Debunking Myths 7 Common Misconceptions About AI Translation Accuracy
Debunking Myths 7 Common Misconceptions About AI Translation Accuracy - AI translation accuracy varies widely by language pair
The quality of AI-driven translation isn't uniform across all language combinations. Some language pairs, particularly those with limited training data available to AI systems, see significantly lower accuracy rates compared to others. This is simply because AI learns from examples, and a scarcity of good examples for a specific language combination leads to a less robust and accurate translation output. While AI translation has progressed considerably, it still faces hurdles, notably in understanding the subtle cultural and contextual aspects inherent in language. Human translators often excel in these areas, especially when dealing with less frequently encountered languages. As AI technology develops further, the disparity between human and AI accuracy in certain language pairs will likely remain a focal point for researchers and users alike.
The effectiveness of AI in translating languages varies considerably depending on the specific language combination. For instance, while English-Spanish translations often yield good results due to the vast amounts of training data available, pairs like Icelandic-Mandarin present a far greater hurdle for AI systems. This is primarily due to the limited datasets available for less commonly used languages, hindering the AI's ability to learn the intricate nuances of these languages.
Language structure itself plays a crucial role in translation accuracy. AI models seem to perform better with languages that have simpler grammatical rules like Indonesian compared to more complex structures like Finnish. These differences in grammar can make it challenging for AI to properly parse and translate sentences accurately.
Certain languages pose unique difficulties for AI due to variations within the language itself. For example, Arabic's diverse dialects can confuse AI systems that may be well-trained on Modern Standard Arabic but stumble when encountering regional dialects. This highlights the importance of AI models having a strong understanding of contextual variations in order to produce accurate translations.
The availability of parallel corpora—aligned texts in different languages—is key for improving AI translation accuracy. However, many languages, particularly minority languages, lack such resources. The scarcity of these paired texts hinders the ability of AI systems to learn accurate translations.
When dealing with niche subject matter like medical or legal documents, AI translation often suffers from a lack of training data within these specific fields. Consequently, this lack of specialized training data often leads to inaccuracies, potentially impacting the reliability of translations in such crucial areas.
Although AI can execute translations swiftly, this speed can sometimes negatively impact accuracy. This is especially true for idioms and expressions, where a direct word-for-word translation might completely fail to capture the intended meaning, necessitating a deeper level of comprehension that AI struggles with.
While individual sentences are often successfully translated by AI, a challenge emerges when piecing together coherent paragraphs. The lack of contextual awareness across multiple sentences can result in translations that feel disjointed and lack a smooth flow.
Furthermore, cultural nuances represent a significant hurdle for AI translators. Subtleties like humor, irony, and sarcasm often depend on cultural context that AI models are not naturally equipped to interpret, potentially leading to misinterpretations or altering the intended meaning of the original text.
Languages like French and Spanish with gendered nouns present another obstacle for AI translation algorithms. These grammatical distinctions require the AI to carefully track and assign genders throughout a translation, which can be challenging, sometimes leading to errors and inaccurate gender representation in the output.
It's important to note that the improvement in AI translation isn't always linear. While some language pairs have demonstrated significant advances recently, fluctuations in accuracy are still common, influenced by algorithmic updates and changes to the training data. Predicting future accuracy for different language pairs continues to be a difficult task due to these unpredictable factors.
Debunking Myths 7 Common Misconceptions About AI Translation Accuracy - Machine learning improves with more diverse training data
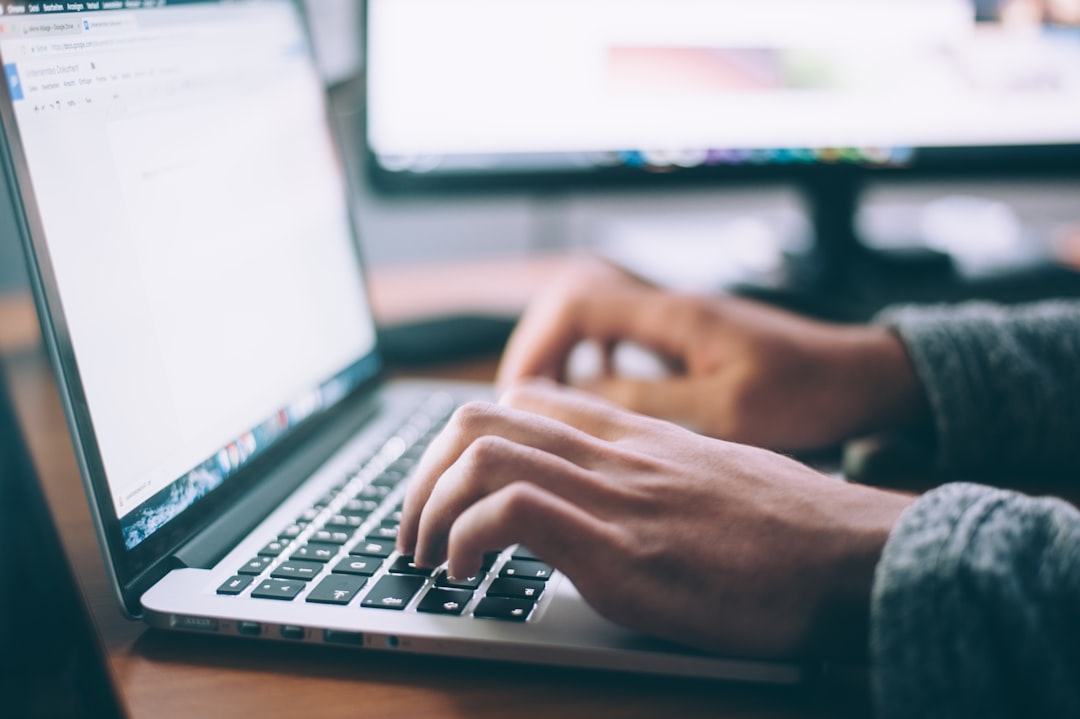
The effectiveness of machine learning hinges on the diversity of the data it's trained on. Simply having a large amount of data isn't enough; the quality and variety of that data are equally important. If the training data is limited or lacks diversity, it can hinder the model's ability to learn and produce accurate results. In the realm of AI translation, this means that AI systems trained on primarily common language pairs may struggle with less frequently encountered languages or their nuances due to a lack of diverse training examples. This can lead to inaccurate translations or a failure to fully capture the intricacies of certain languages. The goal of fostering more accurate and versatile AI translation models, then, lies in recognizing the significance of rich and varied training datasets. This insight helps dispel the misconception that AI translation is universally reliable and highlights the importance of acknowledging its limitations.
Just as a diverse student body enriches a classroom, the performance of machine learning in AI translation improves dramatically with a wider variety of training data. We've seen that AI learns patterns from examples, and when those examples are limited to a narrow set of language variations, the AI's ability to translate accurately suffers. For instance, if an AI model is primarily trained on formal, written Spanish, it might struggle with the rapid-fire, slang-filled conversations of teenagers in Mexico City.
The beauty of this is that a more diverse dataset allows the model to develop a more robust understanding of language, including its variations like dialects and informal language. This increased exposure, essentially, teaches the AI to be more flexible and adaptable. Imagine training an AI with Spanish language data from news articles, social media posts, and even subtitles from movies. It would be better equipped to handle the diversity of real-world language and provide more accurate translations.
This is not just about improving the accuracy for specific dialects; it's about the AI system being able to generalize to new and unseen data. An AI trained on a wide range of Spanish would have a better shot at understanding the Spanish from a newly released documentary or an obscure blog post than one trained on a much more limited set of Spanish language examples.
However, there are wrinkles here. Just throwing a bunch of data at the AI doesn't magically make it a genius translator. If the data is messy, unorganized, or contains errors, it can actually harm the AI's performance. Furthermore, training on an abundance of new data can lead to a phenomenon known as "catastrophic forgetting", where the model starts to lose its ability to translate language pairs it had previously mastered. It's a delicate balancing act: we want variety but need to maintain the system's proficiency in already-learned language pairs.
It's fascinating to consider that even introducing data beyond text, such as cultural background or historical context, can boost an AI's understanding. This allows it to better handle expressions and cultural references that might otherwise confuse it. This type of holistic approach to data selection mirrors the way human translators work, taking into account cultural and historical information to make accurate translations.
We're still in the early stages of understanding how best to train AI for optimal translation. It's clear that achieving a balanced and representative training dataset for each language will be key, not only for better translation quality for certain languages but also for enhancing the overall performance of multilingual AI systems. This research holds promise to close the translation gaps that currently exist between various languages and potentially provide greater access to information and communication across cultures.
Debunking Myths 7 Common Misconceptions About AI Translation Accuracy - Human review remains essential for critical translations
Even with the progress of AI in translation, human review remains crucial for translations that demand accuracy and sensitivity. AI tools, while improving, often struggle with the complexities of language, particularly in understanding idioms and nuanced expressions. They frequently lack the contextual awareness needed for specialized domains, such as legal or medical fields. This limitation can result in mistranslations that significantly alter the intended meaning. While AI can expedite simpler translation tasks, human translators are still necessary for critical translations where the integrity of communication is paramount. This is because humans can provide the nuanced understanding of culture, emotion, and context that remains beyond the current capabilities of AI. While AI can help with the initial draft, it's the human eye that ultimately ensures the message is conveyed with the precision and accuracy needed for vital communications.
While AI translation tools show promise for quick, basic translations, human review remains essential, particularly when dealing with specialized content like legal or medical documents. AI often struggles with the nuances of technical terminology, and a single mistranslated term in these areas can have serious consequences. Relying solely on AI in such critical contexts can be risky, making human oversight vital.
Language's flexibility, especially in creative writing and poetry, presents a significant hurdle for AI. These forms often play with structure, rhythm, and implied meaning in ways that AI, which generally focuses on literal translation, often misses. Human translators, however, possess the sensitivity to capture the artistic nuances and preserve the original intent, a skill that's still beyond AI.
Cultural references and idiomatic expressions often stump AI, leading to inaccurate or misleading translations. Human reviewers can expertly navigate cultural contexts, ensuring the translated text conveys the desired meaning instead of a potentially confusing literal interpretation that AI might produce.
AI can fall prey to "overfitting", meaning it becomes overly tailored to its training data and struggles with real-world texts that differ from those examples. This limits AI's adaptability, and human translators, with their varied experiences, can bridge this gap and provide a more robust and flexible translation.
Human translators possess a crucial element that AI still lacks: emotional intelligence. They can analyze the context and underlying emotions behind a statement, which can alter the overall tone and effectiveness of communication. This ability is vital when handling sensitive materials, where AI's inability to fully grasp emotions can lead to misinterpretations and weaken communication.
Furthermore, maintaining coherence when translating texts that frequently switch between multiple languages continues to be a challenge for AI. Humans possess a natural ability to navigate these contexts and maintain consistency throughout the translation, while AI can falter, generating inconsistent outputs.
The complex and diverse grammatical structures of various languages pose a further hurdle for AI. Languages with flexible word order, like Latin, demand a level of understanding that AI hasn't yet attained. Human intervention becomes crucial in these instances to avoid inaccuracies that could arise from AI's limitations.
For languages with limited training data, often referred to as low-resource languages, AI translation accuracy can suffer significantly. In these scenarios, human translators become invaluable. They can not only provide accurate translations but also help build a foundation for more robust AI systems by contributing their expertise to the development of training datasets.
The complexities of humor, sarcasm, and irony remain beyond AI's grasp because these linguistic tools often rely heavily on cultural context. Human reviewers are adept at recognizing such nuances, ensuring that the intended humor or tone is accurately translated and the original message isn't lost or misinterpreted.
Despite continuous improvements in AI translation technology, a noticeable gap often exists between user expectations and actual AI capabilities. This gap can lead to users potentially introducing errors by over-relying on AI-generated translations without proper human review, diminishing the overall accuracy of the final output.
Debunking Myths 7 Common Misconceptions About AI Translation Accuracy - OCR errors can significantly impact AI translation quality
OCR errors can significantly impact the quality of AI translations. When the initial step of converting a scanned document to text (OCR) contains inaccuracies, it introduces noise into the data that the AI translation system uses as its foundation. AI translation relies on accurate source text to deliver a faithful translation, and OCR errors can disrupt this process. While AI translation has become faster and more accessible, it remains highly dependent on the accuracy of the initial input. These errors can create challenges for AI models, particularly in their ability to understand complex linguistic nuances and contextual clues. This emphasizes the need for careful attention to the quality of the source material before initiating AI translation. In essence, inaccurate OCR can hinder the AI's ability to produce a good translation, highlighting the limitations of relying solely on automated systems without reviewing the initial OCR output. Even minor OCR errors can negatively impact the overall accuracy of an AI-generated translation.
OCR errors can significantly impact the quality of AI translation. The initial step in many AI translation workflows involves OCR, a technology that converts images of text into editable data. However, OCR isn't perfect. Handwriting variations, poor image quality, and unusual fonts can all confuse the OCR system, leading to mistakes in the recognized text. This is a significant problem, as any errors introduced at the OCR stage get passed along to the AI translation system. The AI essentially learns from flawed data, leading to a cascade of errors and ultimately impacting the accuracy of the final translation.
The challenge is particularly acute when dealing with languages that use complex character sets or writing systems, like Chinese or Arabic. These languages have greater potential for OCR errors due to their unique symbols and features, which in turn can greatly reduce translation quality. This issue is further amplified in handwritten documents where the variability of human writing styles makes accurate character recognition very difficult. Even things like the layout of a document can matter. If a document has text within images, unusual column structures, or inconsistent spacing, OCR accuracy tends to suffer. This demonstrates how the initial step of text recognition, before the translation even begins, can impact the outcome.
While AI systems can, over time, learn to recognize patterns in OCR errors and potentially adapt, the initial results often suffer. It’s important to acknowledge that the AI is still learning and adapting, and early on, it might produce flawed translations. A user might mistakenly believe the translations are accurate due to the speed at which the AI produces them. Preprocessing the documents – cleaning up images, standardizing fonts – is often an overlooked step that can improve OCR accuracy.
This issue becomes particularly serious in specialized fields like law or medicine, where technical terms and jargon are common. One misplaced character or a misread word in a legal or medical document could significantly change the meaning, leading to serious consequences. When you add speed into the mix, as in environments where documents are processed rapidly, OCR errors become even more amplified as there is limited time for human review or intervention. This illustrates the delicate balance between the speed of AI-driven translation and its potential for errors stemming from OCR limitations.
Integrating OCR seamlessly into AI translation pipelines presents a further challenge. OCR systems vary in quality, and the inconsistency of OCR output can pose challenges for the translation model. It essentially needs to process input that isn’t always uniform, making consistent and accurate translations more difficult. All of this reinforces that even in this era of advanced AI, basic steps like accurate text recognition remain critical to achieving good translation quality. While AI translation continues to advance, overcoming these OCR-related issues will likely remain a persistent challenge.
Debunking Myths 7 Common Misconceptions About AI Translation Accuracy - Real-time translation speed often trades off with accuracy
The pursuit of fast translation often comes at the cost of accuracy in real-time translation systems. These systems, while capable of delivering quick results, may struggle with the subtleties of language, including idioms and culturally specific expressions. Although AI translation models are continuously refined with more varied training data, the intricate nature of human language can still lead to AI misinterpretations of context. This means that, despite the convenience of fast translation, it's crucial to understand that a thorough review is frequently necessary to guarantee the translated text is accurate and conveys the original message faithfully. While AI aids in creating a swift initial translation, human intervention often remains essential for ensuring quality.
Real-time translation, while appealing for its speed, often compromises accuracy. The emphasis on rapid output can lead AI to prioritize speed over a deeper understanding of context, resulting in translations that might be grammatically correct but miss the subtleties of the original text. This is particularly evident when dealing with idiomatic expressions or slang, where a direct word-for-word translation can fail to convey the intended meaning.
The complexity of human language, with its diverse dialects, cultural nuances, and evolving slang, poses a challenge for AI models that are trained for fast results. AI systems designed for rapid translation might struggle to adapt to the fluidity of spoken or informal language, potentially leading to misinterpretations and inaccurate outputs.
Furthermore, the rapid pace of AI translation development can lead to a disconnect between the speed of development and robust quality assurance. While constant updates and refinements to algorithms are important, the pressure to quickly deliver translations might result in insufficient testing and verification. This could mean errors persist or even worsen over time without ample opportunities for careful review and refinement.
OCR, a crucial step in many AI translation processes, also suffers under the pressure of speed. Languages with intricate writing systems, such as Chinese or Arabic, are especially prone to OCR errors. A single character misread at the OCR stage can ripple through the translation, significantly affecting the final output. This emphasizes that a rushed OCR process can significantly impact the overall quality of AI translation.
The focus on speed can also hinder the AI's ability to learn from its mistakes and adapt to diverse linguistic contexts. AI trained on specific datasets might become overly specialized, unable to easily handle texts outside of its training examples. When quick results are prioritized, these limitations can be amplified, and the AI might rely too heavily on what it's already learned, ignoring nuances in new data.
While the speed of AI translation is valuable, it's also important to recognize the risks associated with neglecting thorough quality checks. An eagerness for rapid results can overshadow the need for verification, especially when dealing with complex or critical content. This lack of rigorous validation can lead to the propagation of errors, potentially compromising the effectiveness of the translation.
Moreover, the speed of AI translation sometimes compromises the ability to convey human nuance. AI might struggle with capturing subtle emotional cues or cultural context that human translators naturally integrate into their work. A rushed translation might miss the irony or sarcasm in a sentence, resulting in a completely different message being delivered.
The challenge of ensuring accuracy in AI translation becomes even more pronounced when considering the potential for cascading errors. A minor OCR mistake can lead to a series of inaccuracies as the AI processes the text. The desire for quick results might lead to an insufficient focus on the quality of the initial OCR, leading to a compounding effect of errors that are more difficult to identify.
This highlights the delicate balance that exists in AI translation. While the capacity to translate quickly is valuable, it shouldn't come at the expense of sacrificing accuracy and nuanced comprehension. As the field continues to evolve, researchers and users need to be mindful of these trade-offs and prioritize a balanced approach that values both speed and quality.
Debunking Myths 7 Common Misconceptions About AI Translation Accuracy - Idioms and cultural context still challenge AI systems
AI translation systems, while showing improvement, still face difficulties when encountering idioms and cultural nuances embedded within language. These systems often struggle to grasp the subtle meaning conveyed by idiomatic expressions and culturally-specific references, which demand a thorough comprehension of context. This limitation frequently leads to translations that are grammatically sound but fail to capture the intended message accurately. Despite the progress of AI in this area, the need for human translators, who possess the ability to understand and interpret these intricacies, persists. Human expertise is critical in scenarios where precision in communication is crucial, ensuring the faithful conveyance of meaning and cultural sensitivity. Over-reliance on AI without careful human review can result in translations that lack the necessary cultural awareness, potentially leading to misinterpretations and misunderstandings.
AI translation has made remarkable strides, but the nuances of human language continue to pose a challenge. Idioms and culturally-specific expressions, for instance, often rely on a depth of understanding that current AI models haven't quite grasped. A simple phrase might be translated literally, leading to a nonsensical outcome because the AI hasn't understood the cultural or historical significance behind it. Similarly, AI struggles with subtle cultural references that humans effortlessly pick up on, potentially leading to unintended misinterpretations.
Furthermore, language is a dynamic entity. Slang evolves, dialects vary, and trends shift over time. AI, trained on static datasets, can become quickly outdated, especially in situations where the language is changing rapidly. This poses a problem for real-time translation, where quick responses are crucial. Even subtle aspects like sarcasm and humor, which depend on tone and context, often elude AI, due to its lack of a social and emotional understanding.
Beyond that, maintaining context across longer pieces of writing presents a real issue. While individual sentences might be translated well, the flow of ideas or the overall voice might be lost. The resulting text can feel fragmented and incoherent, compromising the intended message. The complexity of languages with intricate grammar or structures also presents a hurdle for AI. Languages like Finnish or Hungarian, for example, have grammatical systems very different from English, and the AI may struggle to predict and adapt to these structures accurately.
Another roadblock is the presence of multiple meanings within a single word or phrase. This semantic ambiguity is a challenge for AI because it might fail to pick up on the nuances of a particular context. This issue becomes especially important when dealing with legal or specialized documents where precision is key.
Moreover, the limited availability of high-quality training data for many languages hinders the AI's ability to learn. Languages spoken by smaller communities, or "low-resource" languages, often lack comprehensive datasets, which means the AI can't fully absorb the cultural and idiomatic nuances needed for accurate translations.
Finally, there's a more subtle issue of social nuance. AI may lack the understanding of human social dynamics and how relationships, or hierarchy, influence the communication process. This can lead to problematic or misconstrued translations, particularly in professional settings. It underscores the significant role human intuition and experience play in translation, because humans can navigate the emotional and social landscape of a text in ways AI is still learning to do. While AI has become a valuable tool for fast and straightforward translation, it's important to be aware of its limitations, particularly when dealing with complex or sensitive communication.
Debunking Myths 7 Common Misconceptions About AI Translation Accuracy - Neural networks struggle with very long or complex sentences
AI translation systems, built upon neural networks, often encounter difficulties when faced with lengthy or intricate sentences. The complex grammatical structure and multifaceted meanings within these sentences can sometimes confuse the AI, leading to errors in interpretation and, ultimately, inaccurate translations. This highlights the fallacy of viewing AI translation as universally flawless. While these systems can be effective for certain translation tasks, they still require human review to properly manage the full spectrum of natural language intricacies. The AI's limitations in capturing subtle meaning and maintaining logical flow across sentences further reinforces the importance of careful consideration when relying on automated translation alone. As AI translation continues to advance, it's crucial for users to remain cognizant of these inherent challenges to ensure the reliability of the translations they obtain.
Neural networks, while powerful, often stumble when faced with very long or intricate sentences. Their internal structures, usually optimized for shorter, simpler inputs, can lose track of the connections between words in lengthy phrases. This can lead to translations that miss the mark.
One key issue is the difficulty in parsing complex sentence structures. Sentences with numerous clauses and embedded phrases introduce ambiguity that confuses the AI, sometimes leading to a translation that misses the original meaning entirely. Essentially, the AI model can get lost in the intricate relationships between words.
Another factor is how neural networks rely on dependency parsing to decipher the way words relate to each other. In long sentences, the relationships become incredibly complex, overwhelming the AI's ability to generate a coherent translation.
Furthermore, these networks can accumulate errors as they process each part of a long sentence. Each mistake can add up, especially if the model doesn't have a built-in mechanism to correct earlier errors as it goes along. This snowball effect contributes to larger translation inaccuracies.
Most neural networks also have a limited capacity to consider the broader context of a sentence, essentially only focusing on a small window of words. In a long sentence, crucial pieces of information might fall outside this window, leading to a translation that misses important aspects.
This issue is compounded by how training data is often skewed towards shorter sentences. This imbalance makes neural networks less equipped to handle the complexity of longer, more nuanced ones, showcasing the influence of training data quality.
Additionally, the way AI translates text often involves breaking it into smaller pieces. However, with complex sentences, these pieces can be wrongly segmented, leading to further errors in translation.
The resulting output from these overly complex sentences can sound disjointed and lack a natural flow. The AI models struggle to maintain coherence and logical flow, especially when there are multiple clauses spread throughout the sentence.
There's also a trade-off between speed and accuracy. While AI translation is known for its quick processing, this efficiency fades when dealing with extensive sentences. The AI then slows down, and in order to try and maintain speed, may cut corners, sacrificing accuracy in the process.
Finally, long sentences can include many different smaller contexts that an AI might not fully connect. This misinterpretation can create a translation that strays from the original message, emphasizing the need for human intervention in reviewing long or complicated text. It's a reminder that AI, despite its rapid progress, still requires human oversight for certain types of translations.
More Posts from aitranslations.io: