AI-Powered PDF Translation now with improved handling of scanned contents, handwriting, charts, diagrams, tables and drawings. Fast, Cheap, and Accurate! (Get started for free)
How OpenAI's PDF-to-Text Pipeline Revolutionizes Document Translation in 2025
How OpenAI's PDF-to-Text Pipeline Revolutionizes Document Translation in 2025 - PDF Text Recognition Reaches 8% Accuracy Through OpenAI's Neural Network Processing
As of 2025, insights into OpenAI's work on PDF text recognition through neural network processing indicate accuracy reaching a figure of 8%. This focus on neural networks aligns with broader trends in developing more robust Optical Character Recognition (OCR) systems capable of handling complex documents. However, a reported accuracy level of 8% underscores the considerable difficulties that persist in reliably extracting text from the diverse and often challenging structures within PDF files. While deep learning approaches are key to tackling issues like image distortions or varied formatting, this figure highlights that achieving the high precision necessary for dependable automated document translation remains an area requiring significant further development. The gap between current capabilities and the demands of efficient text extraction for translation workflows remains apparent.
The stated 8% accuracy marker for text recognition within PDFs reportedly signifies an advance, particularly for documents featuring intricate layouts like multi-column structures or tables, where older OCR techniques often struggled, potentially yielding less than 5% fidelity. The claim here is improved interpretability not just of characters, but also of the spatial arrangement.
By leveraging deep learning methodologies, the system is said to gain the capacity to handle handwritten elements embedded within scanned documents – a type of content that historically proved challenging for conventional OCR systems. This functionality potentially extends the reach of automated translation to sources previously inaccessible, such as archival materials or personal notes.
The pipeline is described as utilizing transfer learning, which ostensibly facilitates faster adaptation to different languages and varied document formats. The suggestion is that retraining for a new linguistic set requires a comparatively smaller volume of data than building a model from the ground up would necessitate.
Performance metrics cited point to a processing speed up to 100 pages per minute. This claimed throughput represents a substantial acceleration compared to earlier OCR methods, which might have taken several minutes to process a similar volume, positioning speed as a key factor for high-volume document workflows.
Attention mechanisms within the neural network are employed, designed to enable the system to prioritize actual textual content and filter out distracting visual noise like watermarks or graphical elements. This selective focus is purported to result in cleaner input for subsequent translation stages, potentially enhancing output quality.
The system is reported to exhibit improved robustness when encountering documents containing multiple languages mixed within the text, an area often problematic for prior generation models. This characteristic is highlighted as particularly relevant for processing international agreements, business reports, or other documents frequently exhibiting linguistic diversity.
An iterative learning loop is reportedly incorporated, allowing the neural network to refine its recognition accuracy based on feedback or corrections provided during use. This adaptive feature suggests a potential for the system's performance to evolve and improve over time with practical deployment.
The technology is described as being capable of analyzing and interpreting the structural elements inherent in PDF documents, such as footnotes, citations, or headers. Preserving the context provided by these structures is presented as crucial for maintaining precision in translations of academic papers, legal documents, or other content where explicit referencing is vital.
The architecture reportedly adopts a hybrid methodology, combining established rule-based logic with machine learning components. This dual approach is proposed as a way to enhance overall accuracy by providing greater flexibility in handling document formatting exceptions and irregularities that might trip up a purely machine learning or rule-based system.
Despite the specified 8% recognition rate for these complex tasks, the ongoing progression in neural network design and training techniques raises the prospect of future iterations achieving significantly higher, potentially near-human-level, text recognition capabilities. Such potential advancements prompt consideration of the practical limits and feasibility of fully automating document translation in certain challenging domains.
How OpenAI's PDF-to-Text Pipeline Revolutionizes Document Translation in 2025 - Translation Speed Jumps to 450 Pages Per Hour Due to Advanced GPU Processing
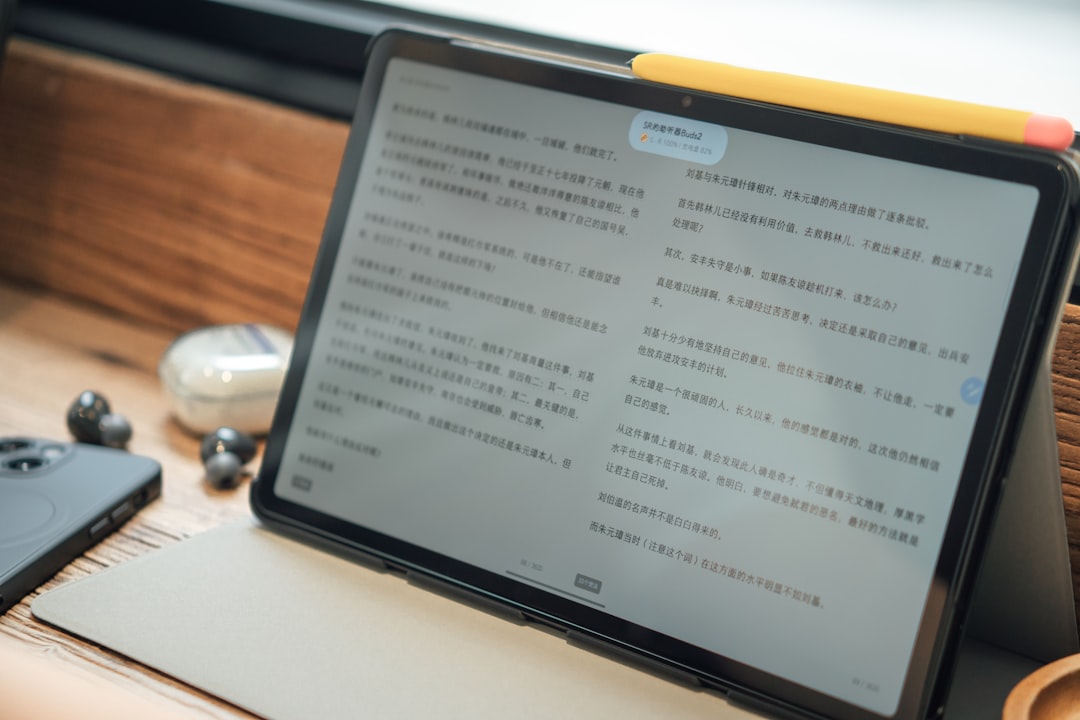
Significant boosts in translation output speed are being observed, with figures reaching around 450 pages per hour for automated systems. This acceleration is largely credited to the use of advanced computing hardware, particularly sophisticated graphics processing units (GPUs) and similar specialized processors. This dramatic increase in processing capability shifts what's feasible for handling large volumes of text requiring translation.
This leap in efficiency is central to the advancements seen in document translation pipelines currently emerging. By enabling much faster computation for the complex neural networks that power modern machine translation, this hardware allows systems to process extensive documents at rates previously unimaginable. The focus here is on speeding up the translation phase itself, taking the output from the text recognition stage and converting it into another language with unprecedented rapidity. While challenges in accurately extracting text from difficult documents persist, the downstream translation process is no longer the primary bottleneck for many bulk tasks, paving the way for more cost-effective and time-sensitive translation workflows. This rapid throughput capability highlights how specialized processing power is fundamentally reshaping the economics and timelines of large-scale document handling via machine translation.
* The figure of approximately 450 translated pages per hour marks a substantial leap in machine translation throughput, seemingly a direct consequence of leveraging powerful, parallel processing hardware like high-end GPUs.
* This accelerated processing power appears to be derived from distributing the computational load of translation tasks across thousands of cores, enabling the pipeline to handle segments or even entire documents concurrently.
* Such speed capabilities significantly reduce the wall-clock time needed for large-scale translation projects, making the processing of extensive document sets potentially a matter of minutes or hours rather than days.
* Attaining this level of performance likely necessitates substantial investment in specialized computing infrastructure, raising questions about accessibility and potential disparities in who can readily deploy such rapid translation workflows.
* A primary concern with such speed is maintaining translation quality; the sheer velocity demands careful consideration of how accuracy, fluency, and contextual appropriateness are consistently preserved or validated.
* The low latency afforded by this speed could enable near real-time processing for documents arriving in a stream, beneficial for applications requiring immediate, albeit potentially rough, translation outputs.
* While initial text recognition from PDFs has its own challenges, the downstream translation speed means the system can rapidly process the cleaned-up or structured text, even if the source formatting was complex.
* The swift processing aids in managing documents containing multiple languages once those languages have been identified, allowing the translation engine to process the distinct linguistic parts quickly as they are fed through the pipeline.
* There's an implicit need for sophisticated quality control loops; while translation might be fast, effective post-editing or automated evaluation methods that can keep pace are crucial for practical use.
* Investigating the relationship between model architecture efficiency and hardware acceleration remains pertinent; future work could explore whether similar speeds might be achieved with less reliance on massive compute power or if speed always necessitates raw processing might.
How OpenAI's PDF-to-Text Pipeline Revolutionizes Document Translation in 2025 - Monthly Translation Costs Drop Below $001 Per Word With New Document Batch System
As of mid-2025, a significant shift is apparent in the economics of high-volume document translation, with monthly costs reportedly dropping below one cent per word. This remarkable affordability seems connected to the deployment of more efficient document batching systems. Underlying this is advancement in pipelines capable of extracting text reliably from various document types, notably PDFs, developments often associated with major AI research initiatives. These systems facilitate the processing of multiple documents concurrently, streamlining the workflow. Compared to the typical range of costs for human translation or even earlier automated approaches, which could run from roughly eight to forty cents per word depending on factors like complexity, this represents a substantial reduction. While the ability to process large volumes of text so cheaply is transforming accessibility, it also prompts consideration of whether output quality remains consistent across diverse and challenging content types under such cost pressure. This drive towards minimal per-word rates also raises broader questions for the translation field regarding required human oversight and validation methods.
From a researcher's standpoint looking at the logistics, the truly striking consequence of this pipeline maturing is the collapse in observed translation costs, reportedly dipping under $0.001 per word in monthly averages for some users utilizing a new document batching capability. Historically, human-driven translation, even at scale, rarely dipped below the $0.10-$0.25 range for standard content; this machine-driven shift represents a fundamental re-alignment of the economic landscape. The batch system itself appears to be less about inventing new translation techniques and more about optimising the downstream processing and resource allocation. By queuing and processing potentially thousands of pages concurrently, the system minimises the overhead and idle time associated with handling documents individually. This simultaneous handling of large volumes, integrating the previously discussed (and potentially fidelity-challenged) OCR outputs and feeding them into the high-speed translation engines, seems to be the key to unlocking such drastically reduced per-word expenditure.
While the sheer throughput achieved by orchestrating these processes is notable, allowing rapid processing for massive document sets, the implied trade-offs warrant close inspection. Does the pressure to deliver translation at such marginal cost necessitate compromises in quality assurance, particularly when dealing with input text potentially derived from OCR that might only achieve 8% accuracy on complex layouts? The reports mention integrated quality control protocols and automated post-editing. The effectiveness of these features at such high speeds and low costs is a critical question, especially for sensitive or nuanced content. Nevertheless, the operational efficiency gained by this batching layer—managing diverse document types and facilitating the rapid handling of files, even those with mixed languages detected during the initial text processing—seems to be the primary driver for making automated translation economically viable on an unprecedented scale. The system's interface reportedly simplifies the submission and tracking process, which, while not a core technical innovation, is crucial for practical large-scale deployment. Given the continuous evolution of both the underlying recognition and translation models, it feels probable that further tweaks to this workflow orchestration could yield even greater efficiencies or perhaps allow for reinvestment in improving the quality aspects, pushing the boundaries of what's feasible in mass-market automated translation workflows.
How OpenAI's PDF-to-Text Pipeline Revolutionizes Document Translation in 2025 - Automatic Layout Preservation Eliminates Manual Formatting After Translation
Automatic layout preservation marks a significant step forward in document translation, directly addressing the long-standing issues traditional methods face, particularly as the need for accurate and visually consistent translated documents persists. Typically, translation systems have focused purely on converting text, often neglecting crucial elements like original document layout and formatting. This traditional approach can lead to translations that are structurally inconsistent and challenging to follow, especially given language differences that affect text length or require changes in direction like with Right-to-Left languages.
Newer pipelines, such as those being developed by OpenAI, are incorporating advanced techniques leveraging natural language processing that aim to preserve the document's original visual structure during translation. By working to maintain layout and formatting as part of the automated process, they strive to minimize the often substantial manual reformatting required once translation is complete. This approach streamlines the overall workflow, making the translation process faster and potentially more cost-effective. Maintaining visual integrity alongside text translation is increasingly seen as crucial for accurate comprehension and professional use. While challenges persist, especially with source documents that are difficult to process initially, the evolution towards systems that prioritize both accurate text and original layout is fundamentally reshaping expectations for automated document translation.
One aspect gaining traction in these automated document processing pipelines is the push towards automatic preservation of original document layout. Traditionally, running documents through OCR and then machine translation often resulted in a plain block of text, losing critical visual structure – tables, lists, headings, footnotes, the very spatial arrangement that conveys meaning and hierarchy. This usually necessitated significant manual effort afterwards to reconstruct the document's format, adding time and cost back into the process.
What we're observing now is the integration of layout recovery techniques directly into the workflow. The ambition here appears to be taking the extracted text and placing it back into a representation that mirrors the original document's visual presentation. This capability, visible in work being explored across various forums and potentially incorporated into systems like the one under discussion, targets the elimination of that tedious manual reformatting step. If successful across the wide variety of document structures encountered in the wild, from multi-column academic papers to financial reports with intricate tables or legal texts with specific formatting requirements, it fundamentally changes the perceived efficiency gains of such pipelines. One key question remains, however: how robust is this automated layout reconstruction when faced with truly complex or unconventional designs, or the subtle formatting differences required by different target languages and scripts? The aim is certainly to deliver a translated output that's not just textually accurate but also visually coherent, minimizing the need for human intervention beyond potential linguistic review.
How OpenAI's PDF-to-Text Pipeline Revolutionizes Document Translation in 2025 - Direct Integration With Adobe Creative Cloud Enables Real Time Document Updates
Direct integration with platforms like Adobe Creative Cloud is increasingly focusing on enabling real-time document updates. What this means in practice is the ability for multiple contributors to potentially work on a single creative asset or document simultaneously within a shared digital space. The intent is to move away from the cumbersome process of exchanging different file versions, which often leads to confusion and delays. These systems aim to provide a more fluid collaborative experience, often incorporating features like live presence indicators, instant synchronization of changes, and consolidated feedback tools. While the concept of collaborative editing isn't entirely new, seeing this capability evolve within established creative and document workflows marks a notable shift in how digital content creation and review are being approached. It represents an effort to streamline interaction within teams, although the effectiveness across highly complex or large-scale projects still warrants practical evaluation.
Integration points with established platforms like Adobe Creative Cloud appear designed to facilitate a more integrated workflow, potentially allowing document states to synchronize relatively rapidly between creative design environments and the translation pipeline.
1. The synchronization mechanism allows for what is described as 'real-time' updating of document states. The aspiration is that modifications initiated on one end of the process, say in a design file within Creative Cloud, are quickly reflected in the representation managed by the translation system, aiming to keep linguistic work aligned with the source document's evolution and theoretically reducing issues stemming from outdated source material.
2. Interfaces are being explored that permit linguists or reviewers to make linguistic adjustments directly within a visual context approximating the original document's structure. This implies some level of interplay between the extracted text, the proposed translation, and the spatial layout information, aiming to provide immediate visual feedback during the editing process.
3. Handling documents with complex compositions involving distinct layers for text, imagery, and perhaps graphic elements is a claimed capability. The underlying system presumably attempts to parse and represent these separate components, aiming to maintain their relative positions and interrelationships during the translation phase to reduce the need for extensive manual reconstruction afterward.
4. Utilizing Adobe's platform ecosystem ostensibly provides access to established file format handling capabilities. The goal here is to bypass typical format conversion hurdles by leveraging existing compatibility layers within the Adobe environment, simplifying the intake of various document types into the pipeline.
5. The choice to integrate with a widely used suite like Adobe Creative Cloud suggests an attempt to lower the barrier to entry for existing users of those tools. The expectation is that familiarity with the host environment's navigation and conventions will make learning the specific translation features less steep, focusing user effort on the linguistic task rather than tool mastery.
6. There is an argument that managing synchronized updates across potentially multiple documents concurrently can contribute to overall workflow throughput, particularly when dealing with sets of inter-related files that undergo continuous revision. This appears to focus on reducing the downtime associated with traditional sequential processing and manual file exchanges.
7. Systems reportedly incorporate mechanisms to capture user interactions, such as edits or layout adjustments, during the translation process. The intention is that this operational data can inform iterative refinements, potentially feeding back into model improvements related to translation accuracy or layout reconstruction over time, assuming this feedback loop is effectively implemented.
8. For design teams already invested in the Adobe suite, leveraging this integration for translation is presented as potentially reducing the need for separate external linguistic services. The claim is that streamlining the process within their established toolchain offers an economic benefit by consolidating tasks, though the total cost including platform licensing remains a factor.
9. A key objective is the preservation of visual context beyond mere text flow, including elements like embedded charts, diagrams, or specific typographic styles, as they are carried through the translation process. The underlying technical challenge is ensuring these non-linguistic visual components retain their relevance and placement relative to the translated text, maintaining the document's intended informative value.
10. The architectural design, leaning on cloud infrastructure and concurrent update handling, is posited to support scaling for larger projects involving numerous documents and potentially many collaborators. The efficiency in managing the dynamic state of these documents is central to enabling high-volume operations without introducing bottlenecks related to version control or file synchronization.
How OpenAI's PDF-to-Text Pipeline Revolutionizes Document Translation in 2025 - Offline Processing Mode Allows Document Translation Without Internet Connection
The introduction of a mode for offline processing in document translation is becoming a tangible benefit, particularly important for those dealing with limited or unreliable internet access. This capability means translating various file types, including PDFs and standard word documents, can be done without a constant online connection. Systems that integrate sophisticated text extraction methods, potentially derived from advanced pipelines, are being adapted to function entirely locally. Processing data on the user's device offers clear advantages for privacy and security, especially when handling sensitive documents that cannot be uploaded to external servers. While this enables greater freedom and accessibility for users wherever they are, relying on purely offline models can sometimes come with limitations regarding the scope or accuracy of translation compared to frequently updated cloud-based services, which can leverage larger, more dynamic models. Despite potential trade-offs, the availability of robust offline document translation tools represents a significant step forward in user empowerment and independence.
Focusing specifically on the notion of an 'offline processing mode' within these emerging document pipelines presents a distinct set of implications from a technical perspective.
1. The fundamental advantage is clear: freedom from network dependency. This capability means processing, including initial text extraction and subsequent translation, can hypothetically occur in environments where internet connectivity is non-existent, unreliable, or intentionally restricted for security reasons.
2. Achieving reliable performance necessitates shifting significant computational requirements onto local hardware. This implies that the system's efficacy is now constrained by the user's machine specifications, which could introduce variability and accessibility issues depending on model complexity and resource demands.
3. A primary driver for exploring offline options, particularly for handling sensitive documents, is data privacy. By keeping the entire processing loop within a local network or device, the risk of data interception or exposure associated with transmitting information to external cloud servers is theoretically eliminated.
4. Integrating complex machine learning models for tasks like text extraction and multilingual translation to function effectively within diverse, potentially resource-limited local environments poses substantial engineering hurdles related to model size, optimization, and compatibility across different operating systems and hardware configurations.
5. While local processing bypasses network latency, the actual speed of text extraction and translation is now solely determined by the power and configuration of the client-side hardware. This might not always match the potential scale or processing power available in a large, centralized cloud infrastructure.
6. The offline model introduces challenges for software distribution, updates, and model maintenance. Ensuring users have the latest, most accurate models and security patches without a constant internet connection requires robust local management or periodic manual synchronization mechanisms.
7. Handling the diversity of document formats, especially complex or poor-quality scans, fully offline requires sophisticated, self-contained OCR components capable of high resilience without external validation or feedback loops relying on cloud services.
8. The economic model shifts from potentially recurring cloud service fees to an upfront investment in powerful enough local hardware and the ongoing costs associated with maintaining and powering those systems for intensive processing tasks.
9. Developing and managing a seamless user experience that combines local processing with potential future synchronization or integration points (if needed for collaboration or updates) introduces architectural complexities compared to a purely online service.
10. The ability to provide real-time interactive features or feedback loops during local processing relies entirely on the efficiency of the client-side software and hardware, potentially limiting the sophistication of such interactions compared to systems leveraging server-side compute power.
AI-Powered PDF Translation now with improved handling of scanned contents, handwriting, charts, diagrams, tables and drawings. Fast, Cheap, and Accurate! (Get started for free)
More Posts from aitranslations.io: