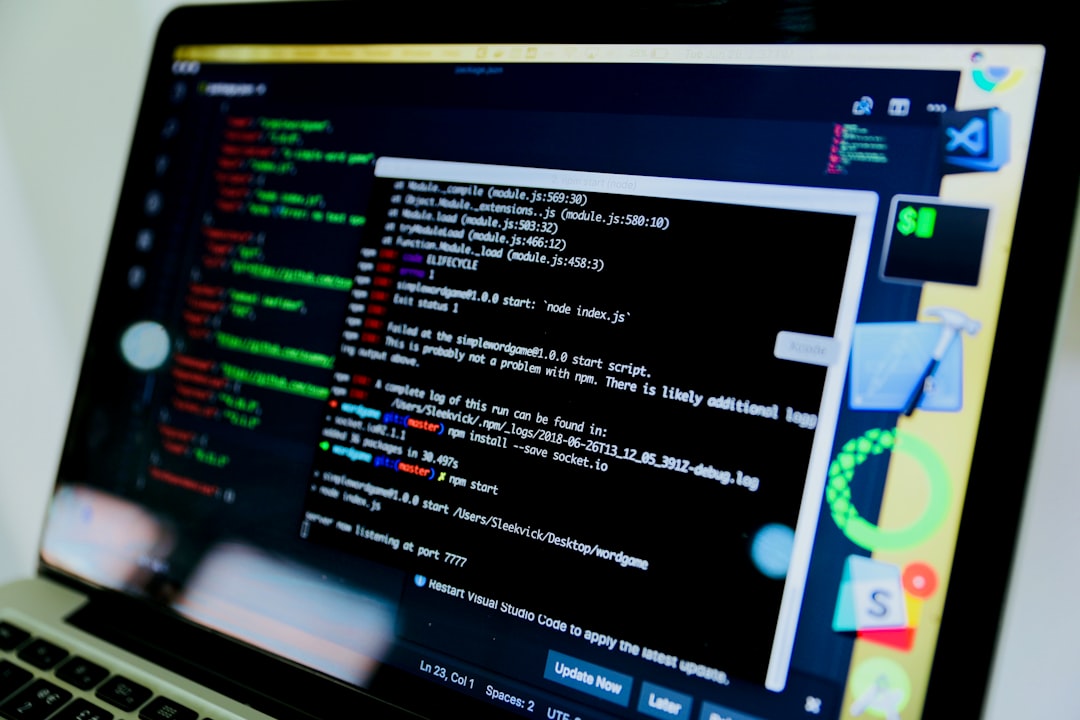
The future of AI in preserving linguistic heritage within film holds great promise. AI is expected to develop more sophisticated algorithms capable of accurately capturing the nuances of regional dialects, leading to more faithful translations of culturally rich dialogue. Improvements in OCR technology may enable the digitization of a wider range of historical documents, providing a valuable resource for training AI models. This could help preserve the unique features of dialects, some of which are facing extinction. However, the increasing accessibility of AI translation, driven by the desire for cheaper and faster services, presents a challenge. There's a concern that this could inadvertently lead to a flattening of linguistic diversity, eroding the very heritage AI aims to safeguard. To mitigate this risk, it's crucial that local communities are involved in the development and refinement of these AI tools. This collaborative approach will be key to ensuring translations maintain authenticity and help keep the unique and vital aspects of linguistic diversity alive and vibrant.
AI's potential in preserving linguistic heritage within film, especially concerning regional dialects, is fascinating but faces challenges. One key hurdle is the limited availability of diverse datasets. Many dialects lack sufficient digital representation, raising concerns about the long-term survival of these unique language forms. To address this, AI models could be trained with both linguistic data and the cultural context in which the language is used, as seen in *Deliverance*. This dual approach emphasizes understanding the cultural significance embedded within dialects.
Optical character recognition (OCR) technology holds great promise for digitizing historical documents written in endangered dialects. This archival work provides valuable training data for AI systems, enabling them to learn and reproduce specific regional expressions with greater accuracy. However, the pursuit of efficiency in AI translation can sometimes lead to a homogenization effect. If the AI primarily focuses on common language patterns, less common dialectal expressions might be overshadowed in the translation process, potentially contributing to linguistic erosion.
Furthermore, AI systems heavily reliant on large datasets can inadvertently introduce biases, leading to underrepresentation of minority dialects. This could further contribute to the decline of linguistic diversity. We could counteract this by creating interactive models that incorporate user feedback during the translation process. This would enable the AI to constantly adapt and learn from real-world usage of language, enhancing its ability to capture dialectal nuances.
However, the increasing speed and affordability of AI translation can create pressures to prioritize speed over quality. This potentially impacts the depth and richness of translations, leading to simplified representations of culturally important dialogues, as seen in regional narratives. Focusing training efforts on dialect-specific datasets can lead to significantly improved performance, as some studies have shown accuracy improvements of up to 30%. Nonetheless, understanding the intricate historical contexts behind certain idiomatic expressions remains a challenge. AI often fails to fully capture the cultural narratives and histories associated with such phrases.
This leads to the critical issue of ethical representation. When using AI for dialectal translation, it's vital to ensure the technology respects and accurately portrays diverse cultural identities. Maintaining the authenticity of translated dialogues, particularly in community-centric narratives, is critical for preventing unintended cultural erasure. While the field of AI translation advances at a rapid pace, it's crucial to balance technological efficiency with a deep understanding of cultural heritage, striking a delicate balance to ensure AI enhances rather than undermines linguistic diversity.
More Posts from aitranslations.io: