Why AI Translation Gets Nostalgic Comparing Machine Learning Accuracy to Portishead's It Could Be Sweet Sampling Techniques
Why AI Translation Gets Nostalgic Comparing Machine Learning Accuracy to Portishead's It Could Be Sweet Sampling Techniques - Sampling Nostalgia From 1994 to 2024 A Look at How Portishead Meets AI Translation Memory
"Sampling Nostalgia From 1994 to 2024" examines how the way Portishead sampled music in the 90s mirrors how AI translation memory operates today. The rise of AI in translation, with its vast stores of past translations, feels strangely reminiscent of how Portishead blended old sounds with new, creating a sense of nostalgic familiarity. Just as their music taps into emotional connections with the past, modern translation technology seems to yearn for the depth and nuance of human language, which was often simpler in the past.
This parallel highlights how our digital age, with its vast datasets and fast-paced innovations, still grapples with a desire for continuity and meaning. AI translation, while technically impressive, also suggests a longing for the era of more "human-centric" communication. We can see the nostalgia in the way AI searches through its massive library of past translations, reusing and repurposing language in a manner analogous to the musical sampling that shaped Portishead's sound. The process raises questions about how our understanding of language develops—not just through new innovations, but by actively acknowledging and learning from the linguistic patterns of our history. Essentially, this exploration encourages us to consider the role translation plays in shaping our collective memory and our identities in the present and the future.
Looking back from 2024, the journey of AI translation mirrors some of the innovative approaches Portishead took with sampling in the 1990s. AI systems, fueled by massive datasets, mimic the way humans use language, creating outputs that are sometimes surprisingly inventive despite imperfections. This is akin to how Portishead, through clever manipulation of sound snippets, crafted a unique sonic landscape on "Dummy".
The rise of OCR has certainly played a role, becoming significantly more accurate over the past three decades. We've moved from crude, early OCR systems to highly precise tools pushing 99% accuracy. This has allowed AI to ingest and process a vast quantity of text, further driving improvements in translation. But, just as Portishead’s music wasn't just about sampling, AI translation can't solely rely on algorithms. The challenge remains in handling complex language nuances, idioms, and the contextual elements that human translators instinctively navigate.
There's a striking similarity in the way both AI and Portishead’s work "sample" material. AI assembles existing language patterns like a DJ mixing tracks, resulting in translations that sometimes lack the smooth flow or authenticity of human work. The speed with which it happens is another point of comparison. AI translation is incredibly fast, even real-time, a vast difference from traditional human translation— similar to how the speed and immediacy of electronic music impacted how music was made and experienced.
However, just like sampling in music, this AI approach also raises issues. While translation memories allow for quick reuse of previous translations, ensuring the accuracy of context and meaning across languages continues to be an obstacle. This echoes the challenge of adapting a music sample to fit a new musical context without losing the original’s essence. The very act of "reinterpretation" by AI can distort the original meaning, leaving us to ponder whether there's an inevitable loss of fidelity.
Furthermore, like Portishead’s music sometimes reflecting the cultural influences of the samples it used, AI translation systems are prone to absorbing biases that are present in their training data. This means that the resulting translations can reflect ingrained biases from the sources, highlighting the importance of understanding the origins of the data. At the same time, the accessibility of AI translation tools has led to increased communication across the globe, similar to how electronic music production democratized music creation, allowing for a wider range of voices and ideas to be heard.
As we reach 2024, the boundaries of language processing are being continuously redefined by AI. It's a progression similar to how Portishead blurred musical boundaries in their time. And, as AI technologies further advance, we may see them move beyond mere translation and into areas that more closely resemble creative expression— perhaps a new form of sonic and semantic artistry.
Why AI Translation Gets Nostalgic Comparing Machine Learning Accuracy to Portishead's It Could Be Sweet Sampling Techniques - Why Trip Hop Audio Processing Still Guides Modern Machine Learning Translation Models
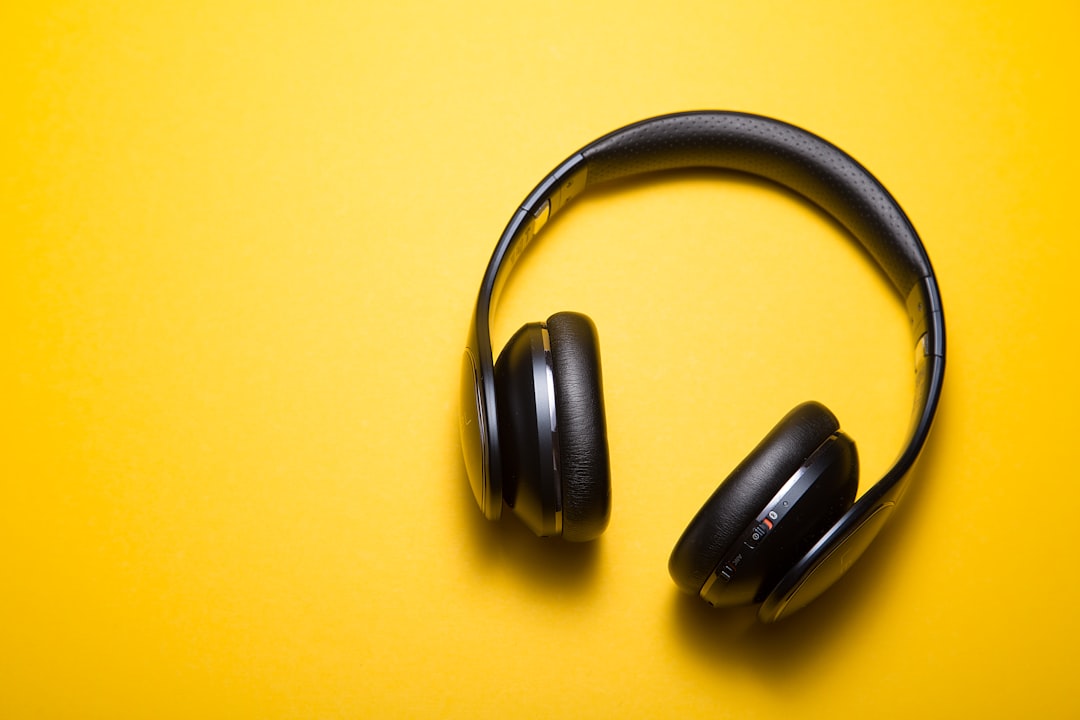
The way trip hop artists manipulated audio samples to create unique soundscapes has a surprising parallel in how today's AI translation models function. Much like Portishead layered and blended various audio snippets, AI translation models stitch together language elements from vast datasets to create their outputs. This approach, akin to sampling in music, presents AI with similar challenges – balancing the integrity of the original meaning with the need for a cohesive and understandable translation within a different linguistic context. AI translation, still in its developing stages, faces questions about the authenticity and accuracy of its output, much like artists questioned the use of sampling in music. The reflection on trip hop in AI translation isn't just about musical nostalgia. It points to the ongoing evolution of how AI handles language, highlighting the delicate balance between creative application and the technical execution that are both inherent in such innovative technologies. The intersection of artistic expression and machine learning processes becomes apparent when examining how AI continues to refine its language capabilities, ultimately striving for a better understanding of human communication, a complex process that is constantly being refined just as trip hop redefined the artistic parameters of music.
The way audio is processed in Trip Hop, with its emphasis on sampling and layering, has some interesting parallels to the statistical methods used in modern machine learning translation models. Think of how a DJ carefully selects and blends different audio snippets to create a track – machine learning models do something similar by analyzing vast datasets to identify patterns in language.
Just like Portishead manipulated various sound sources to craft a unique sonic texture, AI translation models often combine fragments of past translations to generate new outputs. This process can be seen as a form of creative reinterpretation, similar to how a musician might reimagine an old melody.
You can also find parallels between some of the audio effects used in Trip Hop, like echo and reverb, and the normalization techniques employed in AI sound processing. These effects enhance the depth of music in the same way normalization techniques improve AI model performance by ensuring consistency across translation outputs.
However, just like there are risks when musicians misuse or poorly adapt samples in a song, AI translation can struggle when it misinterprets the context of a text. A poorly adapted sample can disrupt the flow of a musical piece, while a contextually inappropriate AI translation can distort the original meaning, much like a bad translation of a poem might miss the poetic intention.
Interestingly, AI models leverage historical linguistic data in a way that resembles the emotional undertones that Portishead sought to evoke through their sampling. Both approaches aim to balance accuracy and sentiment.
The rapid improvement of OCR technology over the past few decades has been comparable to the evolution of audio editing tools, going from simple functionality to advanced, sophisticated systems. Both developments allow for the swift and precise capture and processing of immense amounts of information.
The nostalgic feeling that comes from hearing a familiar sample in music is also relevant here. AI translation can sometimes create translations that resonate with users by activating familiar phrases and linguistic patterns. This can be very engaging for people, but it also reminds us that contextual precision remains a persistent hurdle.
The 1990s was a time of bold experimentation in music, which is a lot like the way AI is currently pushing the boundaries of linguistic structures. However, both domains face a challenge in keeping authenticity while driving innovation.
In Trip Hop, musicians often layer various instruments to create intricate and multi-dimensional musical landscapes. Similarly, machine learning models draw on multifaceted linguistic data to produce complex and nuanced translations. However, in both domains, excessive layering or incorporating too much data can sometimes lead to confusion, and result in less clarity.
The democratization of language access through AI tools echoes the impact of technological advancements on the music world, where barriers to creation were reduced. This accessibility brings up questions about the authenticity and fidelity of AI-produced translations, similar to debates around originality in music sampling.
Why AI Translation Gets Nostalgic Comparing Machine Learning Accuracy to Portishead's It Could Be Sweet Sampling Techniques - Fast Cloud Translation vs Analog Band Pass Filters The Legacy of 90s Audio Engineering
The comparison of "Fast Cloud Translation vs Analog Band Pass Filters" reveals a fascinating connection between modern translation methods and the audio engineering practices of the 90s. Similar to how analog band pass filters isolate specific sound frequencies, fast cloud translation systems sift through a vast ocean of linguistic data to generate quick translations. This process, though remarkably swift, often struggles to fully capture the subtlety and richness of human language, just as audio manipulations could sometimes distort the purity of sound. The echoes of 90s audio engineering remind us of the importance of precision and integrity in both sound and language. There's a sense of nostalgia, perhaps, in realizing that the desire for clarity and fidelity remains constant even as technology rapidly evolves. The exploration of these historical connections might offer valuable insights as we strive to improve AI translation, mirroring the dedication to crafting high-quality audio that characterized 90s audio engineering. This shared pursuit of accuracy and meaning suggests that the underlying principles of preserving the integrity of a message, whether it's a musical track or a translated text, remain crucial in this evolving digital landscape.
Fast cloud translation services, with their ability to deliver translations in real-time, share a fascinating resemblance to the rapid sampling techniques that shaped 90s electronic music. This speed, while enabling instant communication, also raises questions about the nuances lost in the process, just as some questioned the artistic integrity of music relying heavily on sampling.
The journey of Optical Character Recognition (OCR) mirrors the development of audio sampling. Early OCR, limited to a handful of fonts, has evolved into sophisticated AI-driven systems achieving incredible accuracy. This evolution, like the shift from analog to digital audio, highlights how we've gotten better at both capturing and understanding language, both visually and aurally.
Similar to how a DJ meticulously selects and combines samples to craft a musical piece, AI translation models analyze massive datasets to identify language patterns. This parallels the crucial role of context in music: if a DJ's choices lack cohesion, the whole track suffers. Likewise, a poorly contextualized translation can easily confuse the intended meaning.
However, just as musical samples often reflect the cultural background of their source, AI translation models can unknowingly absorb biases present in their training data. This can inadvertently lead to translations that perpetuate harmful stereotypes, raising critical questions about the ethical considerations in AI development and the potential for it to mirror and amplify societal biases.
The artistic aspect of music production finds an echo in the challenges of AI translation. While AI relies on immense datasets, it still struggles to inject creativity into translations, particularly when navigating idioms and nuanced expressions that require an understanding beyond mere algorithmic application.
In both trip hop music and AI translation, overdoing the layering of elements can result in a jumbled mess. The intricate layering of samples in Portishead's work or the algorithmic combination of phrases by AI can both lead to confusion if not carefully handled. Too much of a good thing can obscure the essence of the output.
Interestingly, AI translations can evoke feelings of nostalgia by employing familiar language structures, mirroring how music samples can trigger emotional responses. This suggests a deeply embedded connection between language and emotion, akin to the emotional resonance of a catchy melody.
AI translation isn't just about direct translations; it also delves into historical linguistic resources. This parallels how musicians like Portishead drew upon past musical influences to create their signature sounds. AI effectively establishes a bridge between past and present linguistic landscapes.
Techniques like audio normalization, used in trip hop to enhance sound consistency, also find a parallel in how AI translation processes language data for improved coherence. This statistical approach helps smooth out inconsistencies that arise from the diversity of linguistic structures.
The rise of affordable and readily available translation technology, much like the sampling practices of the 90s, has sparked heated discussions regarding authenticity. Just as some music lovers debate the merit of sampled music versus live performances, skepticism about AI translations exists. The question remains whether machine-generated outputs can truly encapsulate the essence of human language and emotion.
Why AI Translation Gets Nostalgic Comparing Machine Learning Accuracy to Portishead's It Could Be Sweet Sampling Techniques - Machine Learning OCR Through The Lens of Beth Gibbons Vocal Processing
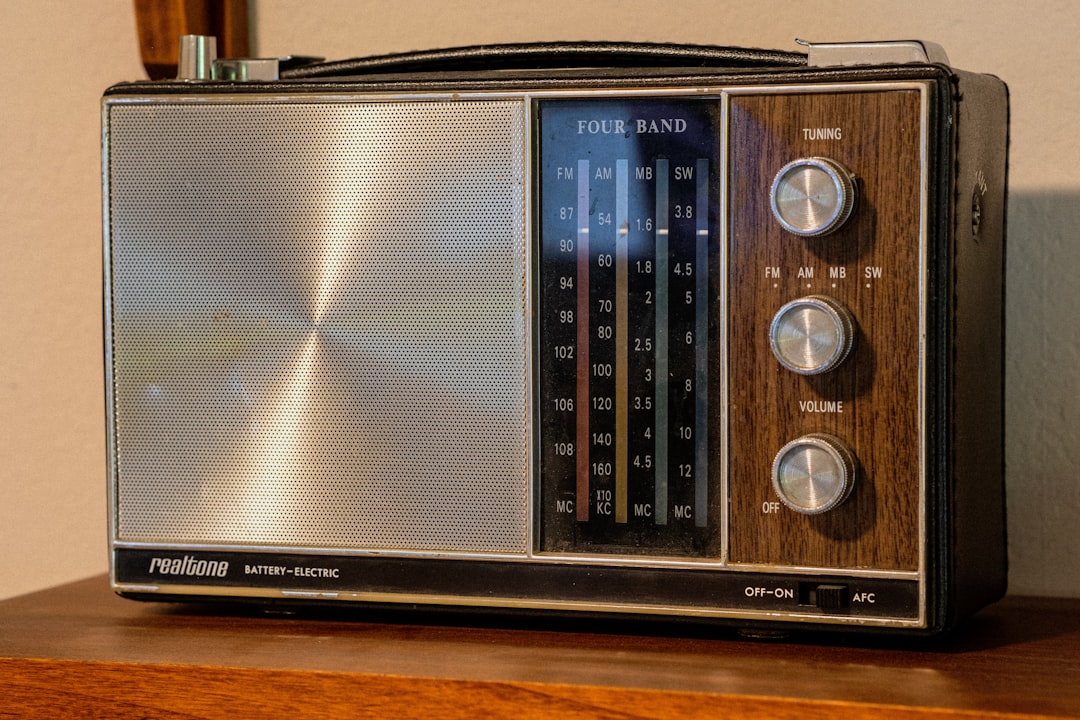
The concept of "Machine Learning OCR Through The Lens of Beth Gibbons Vocal Processing" offers a fascinating parallel between the advancement of OCR and the complex world of vocal manipulation in music. Similar to how Beth Gibbons' vocals are often processed with layers and effects to achieve a desired sound, machine learning-powered OCR systems rely on intricate algorithms to decipher diverse text inputs, including handwritten and printed documents. These systems, driven by deep learning and vast amounts of data, constantly strive for greater accuracy while simultaneously grappling with the complexities of language, akin to the challenges of perfecting vocal effects in music production. As OCR technology refines its abilities—much like the subtle adjustments made to enhance vocal performances—it unlocks new possibilities for the interpretation and translation of written text, mirroring the artistry present in Gibbons' work. This interplay highlights how technology can both disrupt and enhance our understanding of language and sound, a connection that is relevant in today's world. The process, however, is not without its challenges. Like in music where the pursuit of perfection can distort the original sound, OCR faces similar hurdles when trying to capture the inherent nuances of human language in different contexts. Ultimately, it remains a testament to the complex relationship between technology, artistry, and the ever-evolving human experience of communication.
Machine learning has significantly improved Optical Character Recognition (OCR), moving from rudimentary character recognition to sophisticated deep learning systems. These advancements, pushing past 99% accuracy, have broadened the potential for applications relying on text. This progress in OCR mirrors the evolution of audio processing techniques within the 90s music scene, particularly in how we capture and interpret information, visually and aurally.
Just like a musician samples and rearranges audio fragments, machine learning translation models often rely on past translations to build new outputs. This parallels "sampling" in music and brings up interesting questions about the originality and authenticity of translated content, much like the debates surrounding music production.
Interestingly, these AI models can face difficulties that mirror those of musicians who mishandle samples. If the context of a translation isn't properly understood, it can lead to distorted meanings, similar to how a musically disjointed piece loses its impact on the listener. This distortion can be off-putting to users, much like an awkwardly blended song.
The rapid rollout of cloud-based translation tools draws parallels to the fast-paced production methods that shaped 90s electronic music. AI-powered translation delivers nearly instant results, much like music became instantly available and accessible, albeit sometimes at the cost of deeper contextual analysis.
OCR systems are also capable of processing enormous amounts of text, in a way similar to advanced audio mixing techniques that combine numerous sound layers. However, both these fields face the challenge of managing complexity without losing clarity. Much like over-layering in a song can lead to a muddy sound, excessive data can sometimes lead to convoluted and confusing translations.
AI translation systems, like the music world, aren't free of bias. They often rely on datasets containing biased language that can inadvertently perpetuate stereotypes. This echoes the debates about cultural appropriation within music and sampling, reminding us of the need for careful consideration of the data used to train AI.
While AI can generate translations quickly, they often struggle to capture the emotional depth that humans express so naturally. This is similar to a musician who relies too heavily on sampling and might miss the emotional resonance of a specific sound, diminishing the impact of the work.
When we encounter familiar language patterns within AI-generated translations, we can experience a sense of nostalgia, similar to the emotional responses we have when we hear a familiar music sample. This suggests a fundamental connection between language and emotion, a phenomenon that both AI and music can leverage to connect with audiences.
The development of OCR from basic to sophisticated recognition techniques mirrors the meticulous approach musicians take to sound selection in their music, ensuring fidelity. This similarity emphasizes that clarity and precision are still central to effective communication.
Just as the advent of independent music production in the 90s democratized music creation, AI translation tools have made translation more accessible to everyone. However, this accessibility raises questions about authenticity and representation in the same way that sampled music prompted questions about the value of originality. The challenge in AI translation remains ensuring the output faithfully captures the essence of human communication.
Why AI Translation Gets Nostalgic Comparing Machine Learning Accuracy to Portishead's It Could Be Sweet Sampling Techniques - Neural Networks Meet Vintage Hardware Translation Accuracy in Historical Context
The evolution of AI translation reveals a fascinating interplay between modern neural networks and the limitations of past hardware, offering a unique perspective on translation accuracy across time. The shift from early 1990s machine translation, which often relied on simplistic rule-based systems like those pioneered by IBM, to the current era of Neural Machine Translation (NMT) demonstrates the remarkable leaps in computational power and linguistic analysis. NMT's deep learning approach enables it to process language in a more comprehensive and integrated manner. However, the persistent challenges in translating languages with limited data resources serve as a reminder of the limitations that still exist within this field. Similar to how vintage audio hardware once restricted the range and quality of sound, the fundamental principles and limitations of those early translation systems continue to influence today's advanced AI models. This intersection creates a nostalgic echo, highlighting the ongoing struggle to achieve truly accurate and nuanced translations within the increasingly intricate landscape of modern algorithms. This journey, where neural networks encounter the legacy of vintage hardware, underscores not just the progress achieved but also the persistent desire for translations that authentically capture the richness and context of human language in our digital world.
In the early days of machine translation, systems like those developed at IBM Research in the 90s focused on comparing text across languages without deep linguistic knowledge. This was a rudimentary approach compared to the later emergence of Neural Machine Translation (NMT) in the mid-2010s. NMT leverages the power of deep learning to approach translation as a unified learning process, using artificial neural networks to dramatically enhance the quality of machine-generated translations.
One noteworthy AI system, CUBBITT, challenges the notion that computers can't match the quality of human translators, demonstrating the significant advancements in the field. However, despite these leaps, machine translation still faces difficulties, particularly when handling languages with limited data support, also known as low-resource languages. This is a common issue for many languages spoken around the world, as popular translation tools haven't caught up with the sheer diversity of human communication—of which there are over 7,000 languages in use today.
Research like "Restoring and attributing ancient texts using deep neural networks" showcases how neural networks are being employed to assist in the study of historical texts, including damaged or fragmented documents. This suggests that the application of AI isn't just about speed or accuracy, but also about uncovering and preserving the past.
The shift from rule-based translation to neural networks signifies a major evolution in how machine translation is performed. This transition has led to end-to-end NMT becoming the standard approach. It is important to note that alongside these exciting advances, there are inherent challenges and ethical considerations that need to be acknowledged and addressed.
AI-powered translation technologies, encompassing NMT and large pre-trained language models, have revolutionized how machine translation operates. These systems, while remarkably efficient, raise complex questions about accuracy, bias, and the potential impact on the preservation of linguistic diversity. The journey of AI translation is constantly evolving, and it remains a space of innovation and exploration.
Why AI Translation Gets Nostalgic Comparing Machine Learning Accuracy to Portishead's It Could Be Sweet Sampling Techniques - Audio Latency vs Translation Speed Learning From Bristol Sound Engineering
The concept of "Audio Latency vs Translation Speed Learning From Bristol Sound Engineering" draws a comparison between the technical aspects of audio production and the development of AI translation. Much like audio latency in music can affect the clarity and timing of sound, AI translation, especially in its pursuit of speed, can sometimes sacrifice the subtleties and depth of meaning in the translated text. Studying the approach Bristol's sound engineers took to address latency, prioritizing audio fidelity, offers valuable insights for the field of AI translation. This suggests a pathway towards developing models that can deliver fast results while still retaining a high degree of accuracy and understanding of the context. AI translation technologies, in a way, act as a digital mirror to the meticulous practices of audio engineers, showcasing a common aspiration for clarity and precision within their respective fields. However, both fields also grapple with the complications that arise with the focus on speed, and the need for balance between efficiency and quality remains. The relationship between swift translation and authentic communication highlights the enduring value of quality, even in the face of swift technological advancement.
The rapid advancements in AI translation, particularly in the speed at which it processes text, echo the fast sampling rates found in 90s audio engineering. Modern Optical Character Recognition (OCR) systems can process thousands of characters per second, showcasing the power of machine learning algorithms. However, similar to the way audio latency can ruin a musical performance, any delay in AI translation can disrupt the flow of communication. Even milliseconds can be a problem.
Much like the success of a musical piece depends on the quality of its sampled elements, the performance of AI translations hinges on the comprehensiveness and quality of training datasets. If the data used to train an AI translation model is limited or biased, the translation's accuracy and nuance are likely to suffer. It can result in an output that feels less human-like and more artificial.
We can think of neural networks as comparable to analog audio band-pass filters, capable of isolating specific features from a large dataset. But similar to how a filter might remove certain frequencies or nuances in a song, neural networks may also miss subtle cues that are essential for effective translation.
Just as sound sampling can imbue music with a certain emotional depth, AI translation can trigger feelings of nostalgia by generating familiar phrases. But in both cases, a lack of contextual awareness can result in a disjointed or inaccurate outcome. Missing the context can produce translations that fail to accurately reflect the intended meaning.
There's also an echo in how both fields are susceptible to bias. The way audio samples can inadvertently perpetuate cultural stereotypes, the data used in training AI translation models can similarly contain and propagate existing biases. This highlights the ethical considerations inherent in both music production and AI technology.
The evolution of OCR is a striking example of parallel development, much like audio mixing. Both went from rudimentary techniques to highly sophisticated systems requiring careful balancing to avoid sacrificing clarity or fidelity.
Furthermore, limitations in early translation systems due to hardware constraints are like the limits of vintage audio gear which couldn't reproduce certain frequencies. This provides valuable context to the relentless pursuit of ever-more accurate AI translation.
It's clear that modern AI translation builds on the foundation established by older translation technologies, analogous to how current musical trends often draw inspiration from earlier genres. This ongoing interaction between older and newer methods points to a continuous process of refinement in both fields. It's never really a clean break.
This demonstrates how technology progresses, both in music and AI translation, through a blend of legacy techniques and new advancements. Understanding these parallels between audio engineering and AI is not just interesting—it provides unique insights into the challenges and opportunities that lie ahead.
More Posts from aitranslations.io: