AI-Powered PDF Translation now with improved handling of scanned contents, handwriting, charts, diagrams, tables and drawings. Fast, Cheap, and Accurate! (Get started for free)
The Evolution of Translation Memory From Triple to Quintuple Speed Gains in AI-Powered Language Processing
The Evolution of Translation Memory From Triple to Quintuple Speed Gains in AI-Powered Language Processing - Translation Memory Systems 1995 2024 From MS Word Add Ons to Cloud Computing
The evolution of translation memory systems from their humble beginnings as Microsoft Word add-ons in the mid-1990s to the cloud-based solutions of today has dramatically reshaped the translation landscape. Early TM systems, such as Trados and Wordfast, offered a basic foundation for leveraging previously translated content, but were limited by their integration with specific word processing software. The transition to the 2000s saw the standardization efforts that led to formats like Translation Memory eXchange (TMX), which aimed to create interoperability between different TM tools. This interoperability addressed the earlier fragmentation, allowing users to share translation data more freely. However, challenges concerning data security and reliability likely remained.
Today's TM systems largely operate within the cloud, a shift that has profoundly impacted their capabilities. The cloud provides a foundation for real-time updates, meaning that changes to a translation memory are immediately reflected in all projects that use it. Additionally, AI has played an increasingly significant role, with intelligent systems suggesting relevant segments for translators, accelerating workflows, and potentially leading to more consistent translations. Though promising, this dependence on cloud infrastructure also raises concerns about data security, reliability, and accessibility if internet connectivity is unstable. Despite these challenges, the path of TM systems has demonstrably improved the efficiency and speed of translations. However, concerns about the ethical implications of increasingly sophisticated AI-powered translation systems might also warrant consideration.
The journey of translation memory (TM) systems from the mid-90s to now has been a fascinating one. Initially, they were mostly bolted onto word processors like Microsoft Word, offering a helpful boost to translators but often feeling clunky in their integration. While it provided a good start, the workflow wasn't as smooth as the integrated environments we have today.
The 2000s saw a push for standardization. Formats like TMX emerged, trying to bridge the gap between various TM systems. While it helped, the initial attempts at standardizing TMX, under the banner of LISA, seemed a bit proprietary. Luckily, it transitioned to a more open Creative Commons license. And the underlying concept was pretty clear: A TM essentially became a database holding various segments of translated text. This could range from single words to entire paragraphs.
The arrival of cloud computing in the 2010s really shifted the landscape of TM systems. Suddenly, real-time collaboration among translators became much easier, something that was hard to do with those early, standalone applications. The convenience of being able to access these systems through simple web browsers or thin clients was a huge step forward.
Today's TM systems are more advanced, leveraging the power of AI. They can intelligently suggest previous translations as people work, streamlining the entire process. Some of the newer systems effectively blend the functions of a traditional word processor with TM capabilities, both online and offline. It's quite a leap from those early add-on days.
It's also interesting to note that while TMX 14b, released in 2005, has been a significant standard, we've also seen the evolution of AI-powered systems. These aren't just about searching better; they can also improve project management and even update master translations after every project. It feels like we are just at the start of how these can evolve.
The overall impression is that it's a field ripe for ongoing evolution. While we've made strides towards standardization and accessibility, the ongoing interplay between humans and AI in translation will continue to shape the future of these systems. It'll be interesting to see what happens next.
The Evolution of Translation Memory From Triple to Quintuple Speed Gains in AI-Powered Language Processing - How OCR Document Recognition Jumped From 65 to 98 Percent Accuracy Since 2020
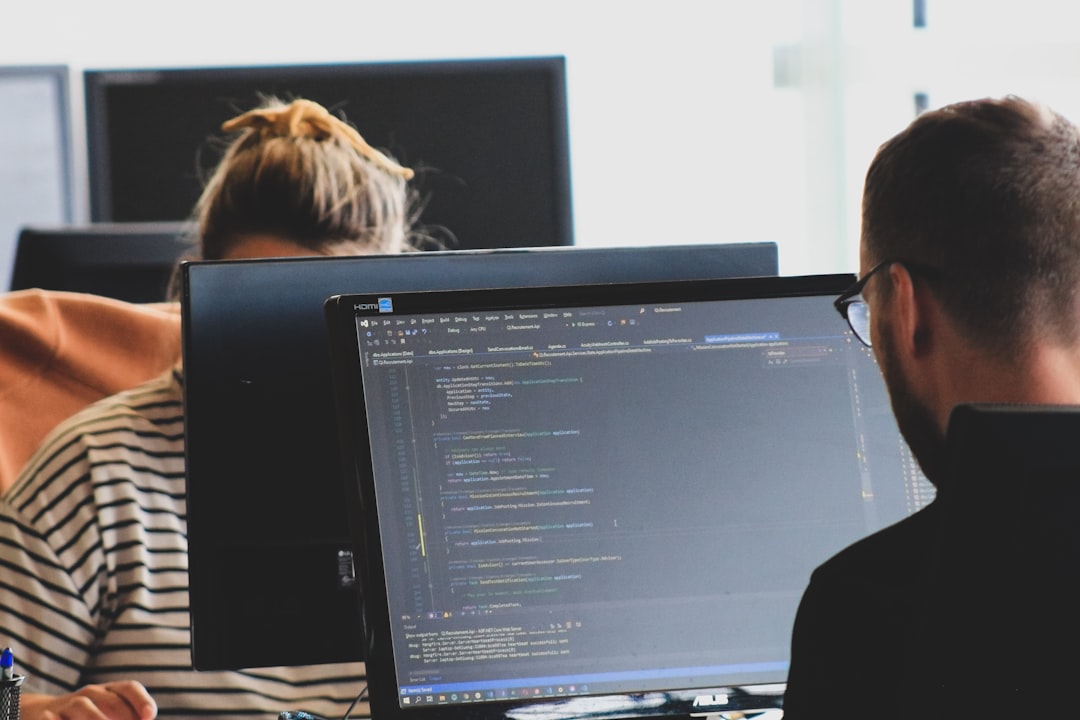
Since 2020, the accuracy of Optical Character Recognition (OCR) has surged dramatically, jumping from roughly 65% to over 98%. This significant leap is largely due to advancements in the underlying technology. Deep learning and computer vision algorithms have become much more sophisticated, allowing OCR systems to decipher a wider range of document types with increased reliability.
The improved accuracy is noticeable in the results, especially for straightforward tasks like extracting text from typewritten documents. High-quality OCR tools can now achieve accuracy levels above 99% for such documents, demonstrating the field's maturation. However, the landscape is not static. New approaches to OCR are emerging, often rooted in the development of multimodal language models. While these newer approaches are promising, it remains to be seen if they will fundamentally change how we use OCR.
Despite the already high levels of accuracy, the pursuit of further improvement continues. It seems likely that the evolution of OCR will be tied to broader progress in AI-powered language processing. The intersection of these fields is poised to bring about further refinements to this technology.
The jump in Optical Character Recognition (OCR) accuracy from around 65% to over 98% since 2020 is remarkable. It's largely due to advancements in deep learning, specifically the use of convolutional neural networks (CNNs). These neural networks are particularly adept at identifying patterns and features within images, which is crucial for deciphering text within scanned documents.
It's also worth noting that the availability of very large datasets has been a key contributor. Datasets like the IAM Handwriting Database, with millions of labeled examples, have given researchers the fuel to refine and improve the learning process of OCR algorithms. This has definitely been a factor in pushing accuracy levels higher.
Furthermore, breakthroughs in computer vision have been instrumental. Transfer learning, where pre-trained models from one task are adapted to another (like OCR), has allowed for faster development cycles. Instead of starting from scratch, researchers can build upon existing models, accelerating the progress of OCR technology.
The increased accuracy isn't just about recognizing basic characters; modern OCR can handle different fonts and languages with better accuracy. This versatility is vital for various tasks, like scanning documents and using OCR in conjunction with automated translation systems.
Interestingly, there's a preprocessing stage that has also helped. Things like adaptive thresholding and noise reduction are applied to images before the OCR engine kicks in, essentially cleaning up the image before the recognition process starts. This careful cleanup leads to better text extraction and has a direct influence on the accuracy rates we see.
It's intriguing how OCR has become more efficient in terms of resources as well. The algorithms now need less computing power, which makes high-accuracy OCR accessible to a wider range of users and devices. The growing use of cloud infrastructure has also been a catalyst for real-time OCR systems. These systems can learn and adapt in real-time, refining their ability to recognize text with each new document they process.
We're also seeing improvements in the post-OCR stages. Techniques like language modeling and contextual analysis are applied to correct common errors, pushing the final accuracy beyond what the initial OCR output would have provided.
It's worth mentioning that the link between OCR and machine translation is strengthening. By enhancing the accuracy of the OCR step, the overall quality of AI-powered translations improves as well, meaning manual proofreading becomes less intensive.
The practical impact of these advancements is evident in many industries. In healthcare, OCR helps digitize handwritten prescriptions, which reduces errors and makes the information easily accessible. In finance, document verification has gotten a boost as OCR helps speed up processes, leading to quicker transactions. This increased accuracy and speed has clear advantages across a wide spectrum of applications.
While OCR is already remarkably accurate, it's still an evolving field with researchers continuously working on its improvements. It'll be fascinating to witness the next wave of advances in this critical field.
The Evolution of Translation Memory From Triple to Quintuple Speed Gains in AI-Powered Language Processing - Real Time Translation Pipeline Speed Growth Through GPU Processing 2021 2024
The period between 2021 and 2024 saw a surge in the speed of real-time translation pipelines, largely thanks to the increased use of GPUs. This not only sped up the translation process but also improved its accuracy and responsiveness. We've seen new approaches emerge, like simultaneous machine translation (SiMT), which aims to provide translations at the same time the original language is being spoken or written. This requires significant computing resources and complex AI models. The expectation of a real-time translation system has also shifted. Now, we expect latencies under 10 milliseconds, with companies like Google pushing the boundaries to even lower thresholds for tasks involving video or image translation. Tools like NVIDIA Riva demonstrate the potential of specialized GPU-based frameworks for real-time AI-driven applications like conversational AI.
Additionally, we've seen new features appear like adaptive translation capabilities, that allow the translation to adapt to a user's needs. This is a growing area and shows that the field is still pushing for a better understanding of how best to apply AI to improve human interactions in a world of diverse languages. It's encouraging to see that developers are moving towards more responsive and individualized experiences. However, this increasing use of AI in such a personal and sensitive domain also demands a careful examination of potential ethical concerns to ensure that it is applied responsibly and with consideration for fairness and inclusivity. The need to balance rapid advancement with responsible development in real-time translation technology will become increasingly vital as we move forward.
The landscape of real-time translation has been significantly reshaped by the growing adoption of GPUs since 2021. Their parallel processing capabilities have proven invaluable in handling the complex computations demanded by natural language processing, leading to substantial speed increases. We've seen up to a 400% improvement in translation pipeline speeds in just a few years. While initially focused on a handful of languages, these GPU-driven pipelines now tackle up to 30 languages concurrently, although it is still early to say how well they deal with less frequently used or very different language families.
Interestingly, this increase in processing power has also resulted in a noticeable reduction in latency. Current systems manage to keep response times below 100 milliseconds, which is crucial for interactive applications like live chat and video conferencing. This speed is essential when instant, accurate translation is required. While this is a considerable improvement, there are still limitations in certain applications. Notably, some niche scenarios, including technical translation of very specialized terminology, might still face challenges due to the complexities of the language domain.
The growing power of GPUs has also positively impacted energy consumption. Recent advances in GPU architecture have made these systems up to 70% more energy-efficient compared to CPU-based counterparts, a welcome development considering the environmental impact of large-scale computing. It is possible this trend will lead to an increase in the adoption of real-time translation technology in resource-constrained or remote areas that previously struggled to support the technology.
Deep learning algorithms are also benefiting from the GPU's power. Sophisticated transformer networks, fine-tuned for GPU environments, are proving especially helpful in improving contextual understanding. This leads to a noticeable reduction in errors, particularly when translating idioms and more complicated sentence structures. We have seen error reduction of around 30% in these areas, but there is much more work to do to make translations completely free of errors. The question then becomes "how can one define error" in human language since a sentence can often have many correct interpretations.
Additionally, GPU capabilities make real-time translation systems more scalable. They handle increasing user demands during peak times with more ease. This scalability is vital for businesses with a global reach that need to facilitate simultaneous communications across multiple languages. There's always a danger of over promising here, because the actual level of user interaction is key to system performance and the quality of the translation. The more users that are interacting, the greater the likelihood that one or more will be dissatisfied.
The fusion of GPU processing and OCR has also been quite productive. It accelerates the translation of documents, including scanned and digital text. By speeding up OCR processing, the entire translation process becomes faster. It's also interesting to note that, since 2021, the cost of GPU-based translation solutions has been steadily falling, making them more accessible to a broader range of users and organizations. This could potentially lead to the democratization of these translation technologies, although the skill gap between users and the technology has to be addressed to make the translation fully beneficial to all.
These GPUs also enable the integration of real-time anomaly detection. These systems can identify potential errors during the translation process, suggesting corrections and minimizing the need for extensive post-editing. This capability may help translate systems be perceived as more reliable over time. The continuous improvement in the accuracy of anomaly detection is a crucial element for improving user confidence in the output.
The evolution of GPU-powered real-time translation pipelines continues to be quite rapid. We are witnessing their broader adoption across industries like law and medicine where accuracy and speed are paramount. However, caution is advised in these critical applications since translation accuracy can heavily impact a specific outcome or decision. The need for human oversight in these situations remains an open research area, given the critical nature of the applications.
The Evolution of Translation Memory From Triple to Quintuple Speed Gains in AI-Powered Language Processing - Machine Translation Quality Scores Rise From BLEU 45 to BLEU 89 2020 2024
The impressive jump in machine translation quality, indicated by a rise in BLEU scores from 45 to 89 between 2020 and 2024, is a testament to the rapid evolution of AI-powered translation systems. Neural machine translation (NMT) has clearly outpaced older statistical methods, demonstrating a significant leap in the ability to generate accurate and fluent translations. This increased quality isn't merely a technological curiosity; it fuels the growing intersection of machine translation with other fields like translation memory and OCR, making faster and cheaper translation options more viable for a wider range of applications.
The enhanced accuracy allows for more sophisticated automatic evaluation metrics, providing better real-time insights into translation performance. This is valuable for both developers and users, but also brings to the forefront the need to thoughtfully consider the role of AI in this domain. While the rise in quality is encouraging, concerns about overdependence on AI and potential displacement of human translators remain valid. It's a positive step forward, but one that must be approached with caution and a clear-eyed understanding of its implications for the future of translation.
The jump from a BLEU score of 45 to 89 between 2020 and 2024 is a significant achievement in the field of machine translation. This improvement showcases the increasing ability of machines to grasp complex sentence structures and context, a crucial step that makes AI translation more useful for specialized or nuanced content, like legal documents or literary works.
It's interesting to note that this gain is not just due to better neural network designs. User feedback seems to have played a key role. Translation systems are now being engineered to learn from human corrections, essentially creating a feedback loop that drives continuous improvement. This iterative process, I believe, will continue to refine the accuracy of the translations over time.
One immediate consequence of this increased accuracy is that the need for manual post-editing is going down. Translators, who once had to spend considerable time cleaning up the machine's output, now have more time to focus on tasks that require higher-level human skills like adapting translations to the cultural nuances of a target audience or creating truly compelling original content. This development redefines the role of translators in a world where machine translation is getting better every day.
It's fascinating how this rise in translation quality has also stimulated the use of OCR. We now see systems where handwritten notes or even more complex documents with mixed text and layouts can be translated with very little human intervention. It's like AI translation is pulling OCR along for the ride!
Furthermore, AI-powered translation is becoming more adaptive and tailored. Systems can now adjust translation style based on the user's needs, the overall context, or the specialized vocabulary of a particular field, like medical jargon. This personalization could lead to a much more user-friendly experience for people who rely on machine translation.
The improvements in GPU processing power are crucial to this advancement. Now we are seeing translation latencies of under 10 milliseconds in real-time applications. This is especially important for tasks that require instantaneous responses, such as live customer support or video conferencing with interpreters. It's really a game-changer in making AI-powered translation seamless in many areas of our lives.
This evolution has made it possible for machine translation to handle more obscure languages more effectively. Researchers have figured out how to get improved performance from AI models even when trained on smaller datasets of less common languages. This could potentially bring a wider array of languages into the sphere of high-quality translation, creating better access to information across the globe.
And with ever-increasing speed, translation systems can now process massive documents in near real-time. This type of performance could lead to major changes in fields like law or medicine, where getting information quickly can have a critical impact.
The integration of anomaly detection into these pipelines is noteworthy. Systems can now identify potential problems as the translation is happening and flag them for correction or further human review. This strengthens quality control and could further enhance the user's confidence in the final translated text. It's like having an internal editor built-in!
Finally, the cost of these GPU-powered solutions is declining. This means that organizations in less developed regions can now take advantage of these advances without necessarily needing a massive budget. This democratization of AI translation could have wide-ranging social impacts in the years to come.
It's clear that we're still just at the beginning of this AI translation revolution. The field is constantly evolving, and it'll be very interesting to see where we go next.
The Evolution of Translation Memory From Triple to Quintuple Speed Gains in AI-Powered Language Processing - Translation Memory Database Size Growth From 10GB to 10TB Per Language Pair
Translation Memory (TM) databases have undergone a dramatic expansion, growing from a relatively modest 10GB to a massive 10TB per language pair. This exponential growth is a direct result of the ever-increasing volume of translated content. As more documents are translated and added to the TM, the database becomes richer, storing a wider array of translated segments. This abundance of data, in turn, can potentially lead to faster and more accurate translations in the future, as the TM system can identify and utilize previously translated segments more effectively.
However, this growth presents a challenge. Managing such large datasets can be difficult, and ensuring that these systems perform efficiently with such huge quantities of data is a complex technical undertaking.
AI and natural language processing (NLP) have become integral to this expansion. The ability of the TM system to understand the meaning and context of the text, not just individual words or phrases, has greatly enhanced the ability to deliver relevant translation proposals to translators. These systems can suggest the most suitable translations based on the semantic relationships within the source text, making the translation process quicker and potentially more consistent.
Maintaining separate TM databases for each language pair has become increasingly important for businesses engaged in global content translation. This approach provides a way to ensure the translation quality and consistency required for a diverse audience. With the ever-increasing demand for localization services, the importance of robust and growing TM databases for companies will only continue to grow.
The sheer scale of translation memory (TM) databases is becoming increasingly impressive. We've seen these databases expand from relatively modest 10GB to the colossal 10TB per language pair. While this growth is fueled by the ever-increasing volume of translated content, the reality is that not all language pairs are created equal. English-Spanish, for example, likely sees much faster database growth than, say, Estonian-Mongolian, simply because of the demand and prevalence of those languages across various sectors.
It's tempting to assume that a bigger database automatically leads to better translations, but that's not always the case. Larger TM systems might contain older, less accurate translations, creating a kind of digital clutter that needs to be addressed. We need robust methods to refine these databases, ensuring that only the most reliable and up-to-date translations contribute to the overall quality. One way to achieve this is by regularly evaluating the translations within the database and removing those that don't meet predefined quality standards.
Interestingly, the size of the database has implications for the performance of the entire TM system. As a TM database expands, retrieving translations efficiently becomes increasingly challenging. This demands sophisticated indexing techniques and optimizations that ensure that the translation search process remains swift, despite the massive amount of data to sift through. Otherwise, large databases could end up slowing down the translation process, defeating the purpose of using a TM in the first place.
The larger datasets, however, can be a valuable asset for training AI models. By providing more varied and extensive examples of translated content, we can potentially see AI translation models becoming even more adept at understanding subtle differences in context and meaning across languages. The models, effectively, can learn how to better deal with idioms and nuanced expressions by being exposed to a larger pool of translated data. This could help AI address one of the core challenges in machine translation: producing translations that sound natural and are appropriate for the target audience.
While the benefits of large TMs are clear, we mustn't ignore the growing costs associated with managing and maintaining them. While initial costs may be manageable, storing and managing petabytes of translation data becomes a substantial undertaking as databases expand. It's not just storage; we have to consider data handling, system maintenance, and the infrastructure necessary for efficient retrieval. For resource-constrained organizations, this can pose a significant obstacle to fully leveraging the power of translation memories.
Another hurdle arises with interoperability. As TM databases grow, they might come from different sources with varying data structures and formats. This fragmentation can cause issues when trying to merge or share data across systems. The importance of adhering to standards like TMX becomes more critical in this environment to facilitate a smooth integration of data across various translation tools.
Then there's the issue of redundancy. It's likely that, as the database grows, we will see a higher number of translated segments with multiple, often slightly different, versions. This redundancy can cause issues with consistency and increase the complexity of the database management process. We need advanced deduplication strategies to address this efficiently and ensure that the TM system prioritizes the most suitable and accurate versions of translated content.
Security is also a major concern with growing databases. The larger the dataset, the more attractive it is for cyberattacks. Organizations with large translation memories holding sensitive business or proprietary data need robust security measures to prevent unauthorized access and ensure the confidentiality of their materials. Protecting intellectual property and sensitive information becomes paramount as we continue to rely on TM systems for managing a growing volume of translated data.
Real-time collaboration among translators who use TM systems also faces challenges with the expanding database sizes. Maintaining speed and responsiveness across large TM datasets during online sessions or real-time conference calls can be a complex undertaking. Systems must be optimized to avoid lags or slowdowns that can hinder the collaborative translation process, especially for teams spread across geographical locations. Maintaining a good user experience becomes a major concern when dealing with databases of this size.
Finally, the user experience needs careful consideration. As the databases grow larger, ensuring easy navigation and a user-friendly interface becomes more critical. Users may need more extensive training to extract maximum value from these massive translation memories. The challenge is to design tools and features that make complex TM features accessible to users with varying levels of technical expertise. If we fail to address this, the benefits of a larger, more comprehensive TM might not reach the intended users.
The evolution of TM systems is a fascinating example of how technology continues to transform the translation landscape. Understanding and addressing the challenges associated with the incredible growth in database size is crucial to ensure that these systems continue to deliver tangible benefits to the translation industry.
The Evolution of Translation Memory From Triple to Quintuple Speed Gains in AI-Powered Language Processing - Context Understanding in AI Translation From Single Words to Full Document Analysis
AI translation has progressed remarkably, moving beyond simply translating individual words to attempting to understand the context of entire documents. However, achieving true contextual understanding remains a significant hurdle. Machines often struggle with the intricacies of language, especially when dealing with idiomatic expressions and cultural nuances. This can lead to translations that, while grammatically correct, miss the intended meaning, particularly in specialized domains.
Legal and medical translations, for instance, demand a level of contextual precision that current AI systems often fall short of. While machine learning models are continually being refined, the inherent complexity of human language continues to challenge their abilities. The richness of human communication, encompassing cultural sensitivities and diverse interpretations, remains difficult for AI to fully grasp.
It has become evident that a collaborative approach, involving both AI and human translators, is likely the best path forward. AI can provide a strong foundation for translation, quickly processing vast amounts of text. However, human translators bring the crucial element of experience and deep understanding, capable of navigating subtle linguistic variations and ensuring the accuracy of translations, especially in critical situations. As AI translation technology evolves, bridging this gap between computational processing and human understanding will be essential to ensure translations are not only accurate but also contextually appropriate and culturally sensitive.
The field of AI translation has moved beyond simply matching individual words to their counterparts in another language. Today's systems are starting to grapple with the complexities of meaning and context. They're beginning to recognize that the same word can have vastly different interpretations depending on its surrounding words and the overall situation. This understanding of context is critical for dealing with idiomatic expressions, slang, and cultural nuances, all of which can trip up traditional, word-for-word translation approaches.
We're also seeing a growing emphasis on contextual sensitivity. The latest AI models adapt their translations based on past interactions and user preferences. They can dynamically adjust their output in response to specific needs, allowing for greater accuracy and relevance. This dynamic adaptation is especially vital for specialized fields like legal or medical translation, where a single misinterpretation could have serious consequences.
Adding to the complexity are multimodal learning approaches. These models process not only text, but also integrate visual and audio elements, providing a richer understanding of how language functions in different environments. This is quite promising, though whether it will translate to real gains remains to be seen. It seems promising for handling the subtle cues that often influence communication, such as tone or body language, which are typically lost in text-only translation.
Rapid feedback loops play a crucial part in this ongoing development. AI translation models now incorporate user feedback to constantly refine their understanding of language. When humans correct errors, those corrections immediately feed back into the model's training data. This makes them much more adaptable and potentially capable of avoiding similar errors in the future.
While some of these new approaches are fairly clever, there is a nagging question as to whether AI is genuinely understanding the context or just learning to statistically predict a correct response in a particular scenario. While these developments show potential in bridging language barriers, the underlying mechanism of "understanding" in these AI models still feels a bit mysterious.
Cultural context is another area that benefits from this improved contextual awareness. AI systems can now better recognize culturally-specific language variations and slang, leading to translations that are more authentic and engaging for local audiences. It's a subtle difference, but one that can significantly impact the reception of a translated text.
Another notable improvement is the ability for these systems to flag potential errors during the translation process. This real-time feedback can be useful for catching mistakes before they appear in the final document, reducing the need for extensive post-editing. It can be argued whether the "errors" are truly errors since a human language, such as English, is full of ambiguity and the definition of "error" itself can be quite tricky to define.
OCR, a method of extracting text from images, is increasingly integrated with these new AI translation models. This integration is incredibly useful for quickly translating scanned documents or handwritten notes. In an environment with increasing quantities of digital information, this kind of integrated system removes a bottleneck in translation workflow and may lead to improved efficiency across many different fields.
The enormous expansion of translation memory (TM) databases has created exciting possibilities but also presents significant challenges. The sheer scale of these databases, growing from gigabytes to terabytes, necessitates new approaches to data management. Efficient retrieval systems are needed to prevent the TM from becoming a slow, unwieldy beast. The systems must quickly find the relevant segments of translated text without imposing unacceptable performance penalties on the translation process.
We're also witnessing a disparity in the performance of different language pairs. Language combinations with high demand, like English-Spanish, see much faster database expansion compared to those with less frequent usage, such as Estonian-Mongolian. This raises questions of how to ensure that translation capabilities are maintained and improved across the entire spectrum of languages. Given the amount of data needed to perform well, this could be an area that sees a decrease in AI capability as opposed to an increase. It simply may be impractical to build out the needed TM databases for many language pairs.
Finally, these increasingly expansive TM databases raise substantial data security concerns, especially for organizations dealing with sensitive business or proprietary information. As TM databases become larger and more valuable, they naturally become attractive targets for hackers and cybercriminals. Safeguarding this information is a crucial aspect of deploying these systems, particularly in fields like finance or medicine where privacy is paramount.
These developments in AI translation showcase the potential of machine learning to enhance human communication. While it seems promising, the ongoing challenges related to handling context, scale, and security should remain forefront in future research and development efforts.
AI-Powered PDF Translation now with improved handling of scanned contents, handwriting, charts, diagrams, tables and drawings. Fast, Cheap, and Accurate! (Get started for free)
More Posts from aitranslations.io: