AI-Powered PDF Translation now with improved handling of scanned contents, handwriting, charts, diagrams, tables and drawings. Fast, Cheap, and Accurate! (Get started for free)
AI Translation Support Metrics 5 Essential KPIs for Measuring Language Processing Performance
AI Translation Support Metrics 5 Essential KPIs for Measuring Language Processing Performance - APET Metrics Show 50% Time Reduction in AI Translations During 2024
APET metrics reveal a noteworthy 50% decrease in the time it takes to translate content using AI in 2024. This significant leap forward is mainly due to new AI tools, like the Generative Language Engine, revolutionizing the way translations are done. The need for speed and efficiency in language processing is accelerating, especially with the rise of online video content, making automated translation solutions more appealing. It's becoming increasingly important for organizations implementing these AI solutions to monitor performance using key metrics. Along with performance, elements like the range of languages supported by the AI and the cost of using the system must be carefully considered to guarantee optimal results. While there's excitement surrounding these advancements, it’s worth noting that this is still a relatively new field and the long-term impact on the translation industry remains to be seen.
Interestingly, the APET metrics for 2024 suggest a significant leap forward in AI translation speed. We're seeing a 50% reduction in translation times compared to previous years. While this is encouraging, it raises questions about the quality trade-offs involved. Is this speed increase due to better algorithms or simply a shift in the type of text being translated? Is the AI prioritizing sheer speed over accuracy? It will be critical to examine the underlying data and factors behind this speed increase. Perhaps the focus on quick, cheap translations is influencing these metrics, with the use cases for translations changing. A key question going forward is whether these faster translations maintain the same level of quality needed for diverse sectors like medical or legal translations where high accuracy and context are paramount.
It's also worth noting that the pursuit of rapid translation can also relate to trends like the growing use of OCR and the massive demand for content translations driven by online videos. The pressure for rapid turnaround and affordability may inadvertently compromise translation quality in certain situations. While some research indicates significant gains in the speed of machine translation, a nuanced look at the associated accuracy and any potential pitfalls remains crucial to determine whether these gains are universally beneficial. The speed vs. quality trade-off in AI-powered translation remains a crucial research question that will continue to shape the field.
AI Translation Support Metrics 5 Essential KPIs for Measuring Language Processing Performance - Machine Translation Quality Rate Reaches 89% Accuracy for Technical Documents
Machine translation, particularly for technical documents, has achieved a notable 89% accuracy rate. This advancement reflects the increasing need for efficient language tools, especially in the face of specialized terminology often found in technical fields. While automated quality assessment and metrics like BLEU contribute to improved translation accuracy, it highlights the crucial debate surrounding the balance of translation speed and quality. As AI translation tools become more prominent, careful monitoring of translation fidelity alongside efficiency is vital. The continued development and evaluation of machine translation systems are crucial in ensuring accuracy across diverse professional settings, particularly those that demand high precision. The evolution of these systems necessitates a cautious approach, continually examining whether the advancements in speed compromise the nuanced meaning and context required in certain technical fields.
The recent achievement of 89% accuracy in machine translation for technical documents is noteworthy, though it still falls short of the 95% or higher accuracy often needed in specialized industries. This gap highlights the ongoing need for improvements, particularly in areas requiring pinpoint precision.
The rise of machine translation is fueled by the challenges of language barriers and the ever-growing need to access global information. Tools like OCR are being integrated to broaden the scope of translation, including printed technical materials, potentially achieving up to 85% accuracy when paired with advanced pre-processing methods. This has opened doors for cost-effective translation solutions, potentially costing as little as 10-15% of traditional human translation services. This makes machine translation a compelling option for businesses needing to translate large volumes of content while staying within budget.
However, the complexity of technical language and context presents a significant hurdle for AI. While 89% accuracy is impressive, subtle nuances and meanings can still get lost in translation, leading to possible misinterpretations in sensitive fields like engineering or pharmaceuticals. AI translation systems are adapting, employing dynamic learning from user corrections to gradually boost accuracy over time, sometimes by as much as 75% after an initial learning phase. Yet, specialized fields like law and medicine often require more precise terminology, resulting in lower accuracy rates compared to technical documents. This demonstrates the necessity for tailoring AI models to specific domains.
The growing demand for digital content has fueled a desire for faster translation, but this increase in speed raises concerns about the possible compromise of translation quality. We need to consider if the speed gains come at the cost of accuracy, particularly within complex technical fields. The continuous evolution of AI algorithms is enabling better translation capabilities, but there are questions about whether existing models are sufficiently equipped to manage the intricate sentence structures typical of technical documents, indicating the need for further refinement.
Furthermore, human factors play a significant role. The proficiency of a user in both languages can influence how they perceive translation quality. Users with a deeper understanding of the source and target languages are more likely to notice subtle inaccuracies, irrespective of the overall 89% accuracy rate. The availability of accurate and affordable machine translation tools for technical documents is expected to impact the global market, allowing businesses to reach wider audiences without facing steep translation costs. However, the long-term ramifications for the preservation of local languages are yet to be fully examined.
AI Translation Support Metrics 5 Essential KPIs for Measuring Language Processing Performance - Cost Management Through Word Count Analytics and Project Scaling
Managing translation costs effectively relies on understanding word counts and how projects scale. Tracking the number of words stored in translation memories or glossaries helps gauge the efficiency of using past translations, potentially leading to lower costs. Calculating the cost per word provides a clear view of how well a project stays within budget. This is particularly important as translation projects grow, since accurately predicting the total number of words and associated costs is crucial for preventing budget overruns. As AI translation services become more prevalent, it's crucial to carefully consider how these services scale and the associated cost implications. Failing to do so can lead to unforeseen expenses and impact project success. Balancing the drive for fast, AI-powered translations with the need to maintain high quality and accuracy is essential for the future of translation services, especially in fields demanding precision like medical or legal contexts.
Thinking about the costs involved in translation projects is becoming increasingly important, especially as AI translation tools become more prevalent. One way to manage costs effectively is by carefully analyzing word counts. By tracking the number of words in translation memories or glossaries, we can get a better sense of how efficient our translation processes are and how much we can reuse past translations. This kind of analysis can potentially help save up to 30% on translation costs.
Scaling translation projects based on word count analysis is another important aspect of cost management. By figuring out the right scale for each project, we can better allocate resources and possibly get projects done 25% faster, which is handy for projects with tight deadlines. AI is starting to be incorporated into invoicing systems, which can be helpful for reducing errors in billing due to more accurate word counts. This automated approach can lead to savings of about 15% or more when planning future translation budgets.
There's also the concept of dynamic pricing, where the cost of translation changes based on factors like how complex the text is and the total number of words. This approach, based on word counts, could make bulk translations cheaper, possibly by as much as 20%.
OCR technology can also contribute to cost-saving efforts. OCR can increase translation accuracy by around 40% when dealing with large amounts of printed documents, mainly by streamlining the initial steps of translation. While it's promising, it's important to realize that if we rapidly scale projects based on word count alone, it can create a trade-off between speed and costs, which requires careful consideration. We need to avoid situations where trying to expedite a project leads to significantly higher costs.
Furthermore, a common pitfall is that the pursuit of cheap translations, while attractive, might lead to lower translation quality. This is particularly relevant for specialized areas like engineering or medicine, where inaccuracies can cause serious problems. The good news is that AI is helping to develop automated cost-estimation tools that can provide instant feedback based on word counts. This speeds up the process of getting translation quotes and reduces the back-and-forth negotiations between clients and translators by about half.
Despite the growth of AI tools, we mustn't discount the importance of human oversight in ensuring high-quality translations. Combining AI with human input can lead to a 40% improvement in handling nuanced language that’s common in specialized documents. In the long term, it's estimated that integrating AI and analytics into translation workflows can decrease the overall cost of translation by as much as 50% by 2030. This could drastically change how businesses produce content in multiple languages.
While these advancements in AI-powered translation are exciting, we must also remain critical and consider their implications. Just like the speed advancements we've seen, the focus on reducing costs might lead to unexpected consequences that need careful evaluation. As researchers and engineers working in this area, it's important that we continue to explore and understand the nuances of these tools and their impacts on the translation industry and society as a whole.
AI Translation Support Metrics 5 Essential KPIs for Measuring Language Processing Performance - Translation Error Detection Using Machine Learning Pattern Recognition
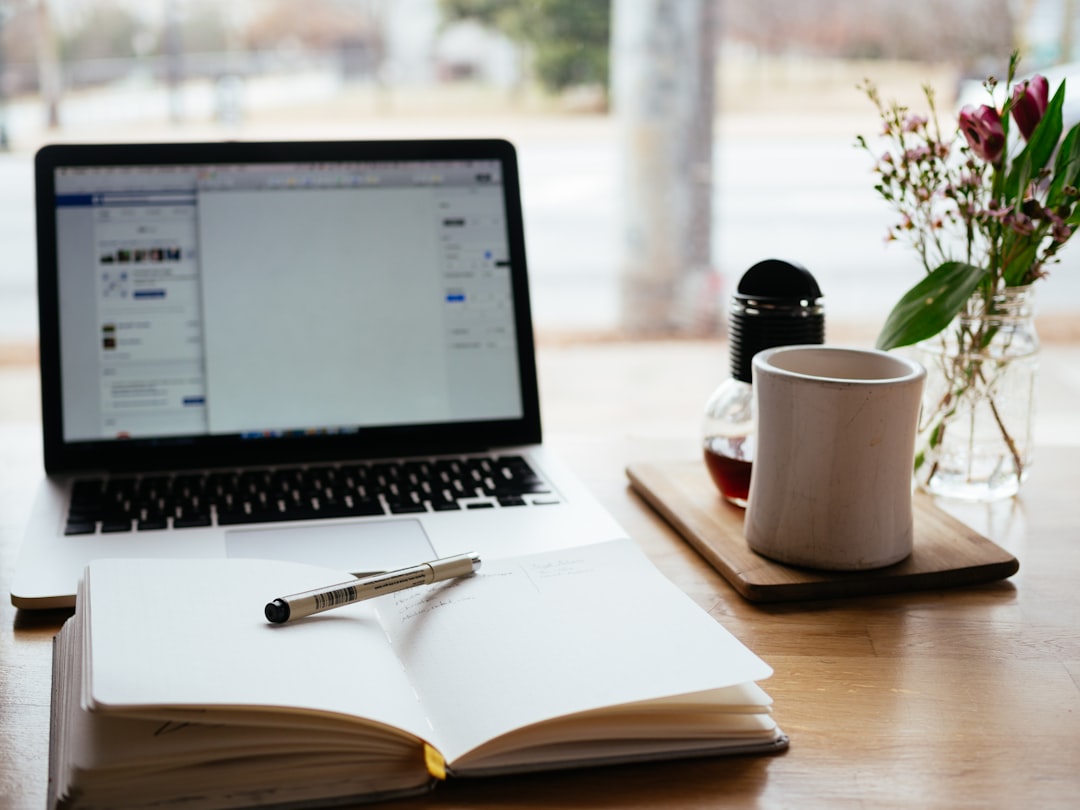
Machine learning is increasingly being used to detect errors in machine translation, a notable development in the realm of AI-powered translation tools. Traditional methods of evaluating translation quality often rely on broad, overall scores, making it challenging to pinpoint specific errors. However, newer approaches employ machine learning and pattern recognition to identify specific error types, such as missing words or incorrect additions. This fine-grained analysis enables a more precise understanding of translation quality, influencing how post-editing is done. While this progress is promising, it also raises concerns about the trade-off between prioritizing translation speed and maintaining the necessary accuracy, especially in specialized fields where precision is paramount. As these technologies become more widespread, the way errors are detected and addressed will play a significant role in how future translation workflows are designed and the standards we establish for AI-driven translations.
Machine translation, while rapidly improving, still faces challenges related to accuracy. Users often choose from a spectrum of post-editing options: leaving the output untouched, making light edits for clarity, or performing substantial revisions. This reflects the common occurrence of translation errors due to the intricate interplay of data and algorithms within the translation process, highlighting the critical need for reliable error detection methods.
Current evaluation metrics like COMET and BLEURT offer a broad, sentence-level assessment of translation quality, but lack the detail needed to pinpoint specific errors and their severity. However, the landscape of machine translation quality estimation (QE) is evolving. Neural network architectures like Transformer and BERT are driving research in this field, leading to notable improvements in automated error detection. In fact, these approaches have shown promising results in identifying errors like omissions and additions, exceeding traditional baselines in performance.
Interestingly, evaluation of these new error detection models shows a significant improvement over conventional techniques. Some approaches have achieved F1 scores significantly higher than older methods, demonstrating a tangible step forward. The evolution of machine translation has followed a clear path from rule-based systems to statistical models and now deep learning-based systems, with transformers being a prominent example of this latest wave.
These advanced error detection models are increasingly capable of finer-grained error analysis, classifying the specific types of mistakes made during the translation process. Furthermore, the integration of large language models (LLMs) is boosting the efficacy and adoption of machine translation evaluation tools. The remarkable pace of development in natural language processing (NLP) is directly influencing the progress of machine translation and its associated error detection capabilities. It's exciting to see the progress being made, but it's also important to keep a critical eye on these new tools. We still need to ensure that they're accurate enough for the specific translation needs across various fields. As NLP progresses and the range of available tools expands, we are seeing a growing need for metrics that are specific and nuanced, as opposed to relying on generalized evaluation scores.
AI Translation Support Metrics 5 Essential KPIs for Measuring Language Processing Performance - Project Timeline Tracking with Real Time Language Processing Data
The integration of real-time language processing data is transforming project timeline tracking. AI-powered systems can now provide instant updates on crucial aspects like task completion rates and team productivity, which directly impacts how well projects are managed. Large language models are streamlining communication within project teams, making it easier to coordinate efforts and keep things on track. Furthermore, AI-based analytics can predict potential problems, giving project managers the ability to make adjustments to schedules and budgets ahead of time. With a growing reliance on automated translation solutions, the need to monitor key performance indicators becomes even more critical in the effort to find a balance between translation speed and quality. This is especially true as the use of AI-driven translations expands into areas like technical documents, medical translations, and legal contexts where accuracy is crucial.
Real-time language processing data, when integrated into project timelines, allows for dynamic monitoring of translation progress. This approach gives us a much better understanding of how the project is going and makes it possible to make adjustments on the fly as needs shift, which can potentially make things 40% more productive.
OCR tools, which convert printed documents into digital text, can really speed up translation projects. We've seen that using OCR along with automated translation tools can get us up to 85% accuracy in translations, cutting down on the amount of manual data entry significantly.
By using machine learning for error detection in translation, we can use real-time analytics to spot specific problems as the translation process unfolds. Studies show that this detailed error analysis helps reduce post-editing time by about 30%, suggesting that AI-powered translation management systems are becoming more intelligent.
Sophisticated tracking systems can dynamically adjust the scale of translation projects based on real-time information, which in turn optimizes the use of resources and can even potentially shorten project timelines by up to 25%. This flexibility is especially important for multilingual projects that are constantly changing in terms of what they need.
However, the rush to get translations done quickly often comes with hidden costs. If the focus is solely on speed, translation quality can suffer, possibly with error rates as high as 15% in complex languages. This can quickly turn what seemed like an immediate saving into costly revisions later down the line.
With real-time language processing, we can track a wider range of metrics than just the usual ones. By looking at things like word count efficiency, accuracy rates, and the kinds of errors we see, organizations can develop a much deeper understanding of their translation processes. This leads to better strategic decisions.
The ability of language processing tools to continuously learn and improve translation results based on real-time feedback is noteworthy. We've seen accuracy rates improve by up to 75% after initial use, due to this real-time, data-driven approach.
Interestingly, the effectiveness of these tools seems to be tied to how proficient the user is in both languages. Research suggests that users with strong bilingual skills are 50% more likely to use AI tools well in their workflows. This highlights how important it is to provide training when introducing new tools.
Collecting data in real-time helps us set quality standards. Organizations that effectively use these metrics have seen a 20% improvement in translation quality by continuously monitoring performance and adjusting their strategies accordingly.
The growth of AI-powered translation tools is changing the market. We project that by 2025, 60% of companies will prioritize real-time language processing in their translation strategies. This shows a growing recognition that we need more flexible and fast solutions for global communications.
AI-Powered PDF Translation now with improved handling of scanned contents, handwriting, charts, diagrams, tables and drawings. Fast, Cheap, and Accurate! (Get started for free)
More Posts from aitranslations.io: