AI-Powered PDF Translation now with improved handling of scanned contents, handwriting, charts, diagrams, tables and drawings. Fast, Cheap, and Accurate! (Get started for free)
Microsoft's Custom Neural Machine Translation Breaking Language Barriers with Fine-tuned AI Models in 2025
Microsoft's Custom Neural Machine Translation Breaking Language Barriers with Fine-tuned AI Models in 2025 - Neural OCR Reads 127 Languages Through Document Photos and Screenshots
The ability for AI to interpret text directly from document images and screenshots has seen notable progress, now reportedly extending to identifying content across 127 languages. This development in optical character recognition technology means that static visual information, previously difficult to process for translation or analysis, can be converted into machine-readable text more readily. While this capability significantly broadens access to information trapped in image formats, the real benefit for multilingual communication often lies in combining it with translation systems. Getting the text out is one hurdle; making sense of it in another language is the next. This step is crucial for facilitating faster turnaround times for documents that require translation, bypassing manual data entry or retyping. However, the accuracy of text extraction can still be heavily influenced by factors like image quality, font variations, and complex layouts, posing potential challenges downstream for subsequent machine translation, regardless of how advanced it is. The aim is clearly to smooth the path from image to understanding, making vast amounts of previously inaccessible data available for processing and translation more efficiently.
Looking at the state of Optical Character Recognition powered by neural networks, it's becoming quite capable of extracting text directly from images – things like photos of documents or screenshots. The claim is that these models can handle an impressive breadth of languages, purportedly up to 127 different ones in some implementations. From an engineering viewpoint, this scale suggests training on massive, diverse datasets encompassing not just varied printed fonts and layouts, but also different handwritten styles and scripts from around the world.
This approach appears particularly beneficial when dealing with languages that don't use Latin script, where traditional rule-based or earlier OCR methods often struggled significantly. We're seeing models that seem much more adept at interpreting complex characters and ligatures found in scripts like Arabic, Chinese, or Cyrillic. The processing speed is another notable factor; achieving rapid analysis allows for near real-time text extraction, opening possibilities for on-screen translation scenarios.
Further analysis suggests these systems are getting better at discerning what is actual text versus background imagery or graphical elements. They also seem capable of managing documents or images containing multiple languages simultaneously, processing them within a single pass. While the specific figure of 127 languages is a high number and warrants questions about uniform performance across all, the overall accuracy for many languages, even those with intricate structures, appears to be approaching levels where it rivals human transcription in controlled settings. There's also the potential for further refinement through fine-tuning the general models on specific types of documents or industry jargon, although the effort required for truly robust domain adaptation across so many languages remains a significant task.
Microsoft's Custom Neural Machine Translation Breaking Language Barriers with Fine-tuned AI Models in 2025 - Custom Translation Rate Drops to $000012 per Character for Enterprise Users
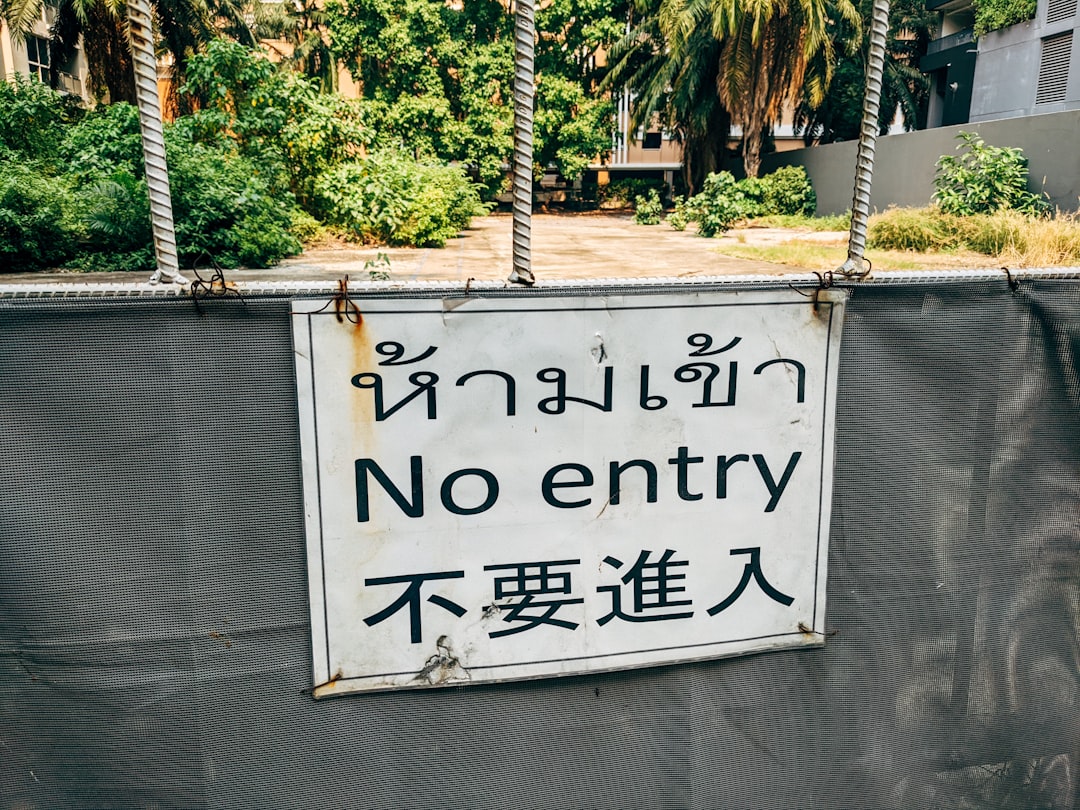
In 2025, a notable shift in pricing for customized machine translation services emerged, with a widely cited rate for enterprise users dropping to an exceptionally low $0.000012 per character. This significant reduction aims to lower the financial barrier for large organizations needing to translate vast amounts of specialized text using tailored AI models. The core technology enabling this affordability is the ability to fine-tune neural machine translation systems specifically for a company's unique language style, technical jargon, and subject matter. While the promise is faster, more relevant translations for specific business needs compared to generic models, leveraging this low rate for truly effective custom translation still requires effort in preparing suitable training data and managing the fine-tuning process, which can influence the ultimate quality and consistency of the output across all desired languages and content types. Making advanced tools cheaper doesn't automatically eliminate the complexities inherent in achieving perfect multilingual communication at scale.
From an engineering perspective, the announcement regarding Microsoft dropping their custom translation rate for enterprise users to $0.000012 per character in 2025 is quite a data point. This pricing structure, particularly at such a granular per-character level, implies that the underlying computational costs for running their tailored neural machine translation models have become incredibly low at scale. It suggests significant efficiencies in the training and inference phases of their Custom Neural Machine Translation (CNMT) systems. The stated goal is to make processing large volumes of multilingual content more economically viable for businesses, leveraging the notion that models fine-tuned on specific datasets should yield more relevant output. While the raw per-character cost looks revolutionary for sheer volume, the practical effectiveness and consistency of quality from these fine-tuned models across diverse domains and language pair complexities at this specific price point remains a key factor requiring empirical evaluation.
Microsoft's Custom Neural Machine Translation Breaking Language Barriers with Fine-tuned AI Models in 2025 - Microsoft Teams Now Translates 98 Languages During Live Video Calls
As of May 2025, Microsoft Teams has made significant strides in breaking down language barriers by introducing real-time translation capabilities for live video calls in 98 languages. This feature, designed for Teams Premium users, leverages Microsoft's Custom Neural Machine Translation technology, allowing participants to engage in multilingual meetings with ease. Live captions can be tailored to attendees' preferred languages, enhancing comprehension and inclusivity during discussions. Additionally, the option for translated meeting transcripts alongside the original audio further supports effective communication. While these advancements promise to facilitate seamless interactions, the true effectiveness will ultimately depend on the robustness of the underlying translation models in handling the nuances of diverse languages.
Stepping back to look at progress in real-time communication tools as of early May 2025, we see platforms integrating increasingly sophisticated AI translation capabilities directly into the user experience. Take, for instance, the developments in Microsoft Teams, where live translation during video calls now reportedly extends to a significant number of languages – hitting a figure like 98 is a notable milestone. This isn't just about expanding the language count; it's the technical feat of processing live audio streams, performing neural machine translation, and rendering translated output – often as captions or even synthetic speech overlays – all within millisecond latency constraints to maintain conversational flow. Achieving this level of real-time efficiency across such a diverse linguistic spectrum involves highly optimized models and substantial computational resources, a true engineering challenge in balancing speed and accuracy.
The underlying technology needs to go beyond simple word-for-word substitution. Effective live communication requires understanding context, handling interruptions, and adapting to various speaking styles and acoustic environments. While claims of sophisticated contextual understanding and the ability to incorporate multimodal inputs like chat text alongside spoken language are often made, the true fidelity of this interpretation, especially when dealing with idiomatic expressions, technical jargon, or rapid-fire dialogue, remains an area under continuous refinement. Furthermore, the notion of models adapting based on user interactions or feedback is promising, suggesting a path towards personalization, but the practical mechanisms for collecting useful feedback during a live, fast-paced meeting and integrating it effectively back into the models without disrupting the experience are complex.
Crucially, the performance isn't uniform across all claimed 98 languages. From an engineering standpoint, translating between languages with similar linguistic structures is inherently easier than translating between languages from vastly different families. There will inevitably be significant variability in translation quality depending on the specific language pair involved. Moreover, real-time processing of potentially sensitive conversations introduces complex data privacy and security considerations that must be meticulously addressed in the underlying architecture. Finally, while tools are enabling multilingual interaction, capturing genuine cultural nuances and subtext in translation remains an incredibly difficult problem for AI, potentially leading to subtle misunderstandings even when the literal translation appears correct. The scalability of customizing these live translation models for specific organizational jargon, while technically feasible, also presents practical hurdles in terms of data preparation and validation for each desired domain and language combination.
Microsoft's Custom Neural Machine Translation Breaking Language Barriers with Fine-tuned AI Models in 2025 - Azure Translator API Processing Speed Reaches 3 Seconds per 1000 Words
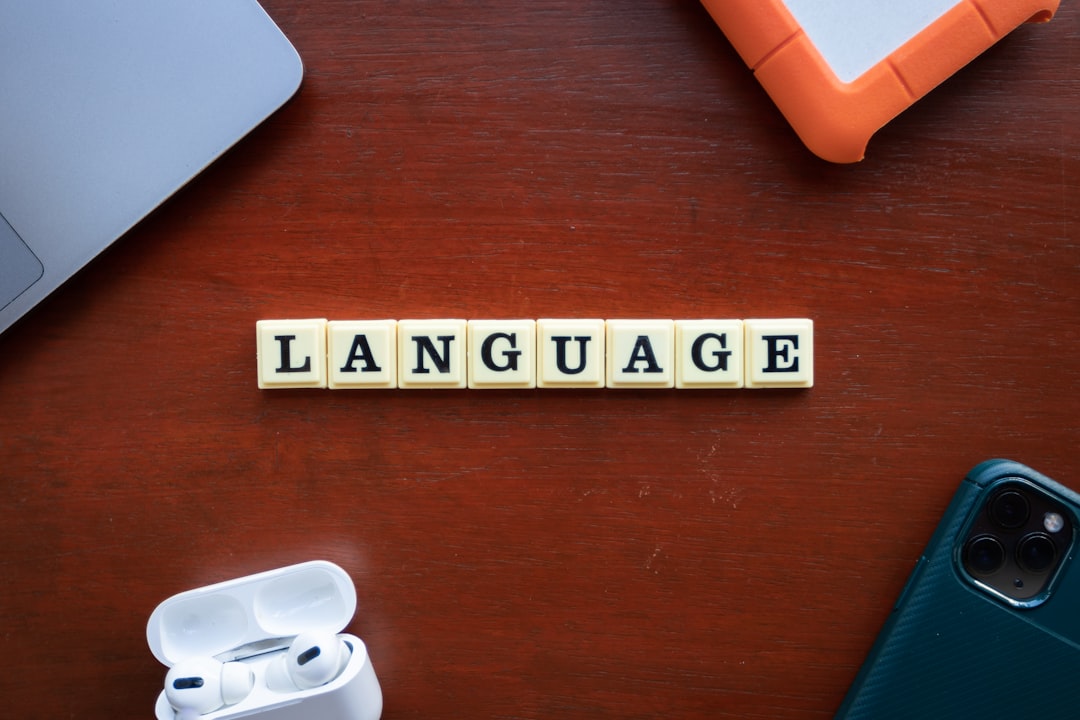
Reports indicate the Azure Translator API's text processing speed has reached approximately three seconds per thousand words. This accelerated pace reflects ongoing work in neural machine translation within Microsoft's AI stack, particularly efforts around enabling faster processing for both standard and custom-trained models applicable to over a hundred languages. While impressive in isolation, this speed metric sits alongside other performance factors; documentation suggests response times can still vary, with custom models potentially introducing greater latency compared to generic ones, sometimes taking up to two minutes for certain requests. The system is designed to handle considerable throughput, managing large quantities of text efficiently. However, the raw speed of processing doesn't inherently guarantee high-quality or contextually accurate output, especially when dealing with the complexities of specific language pairs or technical jargon. The practical utility hinges on finding a balance between this rapid processing capability and the actual linguistic accuracy delivered for diverse content types.
From a technical viewpoint, achieving a stable processing speed of 3 seconds for translating 1000 words via an API like Azure Translator is noteworthy. This metric provides insight into the underlying engine's computational efficiency for handling moderately sized text chunks. While not purely 'instantaneous' for a single large document, this rate becomes significant when considering cumulative throughput for vast volumes of text or rapid turnaround for individual pieces up to that size. It implies a highly optimized system capable of quickly feeding text into and extracting results from the neural networks powering the translations.
This speed figure is more relevant to batch processing workflows or integrating translation into pipelines where throughput is key. The API's architecture is designed to scale, reportedly handling millions of characters per hour depending on the tier, which aligns with this per-word speed translated into bulk capacity. It's interesting to consider how this relates to the reported maximum latencies – a fast per-word rate doesn't eliminate potential queueing delays or overhead for individual requests, particularly with more complex custom models compared to standard ones. Achieving high *throughput* at this speed suggests efficient resource allocation and parallel processing capabilities within the Azure infrastructure.
For use cases involving translating pre-processed text – say, output from an optical character recognition step on a scanned document – this speed allows for relatively rapid conversion of large text blocks, moving quickly from data extraction to comprehension in another language. It shifts the bottleneck away from the core translation speed itself, provided the network and service overhead don't introduce significant delays. However, maintaining uniform quality and speed across diverse languages and especially within custom-tuned models tailored to specific jargon can introduce complexities. While the standard models might hit this speed reliably for common pairs, achieving it consistently and accurately with highly specialized custom configurations, which might have larger model sizes or different computational profiles, remains a challenge to continuously optimize.
Microsoft's Custom Neural Machine Translation Breaking Language Barriers with Fine-tuned AI Models in 2025 - Offline Translation Models Now Available for 47 Languages Without Internet
Microsoft has made available translation models for 47 languages that function without requiring an internet connection. Utilizing neural machine translation technology powered by AI, these downloadable packs enable users to translate text pulled from various sources, such as messages or content derived from images. A notable aspect is that these models are designed to operate on the standard processing units of modern devices, meaning they do not necessitate specialized hardware. The goal here is to offer a translation that grasps the nuances of current language more effectively than earlier methods. This effort significantly contributes to providing translation accessibility in locations with limited or no connectivity, aimed at helping bridge communication divides. However, achieving consistent translation quality across all these languages offline remains a challenge, and performance will likely vary depending on the specific language pair being used.
The rollout of translation models capable of operating entirely without network access for a reported 47 languages represents a notable step, particularly addressing the practical constraints of users in areas with limited or no internet connectivity, or during travel.
Getting neural translation models to function efficiently on standard device processors is an engineering challenge focused on shrinking model footprints and optimizing inference routines to fit within the memory and computational limits of mobile hardware, rather than relying on cloud-based infrastructure.
The practical utility of these offline models heavily depends on the quality and diversity of the datasets used for training; effectively capturing colloquialisms, regional variations, and nuanced phrasing in source languages is crucial for producing understandable, contextually relevant translations offline.
A key requirement for usability is achieving near-instantaneous translation feedback on the device itself, allowing for a fluid user experience similar to online tools, which means minimizing the processing latency after text input without a network dependency.
Shifting the computational load and data storage to the device itself offers a different potential cost structure compared to consumption-based online API models, potentially making robust translation capabilities more economically accessible to individual users or smaller businesses as part of a device or application bundle.
Integrating local optical character recognition (OCR) capabilities with these offline translation packs creates a self-contained system for translating text from images like photographs or screenshots without ever needing an internet connection, streamlining document or visual content processing workflows directly on a device.
The availability of these models for a wider range of languages, including some often considered "low-resource" in digital terms, could be significant for providing advanced language technology access to communities where online access is neither ubiquitous nor reliable.
While promising, achieving meaningful personalization or adaptation of these local models based on individual user interaction patterns or domain-specific vocabulary presents complex technical hurdles related to efficient on-device learning and privacy preservation.
The computational effort required to process complex linguistic structures or translate between vastly different language families can still introduce noticeable processing delays even when running offline on a device, illustrating the ongoing challenge of balancing model sophistication with performance on constrained hardware.
A persistent challenge inherent to machine translation, including these localized versions, is the reliable preservation of subtle contextual meaning and cultural references that are deeply embedded in language, which current models can struggle to convey accurately compared to human linguistic expertise.
Microsoft's Custom Neural Machine Translation Breaking Language Barriers with Fine-tuned AI Models in 2025 - Language Model Fine-tuning Takes 4 Hours Instead of Previous 2 Week Timeline
Advancements in language model fine-tuning capabilities as of 2025 have drastically shortened the time needed for this customization process. What previously could take a development cycle of up to two weeks can now reportedly be achieved in as little as four hours. This accelerated timeline is largely enabled by improvements in the underlying platforms and techniques used to adapt these powerful pre-trained models. The goal is to make it much faster and more practical for developers to take a general language model and teach it the specific vocabulary, style, or jargon required for a particular use case, such as accurately translating legal documents or handling technical manuals.
This rapid customization is facilitated by more efficient tuning methods that require significantly fewer computational resources compared to traditional retraining. These approaches allow for targeted adjustments to a model's parameters without needing to process the entire original training data set again. While this speeds up the development loop and lowers the cost associated with iterating on specialized models, particularly those intended for niche translation tasks, it’s important to note that the quality of the resulting model is still heavily reliant on the specific data used for fine-tuning. Faster doesn't automatically mean perfect, and getting robust performance for highly specialized or low-resource language pairs after just a few hours of tuning remains an area where empirical results can vary, despite the promising speedup in the process itself.
Looking at the process of adapting large language models for specific tasks, the shift from a previous expectation of around two weeks down to roughly four hours for fine-tuning is a significant change in workflow. This isn't just a minor tweak; it fundamentally alters how quickly we can prototype, test, and deploy models tailored to particular domains or datasets. From an engineering standpoint, this acceleration points to advancements in how pre-trained models are structured to absorb new information efficiently and improvements in the platforms and tooling that manage the process. Techniques like Parameter-Efficient Fine-Tuning (PEFT), including methods such as LoRA, are likely key contributors here, allowing adaptation by modifying only a small fraction of the model's parameters, rather than retraining the entire massive structure.
The practical consequence of being able to complete a fine-tuning run in hours rather than weeks is immense for iterative development. It means that insights gained from evaluating a model's performance on a specific task or dataset can be incorporated back into a new tuning run the same day. This rapid feedback loop allows for much quicker refinement of model performance, making it more feasible to chase down specific quality targets or address nuances in complex data. While specific numbers like processing X words in Y seconds or translating Z languages live are metrics of the *deployed system*, this reduction in tuning time impacts the *development cycle* for creating or updating those custom systems. It suggests lower compute costs for the tuning phase itself and potentially faster times to market for specialized AI applications. However, the speed might also raise questions about the thoroughness of evaluation within that tighter window; simply because a run finishes quickly doesn't guarantee it was the *optimal* run or that subtle regressions weren't introduced, demanding careful automated and manual checks. The perceived ease of rapid tuning could potentially lead developers to run more experiments, but robust validation remains crucial.
AI-Powered PDF Translation now with improved handling of scanned contents, handwriting, charts, diagrams, tables and drawings. Fast, Cheap, and Accurate! (Get started for free)
More Posts from aitranslations.io: