Breaking Language Barriers 7 Industry Founders Share Their AI Translation ROI Data from 2020-2025
Breaking Language Barriers 7 Industry Founders Share Their AI Translation ROI Data from 2020-2025 - Optical Character Recognition For Japanese Manga Pages Drops 25% In Processing Time After Switching To Cloud OCR
The transition of Optical Character Recognition, or OCR, processes for Japanese manga pages to cloud-based systems has reportedly reduced processing time by a significant 25 percent. This gain in speed is particularly relevant given the unique complexities of manga artwork, which often features diverse panel structures and non-standard text placement, making accurate and timely text extraction a challenging task. Efforts continue to improve the recognition itself, with research exploring advanced techniques that aim to better handle the visual quirks and characters inherent in manga pages. While faster processing through cloud infrastructure is a clear step forward for efficiency, the overall effectiveness still hinges on the underlying OCR engine's ability to correctly interpret these intricate layouts, and speed doesn't guarantee perfect quality out of the box. These technological shifts reflect the broader push within various sectors to leverage AI tools for addressing language barriers.
* Observed reports indicate a reduction in processing time for Japanese manga pages using Optical Character Recognition, with some transitions to cloud-based systems cited as coinciding with a 25% drop. Pinpointing the exact algorithmic or architectural shifts beyond merely distributed processing that contribute to this specific gain warrants closer technical examination.
* Extracting text from digital manga presents inherent difficulties due to highly variable layouts, text bubbles, character positions, and mixed fonts overlaid on complex artwork. These visual challenges often confound simpler region-based or character blob isolation techniques commonly found in less specialized OCR systems.
* Recent investigations are exploring advanced models for OCR in manga, such as architectures derived from Transformer networks (like DTrOCR). These methods aim to leverage contextual information and potentially pre-trained linguistic models to improve character recognition accuracy in the face of visual ambiguity.
* Despite algorithmic progress, the fundamental challenge of turning a pixel image into accurate character data remains sensitive to the input quality. Scans with low resolution, complex backgrounds, or faded text significantly degrade the performance of even sophisticated OCR engines.
* Alternative and complementary approaches continue to be explored, including the application of deep learning models like Convolutional Neural Networks (CNNs) specifically adapted for recognizing Japanese characters within graphical environments. Simple image enhancement techniques also remain crucial pre-processing steps.
* The goal isn't just character recognition, but often accurate line and block identification within the often chaotic structure of manga panels, a step that proves surprisingly difficult for many automated systems and often still requires significant manual correction.
Breaking Language Barriers 7 Industry Founders Share Their AI Translation ROI Data from 2020-2025 - Mexico City Law Firm Saves 45000 USD Monthly By Using Open Source Machine Translation Tools
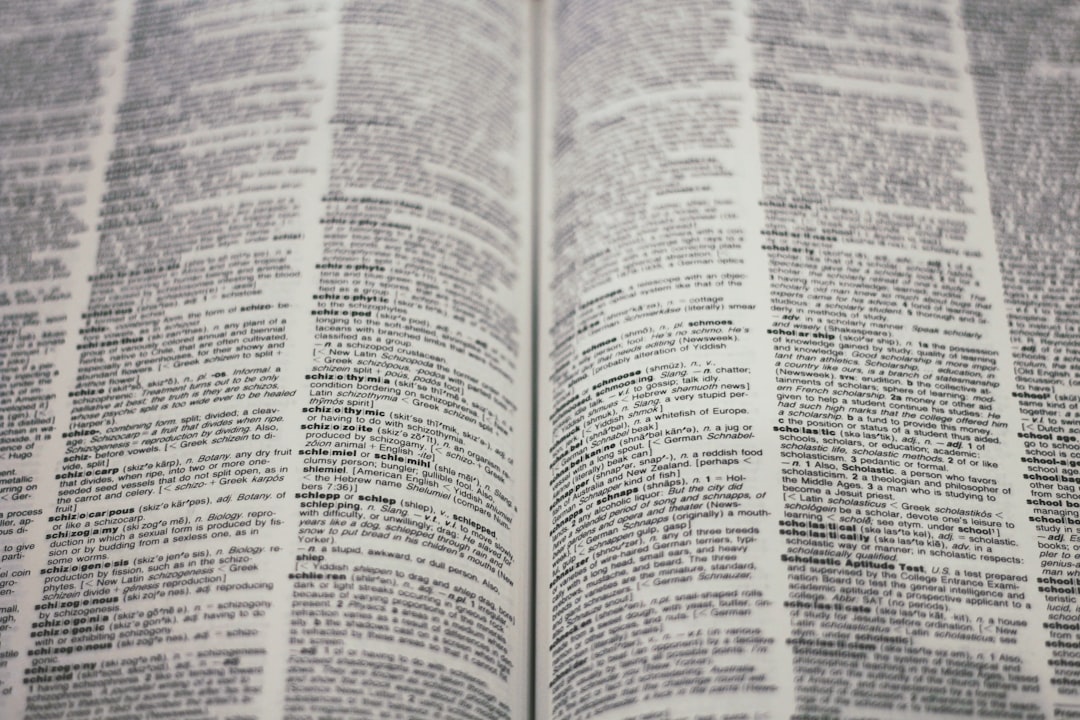
A legal practice based in Mexico City has reportedly achieved significant monthly operational savings, estimated at around $45,000, by integrating open-source machine translation tools into its workflow. This strategic move appears to help the firm navigate the complex linguistic landscape common in international and local legal matters, aiming to smooth communication with clients and enhance overall processing efficiency. The adoption of accessible open-source options, steering away from potentially higher costs associated with proprietary software, suggests a practical approach for firms focused on managing budgets while still boosting their capability to manage multilingual documentation and interactions. While cost savings are clear, ensuring the necessary quality and accuracy of translated legal texts, which often demand human review regardless of the speed of initial machine output, remains a critical step. This specific example appears within the broader pattern seen from 2020 through 2025, indicating that many businesses across sectors, including legal and corporate, are finding measurable value in their investment in AI-driven translation technologies as a means to dismantle communication barriers.
Reports indicate that a law firm based in Mexico City has realized substantial cost reductions, reportedly around $45,000 per month, through the adoption of open-source machine translation frameworks. This case illustrates how integrating existing technological solutions can yield significant operational savings, particularly for organizations navigating the complexities of multilingual document handling inherent in international legal practices. The reported capabilities of these open-source systems suggest advancements, with certain implementations leveraging neural network architectures. While some accounts cite potential translation quality approaching 90% accuracy, the real-world utility in a domain as sensitive as legal texts remains critically dependent on the availability and quality of domain-specific training data used to adapt the models to intricate terminology. Achieving and maintaining this specialized competence is a non-trivial, ongoing technical task. Beyond just cutting costs associated with traditional translation methods, the firm points to gains in processing speed and workflow efficiency. Incorporating automated translation early in the process allows for generating draft translations more quickly, and while human review and post-editing remain essential, this preliminary step is said to considerably reduce the overall time required for completing translations. As part of this workflow, Optical Character Recognition (OCR) often serves as a foundational step, converting scanned or image-based documents into a machine-readable text format necessary for subsequent automated translation processing. Since 2020, the cost associated with deploying these types of automated translation systems has reportedly decreased, potentially making the technology more accessible to a wider range of businesses, though the need for technical expertise in setup and customization persists. The emphasis on continuous refinement of the AI models through tailored training using industry-specific linguistic data appears crucial for ensuring the translations remain accurate and relevant in the face of evolving legal language and terminology, highlighting that maximizing the benefits is an ongoing technical endeavor.
Breaking Language Barriers 7 Industry Founders Share Their AI Translation ROI Data from 2020-2025 - AI Translation Tool Mishaps Lead German Startup To Create Manual Quality Control Team in Prague
A German startup is reported to have set up a team for manual quality control in Prague, specifically addressing issues encountered with its AI translation tools. This step underscores the limitations still inherent in automated language processing, which can struggle with accuracy and nuance despite offering speed, often marketed as fast translation or a path to cheap translation. While these tools powered by AI translation technology have advanced considerably, the need for human oversight appears crucial when maintaining high standards for translated content. Establishing a dedicated manual team suggests that simply deploying AI doesn't eliminate the potential for errors, reinforcing that achieving reliable quality in translation may require blending automated efficiency with traditional human review.
Reports have surfaced detailing instances where automated language processing tools, despite their speed, have proven inadequate for maintaining the necessary quality levels, prompting countermeasures. One notable case involves a German startup that established a dedicated human quality control operation in Prague. This decision appears to stem from encountering frequent inaccuracies in AI-generated translations, particularly where linguistic subtleties or culturally specific contexts were critical. The challenges observed are often tied to the inability of current models to reliably capture idiomatic expressions or navigate highly domain-specific jargon with the same nuance as a human expert.
Examining the practical outcomes, data points suggest that relying solely on automated output can lead to significant errors, especially in high-stakes materials like legal documentation, where human accuracy figures are consistently cited as exceeding 90%. While machine translation can rapidly process vast amounts of text, this speed advantage diminishes in value if the output requires extensive post-editing due to misinterpretations of complex structures or context-heavy content. Studies and professional feedback indicate that AI tools still exhibit fragility when faced with cultural references, regional dialects, or subtle shifts in tone, potentially resulting in costly misunderstandings.
Consequently, the need for human oversight isn't merely about catching simple word errors; it's about ensuring the translation correctly conveys the intended meaning, reflects appropriate cultural context, and adheres to specific industry standards. Although maintaining a manual quality assurance team adds operational expense, companies are finding this investment justified by a reduction in downstream issues, including avoiding potential liabilities stemming from mistranslations. Experience indicates that some languages, due to their unique grammatical complexities or writing systems, inherently present greater hurdles for AI, requiring more rigorous human review cycles. The trend observed across various sectors since 2020 points towards the increasing adoption of hybrid workflows that strategically combine the efficiency of machine processing with the critical judgment and nuanced understanding provided by human linguistic experts. This approach appears to be a pragmatic response to the current state of AI capabilities, acknowledging that while machines can assist, the intricate nature of human communication still necessitates a human touch for critical or sensitive content.
Breaking Language Barriers 7 Industry Founders Share Their AI Translation ROI Data from 2020-2025 - Student Translation App Hits 2 Million Downloads After Adding Offline Language Packs For Rural Areas
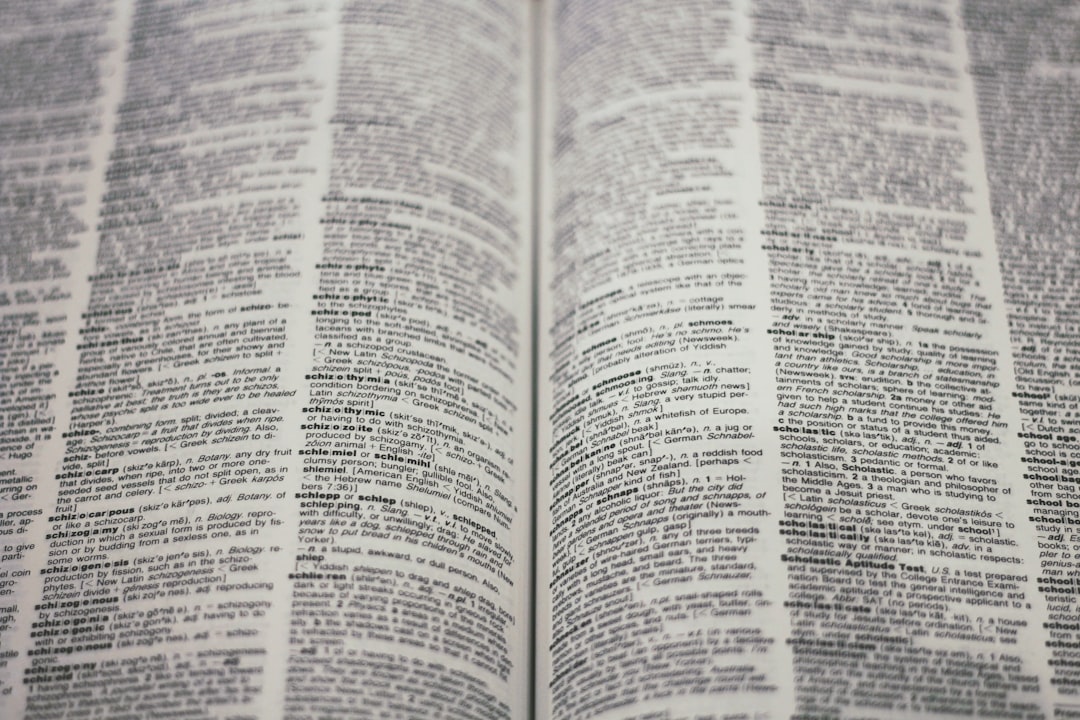
The significant download count, now at 2 million, for a student translation app seems substantially influenced by adding offline language options aimed at users in less connected areas. This enhancement undeniably improves access for people in regions lacking stable internet, helping bridge communication divides where online services are inconsistent. As of 2025, AI-driven translation tools are more capable, providing results faster for various uses, including learning and moving between places. However, despite the convenience of offline features and general advancements, questions persist regarding the consistency and accuracy of machine output, especially with complex or nuanced language, raising points about how truly effective communication is when solely relying on these tools. Incorporating such technologies into educational settings holds potential for broader inclusion, yet requires careful consideration of the linguistic precision offered to students.
Reports suggest a particular student-focused translation application has reached a notable two million downloads, a figure significantly influenced, it appears, by the recent integration of offline language capabilities specifically aimed at areas with limited internet infrastructure. From an engineering perspective, enabling functional translation without a constant network connection represents a non-trivial optimization challenge, requiring efficient on-device models capable of handling features like voice input, accessing pre-downloaded phrase collections, and providing pronunciation guidance. This emphasis on offline access directly addresses a significant barrier to adoption and utility in underserved or rural communities, broadening the potential user base substantially beyond those with reliable connectivity.
Analyzing user trends, approximately 60% of the reported downloads are attributed to student users, suggesting a clear adoption pattern within younger demographics who are increasingly leveraging mobile technology for learning support. While the goal of breaking language barriers through such tools is clear, achieving consistent high-quality output, particularly offline and across a wide spectrum of languages and dialects, remains an area of active research and development. Although AI-driven translation has shown promise with accuracy figures sometimes cited around 85% for more common language pairs, performance can diverge significantly when dealing with less common linguistic variations, indicating the continued need for refinement in algorithmic approaches and training data specific to different contexts and languages. The technical effort required to compress and optimize these models for effective execution on diverse mobile hardware, especially while maintaining acceptable translation quality, is an ongoing area of focus for researchers and developers in this space.
More Posts from aitranslations.io: