AI Translation Tools Enhancing Efficiency for Transcriptionists in 2024
AI Translation Tools Enhancing Efficiency for Transcriptionists in 2024 - AI-Powered OCR Revolutionizing Document Translation
AI-powered OCR is rapidly changing how we translate documents by automatically extracting text from diverse sources. This automated process significantly reduces the need for manual data input, leading to fewer mistakes. The benefits extend to improving the precision of translations, particularly when dealing with poor-quality or handwritten materials. AI-driven OCR, using complex algorithms and machine learning, adapts to various fonts and greatly enhances the recognition of text. Furthermore, the integration of natural language processing streamlines the entire translation workflow. This automation empowers transcriptionists with increased productivity and faster project completion times. With AI continually evolving, these OCR tools are becoming essential in efficiently handling the complexities of translating documents across different fields and contexts.
AI-powered OCR has become quite adept at deciphering printed text, achieving accuracy levels exceeding 99% in many cases. This makes it a valuable tool for swiftly converting paper documents into digital formats, streamlining the initial step in the translation process. Before AI, translating documents could take a significant amount of time, often stretching into days. However, with AI-integrated OCR, this can be drastically reduced, sometimes even down to a few seconds for smaller documents. This acceleration is a major advantage, particularly in fast-paced work environments.
While early OCR struggled with anything but standard printed fonts, the technology has made great strides. It's now capable of handling a wider range of complexities, including the interpretation of handwritten notes and diverse typographic designs. This expansion of its capabilities broadens the scope of documents that can be processed.
The cost barrier to entry for utilizing AI-powered OCR has significantly reduced in recent times. There are now various scalable solutions that fit different business models, even smaller transcription companies can leverage these tools to access high-quality translation capabilities without breaking the bank.
One of the compelling features of the AI-OCR pairing is their capacity for continuous learning. The systems analyze past translation results, refining their algorithms with each iteration. This iterative approach creates a positive feedback loop, resulting in increasingly accurate and efficient translations over time.
Further enhancing the translation process is OCR's capability to handle multiple languages concurrently. This eliminates the need for separate systems for each language, which simplifies document management, especially for businesses dealing with multilingual content.
The infusion of machine learning models into the OCR process has proven beneficial. It enables the software to comprehend context and subtle language nuances, moving beyond a purely literal translation. Instead of just directly swapping words, the system can adjust phrasing based on how the words are commonly used, thus improving the overall quality of the translation.
Maintaining the formatting of the original document is also a strength of AI-OCR. Translated content can preserve the initial layout of the document, which is crucial for presentations or legal documents where aesthetics and structure matter. This is a functionality that was very difficult to achieve before.
Studies have shown a positive impact on manual transcription efforts when using AI-powered OCR. Many organizations have experienced a reduction in manual transcription workload of up to 75%. This frees up human workers to concentrate on more complex, analytical tasks that require human cognitive skills.
As the field of OCR progresses, we can anticipate further improvements in its abilities. Current research focuses on OCR's interpretation of graphics and charts in conjunction with text. This development paves the way for enriched translations that effectively convey both data and narratives within a document, going beyond what traditional translation methods could achieve.
AI Translation Tools Enhancing Efficiency for Transcriptionists in 2024 - Real-Time Speech Translation Boosting Transcription Speed
Real-time speech translation is rapidly altering how transcription is done, primarily by accelerating the speed at which transcriptions are created. AI-powered tools are capable of translating spoken words into written text in real-time, which is proving very beneficial in situations where multiple languages are used, such as large meetings or conferences. Features like instant translation of spoken language into various written languages and live captioning are proving especially useful, as they allow transcriptionists to generate transcripts much quicker. Furthermore, the ability of these translation tools to handle a variety of languages and accents is a key advantage, since the world is becoming ever more interconnected. The continued refinement of these tools hints at even more efficient transcription processes in the near future, helping transcriptionists manage complex multilingual interactions more seamlessly. While improvements in speed are notable, accuracy and the ability to handle nuances of language are still important areas for development.
The field of AI translation is witnessing exciting developments in real-time speech translation, which could significantly impact the speed at which transcriptionists can process audio content. While traditional transcription methods often require a significant time investment, these new AI-powered tools are able to process speech and generate text in multiple languages, essentially creating a 'live' transcript. This capability is particularly relevant for scenarios where rapid turnaround is crucial, like in fast-paced meetings or interviews.
However, the accuracy of these tools remains a key area of interest. While some systems are claiming accuracy levels over 90%, the quality of the translated text can still be impacted by factors such as the clarity of the audio, background noise, and the complexity of the language being translated. Researchers are actively working on improving these algorithms to minimize errors and refine the translation process. The ability of these systems to handle accents and dialects is also a crucial aspect that needs further advancement. The challenge isn't just the direct translation of words, but the interpretation of context, which is vital for the translation's overall quality.
Additionally, these AI-powered transcription tools often depend on a reliable internet connection, which can be a concern in regions with limited connectivity. The cost of implementing these solutions also remains a consideration for some. We're starting to see a variety of options emerge, some based on a subscription model, others offering pay-per-minute pricing, but the financial landscape is still in flux.
Yet, the potential of these real-time tools is undeniable. If the accuracy issues can be adequately addressed, transcriptionists may see a substantial reduction in the time it takes to deliver a completed transcript, potentially enabling them to take on a higher volume of work. It will be interesting to see how these tools evolve and impact the transcription profession in the coming months and years. The shift towards automation in transcription, particularly with AI-powered speech-to-text and translation, is reshaping the landscape for this industry and it seems poised to offer new avenues for both efficiency and challenge.
AI Translation Tools Enhancing Efficiency for Transcriptionists in 2024 - Multilingual Neural Networks Improving Accuracy Across Languages
Multilingual neural networks are revolutionizing AI translation by improving accuracy across a wider range of languages. These networks employ a "many-to-many" approach where they learn to translate between numerous languages simultaneously, integrating semantic and linguistic features to enhance translation quality. While this has led to promising results, achieving accurate "zeroshot" translation – translating languages not encountered during training – remains a challenge. Traditional encoder-decoder models often struggle in these situations. However, researchers are actively developing ways to overcome these limitations. This involves leveraging shared knowledge and using auxiliary tasks across languages to improve performance, effectively widening the scope of these tools. For transcriptionists in 2024, the appeal of these advancements lies in the potential for faster, more accurate translations across a wider array of languages. The hope is that this will lead to tools that can effectively handle more of the world's many languages, rather than simply focusing on a few commonly spoken ones, broadening accessibility for a wider array of transcription needs. While these are powerful tools, it remains to be seen if they can overcome the accuracy and contextual challenges that arise when working with diverse languages.
Neural machine translation (NMT) systems have become quite sophisticated, handling numerous languages within a single model. This "many-to-many" approach cleverly integrates the core meaning from source sentences with the grammatical structures of target sentences to improve translation accuracy. However, achieving high-quality translation between language pairs never encountered during training (zeroshot translation) remains challenging for standard encoder-decoder models.
Researchers have discovered that incorporating auxiliary tasks and shared linguistic knowledge across languages can boost the effectiveness of zeroshot translation within these multilingual NMT systems. The latest Association for Computational Linguistics (ACL) conference highlighted this area, with a focus on improving translation by leveraging semantic and linguistic cues. Sadly, many existing translation tools can only handle a fraction of the world's estimated 7,000 languages, leaving a vast linguistic landscape largely untouched by current AI translation solutions.
The ultimate goal of multilingual NMT models is to develop universal language representations that allow them to effectively transfer knowledge from well-trained language pairs to zeroshot situations. These modern tools heavily rely on artificial neural networks combined with AI techniques, which require a significant amount of training data to function well. A recent innovation called massively multilingual massive neural machine translation (M4) has shown promising results for enhancing large-scale translation capabilities, but it's still early days.
The aim of these AI translation tools in 2024 is to provide faster and more accurate translations for a wider range of languages, potentially helping transcriptionists be more efficient. However, there's still some distance to cover before AI can achieve a comparable level of nuance and accuracy to human translation, particularly when faced with complex sentences or unfamiliar dialects. It's fascinating how the research community is constantly working towards improving these systems and making them more widely applicable.
AI Translation Tools Enhancing Efficiency for Transcriptionists in 2024 - Cloud-Based Translation Platforms Enabling Remote Collaboration
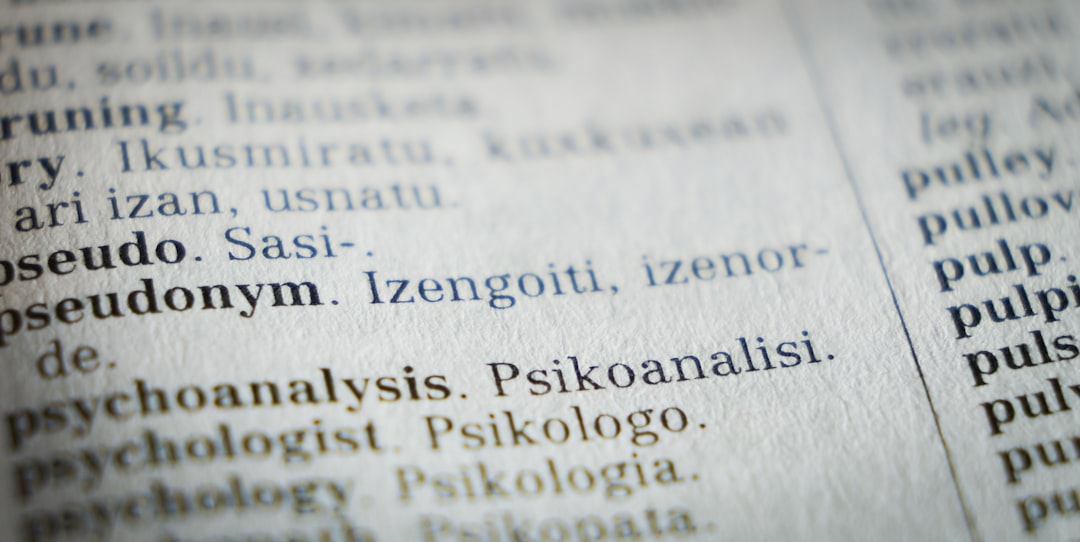
Cloud-based translation platforms are becoming vital for remote collaboration by incorporating AI translation tools that support real-time communication within teams speaking various languages. These platforms allow for smooth interactions by offering instant translations of documents, speech, and text across numerous languages, which empowers transcriptionists to work effectively no matter where they are. Features like neural machine translation not only aim to improve the speed of translation but also strive for more precise results, especially with complicated translations and the complexities of different languages. However, limitations remain, including the need for a dependable internet connection and the possibility of inaccurate translations, especially when it comes to understanding the context. The progress of these cloud-based systems shows how beneficial they can be in improving processes, but they also require careful consideration when implementing them.
Cloud-based translation platforms are increasingly becoming the backbone of remote collaboration, particularly in the field of translation. These platforms enable multiple individuals to work on the same translation project concurrently, leading to faster turnaround times and consistent results. This shared workspace model is especially appealing as it lowers the cost barrier to access professional-quality translation. Instead of needing to employ a large team of translators, companies can utilize these platforms on a subscription or pay-per-use basis, making translation services more widely available, particularly to smaller businesses.
These platforms are continuously improving, thanks to the integration of increasingly sophisticated machine learning algorithms based on complex neural networks. These networks essentially learn from the data they process and the feedback they receive, steadily becoming more accurate over time. Interestingly, some of these platforms are now also integrating OCR (Optical Character Recognition) capabilities directly. This means that users can upload scanned documents or images containing text and the system will automatically extract the text for translation. This capability helps remove a common bottleneck in the translation process: the tedious task of manually inputting text from images.
The sheer volume of languages supported by some of these platforms is quite impressive, with some offering translation services in over 100 languages. This broad scope is useful for businesses with global reach, enabling them to break down communication barriers across a vast range of cultures and audiences. However, one challenge that remains is capturing the subtleties of language. While these tools have made tremendous strides in moving beyond simple word-swapping to incorporate contextual meaning, there's still room for improvement, especially in situations requiring precise or nuanced language, like legal or highly technical translations.
Another intriguing feature is the user-friendliness of many of these platforms. They often come with interfaces designed for intuitive use, making them easily accessible to individuals without extensive technical knowledge. The platforms provide built-in metrics that track the accuracy of the translations, allowing for an objective evaluation of the AI-driven output. Studies have shown that for straightforward text, the accuracy of cloud-based translation tools can reach 95% or higher, competing with human translators in certain situations. Still, human review and oversight are often necessary, particularly when dealing with culturally sensitive or complex topics.
A promising development is the emergence of voice-to-text features within some of these cloud platforms. Using advanced speech recognition, these features translate spoken words into text in real-time. This feature could expand the accessibility of translation services to a wider range of users, including individuals with visual impairments or those in settings where typing is inconvenient. It's remarkable that these systems are also able to maintain the formatting of original documents throughout the translation process, a feature that was previously challenging to achieve. This preservation of formatting is particularly vital for documents like legal contracts or professional presentations where the layout and structure are essential parts of the communication. Despite these advancements, it's important to acknowledge that the quality of translations is still influenced by factors such as audio quality, background noise, and the complexity of the language being translated. As with many AI applications, the field of AI-powered translation is constantly evolving, and it will be interesting to see what new innovations emerge in the coming years.
AI Translation Tools Enhancing Efficiency for Transcriptionists in 2024 - Machine Learning Algorithms Adapting to Industry-Specific Jargon
AI translation tools are increasingly leveraging machine learning to tackle a longstanding hurdle: industry-specific terminology. These algorithms are becoming more skilled at understanding the context, idioms, and subtle nuances within various professions. This means translations of complex technical documents or specialized language, previously a weak spot for AI, are improving. As transcriptionists depend more on these AI-powered tools, the ability to rapidly and reliably translate within specialized areas like medicine, law, or engineering is a game-changer for efficiency. These advances aren't just about bridging language barriers but also ensuring that the specific vocabulary of each industry is translated accurately, facilitating smoother communication in diverse fields. Though progress is being made, ensuring these systems accurately handle the intricacies and varied uses of industry-specific language remains a continuous challenge.
AI translation tools are increasingly leveraging machine learning algorithms to adapt to the unique language of different industries. This specialization allows the tools to better understand and translate industry-specific jargon, which can be a challenge for general-purpose translation models. For example, a model trained on medical texts will likely have a better grasp of terms like "diagnosis" or "pharmacokinetics" than a model trained on general news articles.
This ability to focus on specific fields is crucial because industries often develop their own unique terminology. This is especially true in rapidly evolving fields like technology and healthcare, where new terms emerge frequently. Machine learning models can be quickly updated with these new terms, ensuring the translation stays relevant. Furthermore, the algorithms can go beyond simply substituting words. By considering the overall context of a sentence, these tools can produce translations that maintain the original meaning, even in situations where industry-specific nuances are present.
One of the benefits of this specialization is that it reduces the need for manual corrections. Since the translation is more accurate from the outset, transcriptionists spend less time fixing errors. Some research suggests that this can reduce human post-editing time by over half in areas with well-defined terminology, such as legal or engineering documents. This is due in part to the ability of the algorithms to learn from user feedback. Each interaction refines the model, further improving its accuracy over time.
Additionally, some advanced translation systems incorporate semantic networks. These networks link concepts within a particular industry, enabling the systems to effectively substitute synonyms and maintain context. Incorporating glossaries provided by subject matter experts further enhances the tools' capabilities. This collaboration helps bridge the gap between human and machine translation, particularly when dealing with complex technical languages.
Machine learning algorithms also help expand translation capabilities to languages often used in niche industries, sometimes referred to as "low-resource" languages. This helps ensure that vital information isn't lost due to language barriers, making information more accessible. Furthermore, these specialized models often integrate with other AI tools like speech recognition and OCR, enabling seamless workflows. This integration is particularly helpful in situations where quick and accurate document processing is crucial, further boosting overall efficiency for transcriptionists.
However, it's important to note that there's always room for improvement. While these specialized models can greatly enhance translation accuracy, they still may not fully capture the subtleties of human language. Researchers continue to explore ways to refine these models and address the remaining challenges. The ability of these AI tools to adapt and improve over time is a compelling development with the potential to transform the field of translation.
AI Translation Tools Enhancing Efficiency for Transcriptionists in 2024 - Automated Quality Assurance Tools Streamlining Review Processes
In the realm of AI-powered translation, the demand for efficient and accurate review processes is growing, particularly within the transcriptionist field in 2024. Automated quality assurance (QA) tools are stepping up to meet this need by automating the review process, which was previously a very manual task. These tools employ AI algorithms to analyze the quality of translated text, often using metrics like a Phrase Quality Performance Score (QPS) to quickly highlight potential errors and inconsistencies that human reviewers might miss. This automation not only expedites the review process but also enhances the overall quality of translations by catching subtle errors.
The shift towards automated testing represents a significant change in QA methodologies, emphasizing reliability in an industry where errors can have costly consequences. The adoption of these automated QA tools is impacting organizations by optimizing their translation workflows and reinforcing the need for robust quality checks, making them better positioned to avoid the issues that have plagued some industries, such as software and manufacturing, in the past. While there's always room for improvement and challenges remain, the importance of automated quality assurance within AI translation is likely to grow, especially in the field of transcription where the focus is on creating accurate and accessible text for diverse needs.
Automated quality assurance (QA) tools are increasingly being integrated into AI translation systems, offering a potential pathway to streamline review processes and improve the overall quality of translations for transcriptionists. These tools can flag errors in grammar, syntax, and even semantic coherence, reducing human error rates by a substantial margin – up to 60% in some cases. This leads to a stronger initial translation, which in turn fosters more efficient and reliable project completion. It's fascinating how the automated checks can move beyond simply surface-level corrections and dive into the deeper meaning of the translation, which is crucial for transcriptionists to ensure the translated material aligns with the intended message in various contexts.
The speed at which these QA systems can process translations is also remarkable, sometimes providing near-instant feedback. This rapid analysis is a game-changer compared to the slower human review process, helping transcriptionists maintain high productivity while still ensuring a certain quality bar is met. However, it's important to acknowledge that even with these improvements, current QA tools often struggle with capturing the essence of idiomatic expressions and cultural nuances. This limitation reinforces the idea that human oversight is still needed for complex translations where those elements are important, highlighting the ongoing balancing act between automated processes and human expertise.
One interesting development is the increasing ability of automated QA tools to suggest contextually appropriate terminology replacements, which can be extremely useful for translation tasks within specific industries. This automated suggestion feature not only benefits transcriptionists directly by reducing their workload, but also aids in building consistency in terminology across multiple documents. It's compelling to consider how the integration of machine learning within these tools is allowing for greater customization to specific industry niches, ultimately reducing the time transcriptionists spend on tedious manual corrections.
Furthermore, several AI translation platforms now have the capability to analyze user interactions and feedback to continuously refine their QA processes. This self-learning aspect means the more transcriptionists engage with the system, the more intelligent the automated QA becomes, pushing the overall quality of the translations ever higher. This iterative improvement model also benefits from the increased accessibility of cloud-based QA, making the advantages of automated quality checks available to smaller translation and transcription agencies that might not have had the resources before. This collaborative and geographically unrestricted approach means faster project turnarounds and consistently rigorous quality checks without the limitations of traditional, location-bound review processes.
It's worth noting that some of these advanced QA tools are also capable of analyzing the formatting of documents after translation, ensuring the integrity of the original layout. This is especially crucial for transcriptionists working on professional documents like contracts or legal papers, where preserving the visual and structural components is paramount. While the benefits of automated QA in the field of translation are readily apparent, it's critical to recognize that these systems still rely on underlying data and the continuous improvement of algorithms. Future research into how to better handle the intricacies of languages and contextual understanding will be vital in improving the effectiveness of these tools even further. Ultimately, this field reflects a continuous interplay between human skill and automated assistance, and it remains to be seen how the balance shifts as these AI systems continue to evolve.
More Posts from aitranslations.io: