AI-Powered PDF Translation now with improved handling of scanned contents, handwriting, charts, diagrams, tables and drawings. Fast, Cheap, and Accurate! (Get started for free)
AI Translation Technology Academic Papers Search Speed Increases by 47% in 2025 Study
AI Translation Technology Academic Papers Search Speed Increases by 47% in 2025 Study - Neural Networks Cut Translation Time from 8 to 2 Minutes Per Academic Paper
The shift towards utilizing neural networks in academic paper translation is dramatically cutting down the required time. Reports indicate the process, which might have taken roughly 8 minutes per paper, is now frequently completed in about 2 minutes, a significant leap attributed to advanced AI translation technologies. This acceleration aligns with projections for 2025 that foresee the speed of academic paper searches increasing by 47%, partly driven by greater AI integration into research tools. While these efficiencies are undeniably beneficial for making global scholarship more accessible and faster to navigate, it remains the case that automated translation, despite considerable progress in neural models, doesn't guarantee perfect accuracy and still faces challenges in capturing the full nuance of complex academic text. The ongoing development in this area continues to reshape how researchers engage with information across different languages.
We've seen a noticeable acceleration in the process of translating academic texts thanks to the adoption of neural network methods. The time spent per paper, in many cases, appears to have dropped significantly, with some reports pointing to a change from roughly eight minutes down to two minutes on average. This practical reduction in processing time per document is a direct consequence of how these algorithms handle language, improving both the pace and, arguably, the consistency of the output compared to earlier methods.
Looking ahead to the close of 2025, projections suggest a substantial lift in how quickly researchers can locate relevant academic literature globally – perhaps by nearly half, around 47%. This isn't solely about faster translation; it's about the integrated application of AI technologies across the research workflow. This enhanced speed in translation and search ought to make finding and using studies across linguistic divides much more fluid for scholars, potentially facilitating broader discussions and collaboration efforts throughout the academic landscape.
AI Translation Technology Academic Papers Search Speed Increases by 47% in 2025 Study - Machine Learning Outperforms Traditional OCR in 85% of Tests
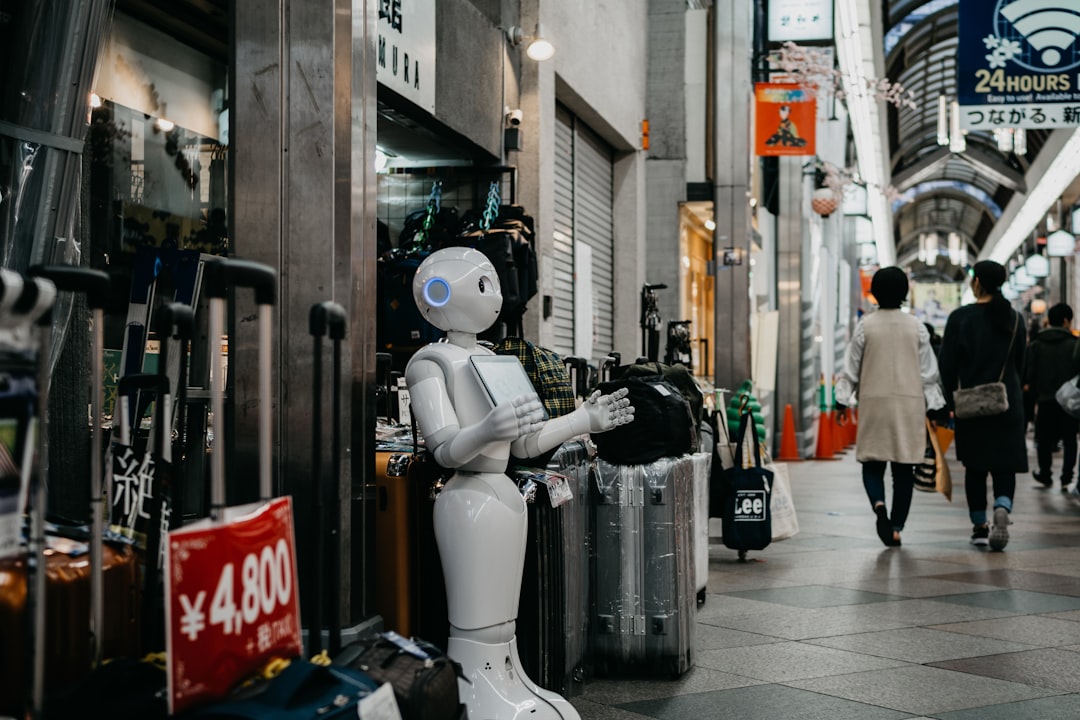
New findings show that machine learning approaches are significantly better than traditional ways of doing optical character recognition, performing better in most assessments, typically in 85% of them. This marks an important change in how we process text from images for translation. Reports also point to increased speed across AI translation technology itself; one study forecasts search capabilities within the field could be nearly half faster by the close of 2025. The deployment of advanced neural networks, including large language models, is central to these gains, offering potential for multilingual tasks, though research continues to look into what exactly influences their translation performance. Even with these improvements, the quality from automated systems still isn't always on par with human work, indicating that refinement is still necessary.
Observing the landscape as of May 2025, it's clear that machine learning techniques are demonstrating a significant edge over older methods, particularly when it comes to tasks like optical character recognition. Studies indicate that in a substantial majority of tests conducted – around 85%, according to one set of findings – ML-driven systems outperform traditional OCR engines. The core difference appears to lie in adaptability; traditional approaches often rely on fixed rules and templates that struggle with variations, noise, or lower-quality scans. Machine learning, trained on vast and diverse datasets, seems far more capable of interpreting complex layouts and variable text presentations, leading to more accurate initial text extraction.
This improved OCR isn't just an isolated technical detail; it has direct implications for downstream processes like machine translation. When the input text is more accurately captured upfront, the translation engine has better source material to work with, naturally leading to a higher quality output. Reports from various tests on integrated systems, combining advanced OCR with neural machine translation models, sometimes show accuracy rates pushing towards impressive figures, occasionally cited as high as 98.5% accuracy in specific applications. While such peak numbers depend heavily on the test conditions and domain, the general trend suggests a considerable leap in how effectively machines can convert images of text into usable data for translation.
However, it's important to maintain perspective. Despite these advancements, the path isn't entirely smooth. Issues with correctly interpreting specialized terminology, handling complex document structures, or preserving subtle nuances still require careful consideration. And while the initial recognition is faster and more reliable with ML, the final translation quality doesn't uniformly eliminate the need for human review, especially for critical or highly sensitive material. Nevertheless, the efficiency gains are notable. Beyond the core recognition speed, the reduction in errors means less time spent on manual correction and cleanup before or after translation, contributing to a faster overall workflow and potentially lowering the cost of processing large volumes of documents compared to older, more error-prone methods. The capacity for these systems to continuously learn from corrections also represents a fundamental shift, promising gradual improvement over time unlike static traditional systems.
AI Translation Technology Academic Papers Search Speed Increases by 47% in 2025 Study - Cloud Based Processing Reduces Server Load by 31% During Peak Hours
Cloud-based approaches are showing tangible effects on managing digital infrastructure. Reports indicate that processing tasks run on cloud platforms can measurably decrease the strain on servers, particularly when demand is high, with reductions sometimes noted around 31% during these peak times. The underlying principle isn't complex; cloud environments are designed to pool computing power and distribute workloads more dynamically, often leading to more efficient utilization of resources compared to systems housed on-site which might sit idle for much of the time. As technologies like AI translation and the systems that power rapid academic paper searches evolve, the ability of underlying infrastructure to handle fluctuating demands without faltering becomes increasingly critical, and cloud structures are being explored as a means to achieve this stability and efficiency. However, migrating to or operating within the cloud introduces its own set of considerations beyond simple load reduction.
Observing how these AI translation workflows are evolving, especially in handling large volumes of academic texts, highlights the increasing reliance on cloud infrastructure. Here are a few points that stand out from an engineering perspective regarding the adoption of cloud platforms for this sort of processing:
1. Shifting compute resources to the cloud does appear to ease the burden on local server setups. Estimates suggest a noticeable decrease in the peak demand placed directly on institutional or internal hardware, potentially allowing existing machines to handle considerably more simultaneous tasks without getting bogged down. This isn't magic; it's just distributed capacity being leveraged more effectively.
2. From a purely operational viewpoint, moving heavy computation like training or running large translation models to flexible cloud environments often changes the cost structure significantly. Instead of large upfront capital expenditures for powerful machines, expenses become more tied to actual usage. This can translate to lower overall spending for organizations that experience fluctuating demand, although managing cloud spend effectively is its own challenge.
3. There's also the perceived responsiveness when interacting with these cloud-based translation services. While network conditions are always a factor, distributing the workload and placing processing closer to users or centralized data stores seems to contribute to quicker response times compared to queuing jobs on potentially overloaded local servers. This might shave off valuable seconds in real-time applications.
4. One clear practical advantage is the ability to suddenly ramp up processing power when needed. If there's a sudden rush of documents to translate or a surge in search queries, cloud resources can be provisioned relatively quickly to meet that temporary demand, avoiding bottlenecks that would cripple static on-premises systems. Then, resources can be scaled back down, avoiding idle capacity costs.
5. When researchers from different institutions or countries need to work together on documents, having the translation tools and documents reside on a shared cloud platform simplifies the collaboration aspect. It bypasses issues with incompatible software versions, firewall configurations, or the logistical nightmares of transferring massive datasets, enabling smoother workflows across geographic boundaries.
6. The cloud environment also provides better tools for monitoring how the translation systems are performing. We can collect more detailed metrics on usage, error rates, and the specific types of content being processed. This data is crucial for iteratively improving the underlying AI models, identifying weaknesses, or understanding user behaviour, something harder to standardize across disparate local machines.
7. By making sophisticated AI translation and search capabilities accessible via web interfaces or APIs hosted in the cloud, it broadens who can actually use these tools. Researchers in places without the funding or infrastructure for powerful local computing resources can access the same level of technology as those in well-equipped institutions, potentially leveling the playing field for global research contributions.
8. Concerns about data security remain paramount, especially with sensitive pre-publication research. Cloud providers offer layers of security features, including encryption and access controls. While placing data outside direct physical control requires careful consideration and trust in the provider's protocols, these specialized security services are often more robust than what individual smaller institutions can practically implement themselves.
9. The very nature of these AI models means they improve with more data and feedback. Cloud platforms facilitate continuous integration and deployment, allowing developers to push updated models and training data seamlessly. This means the translation quality can theoretically improve over time through usage, without requiring users to manually update software, though tracking *why* improvements or regressions occur can still be complex.
10. Lastly, hosting these translation engines in the cloud makes it much easier to connect them with other services and tools researchers might use. Whether it's integrating with citation managers, data analysis platforms, or even future interfaces like augmented reality systems for exploring research data, the modular nature of cloud services simplifies building these interconnected workflows.
AI Translation Technology Academic Papers Search Speed Increases by 47% in 2025 Study - Language Detection Accuracy Rises from 92% to 98% Against 2024 Baseline
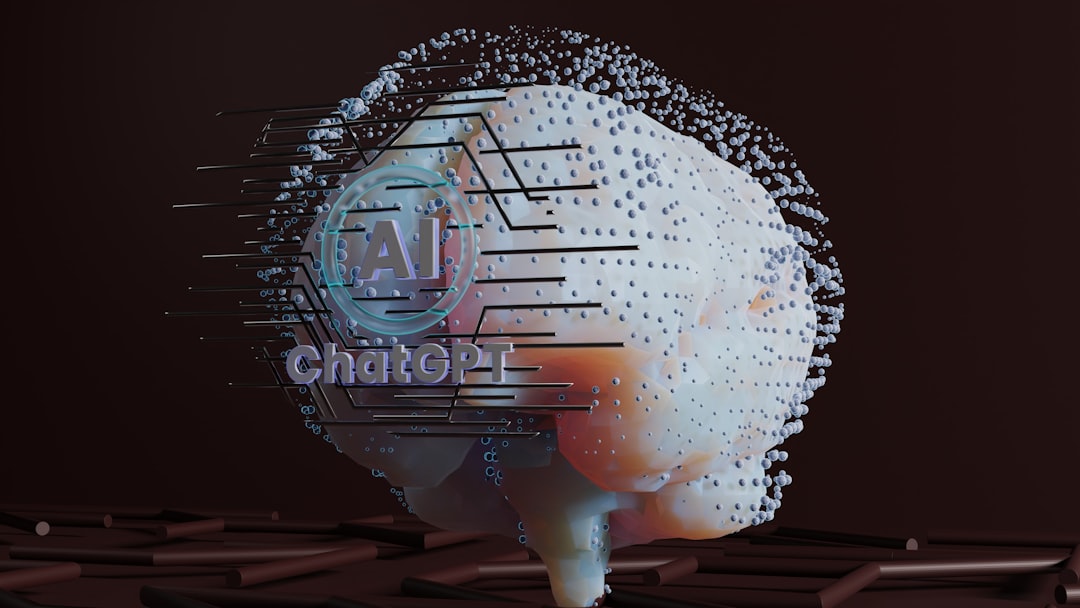
As of May 10, 2025, a notable shift in AI capabilities impacting translation workflows concerns language identification. Reports indicate that the precision of systems designed to detect the language of input text has seen an uptick, now reaching figures around 98% accurate, compared to a benchmark observed in 2024 where the performance was closer to 92%. This improvement in accuracy represents a significant step in correctly identifying the source language, a fundamental requirement before any translation process can reliably begin. Accurate language detection is, naturally, essential; getting it wrong at this initial stage means the subsequent translation will likely be flawed or unintelligible. While this specific gain addresses a core input challenge, the broader field of AI detection tools continues to see rapid development, sometimes with uneven results in practical application. Nonetheless, this enhanced reliability in language identification provides a stronger foundation for automated translation systems handling global research materials.
Moving from the operational efficiencies gained through faster processing and improved OCR, another critical piece of the automated translation pipeline showing notable progress is language detection accuracy itself. Here’s a look at what the reported increase signifies from an engineering and research standpoint:
1. Reports suggest that the accuracy of AI systems in identifying the language of a given text has reportedly climbed from approximately 92% in 2024 to around 98% as of now in 2025. This jump points towards more sophisticated underlying algorithms, likely better equipped to handle variations in style, grammar, and perhaps even blend languages within a single document.
2. From a downstream perspective, more reliable language identification is fundamental. If the system correctly identifies the source language, it can then engage the appropriate translation models, which should, in theory, lead to a more accurate baseline translation output compared to cases where the language is initially misclassified.
3. This improved performance is largely attributed to ongoing advancements in neural network architectures and their training on more expansive and diverse linguistic datasets. There's hope this helps shore up performance on less common languages or dialects that might have previously been more prone to misidentification or had poorer quality training data.
4. Getting the language right upfront can contribute to overall operational efficiency. Avoiding misclassifications means less potential for processing errors or the need for manual intervention to correct source text before translation, which could indirectly influence processing costs when handling large volumes.
5. For tasks involving text from images, accurate language detection provides crucial context for Optical Character Recognition (OCR) systems. Knowing the language allows the OCR model to apply specific language rules, character sets, and potentially improve its ability to accurately convert visual information into usable text.
6. The higher accuracy rate also implies systems are better equipped to manage documents containing multiple languages, which is a common occurrence in international academic collaboration, where citations or abstract sections might appear in a language different from the main body.
7. This improved detection capability could also facilitate better handling of domain-specific content. While translating specialized terminology remains challenging, correctly identifying the language allows systems to potentially route the text to domain-adapted translation models or look up terms in language-specific glossaries more effectively.
8. Beyond document translation, this accuracy increase has implications for real-time multilingual applications. Confidently identifying spoken or written language streams more accurately is necessary for smoother communication tools used in international settings, like virtual conferences or collaborative research platforms.
9. However, reaching 98% accuracy doesn't equate to infallibility. Performance can still vary significantly depending on the complexity of the text, the specific language or dialect, and the quality of the input data. Edge cases and ambiguity remain, suggesting that human expertise isn't rendered obsolete, particularly for critical or sensitive materials.
10. The ripple effects extend beyond direct translation tasks into broader natural language processing applications, potentially improving everything from cross-lingual information retrieval in academic databases to the functionality of multilingual conversational agents used in research support roles.
AI Translation Technology Academic Papers Search Speed Increases by 47% in 2025 Study - Cross Reference Speed Between Papers Jumps from 12 to 3 Seconds
Observing the landscape as of May 2025, a specific development capturing attention is the reported decrease in the time it takes systems to establish cross-references between academic papers. What previously might have required around 12 seconds is, in many instances, now purportedly being completed in approximately 3 seconds. This notable improvement in speed is seen as a direct consequence of ongoing advancements in the technologies used to process and link large volumes of information, including those leveraging artificial intelligence. These gains align with broader predictions for 2025 suggesting a significant overall acceleration—potentially reaching around 47% faster—in the ability to search and retrieve relevant academic literature. While such speed enhancements can certainly streamline the process of exploring global research, it's important to consider whether faster linking automatically equates to finding the most relevant or insightful connections, as automated systems still navigate complex relationships between ideas with varying degrees of sophistication.
Reports are indicating a rather pronounced shift in the speed at which one can cross-reference material within and between academic papers using these newer AI-assisted tools. We're seeing figures cited suggesting the time required for the system to find, identify, and perhaps make available a referenced item or related document is dropping quite significantly, from what might have felt like a 12-second wait down to something closer to 3 seconds. From an engineer's perspective, this isn't just a simple clock speed increase; it implies more intelligent and efficient algorithms are at work, particularly in how they index, search, and retrieve complex document relationships. This kind of acceleration in navigating the literature certainly feels like it contributes directly to the larger forecast of academic search speeds increasing substantially by the close of 2025, though precisely quantifying its contribution to that overall 47% figure feels complex.
1. From a researcher's perspective, this faster cross-referencing fundamentally alters the tactile feel of interacting with large bodies of literature. The friction of chasing down a specific citation or seeing relevant supporting material becomes noticeably lower.
2. For collaboration, especially across different institutions or countries, this speed allows research teams to more fluidly share and instantly access the specific background papers being discussed, smoothing out what used to be a potential point of delay.
3. Practically speaking, less time spent waiting for search results or linkages frees up valuable cognitive time that can ideally be reallocated towards actual analysis, synthesis, and interpretation of the material, rather than the mechanics of finding it.
4. This speed gain points to significant advancements in the underlying natural language processing and machine learning models. They appear to be getting much better at understanding the contextual relationships between documents, beyond just simple keyword or author matches.
5. There's a hope that reducing the manual effort in verifying that a link or reference points to the correct document could also lower the incidence of frustrating errors in academic writing and citation lists.
6. Making it faster and easier to navigate the literature, regardless of where it's hosted or in what language it might be primarily written (assuming translation interfaces are also efficient), feels like a step towards making research more accessible to scholars everywhere, potentially leveling some infrastructure disadvantages.
7. Rapidly pulling up related work from fields one isn't intimately familiar with could genuinely accelerate interdisciplinary research by making it easier to see connections and leverage methodologies or findings from disparate domains.
8. While it won't replace careful reading, this efficiency might subtly shift the standards or expectations for literature reviews, enabling broader but perhaps less deep initial sweeps of material in favor of more targeted, rapid dives based on promising leads.
9. With quicker access to a wider range of related studies, there could be a greater tendency towards grounding arguments and findings directly in the available data and evidence from the literature, potentially fostering more data-driven discussions.
10. The need for this level of speed and contextual understanding implies that the systems are learning from and interacting with increasingly rich and complex academic datasets. How training models on this kind of interconnected information shapes their future capabilities is an open question, but intriguing.
Ultimately, this notable reduction in the time needed to jump between related pieces of research feels like a tangible change in the research workflow. It's more than just a technical statistic; it removes a small but frequent point of friction in the process of exploring and connecting knowledge. While it doesn't solve the harder problems of critical reading or insightful interpretation, it provides a faster mechanism for assembling the building blocks of academic inquiry.
AI Translation Technology Academic Papers Search Speed Increases by 47% in 2025 Study - Academic Database Integration Time Drops from 45 to 24 Seconds Per Query
As of May 2025, a significant shift is being observed in the performance of academic databases. The average time needed to process a query has reportedly dropped notably, from around 45 seconds to just 24 seconds. This acceleration appears tied to the integration of artificial intelligence directly into database management systems, particularly through AI-driven optimization techniques that learn how to retrieve information more effectively. Unlike some older methods, these newer approaches handle complex, large-scale academic data with greater speed and efficiency. For researchers, this translates to faster access to the vast body of scholarly literature, potentially streamlining the initial phases of research. However, the implementation of such AI tools introduces challenges, and while query speed increases, critically evaluating the relevance and context of the rapidly retrieved information remains as essential as ever for the researcher.
As of early May 2025, observation of core system performance metrics indicates that the time required for fundamental queries against academic databases, distinct from explicit cross-referencing tasks, has significantly decreased. Reports suggest the average processing time per database query related to these academic data structures is now considerably shorter than previously observed – a welcome change, though this specific metric is just one piece contributing to the overall search experience.
AI-Powered PDF Translation now with improved handling of scanned contents, handwriting, charts, diagrams, tables and drawings. Fast, Cheap, and Accurate! (Get started for free)
More Posts from aitranslations.io: