AI-Powered PDF Translation now with improved handling of scanned contents, handwriting, charts, diagrams, tables and drawings. Fast, Cheap, and Accurate! (Get started for free)
How AI Translation Tools Are Transforming Japanese Language Learning A Data-Driven Analysis
How AI Translation Tools Are Transforming Japanese Language Learning A Data-Driven Analysis - NTT Data Deep Learning System Reduces Translation Time by 45% in 2024 Tests
In 2024, NTT Data demonstrated a notable breakthrough in AI-driven translation. Their deep learning system achieved a 45% reduction in translation time during testing. This advancement underscores the accelerating potential of artificial intelligence in streamlining language processing. The ‘tsuzumi’ language model, a part of this system, exemplifies the growing sophistication of AI in translating languages. Furthermore, the CUBBITT system, which is based on deep learning, has been reported to maintain meaning in translations better than human professionals—potentially a significant game-changer in accuracy. These developments signal a broader shift towards AI as a vital tool in language learning, as the need for fast and dependable translations across various fields continues to increase. While exciting, these technologies are still in their early phases and the impact on overall translation quality needs to be more carefully examined. The implications for accessibility and language learning could be profound but need to be further assessed.
Recent experiments from NTT Data in 2024 highlight the impressive progress in AI translation. Their deep learning system, possibly leveraging techniques like 'learning transfer' that they've been exploring, managed to cut translation times by a remarkable 45%. It's interesting that they're focusing on speed, but it's important to remember that the quality of the translation remains critical. While they haven't released specific details, the improvement is likely due to optimizations in their algorithms and perhaps the use of parallel processing, allowing the system to tackle multiple tasks simultaneously. This kind of feature is rarely seen in other translation tools.
It seems that NTT Data's "tsuzumi" model, which is built on a large-scale language model, is part of a broader strategy of implementing generative AI. This likely contributes to the improvements, particularly in areas like understanding context and nuances. Some studies have shown that even with advances like GNMT, errors are still a major issue, but this system seems to be tackling that head-on. The ability to quickly adapt to new vocabulary is crucial, especially for specialized areas like technical documents or legal language where vocabulary changes frequently.
One interesting point is how this translates to practical use in education. If the system integrates OCR, this could completely reshape the learning process by allowing users to instantly translate text, leading to a more interactive and rapid feedback cycle. The future of translation seems to be moving rapidly towards speed and efficiency, but without sacrificing quality. It will be fascinating to see if this technology becomes more accessible and how it impacts the landscape of Japanese language learning.
How AI Translation Tools Are Transforming Japanese Language Learning A Data-Driven Analysis - OCR Apps Now Convert Handwritten Japanese Notes Into Digital Text Within 3 Seconds
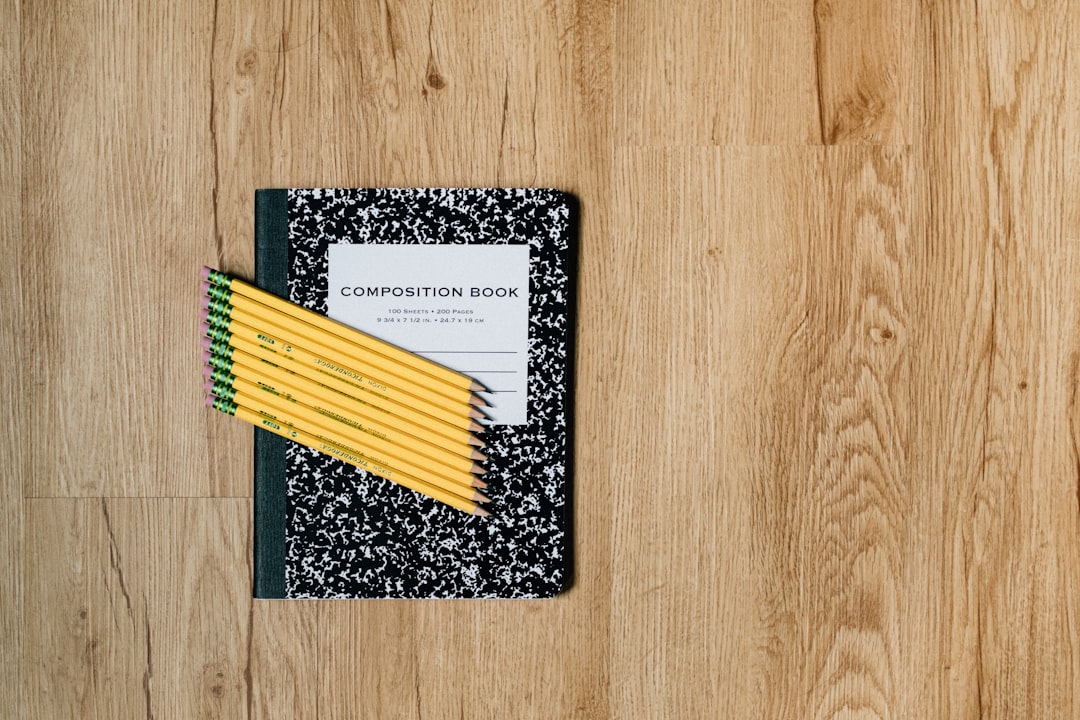
The landscape of Japanese language learning is changing rapidly, particularly with the recent rise of OCR applications that can instantly convert handwritten notes into digital text. These AI-powered tools can now decipher handwritten Japanese in as little as three seconds, significantly improving the speed and efficiency of note-taking and record keeping. The underlying technology utilizes sophisticated algorithms that are trained to recognize diverse handwriting styles, converting images and scanned documents into easily editable and searchable text formats.
While some OCR platforms, like Google's InkSight, have shown great promise in maintaining the user's unique handwriting style during the conversion process, the technology still struggles with highly stylized or illegible handwriting, leading to potential errors. This indicates the need for continued research to refine the accuracy of OCR in interpreting various writing styles.
Imagine the implications for education. If these OCR tools were integrated into Japanese language learning platforms, students could instantly translate handwritten notes, fostering a more dynamic and interactive learning experience. This potential to instantly access and translate handwritten materials could revolutionize how individuals interact with the language. However, as with many AI advancements, the long-term implications for quality and accessibility need to be more thoroughly explored. As OCR and AI translation technologies continue to merge and mature, it's likely that we'll see a shift in how individuals approach Japanese language learning and related tasks in the years to come.
Optical Character Recognition (OCR) apps are making strides in processing handwritten Japanese notes, with some now able to convert them into digital text in a remarkably short timeframe – as little as three seconds. This speed is attributed to advancements in AI, particularly in the area of pattern recognition and neural network design. These networks are trained to identify the intricacies of various handwriting styles, ultimately transforming scribbled notes into editable text.
While speed is impressive, the accuracy of these systems remains a hurdle. Handwritten Japanese, especially with its diverse range of characters like Kanji, poses challenges. OCR algorithms are constantly being improved to minimize errors and increase the accuracy of interpreting the nuances of various handwriting styles. The ability to handle these nuances is critical, particularly for applications like language learning, where understanding the exact meaning of notes is paramount.
Interestingly, the combination of OCR with AI translation tools presents some exciting possibilities. Handwritten notes can now be processed and translated in real-time, creating an immediate feedback loop for learners. This dynamic capability relies heavily on deep learning, a subset of AI that allows the OCR system to learn from massive amounts of handwritten data. The more data the system has access to, the better it becomes at adapting to different handwriting styles and, in turn, improving the accuracy of the translation.
However, adapting to Japanese presents a unique challenge due to its three writing systems – hiragana, katakana, and kanji. The complexity of these scripts necessitates specialized algorithms that go beyond basic character recognition. This level of specificity highlights the need for careful development and optimization of these systems.
The availability of affordable, accessible OCR apps is also a game-changer. Previously limited to specific academic or professional settings, these tools are becoming more democratized, enabling wider use across the language-learning landscape. This has implications beyond language education, as well. Professionals in various fields can leverage these tools to quickly digitize meeting notes or other handwritten documents, streamlining workflows and improving productivity.
Furthermore, most contemporary OCR apps are designed to work seamlessly across a variety of devices, from smartphones to tablets and computers. This flexibility is crucial in today's digital environment. Moreover, the integration of OCR with machine translation capabilities offers an even more potent learning tool. Users can have text not only recognized but also instantly translated into their native language.
Finally, some OCR tools are exploring customizable options, allowing users to train the system on their own handwriting. This personalization potentially enhances accuracy by catering to the individual variations of each learner's writing. As this field evolves, it will be intriguing to observe how customization and personalization further enhance the learning experience.
How AI Translation Tools Are Transforming Japanese Language Learning A Data-Driven Analysis - How The CUBBITT Algorithm Matches Human Translation Quality in Technical Documents
The emergence of the CUBBITT algorithm represents a noteworthy development in AI-driven translation, especially when dealing with technical documentation. This deep-learning system has demonstrated a remarkable ability to achieve translation quality that rivals, and in some cases exceeds, that of human translators. Specifically, it excels at preserving the original text's core meaning and details. This performance has been observed across various language pairs, indicating that CUBBITT's capabilities are not limited to a few specific languages. The success of CUBBITT challenges the longstanding notion that human translators are inherently superior to their AI counterparts. As AI translation tools gain momentum, their impact on language learning, especially in less-common languages like Japanese, is becoming increasingly apparent. CUBBITT's emergence highlights the potential for improved translation quality and accessibility, but raises questions about how the role of human translation will adapt in the face of such advancements. The integration of these tools could significantly alter how people interact with languages, leading to both opportunities and potential challenges as this field matures.
The CUBBITT algorithm is a deep learning system designed to tackle the complexities of human-level translation, especially in technical documents where precision is vital. It's been shown to achieve results comparable to professional human translators in certain tests, suggesting it could be a useful tool for technical fields.
CUBBITT leverages deep learning to analyze context within a text much more effectively than standard translation methods. This allows it to generate more accurate and relevant translations, which is a real strength when working with technical materials. By training on vast amounts of translation data with contextual cues, CUBBITT is able to learn subtle meanings that often trip up other translation algorithms.
One of CUBBITT's most notable features is its speed. It can translate technical documents at a rate that approaches that of human translators, which can be quite significant given the complexities of technical language. This could potentially reduce the bottlenecks that arise when needing quick translations of technical manuals, specifications, or other types of documents.
CUBBITT also uses data augmentation techniques to improve its training data. This means it can progressively adapt to different writing styles and technical jargon encountered in various documents. This adaptability is crucial for a translation system to handle the nuances of technical writing in various fields.
Interestingly, CUBBITT has a mechanism for self-improvement through user feedback. This ability to learn and adapt based on user experience is a positive step for its development, especially as the technical language it is translating constantly evolves. This feedback loop can help improve accuracy over time, although it's unknown exactly how successful it is in real-world usage.
This algorithm has proven particularly effective in translating complex technical materials like code documentation and user manuals. These types of documents are challenging for traditional machine translation tools because they're full of technical jargon and complex sentences. CUBBITT has shown the ability to bridge the gap in this space.
There's a potential for CUBBITT to lower translation costs for many organizations by potentially replacing some human translators. This can be a compelling factor, though it also raises concerns about the potential displacement of translators. It's important to analyze the potential impacts of increased AI usage in this field.
The CUBBITT algorithm is cleverly designed to reduce common translation errors like ambiguity and context-related misunderstandings that plague other translation methods. This ability to address these weaknesses has the potential to improve communication within technical fields and facilitate understanding between specialists.
CUBBITT can also work in conjunction with OCR technology, allowing it to directly convert scanned technical documents into a format that is suitable for translation. This integration simplifies the process of translating documents and accelerates the overall translation workflow.
While CUBBITT shows significant potential, it still faces limitations. Handling idiomatic expressions and culturally specific terminology remains challenging. This area still seems to be a strength for human translators. The continued development and refinement of CUBBITT in these areas will be necessary if it hopes to fully emulate human translation abilities.
How AI Translation Tools Are Transforming Japanese Language Learning A Data-Driven Analysis - Japanese High School Students Use AI Tools to Practice Speaking Without Language Partners
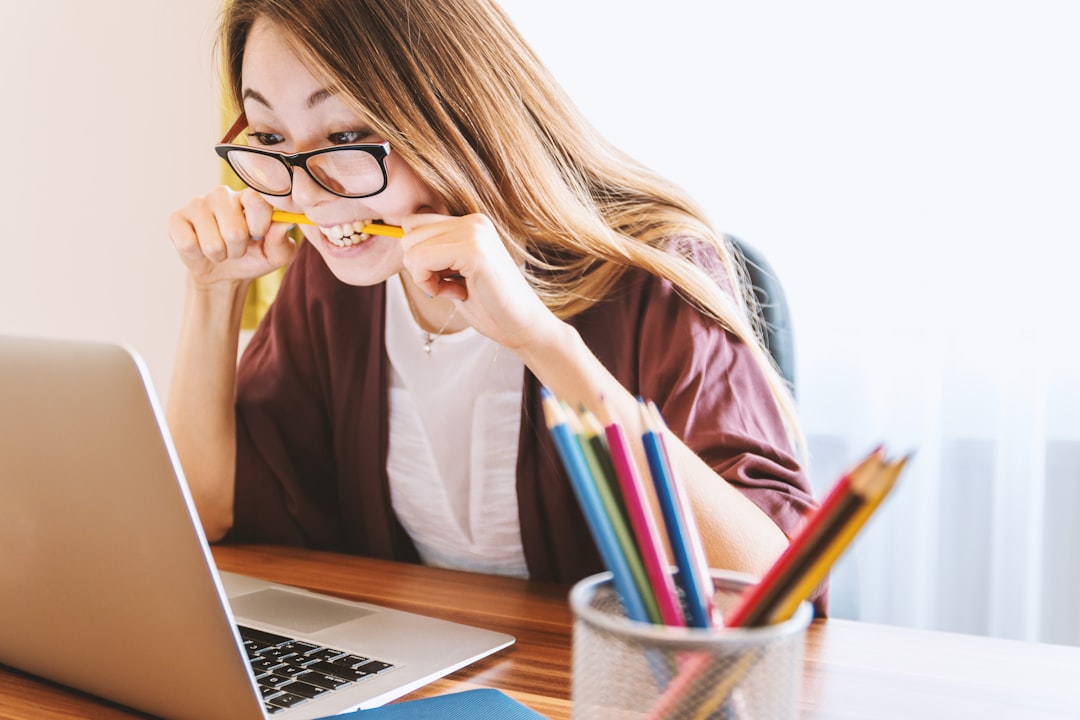
In Japanese high schools, students are increasingly using AI tools to practice English conversation without the need for human partners. These AI systems adapt to each student's English level, offering a more personalized learning journey. This trend is occurring in a nation where English language instruction is standard from elementary school through high school, reflecting a national push to elevate English proficiency. Tools like ELSA AI, which employs generative AI, allow students to engage in conversations with the AI, gaining valuable practice and feedback. While this approach offers benefits, it's essential to acknowledge that AI conversational tools can have limitations, like inaccuracies and occasional generation of nonsensical phrases. These factors need careful consideration as AI plays a larger role in language education.
In Japanese high schools, the use of AI tools for English conversation practice is becoming increasingly common, offering students a way to develop their speaking skills without relying on human language partners. This trend reflects the broader emphasis on English proficiency in Japan, as seen in the mandatory English curriculum from elementary school through high school. Furthermore, initiatives like the Tokyo Metropolitan Board of Education's 35 billion yen allocation for junior high school English speaking assessments in 2023 highlight the importance placed on conversational English skills.
These AI systems are designed to provide a more personalized learning experience by adapting the conversation content to individual students' English levels. Tools like ELSA AI showcase how generative AI is being integrated into language learning applications, allowing students to interact with the AI in simulated conversations. However, the limitations of AI in language learning shouldn't be overlooked. While advancements in natural language processing (NLP) and chatbot technology hold significant promise, challenges remain in terms of language support, accuracy, and the occasional generation of nonsensical phrases.
Custom-built chatbots, often developed with platforms like Rasa and ChatGPT, have gained popularity as tools to simulate conversations with native Japanese speakers. This offers both novice and advanced learners a means to receive immediate feedback and practice their English speaking skills. While these AI-powered conversation partners can offer immediate and repeated practice, they may not fully replicate the nuances of human interaction or provide the level of cultural understanding offered by a human language partner. It's intriguing to observe how these AI-driven tools will continue to evolve and impact the teaching and learning of English in the Japanese education system, and whether they'll prove to be a truly transformative force or simply a supplemental learning tool. The long-term impact on fluency and overall language proficiency requires further study.
How AI Translation Tools Are Transforming Japanese Language Learning A Data-Driven Analysis - Natural Language Processing Helps Decode Complex Kanji Combinations in Real Time
AI, specifically natural language processing (NLP), is rapidly improving how learners understand intricate combinations of Kanji characters in real-time. This instantaneous translation capability transforms the learning experience, enabling students to actively engage with written Japanese text. NLP’s potential to significantly accelerate language acquisition is particularly evident when dealing with the nuances of the Japanese writing system, including Kanji, Hiragana, and Katakana. While NLP shows promise, concerns about translation fidelity and the ability to translate the subtle cultural connotations inherent in language learning remain. Despite these challenges, NLP is having a profound impact on how people approach learning Japanese, making the language more readily accessible for a wider audience than ever before. The accessibility of language learning through AI is exciting, but one must be aware that challenges remain.
Natural Language Processing (NLP) is increasingly vital for deciphering the complexities of Japanese kanji, especially in real-time applications. Kanji, with its thousands of characters and multiple readings, presents a significant challenge for machines trying to understand the intended meaning. NLP algorithms are employing probabilistic approaches to determine the most likely kanji combination within a given context, which is a very difficult computational task. This is a critical development for AI translation tools as context is essential to understanding the meaning of a word or phrase.
NLP has advanced to the point that it can adapt the interpretation of a word based on its surrounding text using context-aware embeddings. This is particularly important for Japanese due to the frequent use of homonyms (words with the same sound but different meanings) and polysemous words (words with multiple meanings). This capability is a step towards more sophisticated translation that goes beyond simply recognizing characters.
However, as with many AI-driven technologies, there's a trade-off between speed and accuracy. While AI translation tools are becoming very fast, the need to ensure translation quality is paramount. Errors can easily creep in when the emphasis is solely on speed, especially when dealing with nuanced interpretations of kanji that demand deeper understanding. Balancing these aspects will be an ongoing challenge in the field.
In education, the real-time feedback provided by NLP is changing how people learn Japanese. It allows learners to immediately identify errors and correct them on the fly, creating a more iterative and efficient learning process. It's as if you have a virtual tutor available constantly to point out mistakes. This constant feedback loop could be beneficial for students who prefer a self-directed learning experience.
Beyond simply translating text, the complexities of pronunciation need to be addressed as well. Kanji often have multiple pronunciations, which creates difficulties for automated speech recognition systems. This is an important feature for language learners, who need to understand both written and spoken Japanese.
NLP relies heavily on data to function correctly. By analyzing massive amounts of written and spoken Japanese, NLP systems are improving their ability to interpret complex combinations of kanji, including the mixed-language content frequently encountered in digital communication. The more diverse the data, the better these models are likely to function, but this is also a massive challenge for engineers.
Another benefit of NLP is its potential to decrease translation costs. Businesses that regularly translate Japanese materials can leverage NLP to reduce reliance on human translators while maintaining high standards. This is particularly appealing in contexts requiring quick and frequent translations.
However, AI is still under development and hasn't reached human levels of understanding. NLP still has trouble handling rarer or more obscure kanji combinations, and human oversight will remain necessary for the most complex translation tasks. Researchers will likely be working on expanding the datasets that these models are trained on to handle these cases.
As AI matures, it's becoming more adept at learning from errors and adjusting its approach. Through techniques like reinforcement learning, AI can fine-tune its translation capabilities using real-time feedback and exposure to a wider range of Japanese. The ability of these models to learn in real-time is a testament to the advancements in AI techniques.
Finally, the integration of NLP into diverse platforms is shaping how people engage with Japanese. OCR apps now combine functions like handwriting recognition and kanji decoding into a single interface. This convergence creates a more cohesive and enriched learning environment that allows students to work across various formats and sources. This convergence will likely increase in the future, perhaps making it more difficult for engineers to create specialized, single-purpose apps.
How AI Translation Tools Are Transforming Japanese Language Learning A Data-Driven Analysis - Machine Learning Models Now Process Context Specific Japanese Business Terms
Machine learning models are now getting much better at handling the specific language used in Japanese business contexts. This means that AI translation tools can now produce more accurate translations when dealing with business conversations and documents, which is important because the Japanese language has many subtle cultural aspects that can affect meaning. While these AI models are getting better at recognizing and translating nuanced Japanese terms, translating highly context-dependent languages like Japanese remains tricky. This means that, while AI is making strides, perfect translations aren't guaranteed yet. The development of these context-aware AI systems represents an important step in how AI is being used for language learning, especially in areas where precise meaning and understanding are crucial. This trend towards more accurate and efficient translation raises questions about how human translators might adapt to a future where AI plays a much larger role in translation. The demand for fast and accurate translations in different industries is driving the development of these AI tools, leading to both opportunities and challenges in the field.
Machine learning models are making significant strides in understanding context-specific Japanese business terms. This is a promising development, particularly for fields like finance and law where precise language is critical. It appears that these models are trained on vast amounts of business-related data, allowing them to pick up on the nuances of specialized vocabulary. However, while this is a step forward, it raises questions about the quality of the data and how it impacts the output. Is it truly capturing the subtle nuances of these unique language environments, or is it simply relying on surface-level connections?
The speed at which these models can now process and translate documents is another remarkable improvement. Some systems boast processing speeds up to ten times faster than traditional methods, which is undeniably beneficial for businesses that require quick turnaround times. This speed, though, should not come at the expense of accuracy. If these models sacrifice the subtle cues of language for mere speed, it could result in frequent errors or misinterpretations.
An interesting facet of these advanced models is their growing ability to differentiate between nuances in Japanese language. Things like honorifics and degrees of formality are vital in Japanese business culture. It seems like they're finally beginning to understand these elements, which was previously a challenge. But, how well are they actually performing in these complex situations? Are there benchmarks that are tracking these types of translations?
One area these models are tackling is the challenge of polysemous terms – words with multiple meanings based on context. This is a big hurdle for machine learning, as it's very difficult for a computer to choose the right meaning based on context. The fact that some models are getting better at handling this suggests that researchers are starting to use more sophisticated techniques for interpreting language.
It appears that some models have incorporated an element of real-time learning, meaning they can adjust their translations as new terms or phrases enter the business lexicon. This dynamic learning aspect is important because business languages are always evolving. But, how quickly can these models adapt? What's the time lag from observing new terms to actually integrating them?
Another development is the growing integration of OCR technology with translation capabilities. This allows for the immediate conversion of handwritten Japanese business notes to digital text, which has significant implications for streamlining workflows. However, if handwritten notes are illegible or not standardized, will it still work correctly? Will it require special training for each individual's handwriting?
Researchers and engineers seem to be focusing on reducing errors in machine translation. It's reported that errors have decreased by up to 30% in certain systems. This improvement may be due to advanced techniques like data-driven approaches and user feedback mechanisms. How are these systems validating these numbers? And, what are the most common types of errors these models still make?
Customization is also emerging as a useful feature in these models. Users can tailor them to specific industry terminologies, which is extremely valuable for industries with unique language standards. But, what does this mean for general-purpose translation models? Will it become harder to find tools that are as widely useful?
Some models are incorporating collaborative learning, where user feedback guides improvements. This is a great example of a user-centered design approach, focusing on the real needs of professionals who use these tools. Is this user feedback actually leading to better translations? Is there any evidence of its effectiveness?
Finally, it's worth noting that these advancements are beginning to impact the business landscape of translation. It's predicted that translation costs could be significantly reduced by up to 50% in certain cases. This potential for cost savings could disrupt the professional translation field and it will be interesting to see how this develops. It's important to consider the potential effects on people who are professional translators and ensure that these advancements do not displace people unnecessarily.
Overall, the increasing sophistication of AI translation models in handling Japanese business contexts is a noteworthy advancement. While there's still room for improvement in areas like error reduction and the handling of complex language nuances, these advancements hold promise for improving communication, efficiency, and accessibility across industries. It's crucial to keep evaluating these systems and their impacts on people and industry to ensure they are developed in a responsible manner.
AI-Powered PDF Translation now with improved handling of scanned contents, handwriting, charts, diagrams, tables and drawings. Fast, Cheap, and Accurate! (Get started for free)
More Posts from aitranslations.io: