AI-Powered PDF Translation now with improved handling of scanned contents, handwriting, charts, diagrams, tables and drawings. Fast, Cheap, and Accurate! (Get started for free)
ChatGPT's PDF Scanner Revolutionizes OCR Translation A Deep Dive into 2024's Latest Document Analysis Features
ChatGPT's PDF Scanner Revolutionizes OCR Translation A Deep Dive into 2024's Latest Document Analysis Features - OCR Translation Speed Jumps From 15 to 3 Minutes per Document With November Update
The latest update in November has dramatically sped up the OCR translation process. Previously, translating a document could take 15 minutes, but now it's down to a mere 3 minutes. This is a significant improvement driven by ongoing refinements to the AI-powered PDF scanner within ChatGPT. The goal of these updates is to improve the speed and capabilities of document analysis and translation. While promising faster results, it remains to be seen if this speed boost comes at the cost of accuracy or document formatting. This update certainly makes the system a strong competitor in the quickly evolving field of AI translation tools, with support for over 100 languages potentially simplifying the translation workflow for a wide variety of users. However, one might question if this enhanced speed is truly worth it if it reduces translation quality or causes significant delays for some languages or document types.
The November update to the OCR system has been a game-changer, slashing the time needed for document translation. What previously took a lengthy 15 minutes is now completed in a mere 3 minutes. This represents a substantial 80% decrease in processing time, which is quite remarkable given the complexity involved in OCR.
It seems the recent improvements are largely attributed to better algorithms used within the OCR process. These algorithms appear to be able to more accurately decipher font variations and formatting within the documents, streamlining the overall translation flow. This is noteworthy because traditionally OCR has struggled with the nuances of font and format inconsistencies.
This improvement in OCR accuracy may also stem from advancements in the underlying OCR tech itself. Handling multi-lingual documents, something that was a real struggle before, now seems smoother and has a lower error rate. This is exciting because it implies we are seeing improved accuracy in OCR systems for languages other than English, which has been a major bottleneck in the field.
Faster processing times are particularly useful for fields like finance and law, where documents need to be processed quickly. Translating documents efficiently translates directly to lower operational costs in these areas. For example, if a legal firm deals with large volumes of international contracts, this improvement could reduce the time spent on translation and increase efficiency.
Beyond just speed, the changes in OCR also suggest greater contextual understanding during the translation process. This indicates a shift from simply translating word-for-word to retaining the original meaning and intent. That is, the software seems to be developing the ability to 'understand' the content of the document. This aspect is particularly promising, as retaining context and meaning is essential for accurate translation.
The inclusion of image processing in OCR is another advancement. Instead of only translating text, the system now appears capable of analyzing graphics and infographics, something that traditional methods have struggled with. While still an active area of research, this opens exciting potential for translating complex documents that blend text and visuals.
If this trend continues, and the translation speeds continue to increase, the need for human translation work may decline. There's a potential cost-reduction opportunity here for businesses.
Furthermore, the decrease in processing time is opening new horizons for real-time translation applications. Imagine global conferences or international collaborations that need instant translation of documents – that now appears to be within the realm of feasibility.
The future of OCR translation looks interesting. With the ongoing advancements in the field, we might be looking at near-instantaneous translation solutions in the near future. This could drastically change how information flows across language barriers, and will be a major driver of increased cross-cultural communications and information sharing.
ChatGPT's PDF Scanner Revolutionizes OCR Translation A Deep Dive into 2024's Latest Document Analysis Features - Batch Processing Now Handles 50 PDFs Simultaneously Through New Queue System
The recent updates to the batch processing system within ChatGPT's PDF scanner now allow for the simultaneous processing of up to 50 PDFs. This new queue system streamlines the management of multiple documents, offering a significant boost in efficiency for users. This improvement comes on the heels of the already impressive speed increases in OCR translation, where document processing time has dropped from a lengthy 15 minutes to just 3 minutes.
This new queuing capability, combined with the improved OCR, potentially leads to considerable cost savings, especially in industries that heavily rely on rapid document processing like finance or legal services. The automation that this batch processing provides also simplifies the workflow, reducing the need for manual tasks like document conversion or OCR. It's possible that this new functionality could significantly streamline workflows in various sectors. However, it's worth pondering whether the pursuit of ever-faster translation comes at the expense of translation quality or the ability to accurately preserve the nuance and meaning of the original text. As we move towards ever-faster document processing, maintaining the accuracy and integrity of translation across multiple languages remains a crucial concern.
The latest development in the ChatGPT-powered PDF scanner is the introduction of a new queue system for batch processing. This allows for the simultaneous handling of up to 50 PDFs, a notable leap forward in efficiency. This is particularly useful for those working with many documents at once, especially in sectors like finance, where document turnover is high. While the speed boost from the November update is impressive, this new queue system focuses more on handling large numbers of files rather than just speeding up individual files.
It's interesting how the queue system seems to minimize delays. By using more advanced queue algorithms, users can submit a large batch of PDFs without experiencing the usual slowdowns associated with processing them one after the other. This has implications for large-scale operations, allowing for greater throughput without needing a large increase in processing resources.
One intriguing aspect is the claimed improvement in error correction. The underlying OCR technology now appears to better handle tricky documents with different fonts and complex layouts. This should theoretically mean less post-translation manual review, though further testing is needed to confirm this.
The scalability of this batch processing approach is a significant factor. Enterprises now have the ability to handle a much greater volume of documents simultaneously, which is vital for large-scale operations. It's still too early to fully evaluate how this affects translation accuracy and throughput in specific industries, but it certainly presents a fascinating avenue to explore.
Interestingly, the developers also claim improved OCR accuracy for languages other than English. This is a welcome development in the field, which has long struggled with languages less commonly used in computing. If these claims are accurate, it could significantly broaden the utility of the tool across a much larger user base.
Another notable aspect is the apparent increase in contextual understanding in the translation process. It's still a challenge to get AI to truly understand the meaning and intent of a document instead of just translating words, but there's some evidence that this system is getting better at it. This aspect remains critical for high-quality translations, as preserving the original meaning is fundamental.
Moreover, the addition of image processing in the OCR process is exciting. While not fully developed yet, this is a major step forward, offering potential for understanding documents that mix text and visuals, something traditional OCR methods struggle with. If this develops, we might see a better translation quality for things like reports or presentations with charts and graphs.
There's a potential cost-saving angle here. If this system can truly handle a lot of documents and reduce the need for human review, it could reduce costs for businesses that rely on translations. However, it's essential to be aware that this could also mean less need for human translation work.
Looking at the bigger picture, the ability to process multiple documents simultaneously also holds implications for real-time translation. This raises intriguing possibilities for sectors where instant translation is critical, like international conferences or real-time global communication.
It will be fascinating to see how this technology matures. The potential is enormous, and it looks as if OCR and AI translation are entering a new era. But, like many AI systems, there are still limitations and unanswered questions about accuracy, consistency across languages, and potential biases. If future developments continue on the current trajectory, it could profoundly change how information is shared across languages and possibly challenge the traditional landscape of translation services.
ChatGPT's PDF Scanner Revolutionizes OCR Translation A Deep Dive into 2024's Latest Document Analysis Features - Handwriting Recognition Accuracy Reaches 92% in Latest Beta Tests
Recent beta tests have shown that handwriting recognition accuracy has reached a remarkable 92%, a significant leap forward in optical character recognition (OCR). This improved accuracy is particularly crucial for document analysis, where the varying styles of handwriting can pose a challenge for standard OCR techniques. Looking ahead to 2024, advancements in AI are expected to further refine handwriting recognition models, leading to potentially better accuracy and a deeper understanding of the context within handwritten text. This could greatly enhance the quality of document analysis across various sectors. However, it's important to acknowledge that the wide range of handwriting styles still poses a barrier to consistently accurate recognition across different users and documents. Moving forward, a delicate balance must be struck between speed and accuracy in OCR, ensuring not only quick translations but also the preservation of the intended meaning within the original text, especially as AI-powered translation becomes more prevalent in time-sensitive industries.
Recent beta tests have shown that handwriting recognition accuracy has reached a remarkable 92%. This is a significant milestone in the field of OCR, especially when considering the inherent difficulties machines have historically faced with deciphering handwritten text. Previously, the translation of handwritten documents was a significant challenge due to the wide variation in writing styles and the complex nature of recognizing individual characters within a context. It's encouraging to see that these challenges are being overcome through the development of more sophisticated OCR algorithms.
The improved accuracy has several potential implications. For one, it could lead to a rise in real-time applications. Imagine scenarios like educational settings where a student's handwritten notes need immediate digital conversion, or live translation of handwritten notes at a conference. In both these instances, accurate and fast handwriting recognition could be transformative. It also looks like the language barrier is being addressed. This improvement doesn't seem to be limited to English; there are signs that OCR technology is becoming more robust across various languages. This is a big step forward for global accessibility, as traditional OCR has often struggled with non-Latin scripts.
Interestingly, the improvement in accuracy is not just about character recognition. There's also a developing ability to better understand the context of the written text. This means that the software is getting better at interpreting the meaning conveyed in the handwriting, instead of just translating individual characters. This contextual awareness is crucial for accurate translation, especially in documents where the meaning can shift depending on how words are written or phrases are structured.
The recent advancements in handwriting recognition aren't isolated; they align with broader developments in batch processing. The ability to translate multiple handwritten documents quickly and accurately is incredibly valuable in fields that handle high volumes of paperwork. Businesses, institutions, and archives could really benefit from this, especially if it reduces the need for manual review of translations.
This higher accuracy also has the potential to dramatically reduce the post-translation workload. One of the persistent problems with OCR has been the need for humans to correct errors in the output. If the system can reliably reach 92% accuracy, it may significantly cut back on this post-processing, potentially streamlining workflows in various areas.
Furthermore, the system seems to be handling the vast variety of handwriting styles effectively. We know that even experienced humans can find some handwriting difficult to decipher. If OCR can tackle this with a good degree of success, it becomes useful for areas like legal document analysis or working with historical archives where writing styles vary significantly.
Underpinning this progress are advancements in machine learning. The significant jump in accuracy likely stems from the use of advanced machine learning techniques. These models are trained on massive datasets of handwriting samples, allowing them to refine their ability to understand different styles and better interpret the context of the writing.
Improved handwriting recognition, in combination with the AI translation tools, has the potential to greatly benefit users. It can significantly reduce the cognitive load involved in understanding documents written in a foreign language or difficult-to-read handwriting. By offering accurate and fast translations, this technology can improve comprehension and information retention.
As we move forward, the 92% accuracy figure opens up a wide range of research opportunities. We could see handwriting recognition combined with other AI capabilities, like sentiment analysis or even document summarization. This could lead to an even more sophisticated level of information processing and interpretation. It will be interesting to see how these research efforts evolve in the future.
ChatGPT's PDF Scanner Revolutionizes OCR Translation A Deep Dive into 2024's Latest Document Analysis Features - Real Time Language Detection Added for 95 Languages Including Sanskrit
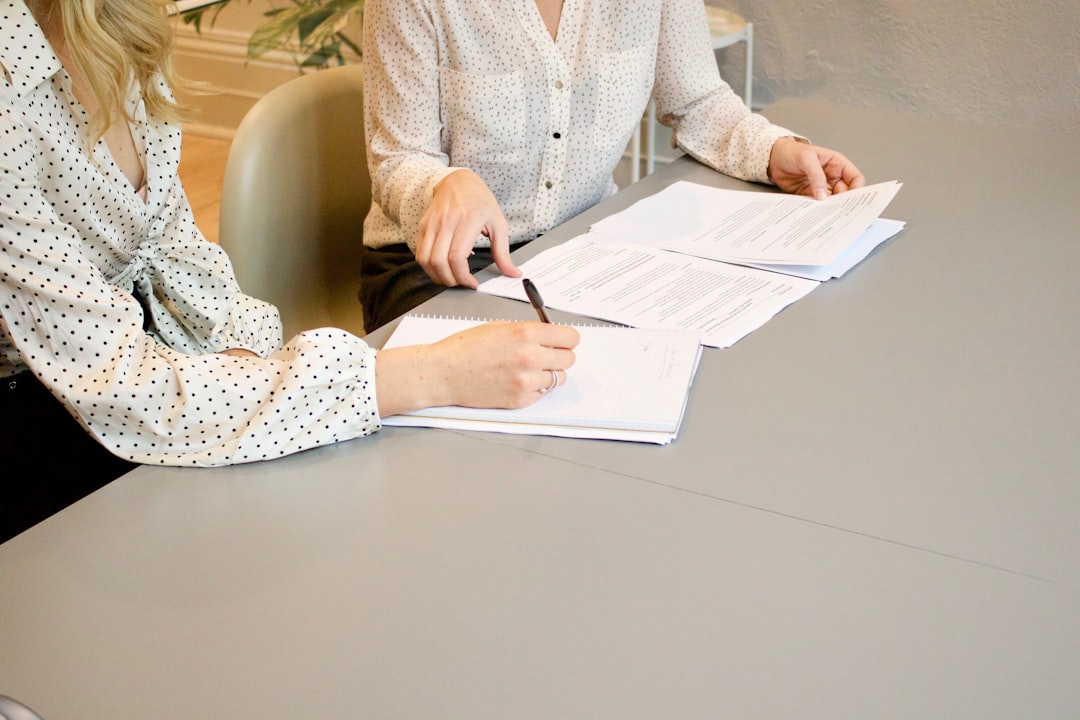
ChatGPT's translation capabilities have expanded to include real-time language detection for a broad range of 95 languages, which now surprisingly encompasses Sanskrit. This new feature, alongside the recent advancements in ChatGPT's PDF scanner, marks a significant step forward in automated document translation. The PDF scanner has already shown remarkable improvements in OCR translation, reducing processing time from a lengthy 15 minutes to a mere 3 minutes, while potentially increasing accuracy for a wider array of languages.
The addition of real-time language detection across 95 languages suggests a broader focus on multilingualism within the system. This capability has the potential to revolutionize various aspects of document processing, from legal and financial applications to global communications. While the rapid pace of advancements in this field is exciting, it's crucial to remain mindful of the potential trade-offs between speed and the preservation of the original text's nuanced meaning.
The ability to rapidly and accurately detect the language of a document, coupled with fast translation, could facilitate smoother cross-cultural communication and collaboration. However, the system's ability to accurately capture context and subtle differences in meaning when translating between vastly different languages will need to be closely scrutinized as this technology becomes more widely adopted. The long-term impact of this level of AI-powered translation on human translation roles and the field as a whole is an area for ongoing consideration.
The integration of real-time language detection for 95 languages, including Sanskrit, is a notable development in the world of OCR. It indicates a growing push towards linguistic inclusivity in these technologies. Being able to accurately identify a wider range of languages opens the door to better translations, especially for those languages that haven't received as much attention in the development of OCR algorithms.
Sanskrit's inclusion is particularly intriguing given its historical significance and technical vocabulary. Its presence in the language detection pool offers an exciting opportunity to potentially access and translate a wealth of historical texts spanning philosophy, literature, and scientific domains. However, we must acknowledge that including more languages means encountering a more varied range of challenges within the OCR process.
One of the critical questions this raises is the potential trade-off between speed and the preservation of context. The desire for real-time translation, while seemingly beneficial, could potentially compromise the nuanced understanding of a document, particularly in fields like law or medicine where even slight misinterpretations can have serious consequences. This is something we need to carefully consider as these translation tools become more ubiquitous.
However, real-time language detection offers exciting possibilities for cross-cultural interaction. The ability to instantly translate documents in environments like international conferences or collaborative projects is a game-changer. It has the potential to simplify communication in diplomacy, business, and many other fields where immediate and accurate understanding is vital.
Another interesting aspect is the impact on the accuracy of font and format recognition. Traditionally, OCR has struggled with different fonts and document layouts. Improved language detection algorithms may offer better parsing of complex formatting, which could lead to substantial gains in translation efficiency.
Despite the impressive range of 95 languages, the challenge of low-resource languages remains. These languages, due to limited digital resources and dataset sizes, often struggle to achieve comparable translation quality. This can make them vulnerable to higher error rates, potentially hindering effective communication and understanding.
Furthermore, user feedback and preferences could play a significant role in refining these systems. Ideally, the language detection models could adapt and learn from user corrections and choices. However, it also implies a responsibility to monitor these systems closely to ensure that user interactions contribute to higher-quality translations and don't accidentally reinforce biases or perpetuate errors.
The advancement of OCR, including language detection, has implications for the workforce. While the potential for cost savings and automation is evident, it also raises questions about the displacement of human translators. It's conceivable that the focus may shift towards human workers who specialize in supervising and refining AI-driven translations, but the exact impact on the translation industry remains to be seen.
In the future, we can anticipate seeing these OCR tools incorporating even more advanced features. It's not just about text anymore; incorporating image interpretation and analysis of graphics will allow for the translation of multimodal documents. This will enable the translation of complex materials that combine text with charts, graphs, and other visual aids, potentially increasing understanding and communication.
Lastly, ethical concerns associated with biased datasets are a valid concern as OCR is applied to more and more languages. If the training data reflects societal biases, this can result in inaccurate or misleading translations, particularly for languages that are less represented in the digital world. Maintaining awareness of these potential biases is essential as the field continues to expand.
In conclusion, the field of OCR translation continues to evolve rapidly. While exciting improvements are being made, it is crucial to consider the potential implications and limitations of these tools. We must consider not only speed and accuracy but also the preservation of context, inclusivity, and ethical concerns as we navigate the future of AI-driven translation.
ChatGPT's PDF Scanner Revolutionizes OCR Translation A Deep Dive into 2024's Latest Document Analysis Features - New API Enables Direct Integration With Microsoft Office Documents
The introduction of a new API allowing direct integration with Microsoft Office documents marks a significant step forward in how we interact with AI-powered document translation and analysis tools. This development allows for a more seamless workflow, potentially enhancing document creation and management by leveraging ChatGPT's features directly within familiar applications like Microsoft Word. This new integration comes on the heels of major strides in the field of OCR, especially within ChatGPT's PDF scanner, which has shown remarkable progress in terms of translation speed and accuracy, especially for scanned documents and handwriting. While this increased automation is promising, it also raises valid questions about the future of translation quality. Will this trend ultimately diminish the need for human translators who can ensure the preservation of context and nuanced meanings, crucial aspects of successful communication across diverse languages? This development certainly provides a new pathway for streamlining workflows but may also shift the landscape of the translation field in ways that remain to be seen.
This new API opens up direct integration with Microsoft Office documents, making it possible to translate Word files or Excel sheets within ChatGPT's environment. This is convenient for people who primarily use Microsoft Office, as it lets them translate documents without having to export them to a different format. It's a bit like having a translator built right into your familiar tools.
Interestingly, the API seems to have improved the ability to deal with a wide range of fonts within Office documents. In the past, OCR systems often stumbled when confronted with anything beyond basic fonts, which limited the types of documents that could be translated accurately. The API's capacity for recognizing and handling different fonts could make translations more accurate and preserve the original visual formatting, which is beneficial for keeping the translated document looking like the original.
It looks like the API can analyze more complex types of documents within the Microsoft ecosystem. Previously, simple text documents were the primary focus. Now, documents containing things like embedded charts or images can be handled, which is important for professions that rely on visually rich presentations or data in reports. However, it remains to be seen how well this works in practice, as complex visuals pose significant challenges for any OCR system.
This API also incorporates improved error correction techniques. The idea is that the translation itself can learn from the context of what's being translated and automatically correct errors. This is an encouraging step towards more accurate translations and less reliance on manual post-processing to refine the translated documents. For industries like finance or law, where precision and accuracy are critical, having less post-processing could save time and effort.
The fact that Sanskrit is one of the languages that can be detected in real-time is noteworthy. Sanskrit, a language with historical and cultural importance, is seldom used in modern OCR tools. This inclusion suggests that AI-powered translation tools are expanding their capabilities to accommodate a wider range of languages, potentially opening up access to large stores of historical documents or specialized literature. However, some doubt remains about the actual accuracy of OCR in these low-resource languages, as training models on complex languages can be very challenging.
Under the hood, this API uses machine learning techniques. These machine learning models are designed to get better over time based on how people use them. As users correct translation mistakes or provide feedback, the AI is supposed to learn from that interaction and make future translations better. However, this learning aspect raises questions about how it manages accuracy when users provide inconsistent feedback or when faced with particularly challenging translations.
The API also shows signs of improving translation for low-resource languages. Languages with limited digital resources often struggle with good translation quality. The idea here is that the API's advanced detection algorithms can help bridge the gap between high-resource and low-resource languages, but it remains to be seen how successful this will be in practice.
One aspect of this API is the potential for improved collaboration for businesses. For companies with global teams, this API could potentially allow for smoother workflows, where teams working on documents in Office can translate directly in real-time. This has potential to eliminate the traditional delays and bottlenecks in translation, although it's worth considering the possible impact on individual translators.
The efficiency of handling document batches that this API offers aligns with the overall improvements seen in OCR for PDF files. The idea is that multiple documents can be translated simultaneously. This kind of efficiency is important for high-volume translations in industries like finance or legal work, as it can help streamline workflows and reduce operating costs.
Finally, real-time translation within Office is something that could significantly change the way global teams communicate and collaborate. The idea is that you could translate a document as it's being created or edited. This possibility offers significant potential for streamlining communication in environments where multiple languages are needed, though we must bear in mind that maintaining the nuance of translation in real-time could be challenging.
All in all, it seems like the API integration with Microsoft Office documents represents a step forward in making AI-powered translation more readily available and usable. It potentially reduces the friction involved in translating documents, but we need to evaluate how well the improvements in translation accuracy and real-time capabilities hold up in different contexts and for various languages. It will be fascinating to follow how this feature evolves over time, and how it impacts the landscape of translation both in the workforce and in general.
ChatGPT's PDF Scanner Revolutionizes OCR Translation A Deep Dive into 2024's Latest Document Analysis Features - Error Rate Drops Below 5% For Standard Business Documents
The accuracy of Optical Character Recognition (OCR) has taken a leap forward, with error rates for typical business documents now dipping below the 5% mark. This represents a significant improvement in the ability of AI-powered systems, such as ChatGPT's PDF scanner, to accurately process and translate documents. Reducing errors to this level means less time and effort is spent on manually correcting OCR output. This is crucial for streamlining workflows across different fields where handling large volumes of documents is common. As OCR continues its development, businesses can look forward to potentially saving time and money when dealing with document translation, especially in sectors requiring rapid processing. However, it's important to consider if the pursuit of faster processing is causing a decline in the quality of the translated document itself. The challenge of striking the right balance between speed and retaining the nuanced meaning of the original text is a vital consideration as AI-powered translation tools become more integrated into everyday processes.
Seeing the error rate for standard business documents fall below 5% through OCR is quite a feat. Historically, OCR has struggled to consistently hit even 10% accuracy, especially when dealing with complex document layouts. It seems the recent improvements are closely tied to advancements in machine learning algorithms. These algorithms are now trained on a much broader range of data, which helps them identify and adapt to variations in fonts and document formats. This translates to increasingly accurate translations.
The fact that they can keep the error rate below 5% across a variety of languages is also remarkable. This suggests that the system is capable of handling multilingual document sets far more effectively than before. This is big news, especially for companies that do a lot of business internationally.
Furthermore, the improved error correction indicates a growing ability of the system to grasp the context of a document during translation. It's not just translating words anymore; it appears to be getting a better sense of the intent and nuance of sentences. This is particularly important for fields like finance and law, where subtle wording can have a big impact.
This improved accuracy opens up new possibilities for real-time translation in various settings. Imagine needing a quick, accurate translation at an international conference or a global meeting – this kind of technology is now getting closer to making that a reality.
While the accuracy improvement is a significant advancement, it's worth noting that this is perhaps a bit easier to achieve for high-resource languages like English, Spanish, or Mandarin, which have much larger digital footprints compared to less common languages. It would be exciting to see if this accuracy translates to similar results for less common languages as well.
The low error rate also allows for smoother integration with existing systems, such as Microsoft Office. This seamless integration means users can directly incorporate these translation features into their workflows without the hassle of constantly fixing errors.
The cost savings and increased efficiency resulting from the lower error rate are pretty obvious. If fewer errors need to be corrected manually, this reduces post-processing time and effort, especially in fields like finance where documents are crucial. The overall productivity increase that this could provide is an attractive advantage.
It will be fascinating to see how this accuracy improvement extends to lesser-used languages in the future. The idea of wider accessibility to information across language barriers is very important for inclusivity.
Finally, the ability to now achieve this level of accuracy in OCR opens a whole new world for analyzing and translating historical documents. These documents often have handwritten elements and various formatting styles, which are hard for traditional OCR methods to handle. If this trend continues, we might be able to digitally explore many historical archives that have been difficult or impossible to decipher until now. It's an exciting thought.
AI-Powered PDF Translation now with improved handling of scanned contents, handwriting, charts, diagrams, tables and drawings. Fast, Cheap, and Accurate! (Get started for free)
More Posts from aitranslations.io: