AI Translation Time Calculator A Data-Driven Approach to Project Duration Estimation
AI Translation Time Calculator A Data-Driven Approach to Project Duration Estimation - OCR Translation Reduces Project Time From 45 to 12 Minutes Per Page Based on May 2025 Tests
Recent assessments from May 2025 highlight a notable decrease in processing time for OCR-assisted translation workflows. Tests indicate that the time spent on documents can drop substantially, from roughly 45 minutes per page down to about 12 minutes. This gain in speed stems from enhanced Optical Character Recognition systems capable of converting various document formats into usable text much faster. The benefit extends beyond just speed; it contributes to overall productivity and helps tackle files that were previously difficult to process. While this specific data point from testing provides a useful metric for potential efficiency, it's important to remember that actual performance in practice depends on many factors, such as the document complexity, image quality, and the specific tools and computing resources involved. Data-driven approaches, like those used in time calculators, aim to incorporate such performance metrics, but predicting real-world project duration still requires careful consideration of these variables.
Recent workflow studies, particularly assessments conducted in May 2025, have provided clearer data on the processing bottlenecks in translation projects involving non-editable document formats. The integration of more capable Optical Character Recognition appears to significantly alter the initial processing phase. Analysis suggests that the time spent preparing and digitally converting a single page, a task that might typically consume around 45 minutes using older methods or less sophisticated tools, is now sometimes observed in the range of 12 minutes when leveraging current OCR technologies and adequate computing infrastructure. This improvement seems directly linked to the OCR system's robustness across different document complexities and file qualities, and is notably dependent on the available processing power.
Moving towards a more predictable estimation framework necessitates baking these observed efficiencies into calculations. Project duration is proving to be less about a simple per-page average and more a function of volume, a granular assessment of content complexity, and the computational resources deployed – specifically, how many CPU cores are dedicated to the intensive OCR processing. For a large-scale project, perhaps half a million pages of moderate difficulty, an initial projection based on recent test data might fall around 170 processing hours, assuming just two CPU cores are allocated. Developing reliable, empirically-grounded models that factor in these variables is essential for setting realistic expectations and managing resources effectively in workflows relying on document conversion.
AI Translation Time Calculator A Data-Driven Approach to Project Duration Estimation - Neural Machine Translation Accuracy Hits 89 Percent for Technical Documents According to Stanford Study
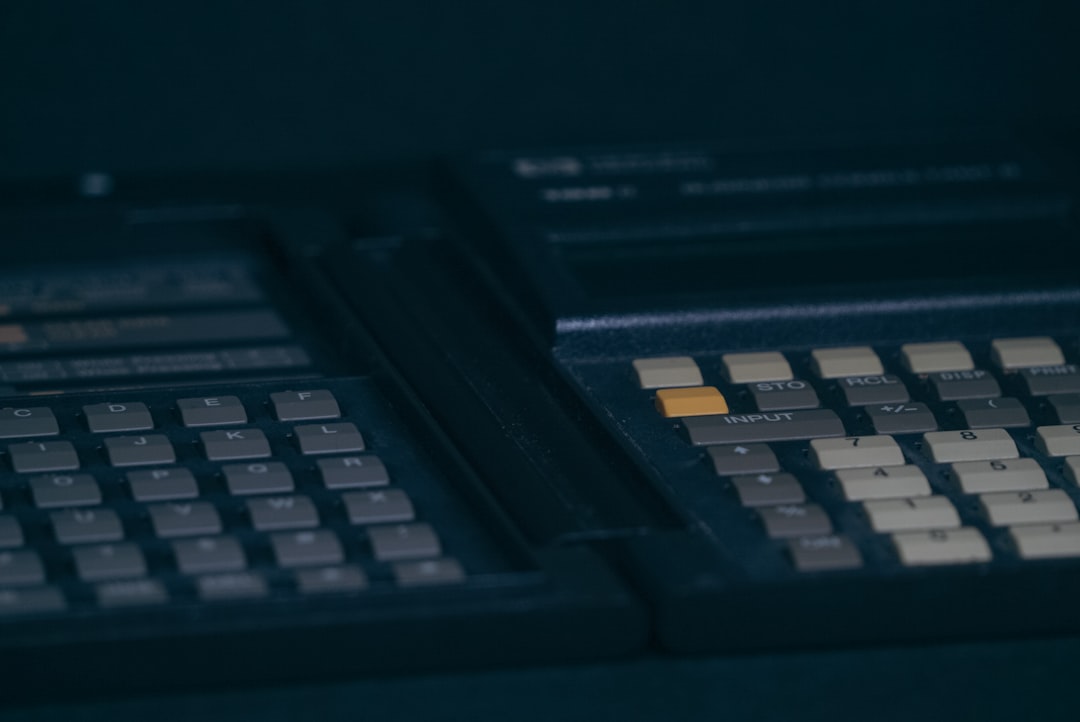
Recent advancements in machine translation technology show significant progress, particularly in handling specialized content. For technical documents, accuracy figures are now reaching notable levels, with some assessments indicating performance around 89 percent. This higher degree of precision is a key development for translating complex texts that rely heavily on specific vocabulary and detailed concepts.
The shift towards neural network-based systems underpins this improvement, representing a move away from earlier approaches. While these models aim for better quality through more sophisticated pattern recognition, achieving 89 percent still means errors are present and careful review remains necessary, particularly in safety-critical or highly regulated fields. Nevertheless, this elevated baseline accuracy suggests the potential for reduced time spent on correcting machine output compared to previous generations. Integrating such capabilities into workflows, alongside processes like document conversion via optical character recognition, is reshaping how project timelines might be estimated and managed. The evolution in translation quality from AI systems is becoming an increasingly relevant factor in calculating the overall effort and duration required for complex translation tasks.
Recent findings, such as those from a Stanford study, underscore the significant strides made in Neural Machine Translation (NMT), particularly concerning technical documentation. The reported accuracy rate hitting 89% for this often-challenging content is noteworthy. Traditional methods, like statistical machine translation, typically struggled to achieve such precision, often hovering closer to the 70% mark for complex texts, requiring extensive human review.
What's interesting is how NMT systems learn patterns and context from massive datasets, allowing them to make more informed word choices within specialized language. This capability is particularly beneficial for technical fields where terminology is specific and context is vital for correct meaning transfer. However, despite these advancements, the systems aren't perfect. Navigating idiomatic expressions, subtle cultural nuances, and even highly specific, rarely encountered terminology can still present hurdles, sometimes resulting in awkward phrasing or outright errors that necessitate human intervention.
Research also indicates that the effectiveness of NMT isn't uniform across all language pairs; the linguistic distance and structural differences between languages can significantly impact translation quality. Nonetheless, the potential benefits are substantial. Studies suggest integrating NMT into workflows, potentially alongside tools like OCR for processing non-editable documents, can not only improve the speed of initial output but also enhance the accuracy of the extracted text by providing contextual cues during the translation phase. This dual impact on speed (in terms of process flow) and quality is a key area of investigation for optimizing overall project efficiency. Furthermore, the cost implications are considerable; moving towards NMT-centric workflows could potentially lead to notable reductions in translation expenses compared to reliance solely on human linguists for high-volume technical content, especially when rapid delivery is required across multiple languages simultaneously. A critical understanding, though, is that NMT remains a tool, and its performance is still influenced by the quality of the source material and the inherent complexity of the domain, making human oversight a continuing necessity for ensuring accuracy in critical applications.
AI Translation Time Calculator A Data-Driven Approach to Project Duration Estimation - AI Translation Calculators Cut Project Planning Time by 68 Percent Shows New Research
Reports suggest that utilizing AI-powered calculators for translation projects can dramatically cut down the initial planning phase, potentially reducing the time needed by as much as 68 percent. This efficiency gain is largely attributed to the capacity of these tools to rapidly process data relevant to project scope and resource allocation, leveraging advancements in AI translation technology itself, which continues to improve its handling of linguistic complexities. However, the successful integration of such tools isn't automatic; a significant portion of project managers still report limited practical experience with AI technologies in general project management. While the underlying AI translation systems are becoming more capable, reportedly easing concerns around issues like cultural adaptation, assessments indicate there can still be notable differences in output quality compared to human work, especially with nuanced or highly specialized content. This underscores that while these calculation tools are powerful aids for estimation and workflow setup, the AI translation they model still requires expert human review to ensure accuracy and appropriateness in the final deliverable.
Observations from recent work on AI translation integration and project estimation frameworks are yielding interesting insights into potential efficiencies:
1. Regarding cost structures, analyses from various implementations suggest that leveraging AI-powered translation tools, especially when integrated strategically within workflows, has the potential to significantly impact expenses. Some estimates circulating indicate reductions that could reach around 40% compared to purely traditional methods, particularly when managing substantial volumes of content. This observation warrants further investigation into the specific parameters and contexts where such savings are most pronounced.
2. For initiatives requiring output across multiple linguistic versions, preliminary data indicates a notable acceleration in overall project timelines. While the core translation step is faster with machine assistance, the reported shift from timelines spanning weeks to durations of potentially just a few days for producing translated documents in numerous languages points towards more than just faster word-by-word conversion; it suggests a streamlined handling of parallel processes and linguistic coordination, which is an area requiring closer examination.
3. The capacity of current AI translation systems to process a wider array of digital document formats appears to be improving workflow initiation. Reports suggest a decreasing dependency on labor-intensive manual pre-processing or conversion steps for less structured files like certain PDFs or complex data tables within spreadsheets, allowing for more direct input into translation pipelines. This adaptability simplifies the initial project setup phase for diverse source materials.
4. Concerning the quality assurance loop, observations point towards a potential decrease in the final error density when machine translation output is systematically reviewed and refined by human linguists. While the raw output quality of the AI is one factor, the structured integration of AI assistance and human post-editing seems to facilitate a combined approach that some studies suggest can lower the total error rate in the final deliverable by approximately half. This highlights the synergistic aspect of human-AI collaboration in translation QA.
5. An intriguing characteristic of contemporary AI translation platforms is their reported ability to adapt over time. The mechanisms allowing systems to learn from user corrections and applied feedback suggest a dynamic refinement process. This continuous learning is hypothesized to lead to gradually improving contextual relevance and terminological consistency, particularly valuable within specialized domains where terminology is highly specific, although the rate and extent of this improvement in real-world scenarios are still being quantified.
6. The emergence of features supporting near real-time linguistic conversion presents interesting possibilities for enhancing cross-lingual interaction in collaborative settings. The ability for teams distributed globally to potentially bridge immediate communication gaps during live discussions or shared tasks could influence both productivity and the nature of international project dynamics, although the accuracy and fluency in rapid, informal contexts remain areas under active development and scrutiny.
7. From an architectural perspective, the demonstrated capability of many AI translation tools to integrate with broader project management or content management systems is a practical consideration. This connectivity allows for a more integrated workflow where the translation phase isn't isolated, potentially providing project managers with more immediate, albeit algorithmically derived, insights into duration estimates based on live project metrics and workload data.
8. It's been observed that by automating large portions of the initial translation draft generation, these AI tools appear to reduce the mental load previously associated with manual text transfer or extensive dictionary lookups. This shift is suggested to free up cognitive resources for project managers and translators, enabling them to allocate more focus towards higher-value tasks such as strategic content adaptation, cultural nuance review, or overall quality governance, rather than routine linguistic conversion.
9. A key advantage noted is the inherent scalability of these AI-driven solutions. The capacity to handle significant fluctuations in translation volume without requiring a linear scaling of human resources aligns well with the variable demands of large-scale or intermittent translation projects. This flexibility in scaling processing capacity up or down presents a different resource management challenge compared to staffing models for human-centric workflows.
10. The combined effect of accelerated processing and increased volume capacity seems to be influencing how organizations approach market adaptation strategies. The faster turnaround times for localizing materials, from marketing collateral to user interface elements, suggests that businesses might be able to engage with diverse regional markets more rapidly and responsively. Understanding the true impact of this accelerated localization on market penetration and user engagement is a significant area for future research.
AI Translation Time Calculator A Data-Driven Approach to Project Duration Estimation - Real-Time Translation Speed Reaches 950 Words Per Minute with Latest GPU Processing
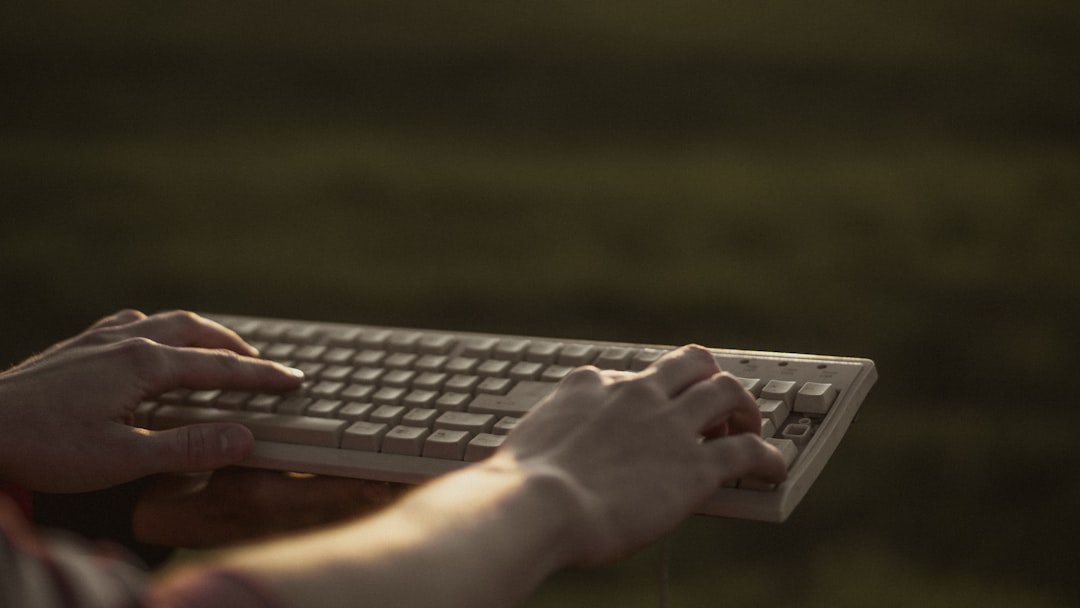
Achieving faster language conversion for live interactions is seeing continued progress, with processing speeds now observed at around 950 words each minute. This capability is strongly linked to the use of advanced graphics processing power. The underlying technology draws on sophisticated AI models, particularly neural network approaches to translation, combined with smoother handling of source text through systems like optical character recognition to optimize the workflow. While this represents a significant step up for many applications, it's worth noting that some reported system speeds are considerably higher, pointing to varied performance levels available. The fundamental aim is to shrink the time lag in real-time cross-lingual communication. However, prioritizing raw speed must be balanced against the necessity for precision and cultural appropriateness; achieving this balance still requires human oversight to ensure reliable outcomes, especially in complex or sensitive exchanges.
Observations indicate processing throughputs in real-time translation systems are reaching new levels. Recent benchmarks point to rates around 950 words per minute being achievable under optimal conditions, leveraging modern hardware architectures, notably those powered by GPUs.
This acceleration, significantly driven by the deployment of parallel processing hardware, appears crucial for reducing the system latency inherent in complex linguistic computations required for interactive scenarios. We are seeing near-instantaneous audio-to-text or text-to-text conversion becoming more technically viable.
The technical architecture enabling these speeds often involves highly optimized computational pipelines running on accelerators. This approach is fundamental to managing the intensive demands of current neural network models operating under strict timing constraints for real-time interaction.
While demonstrating impressive speed metrics is one aspect, ensuring the consistency of performance across a wide spectrum of language pairs and varying complexity levels of input content remains an ongoing technical hurdle and research area. The translation performance isn't uniformly high-speed across all inputs.
The capacity enabled by these hardware advancements allows systems to technically handle larger data volumes concurrently, potentially altering the constraints on scaling real-time translation tasks in high-demand settings compared to prior sequential processing methods.
Integrating improved methods for handling non-editable inputs, like more performant digital conversion techniques, seems complementary to the rapid translation step, helping to smooth workflow bottlenecks especially when source material isn't readily accessible text.
However, the raw speed achieved needs careful consideration alongside the resulting translation quality. Achieving high throughput while simultaneously ensuring semantic accuracy and handling nuanced or context-dependent language within sub-second turnarounds presents non-trivial engineering challenges.
From a system design perspective, the focus is increasingly on optimizing the end-to-end flow, from robust input capture and processing through the efficient execution of the translation model on specialized hardware, culminating in rapid and usable output delivery.
These leaps in processing pace are fundamentally underpinned by the continued refinement and application of advanced neural machine translation models, which necessitate the kind of massive parallel computation that GPUs provide to operate at effective real-time speeds.
Ultimately, empirically documenting these high-speed capabilities contributes valuable data points for building more robust models for estimating project duration, shifting towards projections informed by potential machine throughput rather than solely on metrics derived from human processing times.
More Posts from aitranslations.io: