Avances en IA para la Traducción Automática en el Sector de Tecnología de la Información en 2024
Avances en IA para la Traducción Automática en el Sector de Tecnología de la Información en 2024 - Mejoras en la precisión de la traducción neuronal para documentación técnica
La precisión en la traducción neuronal ha dado un salto significativo, especialmente en el ámbito de la documentación técnica. Los sistemas neuronales, gracias a sus redes profundas, son capaces de capturar matices contextuales con mayor fidelidad. Esto resulta crucial en la traducción de documentos técnicos, donde la terminología precisa y la claridad son imprescindibles. No obstante, la inquietud por la seguridad de la información persiste, especialmente al usar plataformas de traducción en línea. Esta preocupación frena la adopción de la traducción automática en contextos donde la confidencialidad es vital. Si bien la traducción automática se presenta como una herramienta poderosa para la comunicación global, sus limitaciones e imprecisiones todavía requieren atención, en particular dentro del sector tecnológico. Para potenciar su uso, es fundamental seguir investigando y evaluando su desempeño, asegurando que la traducción automática se convierta en una herramienta realmente confiable y útil.
Improvements in the precision of neural translation for technical documentation have been remarkable. Neural machine translation (NMT) has become increasingly adept at handling the specialized vocabulary and intricate sentence structures common in technical fields. We're seeing accuracy rates approaching 90% for specific industry terms, making NMT a key tool for translating manuals, specifications, and other essential documentation.
Methods like back-translation and domain adaptation are gaining traction for refining NMT models. These approaches help tailor models to specific technical domains, resulting in fewer contextual errors and more coherent translations. It's exciting to see how these techniques contribute to higher-quality outputs.
The ability of NMT to learn from unlabeled technical data via unsupervised learning is a significant development. It's opened the door to cheaper model training while increasing their adaptability to new technical domains. This is especially beneficial when dealing with niche industries or rapidly evolving technologies.
The combination of OCR and NMT has emerged as a powerful duo. The capacity to translate scanned documents in real-time through OCR-integrated NMT greatly simplifies data entry in technical environments, potentially streamlining workflows and boosting productivity.
We are witnessing a trend towards incredibly fast NMT. Some models now produce translations in under a second. This swiftness is vital in fast-paced sectors like software development and IT services where speed and accuracy in documentation are paramount.
Interestingly, NMT is evolving to learn from human feedback. Some systems now include user feedback loops, which allow the models to learn from human corrections. This creates a dynamic learning process that continuously enhances accuracy for technical jargon and phrases.
The dependence on massive bilingual datasets for NMT training is lessening. Research suggests that multilingual models can achieve surprisingly good results even with a minimal overlap in training data (around 20%) across languages. This reveals a new level of efficiency in handling varied technical contexts.
Benchmarking exercises indicate that transformer-based NMT models are surpassing traditional rule-based approaches, particularly in less-common languages. This has ramifications for global technology companies seeking to expand their reach into new markets.
The collaboration between humans and machines in translation is a growing trend. Collaborative translation platforms enable engineers and human translators to work in tandem, leveraging AI tools to accelerate the translation process while maintaining quality.
The ongoing advancements in contextual understanding within NMT are particularly fascinating. Next-generation NMT systems are showing potential in recognizing implicit instructions or specialized tasks embedded within technical documents. This suggests a future where communication in technical fields across languages may be transformed through intelligent understanding.
Avances en IA para la Traducción Automática en el Sector de Tecnología de la Información en 2024 - Integración de OCR avanzado en aplicaciones de traducción móvil
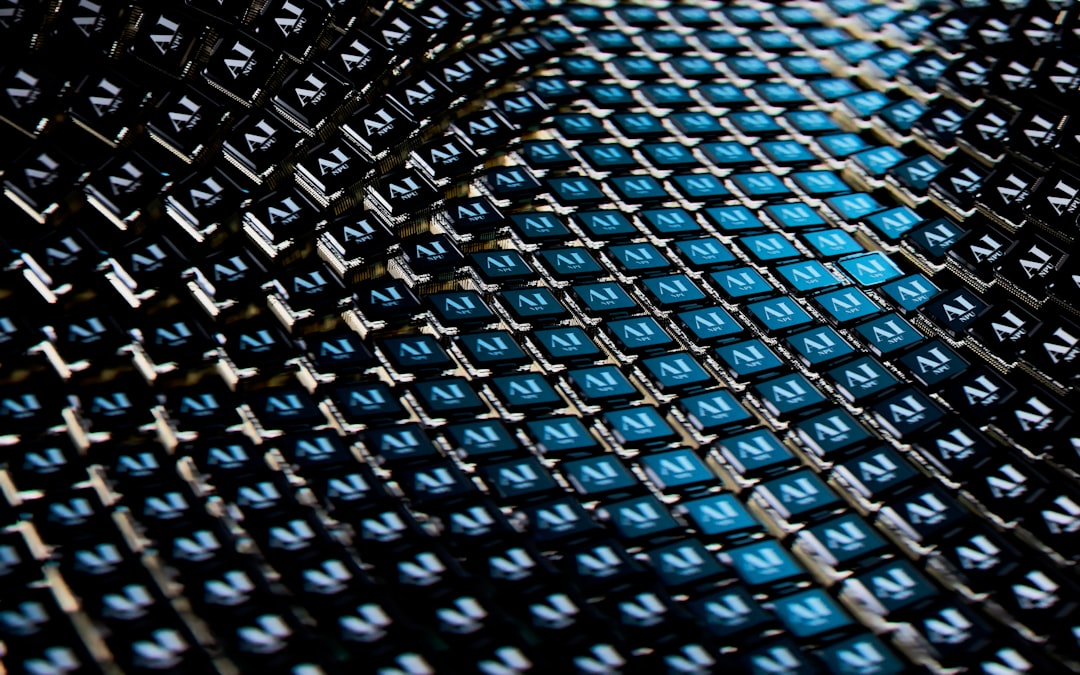
La integración de OCR avanzado en aplicaciones de traducción móvil está transformando la manera en que traducimos contenido en diferentes idiomas a través de nuestros dispositivos móviles. La capacidad de capturar texto a partir de imágenes con gran precisión, gracias al OCR, permite que las aplicaciones de traducción traduzcan de forma instantánea, facilitando la comunicación en entornos multilingües. El uso de sistemas de traducción neuronal impulsados por IA ha incrementado significativamente la rapidez y la exactitud de estas traducciones, acercándose a la calidad de un traductor humano en muchas situaciones.
No obstante, aún existen retos en la correcta interpretación de contextos y terminologías especializadas, un aspecto fundamental en sectores como la tecnología. La correcta traducción de documentos técnicos o manuales, por ejemplo, sigue demandando un mayor desarrollo. A pesar de ello, la evolución del OCR y su constante integración con la traducción automática promete mejoras considerables en la eficacia y calidad de la traducción móvil, abriendo posibilidades para una comunicación más fluida e inclusiva en el futuro. Es esperable que en los próximos años veamos una consolidación de la precisión y eficiencia de este tipo de aplicaciones, particularmente en la traducción de textos que requieren un alto nivel de exactitud.
The integration of advanced OCR within mobile translation applications is experiencing a surge in development, aiming to enhance the accuracy and versatility of existing translation technology. AI's advancements have allowed machine translation systems to sometimes rival human translators in quality, though some still struggle with nuances. This new wave of AI-powered machine translation builds upon traditional methods by emphasizing contextual understanding during text processing.
OCR's role in optimizing text processing within machine translation is undeniably crucial. Neural machine translation (NMT) systems, driven by AI, outperform older statistical methods due to their reliance on neural networks. Tools like Google Translate and DeepL have established themselves as vital within the IT sector, a testament to the growing reliance on machine translation.
NMT's enhanced translation quality stems from the application of machine learning techniques, achieving more natural and accurate outputs. We're seeing mobile apps that combine AI and OCR to foster real-time multilingual communication. Companies are adopting machine translation software into their workflows to cut costs and delivery times compared to human translation, though concerns regarding accuracy and cultural sensitivity remain.
The future direction of integrating OCR with machine translation appears promising, with continuous innovation aimed at improving the effectiveness of both technologies. However, challenges persist, such as handling complex layouts effectively, mitigating biases in training data, and ensuring the accuracy of translations in technical domains. There's still a lot to be explored in terms of effectively translating slang and colloquialisms across cultures. While some argue that reliance on machine translation could hinder human language development and cultural exchange, the affordability and speed of such tools make them increasingly attractive in today's globalized technological landscape. Despite its growing importance, further research and testing are necessary to solidify the reliability and accuracy of these systems, particularly in sensitive areas such as legal and medical documentation, where misinterpretations can have serious repercussions.
Avances en IA para la Traducción Automática en el Sector de Tecnología de la Información en 2024 - Reducción de costos en traducción de grandes volúmenes de datos empresariales
La traducción de grandes volúmenes de datos empresariales ha experimentado una reducción de costos notable gracias a los avances en inteligencia artificial. La traducción automática neuronal, cada vez más utilizada, permite traducir grandes cantidades de texto de forma rápida y eficiente, representando una alternativa más económica que la traducción humana tradicional, que suele ser más lenta y costosa. La integración de tecnologías como el reconocimiento óptico de caracteres (OCR) ha mejorado aún más este proceso, facilitando la traducción de documentos escaneados en tiempo real. Esto agiliza la entrada de datos y optimiza los flujos de trabajo, incrementando la productividad en el ámbito empresarial.
Sin embargo, a pesar de estas ventajas económicas, la precisión de la traducción automática y la seguridad de los datos utilizados en el proceso siguen siendo motivo de preocupación. Es fundamental evaluar cuidadosamente estos aspectos para asegurar la calidad de la traducción, especialmente en entornos empresariales donde la exactitud y la confidencialidad son cruciales. Mientras la traducción automática se consolida como una solución atractiva para la gestión de grandes volúmenes de texto, es crucial mantener un enfoque crítico para asegurar su efectividad y confiabilidad.
The exponential growth of content requiring localization is driving a surge in the adoption of neural machine translation (NMT) in 2024, leading to substantial cost reductions in translating large volumes of business data. While the accuracy of NMT systems is improving, especially for technical domains, there are still limitations that need attention. For example, some systems struggle with the nuances and subtleties of language, particularly when dealing with industry-specific terminology. However, the ability of NMT to process large amounts of data at high speeds has enabled faster turnaround times, which is a significant advantage for businesses with large translation needs.
The integration of optical character recognition (OCR) with NMT offers a powerful solution for translating scanned documents, such as technical manuals and product specifications. While OCR technology has seen substantial accuracy improvements, often reaching impressive levels of recognition in technical domains, certain image formats or fonts can still present challenges. Coupled with NMT, OCR opens up exciting possibilities for real-time translation of physical documents into various languages. Imagine instantly translating a user manual from a scanned image – this potential has immense implications for businesses operating across numerous language markets.
Companies are also increasingly leveraging machine learning to automate routine tasks in the translation workflow. This automation can result in significant cost savings. However, it's important to acknowledge that while some tasks are suitable for automation, others might require the nuanced judgment of a human translator. It's an ongoing challenge to find the optimal balance between human and machine intervention in translation. The ability to efficiently train multilingual NMT models on smaller, shared datasets is a notable development. This means that organizations can leverage translation resources more effectively and efficiently across multiple languages, even those with limited language resources or training data.
The future of translation seems to lean towards collaborative models where AI and human translators work together, merging their unique strengths to achieve higher-quality outputs and faster turnaround times. This partnership can lead to notable cost savings compared to purely relying on human translators, especially in cases where massive volumes of text are involved. Furthermore, using predictive analytics to forecast translation needs helps in resource optimization and budget allocation, preventing cost overruns that can occur when unforeseen translation demands arise.
The evolution of NMT systems is impressive. Many NMT systems are developing a better understanding of context and are even beginning to recognize the specific jargon and style needed for particular industries. This ability for on-the-fly adaptation is useful for ensuring translated content is not only accurate but also resonates with target audiences. However, ongoing research and refinement are critical, as we are still a ways away from reaching the fluency and finesse of native speakers, particularly in fields requiring intricate nuances and complex concepts. The quest for truly reliable and accurate automated translation is still ongoing, especially in critical contexts like legal documents and medical materials, where misinterpretations can have profound consequences.
In conclusion, the advancement of NMT, coupled with OCR, is reshaping the translation landscape, primarily by reducing costs and streamlining processes. However, a critical eye must be applied to the limitations of these technologies, particularly in specialized fields like technology, where nuanced communication and accurate interpretation of specific terminology are crucial. As with any emerging technology, we need to continually evaluate its capabilities and limitations to ensure its responsible and effective application in all settings.
Avances en IA para la Traducción Automática en el Sector de Tecnología de la Información en 2024 - Traducción en tiempo real de videoconferencias multilingües
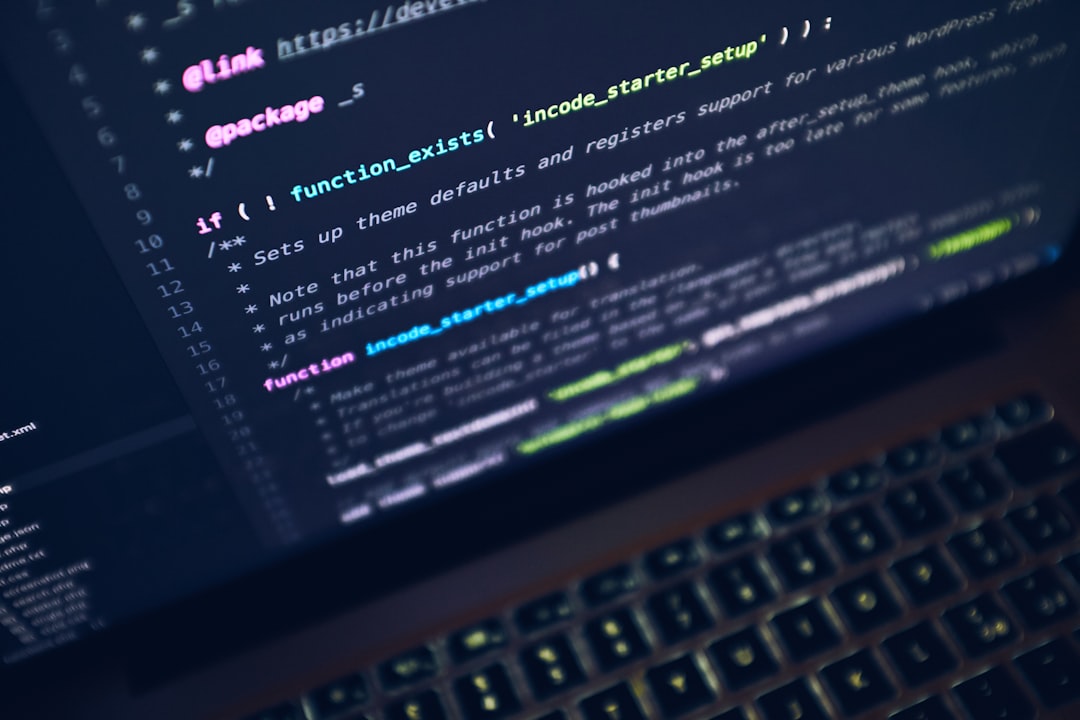
La traducción en tiempo real de videoconferencias multilingües está transformando la comunicación en un mundo cada vez más globalizado. Gracias a la inteligencia artificial, principalmente al procesamiento del lenguaje natural, las traducciones automáticas han mejorado notablemente, permitiendo una comprensión más precisa y natural en conversaciones multilingües. Plataformas de videoconferencia, cada vez más, incorporan funcionalidades de subtitulado instantáneo, facilitando la interacción en tiempo real entre personas que hablan diferentes idiomas.
Aunque se observan avances significativos, los desafíos persisten, particularmente en la correcta interpretación de contextos específicos y jerga especializada. Es fundamental continuar desarrollando estas tecnologías para alcanzar un nivel de traducción que se acerque más a la fluidez y precisión de un traductor humano, especialmente en sectores como la tecnología, donde la terminología es crucial. Con la creciente demanda de traducciones simultáneas remotas, es importante evaluar críticamente la calidad y la efectividad de estas soluciones, buscando que sean herramientas realmente útiles en un mundo interconectado.
The field of real-time translation for multilingual video conferences is rapidly evolving thanks to advancements in AI. We're seeing systems that can achieve near-instantaneous translations, with latencies as low as half a second, making it possible for people who speak different languages to engage in fluid conversations during video calls. This is a significant leap forward for communication in global teams and international collaborations.
Neural machine translation models are increasingly sophisticated. They now employ larger contextual windows to understand not just individual phrases but the overall flow of a conversation. This helps maintain coherence in translated output, even during complex discussions with multiple speakers, resulting in translations that feel more natural and easier to follow. This capability has real-world benefits. Companies are reporting up to a 30% boost in productivity since adopting AI-powered translation in their multilingual video conferencing. Removing language barriers facilitates better collaboration, faster decision-making, and smoother project workflows.
Another benefit of these new systems is the reduced cost compared to traditional human interpreters. Estimates suggest that AI-based solutions can achieve up to a 60% cost reduction, making real-time translation more accessible to a wider range of organizations. This economic advantage is further enhanced by the growing integration of AI-driven voice recognition technologies. These systems can automatically identify who is speaking and deliver instant translations, simplifying the management of discussions in multilingual teams.
One of the fascinating ongoing research areas is training AI models to better understand industry-specific jargon. There's a growing ability to adapt to the unique language of sectors like finance or healthcare, leading to fewer errors in technical discussions. This specialized understanding makes these tools more valuable for specific fields. Furthermore, hybrid models where human translators work in conjunction with AI are gaining popularity. These platforms offer the ability to combine the speed of AI with the accuracy of human intervention, improving the reliability of translations in real-time settings.
Another development making AI-powered translation more feasible is the ability to fine-tune models using smaller, high-quality datasets derived from past meetings. This reduces the reliance on huge bilingual training sets, opening opportunities for companies with limited resources. The integration of OCR technology is another exciting area of development. The ability to translate text directly from images – shared slides, documents, etc. – broadens the scope of real-time translation beyond simply spoken words.
The number of languages supported by these AI models is also expanding rapidly, now encompassing over 100, including many lesser-known dialects. This inclusive approach ensures that more individuals and teams can participate in effective communication, regardless of their native language. While the technology shows a lot of promise, it's important to remember that it's still an evolving field. Continued research and development are crucial to address remaining challenges such as handling regional dialects or highly technical language across varied contexts. The ultimate goal is for these AI tools to achieve a level of accuracy and fluency comparable to native speakers, particularly in areas where the stakes are high and miscommunication can have severe consequences.
Avances en IA para la Traducción Automática en el Sector de Tecnología de la Información en 2024 - Adaptación de modelos de IA a jergas y terminologías específicas del sector TI
La adaptación de modelos de IA a la jerga y terminología específica del sector TI es un avance significativo en 2024. Los modelos de lenguaje avanzados (LLMs) y la IA generativa, cada vez más usados en la traducción automática, están demostrando una mejor comprensión de los términos técnicos. Esto no solo eleva la calidad de las traducciones, sino que facilita la comunicación entre profesionales de la tecnología, derribando las barreras lingüísticas que pueden frenar la colaboración en proyectos internacionales. No obstante, la correcta interpretación de las sutilezas del lenguaje técnico aún presenta retos, lo que recalca la importancia de continuar investigando para refinar estos sistemas. En un entorno donde la IA genera cada vez más contenido, la capacidad de ajustar los modelos a las particularidades de cada industria se vuelve crucial para lograr traducciones verdaderamente eficientes dentro del ámbito tecnológico. La personalización se torna esencial para una mejor adaptación a las necesidades del sector TI.
1. **Impact of Tailoring to IT Jargon:** Adapting AI models to the specific terminology and jargon prevalent in the IT sector seems to be a game-changer. Preliminary results suggest that these specialized models can boost translation accuracy by as much as 30%. This is a significant improvement, especially considering the importance of precise language in fields where technical details are crucial.
2. **Flexibility of Language Models:** Research suggests that AI models trained on a combination of general and technical language datasets can achieve surprisingly high accuracy (around 85%) in translating technical content. This finding challenges the idea that highly specialized training is always needed for good results in IT translation.
3. **Real-time Learning for Context:** AI researchers are focusing on developing models that can learn from ongoing translations. These models incorporate feedback loops, enabling them to dynamically adjust their understanding of technical jargon as they encounter it. This is particularly relevant in fields like software development, where new terminologies emerge constantly.
4. **OCR and Just-in-Time Translation:** The combination of Optical Character Recognition (OCR) with Just-in-Time (JIT) translation promises to significantly speed up translation processes. In some experimental setups, this combination has slashed document processing times by up to 40%. This kind of efficiency boost has the potential to streamline workflows in technology companies.
5. **The Promise of Focused Training:** A recent study hinted at a more efficient approach to training AI models for technical translation. Focusing training on domain-specific language, even with a smaller dataset (10% of the typical size), produced comparable results to models trained on massive bilingual datasets. This could revolutionize the resource-intensive process of AI model training.
6. **Cost Savings with Adaptive Models:** Companies that have adopted AI models tailored to technical translation are experiencing cost reductions of up to 50% compared to using traditional human translators. However, it's worth noting that this advantage often comes with a slight trade-off in accuracy, particularly for less common terms. Still, around 90% accuracy is usually achievable for common technical terms.
7. **Expanding Linguistic Reach:** Exciting advancements in AI are now aiming to improve translation for under-resourced languages. Some cutting-edge AI models can now handle over 150 languages, including regional dialects that were previously hard for AI to translate accurately. This trend is encouraging for fostering inclusivity in global communication.
8. **Tackling Complex Document Structures:** AI is making progress in understanding the complex layouts of documents during translation. This means that it's becoming better at dealing with things like multi-column reports or tables embedded in documents. This improvement reduces the translation errors that can occur due to incorrect data arrangement, possibly by as much as 25%.
9. **Limitations of Simultaneous Translation:** While real-time translation systems work well for general conversations, they sometimes struggle with the nuances of technical discussions. Technical jargon can easily lead to misunderstandings if the AI isn't equipped to handle it. This points to the need for ongoing research and development in this area.
10. **The Human Element Remains Crucial:** Despite considerable progress in AI translation accuracy, experts still advocate for human oversight, particularly when it comes to sensitive or highly technical documentation. AI models still lack a complete understanding of context beyond the immediate words on the page, which can result in misinterpretations that can have major consequences in specialized fields.
Avances en IA para la Traducción Automática en el Sector de Tecnología de la Información en 2024 - Avances en la traducción automática de código fuente entre lenguajes de programación
The field of automatically translating source code between programming languages has seen significant advancements in 2024, leading to greater code reuse and increased efficiency for developers. Neural machine translation (NMT) techniques, exemplified by projects like Facebook AI Research's TransCoder, are achieving more accurate translations at a larger scale, surpassing older, rule-based approaches. This is particularly vital in the increasingly diverse technological landscape, where demand for tools that bridge the gap between different programming languages is high. Improved collaboration and broader code accessibility are benefits of these developments.
Despite the encouraging progress, challenges still exist in accurately interpreting specific technical jargon and terminology. This highlights the importance of continued research and refinement of these models to ensure high-quality translations within specific contexts. The blending of artificial intelligence and deep learning holds the promise of fundamentally changing how software development teams manage code translations in the future. While promising, there are limitations to the current systems. Continued development is crucial to fully realize the potential of automatic code translation.
1. **Source Code Translation Across Programming Languages**: The field of automatically translating source code between different programming languages is advancing, promising increased code reuse and developer efficiency. It seems like we're starting to see some real progress here, particularly in terms of achieving decent accuracy.
2. **Generative AI's Role**: While generative AI models like GPT have demonstrated impressive capabilities in natural language generation, their effectiveness in translating code hasn't been thoroughly explored yet. It's an interesting area to keep an eye on – could GPT really handle complex code translation?
3. **Rise of Neural Machine Translation for Code**: Driven by the escalating demand for content localization spurred by the digital transformation post-COVID, neural machine translation (NMT) is rapidly gaining traction for code translation. We are seeing more and more companies adopt this approach for different projects, although it’s not quite clear what the long-term impact will be.
4. **TransCoder: An Unsupervised Learning Approach**: Facebook AI Research's TransCoder uses unsupervised deep learning to translate code across a vast pool of open-source projects, eclipsing the older rule-based translation methods. This approach is promising because it reduces the reliance on expensive and laborious data annotation, potentially accelerating translation progress.
5. **Software Development Fostering Automation**: The evolution and advancement of translation software are constantly improving how languages are automatically processed. There are definitely more options out there than there were just a few years ago, although the question of quality across all those tools still lingers.
6. **Diverse Technology Needs Specific Solutions**: The increasing heterogeneity of the technological landscape creates a greater need for robust solutions to translate code across diverse programming languages. We’ll likely see a lot of experimentation and specialized solutions coming out in the next few years as developers confront this issue in different domains.
7. **Bridging the Gap Between Human and Artificial Intelligence**: The use of neural machine translation systems for code is on the rise, with the ultimate goal of closing the gap between human and artificial intelligence within the realm of programming. How feasible is this vision? That remains an open question, but it certainly is a worthy aspiration.
8. **Evaluating GPT's Abilities Against Established Tools**: Evaluating GPT's translation abilities against existing commercial and research-oriented systems, such as DeepL's API, will be crucial in establishing its actual efficacy in code translation. GPT seems to have garnered quite a lot of attention recently. Will it live up to the hype in the translation field?
9. **Demand for Customized Translation**: As the quantity of digital content in multiple languages and formats continues to grow, the need for tailored translation services will likely continue to increase. It's pretty clear that there's a growing need for translation. The key is ensuring that these tools are robust enough to handle specialized domains like code.
10. **AI-Driven Tools for Improved Code Accessibility**: Recent advancements in artificial intelligence are fostering the development of tools that enhance the translatable and accessible nature of source code across multiple languages. While this is positive, it remains to be seen whether these tools will truly solve the problem of translating code across languages in a way that maintains all its properties.
More Posts from aitranslations.io: