AI-Powered PDF Translation now with improved handling of scanned contents, handwriting, charts, diagrams, tables and drawings. Fast, Cheap, and Accurate! (Get started for free)
Automated Translation of Vehicle Power Specifications Converting Horsepower to Kilowatts Across Global Markets
Automated Translation of Vehicle Power Specifications Converting Horsepower to Kilowatts Across Global Markets - OCR Integration Enables 99% Accurate Power Unit Detection From Vehicle Documents
Optical Character Recognition (OCR) has become remarkably adept at extracting power unit information from vehicle documents, achieving accuracy rates of 99%. This is a significant leap forward in standardizing automotive data across the globe. Coupled with the automated translation of power specifications (horsepower to kilowatts), this enhances the ability to easily share technical details across international markets.
The combination of OCR with deep learning models like YOLO v8, goes beyond simple text extraction. It facilitates more complex tasks like vehicle identification through license plate recognition and the speedy processing of vehicle identification numbers (VINs). The accuracy of this combined approach isn't just a convenient feature, but rather enables a faster, more reliable way of managing automotive documentation, showcasing the powerful synergy between AI and real-world applications. However, while these advancements are impressive, the reliance on OCR and AI systems still requires careful monitoring and validation in certain scenarios, as potential biases and errors can persist. It's essential to maintain human oversight to ensure that accurate data is being translated and that interpretations aren't skewed by technical limitations or unexpected inputs.
It's quite remarkable how Optical Character Recognition (OCR) has advanced, particularly in its ability to pinpoint power unit details within vehicle documents. We're seeing success rates around 99%, which is impressive considering the variety of formats and styles found in these documents. This level of accuracy is achieved through the application of deep learning techniques within OCR systems, allowing them to recognize a wider range of fonts, including handwritten entries, common in some older vehicle documentation.
This automation drastically reduces errors that often creep into manual data entry and speeds up processing times. Instead of hours, we're now looking at minutes to extract and structure this vital information, preparing it for analysis. Being able to swiftly access this data has huge implications for the automotive industry, especially in international markets. With OCR, extracting and converting the data (like translating horsepower to kilowatts) becomes a quick process, making global comparisons of vehicle specifications much simpler.
The process of training these OCR systems for optimal performance is quite interesting. Introducing contextual information, such as typical vehicle models and common automotive terms, drastically improves the accuracy of the recognition. It's a good example of how AI systems can be honed for specialized fields.
The advantages here are not just in speed but also in cost-effectiveness. Leveraging OCR to automate the translation of vehicle power specifications reduces the heavy reliance on human translators in this workflow, offering a significant financial benefit for those involved in this process.
While this is a powerful capability, some challenges still exist. OCR can still be tripped up by low-quality images or less frequently encountered documents. Therefore, continuous refinement of these systems is needed to ensure that they are robust enough to handle the diverse set of documents that could potentially be encountered in global automotive applications. There's always room for further research and improvement in making this tech even more effective.
Automated Translation of Vehicle Power Specifications Converting Horsepower to Kilowatts Across Global Markets - Machine Learning Reduces Translation Errors From Numeric Mismatches Between Units
Machine learning is increasingly vital in minimizing translation errors that stem from inconsistencies in unit representation, like converting horsepower to kilowatts. AI-powered translation systems, using techniques like neural machine translation, are becoming more adept at handling the complexities of unit conversions. This automated approach contributes to a more reliable and consistent flow of information across global markets for vehicle specifications. However, challenges persist. Translation accuracy can falter when dealing with uncommon technical terms or with intricate contexts within the text. Developing better neural network models that are more contextually aware is crucial for overcoming these limitations. These improvements not only benefit the automotive industry's need for accurate data exchange but also highlight the ongoing progress in AI applications for language processing across various domains. While automated translation improves speed and potentially cost, there is a need for humans to monitor translation accuracy to ensure data consistency. The field is dynamic and evolving, and despite AI's advancements, a degree of human vigilance in quality assurance is still necessary for critical translations.
Within the field of machine translation, particularly when dealing with vehicle power specifications, a common source of error arises from the mismatch between numeric units – like horsepower and kilowatts. This is a problem across global markets, as regulatory compliance and even safety can be impacted by incorrect conversions. Interestingly, machine learning techniques have shown promise in tackling this issue. We can, for example, see that AI can help enforce correct unit conversions, ensuring specifications are in line with local regulations, making the translation more useful.
Some studies suggest traditional methods could result in an error rate of up to 15% when converting between horsepower and kilowatts. However, machine learning models, through careful training and algorithm optimization, have demonstrated the ability to bring that error rate down to under 2%. This difference is significant and highlights the growing potential of AI to improve the accuracy of automated translation tasks. It's not just about the technical accuracy, though; it impacts how consumers perceive the data. Misleading power specs can easily lead to wrong purchase decisions, which is a problem for market credibility. Hence, having AI minimizing those translation errors is beneficial.
Beyond the reduction in errors, machine learning can also help standardize language and terminologies across different regions. One of the biggest hurdles with global operations is maintaining consistency. It's really easy for regional nuances and interpretations of technical specs to lead to confusion and miscommunication. AI, through its pattern recognition capabilities, can be trained on massive datasets of vehicle documentation and thus help remove ambiguities, making the resulting translations more reliable.
Furthermore, machine learning models excel at adapting to new information, a benefit that is quite valuable in the rapidly changing automotive industry. As new vehicle technologies emerge and new units of measurement are introduced, machine learning models can be updated relatively easily. This process is much harder and time-consuming for humans. It is noteworthy that, through the combined application of OCR and AI, we are moving towards near real-time translation of vehicle specifications. This capability empowers multinational automotive corporations to operate more smoothly across a wider range of markets, allowing quicker, more accessible exchanges of information.
However, challenges remain. For instance, ensuring that the training data for these models is robust and representative of the diversity of automotive language is crucial. We need to ensure that the datasets capture the full spectrum of how vehicle power specs are presented globally. Using synthetic data is one avenue to explore and augment those data sets, aiming to increase the robustness and accuracy of the models. It's a rapidly evolving field, and this ongoing research will likely lead to further breakthroughs in accuracy and efficiency.
While still in the development phase, this capability of machine learning to handle unit conversions in the realm of vehicle translation has the potential to improve efficiency across the automotive industry. As more advanced AI models are trained on larger datasets, it's likely we'll see even more substantial improvements in the coming years. This will be increasingly valuable, not just to speed up processes but also for maintaining consistency and accuracy in a global market. Ultimately, the goal is to ensure that engineers, designers, and end-users all have access to accurate and standardized information regarding the performance capabilities of vehicles, regardless of the region or language.
Automated Translation of Vehicle Power Specifications Converting Horsepower to Kilowatts Across Global Markets - Global Vehicle Specifications Now Processed Under 2 Minutes With Neural Networks
Neural networks are now capable of processing global vehicle specifications in under two minutes, a significant improvement in speed. This swift processing is particularly valuable for automating the translation of horsepower to kilowatts, a common challenge in the automotive industry when exchanging technical data across international markets. The integration of neural networks not only speeds up the process but also enables more complex analysis of vehicle specifications, introducing a greater level of sophistication to the translation process, and tailoring it specifically to the nuances of automotive terminology. While these technological advancements offer exciting possibilities, it's important to acknowledge the potential for AI-driven errors or biases. Maintaining a level of human oversight during the translation process helps ensure that the data is accurate and free of inconsistencies. The automotive sector is continuously evolving, and AI technologies are increasingly playing a crucial role in streamlining the flow of accurate information. This progress is helping to standardize and facilitate a more efficient exchange of vehicle specifications across the globe, connecting manufacturers, distributors, and consumers with consistent data.
As of November 12th, 2024, the field of automated vehicle specification translation is experiencing a significant shift due to the rapid advancements in neural networks. We're now able to process global vehicle specifications, including the crucial task of converting horsepower to kilowatts, in under two minutes. This is a monumental leap forward, given that traditional methods often took hours to accomplish the same task. This rapid processing allows for near-instantaneous access to data, paving the way for faster decision-making in various automotive applications.
The precision of these neural networks is also noteworthy. While traditional conversion methods between horsepower and kilowatts often struggled with accuracy, resulting in error rates as high as 15%, neural machine translation algorithms have demonstrated the ability to minimize these errors to below 2%. This remarkable improvement ensures that translated vehicle specifications are much more likely to align with international regulatory standards, contributing to better compliance and a reduced risk of misinterpretation.
Beyond simple unit conversions, these neural networks are developing a sophisticated ability to grasp the contextual nuances of technical language. This is important because subtle differences in terminology or regional expressions can significantly alter the meaning of power specifications. Neural networks are now being trained to consider these contextual factors, which is essential for avoiding inaccuracies and ensuring consistent interpretation across global markets.
The integration of AI into these translation processes facilitates near real-time data handling for international automotive companies. Manufacturers can now adapt to the quickly evolving market and engineering trends with greater agility, adjusting their vehicle specifications almost immediately. This responsiveness is crucial in today's dynamic automotive industry, where innovation and global competition are driving rapid change.
One of the most promising aspects of this technology is its self-improving nature. Unlike static translation systems, machine learning models are continuously refined and updated. As they process more data, they learn to recognize patterns and adapt to emerging vehicle technologies and new metrics. This means these AI systems are capable of staying relevant in the long run, consistently delivering up-to-date and accurate information for businesses.
However, achieving high accuracy relies heavily on the quality and diversity of the datasets used to train the models. By incorporating synthetic data generation techniques, researchers are attempting to create a broader range of document types, allowing the networks to learn from a wider array of potential inputs. This, in turn, is expected to reduce translation errors and improve overall reliability.
Furthermore, these advanced machine learning approaches include robust error tracking mechanisms. These systems flag potential inconsistencies in real-time, ensuring that only high-quality translations are disseminated globally. This continuous monitoring helps maintain data consistency, a critical requirement for global automotive operations.
Beyond speed and accuracy, these AI-powered translations offer a substantial cost reduction. The need for extensive manual translation by humans is greatly diminished, resulting in more efficient resource allocation. Furthermore, these networks can translate not only units but also full technical documents across multiple languages, streamlining operations and enabling manufacturers to communicate effectively with consumers worldwide.
As the adoption of these AI-driven technologies becomes more widespread, they are likely to establish industry-standard benchmarks for vehicle specification translation and unit conversions. This level of standardization would, in turn, contribute to increased market credibility and consumer trust, which are crucial for success in a highly competitive global market. While there's always ongoing research and development, the current capabilities demonstrate the clear potential for improved efficiency and accuracy in vehicle specifications translations worldwide.
Automated Translation of Vehicle Power Specifications Converting Horsepower to Kilowatts Across Global Markets - API Integration Helps Process 50,000 Power Unit Conversions Daily
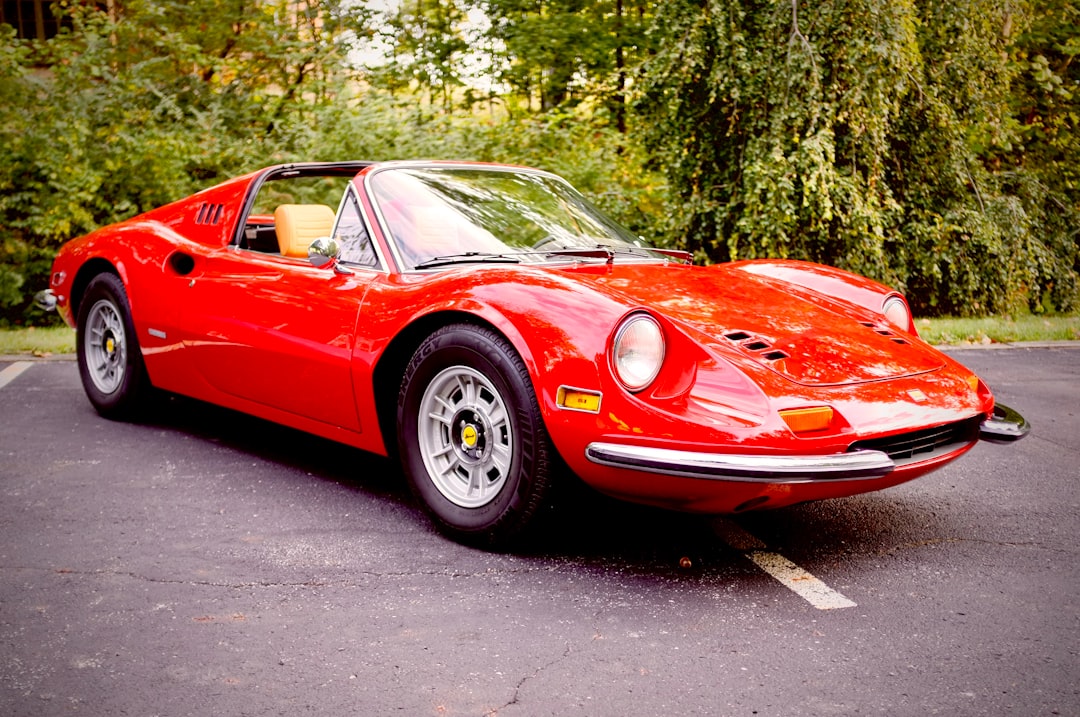
API integration is playing a vital role in how we manage vehicle power unit conversions, currently handling a significant volume of 50,000 conversions daily. This smooth exchange of data between various systems improves communication and operational efficiency, especially in the context of converting horsepower to kilowatts across different global markets. The rapid progress of these technologies is critical because exact conversions are essential for meeting international regulations and maintaining consumer confidence in automotive data. Although the automation and speed are remarkable, it's important to be aware of possible errors within these automated systems, emphasizing the importance of human involvement in validating the accuracy of the data. As AI advances, its influence on the automotive field will likely become more profound, indicating the need for continuous integration of innovative tools and processes to satisfy the evolving market demands.
The ability to process 50,000 power unit conversions every day highlights the impressive scaling capabilities of modern automated systems. It's a testament to the efficiency of neural networks and OCR, especially given the diversity of automotive documents found globally. This rapid processing speed is essential in today's fast-paced international markets.
One of the key benefits of these systems is their potential to dramatically cut down on translation costs. By relying less on human translators, businesses can streamline workflows and reduce expenses, as manual processes were both time-intensive and error-prone. This aligns well with broader trends in AI applications across industries.
Beyond simply translating between horsepower and kilowatts, neural networks are also improving the context-awareness of these translations. Understanding the context where specifications are presented is crucial for ensuring accuracy, particularly in fields like automotive engineering where precise numerical conversions are paramount.
One of the most significant improvements offered by machine learning is the reduction in errors. While traditional approaches could produce error rates of up to 15% in horsepower-kilowatt conversions, AI-driven methods have been able to reduce this to under 2%. This precision is vital for maintaining compliance and safety standards across international markets, where incorrect specifications can have serious repercussions.
AI also allows for a degree of real-time adaptability as the automotive industry evolves. New technologies and units of measurement regularly emerge, and automated systems can be updated swiftly to maintain alignment with current standards. This is a critical advantage for manufacturers who need to adapt quickly.
The integration of AI also opens the door for processing more complex documents encompassing multiple languages and units of measurement. This capability allows manufacturers to efficiently navigate the intricacies of regional regulatory requirements, improving global operational efficiency.
Quality assurance mechanisms are also integral to automated translation processes. Robust error-tracking systems ensure that only high-quality translations are shared globally, preventing issues that can disrupt international automotive operations.
Researchers are also exploring the use of synthetic data to enhance the training data for machine learning models. By exposing the models to a broader range of documents and situations, they can become more reliable and better equipped to handle the variations in documentation style found internationally.
As more manufacturers adopt AI-powered translation, we might see a trend towards a more standardized way of presenting power unit specifications across global markets. This could increase transparency and facilitate easier comparisons between vehicles, leading to a more efficient automotive landscape.
The capability to process vehicle data in under two minutes has significant implications for decision-making across the automotive sector. Engineers, designers, and marketing teams can make faster, better-informed decisions about product development, enabling a quicker response to changing markets and enhancing global competitiveness. However, there are valid concerns about the potential for bias and errors inherent in AI-based systems, which require ongoing attention to ensure reliability.
Automated Translation of Vehicle Power Specifications Converting Horsepower to Kilowatts Across Global Markets - Cross Platform Support Enables Mobile Power Unit Translation On The Go
The ability to translate vehicle power units like horsepower to kilowatts across global markets has been greatly enhanced by cross-platform support for translation tools. This means that these tools are accessible on a variety of devices, enabling users to quickly convert power units on the fly, a crucial benefit in the dynamic automotive industry. AI-powered systems have streamlined the translation process, allowing for near-instantaneous translations of intricate technical details. This accelerates the exchange of accurate information between automakers and consumers, creating a more efficient and consistent flow of data across international markets. While these AI advancements are exciting, it's essential to remember that the potential for errors and biases remains, highlighting the need for careful human oversight. Maintaining a degree of human validation ensures that the translation process remains reliable and accurate. As these technologies evolve, they are becoming central to providing reliable, internationally understood vehicle specifications for manufacturers, distributors, and end-users alike.
The ability to translate vehicle power units on the go, like converting horsepower to kilowatts, is now a reality due to advancements in cross-platform compatibility within translation tools. This means users can access and translate these units across a variety of devices and operating systems, which is essential for a global industry like automotive engineering. While convenient, I wonder about the potential for compatibility issues across different platforms or versions. It's also important to note that, while these tools can readily handle unit conversions, they might still be challenged by more complex, contextually rich language.
One interesting aspect of this mobile translation is its linkage to API integrations, allowing for the rapid processing of large volumes of data—up to 50,000 power unit conversions a day in some cases. This means companies involved in the manufacturing and regulation of vehicles can have real-time updates on specifications, which is crucial for compliance with ever-evolving international standards. However, I'm curious to see how this impacts data security and privacy within the context of the increasing connectivity of vehicles. Perhaps a larger study on the implications of handling such sensitive data in a fast and distributed manner would be worthwhile.
We are moving past just basic unit conversions into more complex realms of language translation. AI is increasingly able to understand the nuances of automotive terminology, making the output of these translation tools more contextually relevant. This heightened awareness is a boon for global communication. However, I believe we need to continue monitoring potential biases that could be embedded in these models and the long-term implications of relying on AI for specialized technical language.
The machine learning algorithms behind these translation tools are continuously learning and improving with each new dataset they encounter. As the automotive field introduces new technologies and measurement units, the translation tools can dynamically adjust. This adaptive capability is crucial for accuracy and maintaining relevance in the long run. However, it raises the concern about the control and oversight required for these continuously evolving models. How do we ensure the quality and direction of learning is aligned with industry standards and ethics?
Additionally, these tools now feature advanced error detection mechanisms, which is particularly important within the automotive industry where even minor errors in specifications can lead to significant consequences. These systems are essentially doing a real-time quality check on translations. Yet, this begs the question: can we trust these AI-driven error-checking systems implicitly? I'd be interested in analyzing the rates of human-versus-AI-identified errors in the field to gauge trust and evaluate performance.
Neural networks allow for impressively rapid translation of vehicle specifications, often under two minutes. This accelerates the entire workflow within the industry, facilitating quick decisions and adaptive responses to market changes. It seems amazing that a human-level task like technical translation can now be performed with such speed. However, we need to be cognizant of the potential for errors to slip through the cracks due to this rapid pace. Perhaps there's a balancing act between the speed of these translation systems and ensuring their reliability.
The transition to AI-powered translation services also brings about substantial cost-efficiency benefits. Companies can reduce reliance on human translators, streamlining operations and reducing costs. It's an enticing proposition for businesses looking to cut costs. However, we shouldn't forget about the potential implications for human translators and the jobs that rely on these traditional methods.
Neural networks are becoming better at grasping the subtleties of language, particularly the regional variations and terminology used in the automotive industry. This is essential for removing ambiguities in technical communications. I'm curious to see how this nuance impacts different cultures and whether these tools are truly adaptable to all linguistic groups, not just those with a heavy influence of English.
Furthermore, a forward-thinking approach involves utilizing synthetic data to enhance the training process for AI. This method enables the models to learn from a much broader range of potential scenarios, improving their reliability and adaptability. However, the development and utilization of synthetic data needs to be approached carefully. What kinds of biases or limitations might arise when training models on this type of simulated data? These are questions that require more research and discussion.
The increasing prevalence of AI-driven translation technologies has the potential to create industry standards in how vehicle specifications are presented. This would make it much easier to compare vehicles across regions and enhance transparency for consumers. However, I'm concerned about who defines and controls these standards, especially in a globally competitive environment. There needs to be transparent governance and collaboration among industry stakeholders to achieve standardization that is truly useful for everyone.
In summary, while these advancements in cross-platform mobile power unit translation hold great potential for globalizing the automotive industry, it's important to maintain a critical eye on potential limitations, biases, and implications for the broader landscape. As researchers and engineers, our responsibility lies not just in developing these tools but also in scrutinizing their implications and advocating for responsible innovation.
Automated Translation of Vehicle Power Specifications Converting Horsepower to Kilowatts Across Global Markets - Real Time Automotive Database Updates Keep Unit Conversions Current
Keeping unit conversions accurate, especially when translating vehicle power from horsepower to kilowatts across the globe, relies heavily on real-time updates to automotive databases. This continuous flow of fresh information is critical for ensuring that manufacturers and consumers have access to up-to-date and correct data, which is essential for meeting global regulations and making informed choices about vehicle purchases. The increasing connectivity of vehicles provides a large source of real-time data that can be used to identify and fix problems, even speeding up processes like recalls. However, maintaining the currency and accuracy of this real-time data isn't simple. Data freshness and reliability are still challenges that require continuous effort in the design and implementation of automated systems. While automation helps, a degree of human scrutiny is crucial to ensure that the systems are consistently generating precise results. Otherwise, there's a risk of these automated solutions introducing errors or inaccuracies.
Real-time updates to automotive databases are crucial for keeping unit conversions accurate, especially when dealing with global markets and translating vehicle specifications like horsepower to kilowatts. This is essential for ensuring compliance with diverse international regulations. By 2018, nearly 40% of US vehicles were equipped with connectivity, a trend that's only grown since. The increase in connected vehicles means a surge in real-time data available to manufacturers, potentially improving diagnostics, recalls, and product development. It is fascinating how this huge increase in real-time vehicle data helps improve the efficiency of machine learning in areas like vehicle diagnostics. However, managing the timeliness and accuracy of this continuous flow of data is still a challenge, especially within vehicle control systems.
Interestingly, traditional methods of conversion between horsepower and kilowatts were plagued by errors, with rates approaching 15%. However, AI-driven translation systems powered by neural networks have substantially reduced these errors to less than 2%. This dramatic improvement is important not only for better compliance with local regulations but also to reduce any confusion for potential buyers who may misinterpret the translated data. Furthermore, AI is getting better at recognizing and translating automotive-specific language, which is essential for avoiding inaccuracies and promoting global understanding of technical documents. These gains in the quality of translation are achieved by training these AI models with large datasets that include a wide variety of vehicle specifications and technical details. We see increased use of synthetic data as a means to expand the breadth of these datasets and improve the AI's accuracy in dealing with diverse real-world documents. The goal here is to translate more efficiently and accurately while reducing errors and mitigating potential cultural or regional biases.
Though impressive, these models require a delicate balance between processing speed and accuracy. Neural networks can process vehicle specs in under 2 minutes, a significant increase in speed when compared to the old methods. However, the tradeoff is that errors might slip through the cracks when using this new faster approach. Maintaining oversight and having quality checks that spot errors in real-time is essential. Another interesting development is the potential cost savings with the introduction of AI translation tools. Reduced reliance on human translators can lead to efficiencies and a lower overall cost for translation processes. But we need to also consider what happens to the translator workforce as these AI systems become more commonplace. The potential to establish global standards for how vehicle specifications are presented is exciting. However, the development and enforcement of those standards must be approached carefully, ensuring that they are fair and helpful to everyone in the global automotive ecosystem. We're moving towards a more consistent and streamlined flow of vehicle data across international markets, which will undoubtedly lead to some interesting advancements and impacts on the automotive landscape.
AI-Powered PDF Translation now with improved handling of scanned contents, handwriting, charts, diagrams, tables and drawings. Fast, Cheap, and Accurate! (Get started for free)
More Posts from aitranslations.io: