Common Machine Translation Pitfalls 7 Lessons from 2,000 Hours of Professional Translation Reviews
Common Machine Translation Pitfalls 7 Lessons from 2,000 Hours of Professional Translation Reviews - Untrained OCR Software Creates Major Format Issues in Legal Documents
Untrained or inadequately configured optical character recognition systems pose significant obstacles when handling the unique properties of legal documentation. These tools frequently struggle to accurately capture the complex layouts, specific structural elements, and diverse formatting prevalent in legal texts. The result is often unreliable conversions that introduce errors, disrupt flow, and scramble data presentation. Such inaccuracies hinder effective data extraction and management, potentially jeopardizing document integrity and complicating necessary record-keeping or compliance efforts. Compounding these technical limitations, the specialized and often nuanced nature of legal language itself can confound basic OCR processes, making it difficult to ensure the fidelity and usability of the digitized output.
Basic optical character recognition, particularly versions not specifically trained on the nuances of legal documentation, frequently introduces significant issues. One fundamental problem lies in its handling of varied typography. Minor differences in font styles, sizes, or even the spacing between characters can lead the software to misinterpret text. This isn't just about aesthetics; confusing similar-looking characters like '1' and 'l' or '0' and 'O', or simply failing to recognise less common typefaces accurately, can fundamentally alter numbers, dates, or names – potentially injecting serious errors into legal text. Challenges are often amplified when dealing with languages that utilise unique scripts, where untrained systems might produce garbled output or omit entire sections of text.
Beyond individual characters, the structure of legal documents presents another hurdle. They are rich in specific formatting elements like complex tables, detailed footnotes, and carefully structured citations. Standard OCR tools often struggle to retain this structure. They might flatten tables into unusable lines of text, discard or misplace footnotes, or fail to identify and correctly render citations. This corruption of the document's layout also affects the flow and alignment of text, causing a loss of logical context which is vital for understanding the original argument and scope of the document. Moreover, handwritten annotations or marginalia, frequently found in legal documents and often holding critical information, are typically ignored entirely by basic OCR, compromising the completeness of the digitised record.
The reliability of OCR output is highly dependent on input quality. Evaluations show that accuracy rates can plummet well below 50% for documents that are poorly scanned, creased, or contain unusual formatting or colours. In a field where precision is paramount, such instability is a major concern. Critically, errors introduced at this initial stage propagate. Feeding flawed text into subsequent processes, such as machine translation, exacerbates the problem. It can lead to inconsistent terminology, as the system may struggle to recognise legal jargon accurately, or result in translations that lack the precise nuance captured in the original text.
The allure of rapid processing and seemingly low initial cost associated with basic, untrained OCR can be misleading. While fast, this speed often comes at the expense of accuracy, creating a need for extensive manual review and correction downstream. Rectifying character misinterpretations, restoring complex formatting, and ensuring the logical flow of the document requires significant human effort during post-editing. This often negates any initial cost savings and can significantly delay subsequent processes, such as translation and review, ultimately creating a false economy and increasing long-term operational costs due to the embedded inefficiencies. The push for speed without robust error mitigation pathways introduces substantial risk.
Common Machine Translation Pitfalls 7 Lessons from 2,000 Hours of Professional Translation Reviews - Raw Machine Translation Fails at Chinese Four Character Idioms
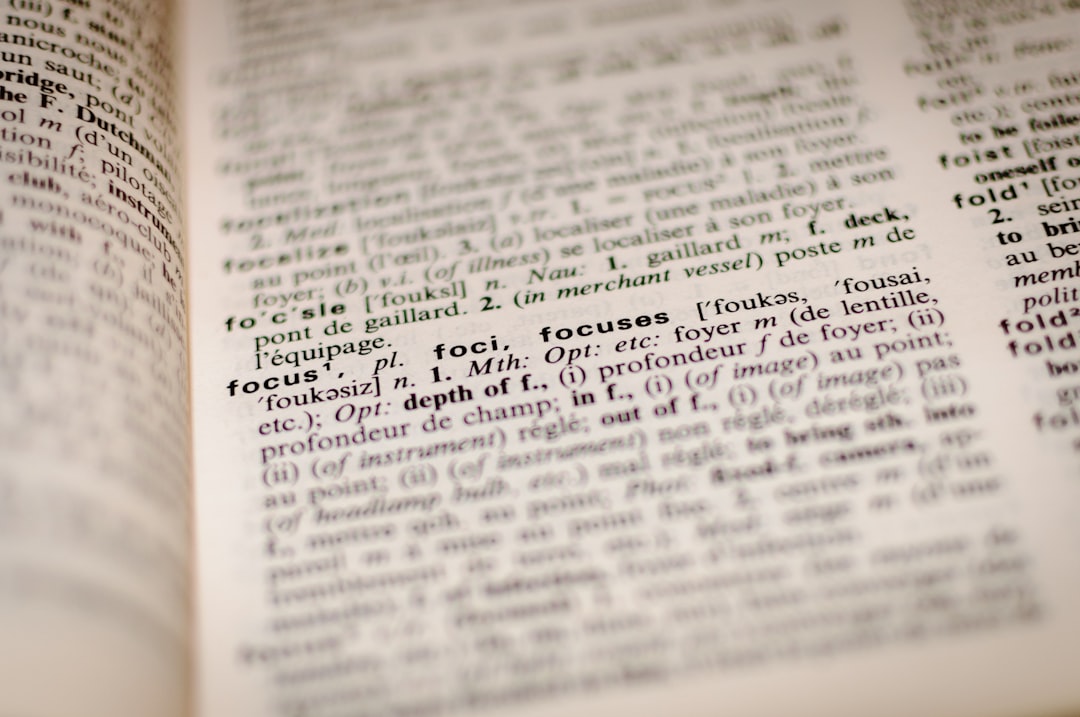
Chinese four-character idioms, known as Chengyu, consistently present a difficult hurdle for raw machine translation systems. Their meanings are frequently not derived from the individual characters themselves, making a straightforward word-for-word translation fundamentally inadequate. Machine algorithms commonly fail to capture the deeper cultural significance and subtle layers of meaning embedded within these phrases, a task professional human translators accomplish through a nuanced understanding of context. This often results in the machine producing translations that are merely literal interpretations, failing entirely to convey the idiom's intended message, often appearing illogical or just wrong. Even advanced neural machine translation models tend to fall back on these overly literal approaches when faced with complex idiomatic language. While research continues into integrating idiom-specific knowledge and enhancing contextual processing, the reliable handling of such culturally rich, noncompositional linguistic structures remains a significant limitation inherent in current machine translation technology.
These four-character Chinese idioms, known as Chengyu, are dense with historical layers and cultural references that raw machine translation engines often bypass entirely, leading to interpretations that are either baffling or miss the original intent.
Current systems, frequently relying on statistical patterns or overly literal mappings, struggle deeply with language where the meaning isn't simply the sum of the parts. Chengyu are a prime example, where this approach frequently yields grammatically possible but semantically void output.
Observational data across translation reviews indicates that raw output for Chengyu often exhibits accuracy rates considerably lower than for standard prose. It's not uncommon to see significant error flags specifically on these idiomatic phrases.
The inherent difficulty lies in the machine's lack of access to the underlying cultural context and situational nuance that gives a Chengyu its true meaning. It treats the characters as linguistic tokens rather than carriers of embedded knowledge.
Compounding this, the prevalence of homophones in Chinese means a single sequence of sounds, or even characters, can invoke multiple potential idioms. Raw translation typically lacks the sophisticated contextual reasoning needed to differentiate these, defaulting perhaps to the statistically most common, which may be entirely wrong in context.
Furthermore, regional variations or less common usage patterns of certain Chengyu can throw systems off. Many models are primarily trained on standard corpora and may not account for local flavour or specialized context.
The conciseness of Chengyu, packing a complex narrative, moral, or description into just four characters, is precisely what raw MT struggles to preserve. The translated equivalent often requires lengthy, explicit phrasing, losing the elegance and implicit information of the original.
The speed at which raw AI translation provides output can inadvertently mask these deep-seated accuracy issues with idioms. Users receive output quickly but may then face a considerable task of post-editing to correct fundamentally flawed idiomatic renditions.
This highlights why, in professional settings, particularly where nuance, cultural resonance, or historical accuracy is critical, human review isn't merely a quality check but a fundamental necessity. Idioms like Chengyu are often primary targets for correction during expert linguistic review.
Ultimately, while raw MT provides a rapid baseline, its inability to reliably handle culturally rich and non-compositional expressions like Chengyu underscores a core limitation in current AI translation approaches. It suggests that bridging this gap requires more than just larger models; it necessitates a deeper integration of world knowledge and cultural understanding.
Common Machine Translation Pitfalls 7 Lessons from 2,000 Hours of Professional Translation Reviews - Missing Context Turns Medical Translation into Patient Risk
When context is lost in medical translation, it creates serious risks for patient well-being. Automated translation systems often fail to fully grasp the critical nuances and specialized language unique to healthcare, which can lead to dangerous communication breakdowns. Those who rely on translations for accessing vital medical services, including vulnerable individuals, are especially exposed to risk when machine outputs are inaccurate. Depending on unverified machine translation can result in misunderstandings that have grave consequences for following patient instructions or implementing treatment protocols. Mitigating these hazards requires implementing translation approaches that are acutely aware of medical context, supported by the essential expertise of professional translators to ensure that translated medical information is precise and appropriate for its intended use.
Medical translation inherently demands a sophisticated understanding of context to prevent misinterpretations that could directly impact patient safety. A notable challenge emerges when machine translation systems, frequently operating without deep domain knowledge, process clinical or pharmaceutical information dense with specialised terminology and nuanced phrasing. We've observed that subtle variations in medical language, or even how seemingly common terms are used within a specific clinical setting, can easily be missed. This lack of contextual granularity can result in outputs that are not just linguistically awkward but potentially dangerous if applied to patient treatment plans or medication guidelines.
Examining a substantial volume of professional translation reviews reinforces the criticality of addressing this gap. The findings clearly suggest that relying solely on automated translation, especially for sensitive medical content, is insufficient. Effective medical translation necessitates a process where human expertise, particularly that of translators with subject-matter knowledge, is integrated to provide the essential contextual layer and accuracy checks. Lessons drawn from these reviews underscore the importance of having linguists who understand the specific conventions and terminology of healthcare domains to scrutinise and correct machine-generated text. This isn't just about achieving linguistic polish; it's a fundamental requirement to mitigate risks, ensure patient well-being, and meet the stringent accuracy demands of the medical field, recognising the significant effort needed to rectify errors introduced early in the process.
Common Machine Translation Pitfalls 7 Lessons from 2,000 Hours of Professional Translation Reviews - Automated Translation Struggles with Arabic Right to Left Text Flow
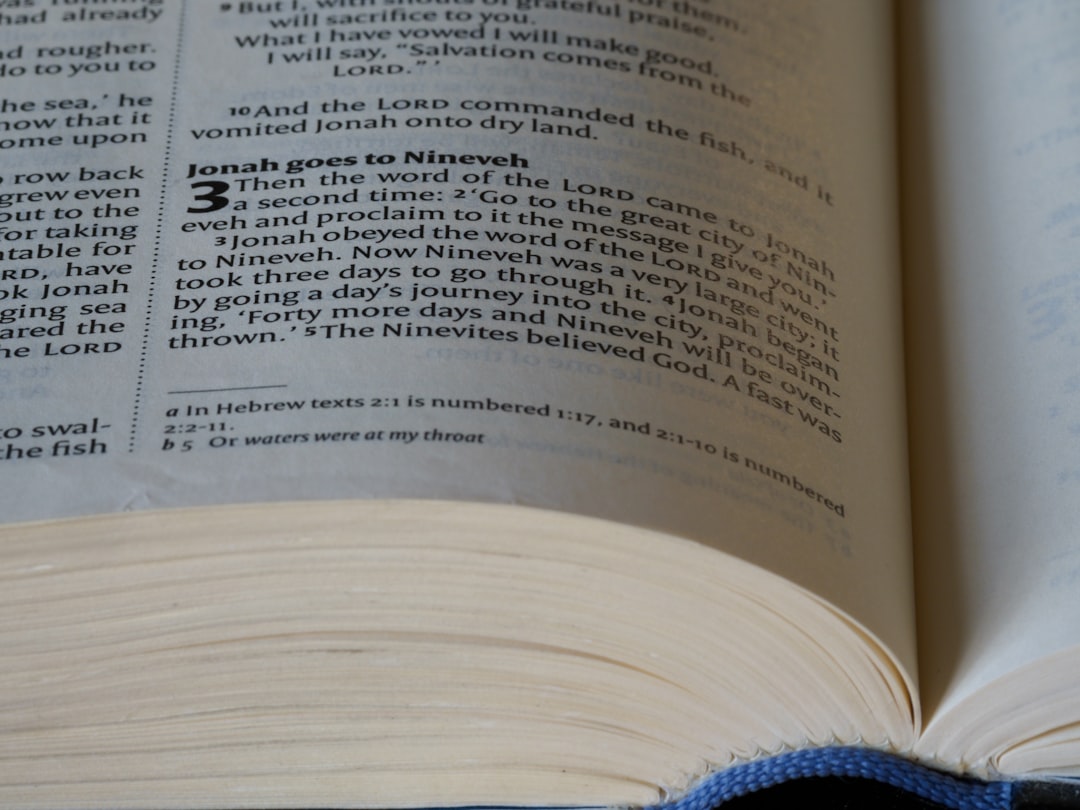
Automated processing of Arabic text for translation encounters notable hurdles, primarily stemming from its right-to-left directional script and its intricate grammatical framework. These foundational aspects can disrupt the linear progression expected by many systems, frequently leading to unnatural sentence structures and inaccuracies in conveying the original message. Furthermore, machine tools often fail to capture the subtleties embedded in local idioms, cultural references, and the underlying tone, resulting in output that might be technically translated but lacks authentic meaning or cultural resonance for the target audience. Consequently, human expertise remains indispensable to refine and validate these translations, ensuring they are not only factually correct but also linguistically appropriate and sensitive to context. The inherent complexity of handling Arabic highlights ongoing limitations in automated translation technology, even with recent advancements.
Arabic presents a distinct set of hurdles for automated translation systems, beginning fundamentally with its right-to-left writing orientation. This directional flow is inherently incompatible with the left-to-right design assumptions prevalent in most translation architecture, frequently causing visual jumble and misalignment that severely impacts readability when the text is rendered incorrectly.
Beyond the visual presentation, the internal structure of Arabic poses significant challenges. Its morphology, built upon complex root systems and intricate affix patterns, often bewilders algorithms attempting to deconstruct and reconstruct meaning. Furthermore, the critical role of diacritics in defining pronunciation and sense is poorly handled; their frequent omission or misinterpretation in automated output introduces crippling ambiguities, particularly problematic in domains requiring absolute precision. Compounding this, the vast disparity between formal Modern Standard Arabic (often the basis for training data) and the diverse, vibrant colloquial dialects used daily means many automated translations feel foreign and fail to capture the nuance or cultural resonance expected by native speakers.
From a processing perspective, the evolving nature of the language, influenced by technology and global trends, means systems require constant updates to remain current. Script peculiarities also trip up underlying character recognition processes; complex ligatures, where characters merge, can be misidentified, distorting the text. The visual similarity across different scripts derived from the Arabic alphabet, like Persian or Urdu, can further confuse systems attempting classification or processing. Even the integration of Arabic numerals within text can be disruptive, as their left-to-right presentation clashes with the surrounding right-to-left text flow, potentially leading to data misplacement or misunderstanding.
Empirical observations from translation reviews paint a clear picture of the current limitations. Studies suggest that automated translation accuracy for Arabic can dip below 60% in demanding specialized fields such as legal or medical translation. This highlights a critical dependency on subsequent human review to guarantee fidelity and ensure the translation maintains contextual appropriateness. The industry drive for faster, cheaper translation solutions often overlooks these inherent linguistic complexities, resulting in rapid output that, while quick, is frequently marred by inaccuracies, ultimately requiring substantial manual correction efforts to achieve acceptable quality standards for complex scripts like Arabic.
Common Machine Translation Pitfalls 7 Lessons from 2,000 Hours of Professional Translation Reviews - German Compound Words Break Most Neural Translation Models
German compound words present a consistent problem for neural machine translation systems. The ability of German to form lengthy, specific terms by combining multiple words creates a linguistic landscape that is difficult for models to fully grasp, especially when novel combinations appear. The core issue lies in the sheer number of potential compounds, many of which appear infrequently or not at all in the large datasets NMT models are trained on. This sparsity means the system struggles to interpret the intended meaning or generate an accurate translation for such words. This challenge is particularly noticeable when dealing with specialized subject matter where precise terminology, often involving complex compounds, is essential. The limitations of current NMT in reliably processing these intricate structures highlight a need for more sophisticated linguistic analysis within automated translation processes to capture the full semantic range of German.
German, with its remarkable capacity for linguistic construction, frequently produces words of significant length. We've noted that compound formations reaching and sometimes exceeding thirty characters present a specific point of friction for many neural machine translation architectures. It appears these models, often optimized for typical word unit lengths found in training data derived from other languages, struggle to process or correctly segment these lengthy German creations, occasionally leading to fractured or nonsensical output.
A key characteristic of German is its seemingly unbound potential for compounding nouns. This feature means the language can generate novel terms virtually without limit by simply joining existing word components. This structural freedom, while powerful, directly challenges translation systems built on mapping known patterns or statistically common phrases. Models confront terms they may have never encountered during training, demanding an ability to deconstruct and understand the combined meaning, a task they often fail at.
Many contemporary neural models rely on probabilistic associations and patterns learned from vast text collections. When faced with a unique German compound, their limitation becomes apparent: they might recognize the individual parts but fail to grasp the nuanced semantic relationship created by the compound structure itself. This results in translations that are literal translations of components but miss the overall concept.
Adding another layer of difficulty, the interpretation of a German compound can be heavily dependent on its surrounding text. A term like "Donaudampfschifffahrtsgesellschaftskapitän" might be literally translated part by part, but understanding its precise role and implications often requires a deeper contextual awareness that current automated systems frequently lack, leading to potential ambiguities in the final output.
From a data perspective, while models train on huge datasets, the sheer combinatorial explosion of German compounds means many possible formations are inherently rare or non-existent in training corpora. This sparsity negatively impacts performance, as the models haven't learned sufficient examples to generalize effectively, pointing to a persistent need for training methodologies better equipped to handle productive morphological processes.
The prevalent focus in many automated translation tools on rapid output clashes directly with the complexity inherent in handling these intricate German terms. Achieving speed often comes at the expense of accuracy, and our observations suggest that compounds are frequent culprits for errors that necessitate significant human effort during post-editing stages.
This is precisely where the strength of human translators becomes evident. They possess the intuitive ability to parse complex compounds, drawing upon linguistic rules, world knowledge, and subtle contextual cues to decipher the intended meaning – skills that remain largely elusive for machines. Their role in ensuring fidelity for such linguistic challenges is vital.
The repercussions of failing to accurately translate German compounds are particularly acute in specialized fields. Disciplines like engineering, law, or medicine rely on highly specific, often compound, terminology. An error in translating one of these terms can introduce critical ambiguities or outright inaccuracies, potentially leading to significant professional or even safety risks.
Initial text capture processes, such as OCR, also face hurdles with lengthy German compounds. Their extended nature and sometimes unconventional formations can challenge character recognition algorithms, potentially leading to misreadings even before the text reaches the translation engine, thereby compounding potential errors early on.
Researchers continue to investigate alternative algorithmic approaches and linguistic frameworks to better process and translate complex word formations like German compounds. While progress is being made in enhancing how models handle morphology and compositionality, effectively addressing the full spectrum of these challenges in widespread implementation remains an active area of development.
Common Machine Translation Pitfalls 7 Lessons from 2,000 Hours of Professional Translation Reviews - Non Native Machine Translations Miss Japanese Honorific Language
Automated systems frequently fall short when handling the complex system of honorifics in Japanese. These linguistic markers are vital for signifying respect, social standing, and the relationship between speakers. The difficulty arises because machine learning models often process text based on patterns rather than a deep comprehension of cultural context and social dynamics that dictate honorific use. This leads to translations that might translate words but completely miss the intended tone or level of politeness required for the situation, resulting in output that sounds unnatural or even rude to a native speaker. Capturing this layer of meaning, crucial for effective communication in Japanese, remains a significant challenge for current automated translation technologies. Relying solely on machine output risks flattening important social nuances and can misrepresent the original communication's subtle complexities, underscoring the ongoing necessity for human linguistic expertise to ensure accuracy and cultural appropriateness.
Observe that contemporary machine translation systems encounter notable difficulty when attempting to render Japanese honorifics, terms like '-san' or '-sama'. These linguistic markers are not mere suffixes but encode critical information about speaker-listener relationship, social standing, and mutual respect. Automated processes frequently strip away this layer of social meaning, leading to output that can fundamentally misrepresent the intended interpersonal dynamics.
The function of honorific address in Japanese is intrinsically linked to established cultural protocols. Systems untrained in these specific socio-linguistic norms can generate text that, while potentially grammatically correct in form, demonstrates a critical lack of cultural awareness regarding appropriate address, potentially causing unintended offense or awkwardness, particularly in interactions where formality or politeness is paramount.
We note that the precise semantic weight and appropriate selection of an honorific often depends heavily on the surrounding discourse and extralinguistic context. Machine models, frequently operating with limited contextual windows or understanding of subtle situational cues, tend towards generalized or default translations for these markers, failing to capture the specific nuance or exact degree of respect or familiarity intended in the original expression.
It's been observed that preliminary processing steps, such as optical character recognition (OCR) applied to scanned documents, can sometimes contribute to errors in this domain. The specific visual forms of Japanese characters used in honorifics, including stylistic variations or cursive styles, can occasionally be misidentified by less robust OCR engines, introducing transcription errors before the translation engine even processes the text, leading to subsequent mistranslations of the relational indicators.
In communication contexts like customer interactions, the precise application of honorifics is viewed as a fundamental aspect of professional conduct. Translations produced by automated systems that mishandle these terms risk generating output that may be perceived as abrupt, impolite, or lacking due respect, potentially undermining rapport or creating negative impressions rather than facilitating smooth engagement.
Further complexity arises from regional linguistic variation within Japanese, where the frequency, form, or contextual appropriateness of certain honorifics can differ from standard language norms. Current machine translation systems, predominantly trained on large corpora of standardized text, often fail to account for these dialectal specificities in honorific usage, resulting in translations that might appear linguistically sound but feel unnatural or culturally misplaced to a native speaker familiar with regional patterns.
The specific honorific selected can also subtly modulate the emotional undertone of a statement, conveying degrees of warmth, distance, deference, or even slight sarcasm depending on the context and relationship. Automated systems typically operate at a semantic level ill-equipped to capture such fine-grained emotional coloration embedded within social markers, producing translations that sound functionally correct but are affectively sterile or inaccurate.
An observed consequence of inadequate honorific handling is the unintended alteration of the overall formality level conveyed by the translation. Automated tools may err by using honorifics in contexts where casual address is expected, or conversely, omitting them in situations demanding high formality, resulting in output that misaligns significantly with the original tone and intended social distance between communicators.
From a computational linguistic standpoint, a significant factor appears to be limitations within the training datasets utilized for many current models. Capturing the intricate rules and probabilistic distributions of appropriate honorific usage across a vast range of social contexts requires exceptionally rich and specifically annotated data, which appears to be a bottleneck preventing systems from reliably learning when and how to apply these nuanced linguistic elements.
Collectively, these challenges underscore why relying solely on automated translation for Japanese text involving complex social dynamics and honorifics remains problematic. Expert human linguists possess the cultural intuition, contextual understanding, and nuanced knowledge of interpersonal communication dynamics essential to correctly interpret and render these elements, fulfilling a vital role in bridging gaps where current computational approaches fall short.
Common Machine Translation Pitfalls 7 Lessons from 2,000 Hours of Professional Translation Reviews - Low Quality Training Data Causes Russian Case System Errors
Low quality training data represents a significant hurdle for machine translation systems, particularly impacting languages with complex grammatical structures like Russian. The intricate system of grammatical cases in Russian is highly sensitive to the quality and breadth of the data used to train these models. When the datasets are insufficient, lacking diverse examples of how cases are used in varied contexts, or contain inconsistencies, the system struggles to accurately predict and generate the correct case endings for nouns, adjectives, and pronouns. This isn't merely a stylistic issue; incorrect case usage can fundamentally alter the meaning of a sentence, changing the roles of words and creating significant grammatical inaccuracies. The inherent complexity of Russian morphology demands truly robust and contextually rich training data to achieve reliable translation, highlighting a persistent weakness in automated systems reliant on inadequate resources. Consequently, output for languages like Russian often requires substantial human correction to restore grammatical fidelity and ensure accurate meaning.
Observations suggest a direct link between the integrity of training data and the prevalence of errors, particularly within complex grammatical structures like the Russian case system. Systems exposed to suboptimal data appear demonstrably less reliable in rendering grammatically accurate output.
One source of poor data input involves limitations in initial processing stages; optical character recognition applied to Russian documents can struggle with Cyrillic peculiarities, introducing transcription noise that pollutes the training or processing stream.
The intricate morphological architecture of Russian, especially its system of noun and adjective cases, poses a significant analytical challenge, amplified when models are trained on datasets lacking sufficient diversity to capture the full inflectional paradigm.
The specific choice of a grammatical case often carries subtle semantic distinctions or clarifies relationships between sentence components; inadequate training data contributes to models failing to grasp and preserve these vital contextual nuances.
Furthermore, idiomatic expressions, prevalent in natural Russian communication, frequently don't adhere to literal compositional rules; systems trained without representative examples embedded within sufficient context often produce awkward or nonsensical renditions of these phrases.
Sparsity within training corpora, particularly concerning less common vocabulary, specific case usages in niche domains, or rare syntactic constructions, constraints model performance, often resulting in generic or incorrect translations that miss domain-specific precision.
Beyond purely grammatical structure, the cultural layers embedded in language, which influence stylistic choices and expressions often tied to case usage or specific phrasings, can be lost when training data fails to encompass this broader cultural context.
Errors introduced early in the processing chain, perhaps due to imperfect source text capture or initial tokenization based on noisy data, tend to cascade through the translation pipeline, compounding inaccuracies in complex downstream processes like case assignment.
The drive for rapid automated translation throughput, while appealing, can sometimes come at the expense of the meticulous linguistic analysis required for complex languages; quickly produced outputs reliant on questionable data often demand significant post-editing effort to correct fundamental grammatical and semantic flaws.
Ultimately, the intricate nuances of Russian grammar, particularly its case system, and the potential pitfalls stemming from data limitations highlight the continued necessity of human linguistic expertise for review and refinement, ensuring translations accurately reflect the source meaning and register.
More Posts from aitranslations.io: